Statistics in Health Sciences, 2023/2024 PDF
Document Details
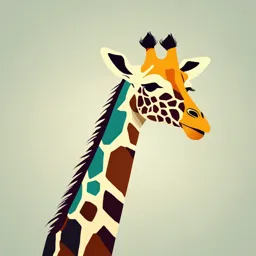
Uploaded by PraiseworthyHammeredDulcimer
Universitat Autònoma de Barcelona
2023
Jose Barrera
Tags
Summary
This document is lecture notes on dealing with missing data in statistical analyses, particularly in epidemiological studies and observational studies. The lecture notes discusses the different types of missing data (MCAR, MAR, and MNAR) and methods for dealing with it.
Full Transcript
B.Sc. Degree in Applied Statistics Statistics in Health Sciences 5. Dealing with missing data Jose Barreraab [email protected] https://sites.google.com/view/josebarrera a ISGlobal Barcelona Institute for Global Health - Campus MAR b Department of Mathematics (UAB) This work is licensed under...
B.Sc. Degree in Applied Statistics Statistics in Health Sciences 5. Dealing with missing data Jose Barreraab [email protected] https://sites.google.com/view/josebarrera a ISGlobal Barcelona Institute for Global Health - Campus MAR b Department of Mathematics (UAB) This work is licensed under a Creative Commons “Attribution-NonCommercial-ShareAlike 4.0 International” license. Statistics in Health Sciences 1 Introduction 2 Types of missing data 3 Dealing with missing data Jose Barrera (ISGlobal & UAB) Statistics in Health Sciences, 2023/2024 2 / 33 Missing data Introduction • Missing data is a common problem in statistical analyses that involve real data sets. • Specifically, this is the case in most of epidemiological studies that are related to non controlled, observational studies. • Next, we classify different types of missing data as well as introduce to how to deal with them. Jose Barrera (ISGlobal & UAB) Statistics in Health Sciences, 2023/2024 3 / 33 Missing data Types of missing data patterns Missing data can be classified according to three different patterns (Rubin [1] ): • MCAR (Missing completely at random) • MAR (Missing at random) • MNAR (Missing not at random) Jose Barrera (ISGlobal & UAB) Statistics in Health Sciences, 2023/2024 4 / 33 Types of missing data: MCAR MCAR (Missing completely at random) • It is the case in which the probability of an observation Xi being missing does not depend on the value of Xi , nor on any of the remaining variables in the data set. • If the probability of missing Xi depends on the probability of missing the value in other variable in the same individual, Yi , this does not affect the assumption of MCAR. Examples • A participant in the study was not able to go to the interview for the health questionnaire because them missed the train. • The scale used to weight individuals has a constant probability of malfunction. Consequences (by comparison with the case of complete data) • Sample size reduction ! statistical power reduction. • Parameter estimates are unbiased. Jose Barrera (ISGlobal & UAB) Statistics in Health Sciences, 2023/2024 5 / 33 Types of missing data: MAR MAR (Missing at random) • It is not MCAR but the probability of missing Xi does not depend on the value of Xi , after stratifying by other variables in the data set potentially related to the probability of missing Xi . • In other words, the propensity of missingness depends on observed data, not missing data. Examples • Depressed people could be less likely to report their physical activity (PA) and, at the same time, be characterized by a low PA. If among depressed people the probability of missing physical activity information was unrelated to its value, missing data would be MAR and depression could be used as a predictor of PA levels. • A survey respondent choosing not to answer a question on income because they believe the privacy of personal information. The missing value for income can be predicted by looking at the answers for the personal information question. Consequences (by comparison with the case of complete data) • Sample size reduction ! statistical power reduction. • Parameter estimates could be biased (there are methods to deal with this problem). Jose Barrera (ISGlobal & UAB) Statistics in Health Sciences, 2023/2024 6 / 33 Types of missing data: MNAR MNAR (Missing not at random) • The missing is not random, it correlates with unobservable characteristics unknown to a researcher (i.e. to variables not present in the data set). • Particular case: the probability of missing Xi depends on the value of Xi itself, and X is unrelated to other observed variables. Examples • When assessing the mental health status X via a health questionnaire among a random sample of the population of interest, if individuals with poor mental health (e.g. those with low scores in X ) are less likely to report their health status than individuals with good mental (e.g. those with high scores in X ), then data are MNAR. • Drugs consumers could be more likely to do not answer about drugs consumption. Consequences (by comparison with the case of complete data) • Sample size reduction ! statistical power reduction. • Parameter estimates could be biased (no solution). Jose Barrera (ISGlobal & UAB) Statistics in Health Sciences, 2023/2024 7 / 33 Dealing with missing data Different approaches There is a number of methods for dealing with missing data... • Complete cases analysis • Mean/median/mode substitution • Regression imputation • Inverse probability weighting imputation • Multiple imputation Jose Barrera (ISGlobal & UAB) Statistics in Health Sciences, 2023/2024 8 / 33 Complete cases analysis Complete cases analysis • All rows of the dataset that are not complete cases regarding the subset of variables of interest are excluded of the analysis.a • Estimates are unbiased only under the assumption of data MCAR. At also applies if the variable with missing data is the response variable of interest. • The sample size reduction implies a decrease in the statistical power ! the probability of false negative increases. • It is usually appropriate if the percentage of complete cases is very high and we assume MCAR. • Alternatives to this method include substitution methods... a This is the default option in R. Jose Barrera (ISGlobal & UAB) Statistics in Health Sciences, 2023/2024 9 / 33 Mean/median/mode substitution Mean/median/mode substitution • Each missing datum is replaced by the sample mean, median or mode of the variable computed using available data. • This method does not add additional information because of the sample mean, median or mode remains unaltered while the variance is underestimated because the sample size is enlarged but no extra variability is added. • In addition, this method could provide unrealistic imputed values (e.g. a 2-year-old baby with 56 kg weight). Jose Barrera (ISGlobal & UAB) Statistics in Health Sciences, 2023/2024 10 / 33 Mean/median/mode substitution Exercise Our aim is to estimate the mean and the variance of glucose levels in blood (X ) in a given population. To do that, we select a random sample S = {x1 , x2 , x3 , . . . , xn 1 , xn } of size n. Then, we notice that all data in the sample have been correctly collected except in the case of xn , which is missing. We denote: • X̂c and V̂c the mean and variance estimates of glucose levels, respectively, using a complete cases analysis (i.e. using Sc ); • X̂m and V̂m the mean and variance estimates of glucose levels, respectively, using a mean substitution analysis. Prove that both methods provide the same mean estimate (i.e. X̂m = X̂c ) while the variance is underestimated when using the mean substitution method (i.e. V̂m < V̂c ). Jose Barrera (ISGlobal & UAB) Statistics in Health Sciences, 2023/2024 11 / 33 Regression imputation Regression imputation • If Xi is missing, its value is rebuilt with the prediction of its mean value resulted of fitting a linear regression model in which the outcome is the variable X and the predictors are the remaining variables in the data set, using all available data. • This method is more sophisticated, realistic than the mean substitution because imputed values within each variable are not all equal due to the values of the remaining variables. • However, the reduction of variance problem persists because, despite no additional information has been used to rebuild the datum, the sample size has been increased. Jose Barrera (ISGlobal & UAB) Statistics in Health Sciences, 2023/2024 12 / 33 Inverse probability weighting (IPW) Inverse probability weighting • Inverse probability weighting (IPW) is a statistical technique to estimate parameters in a population different from that in which the data was collected, which is not a rare situation in the context of observational studies (due, for instance, to cost, time, or ethical issues). • Essentially, each observation is weighted by the inverse of the probability of such an observation being sampled. Hence, the lower the probability of being sampled, the higher the weight of the observation in the analysis. • IPW can be also applied to deal with missing data. Essentially, IPW can be used to inflate the weight for subjects who are under-represented due to a large degree of missing data. For further details: Seaman and White [2] . Jose Barrera (ISGlobal & UAB) Statistics in Health Sciences, 2023/2024 13 / 33 Multiple imputation (MI) Multiple imputation (MI) MI (see Rubin [3] ) is a proper approach to impute realistic values to the missing data and propagate the uncertainty due to the missing data to the results of the analysis of interest. Missing data assumptions • MCAR is desirable but typically unrealistic. • MI techniques assume MAR. • Hence, we make the assumption that missing values can be replaced by predictions derived by the observed data. • This is a fundamental assumption, otherwise we wouldn’t be able to predict plausible values of missing data points from the observed data. – Wade, relax. . . Jose said “multiple imputation”, not “multiple amputation”. � @overdispersion Jose Barrera (ISGlobal & UAB) Statistics in Health Sciences, 2023/2024 14 / 33 Multiple imputation: MICE algorithm Multiple imputation by chained equations (MICE) algorithm 1 2 3 Let {Y1 , Y2 , . . . , Ym 1 , Ym } be the subset of all variables of the data set with at least one missing value, and let {X1 , X2 , . . . , Xl 1 , Xl } be the subset of all variables of the data set that are complete; For every missing datum in {Y1 , Y2 , . . . , Ym substitution); For i = 1, 2, . . . , m 1 2 3 1 , Ym }, perform a simple imputation (e.g. mean/median/mode 1, m, perform the following cycle: Set back to missing all imputed values for Yi in step 2; Using only complete cases, perform a regression model with Yi as the dependent variable and all or some of the other variables as the independent variables (predictors). The regression model used for Yi works under the same assumptions it makes when performing a typical regression model analysis (e.g. linear, logistic, or Poison regression). The predictors are set by the analyst and can be different for each Yi ; Replace the missing values for Yi with predictions (imputations) from the fitted regression model. At the end of the cycle all of the missing values have been replaced with predictions from regressions that reflect the relationships observed in the data. 4 Repeat step 3 until the distribution of the parameters governing the imputations (e.g. the coefficients in the regression models) are stable. Then imputation models are retained. 5 For each i, use the final model to impute M values for each originally missing value of Yi . The M imputations are different because models are used probabilistically (instead of predicting just the mean of Yi ). Jose Barrera (ISGlobal & UAB) Statistics in Health Sciences, 2023/2024 15 / 33 Multiple imputation in regression analysis Multiple imputation in regression analysis A typical application of MI is in the context of linear regression analysis, which is performed as follows: 1 2 3 For each imputed data set j = 1, 2, . . . , M, the parameter of interest, ✓ (e.g. a coefficient of \j ). interest in the regression model) is estimated to get ✓ˆj , as well as its variance, Var(✓ P M ˆ The final estimate of ✓ is the mean of the M estimates, ✓ˆMI = 1 ✓j . M \ =V bW + The final estimate of the variance of ✓ is Var(✓) MI bW = • V bB = • V Comments M+1 b VB , M j=1 where: 1 PM \ j=1 Var(✓j ) is the mean of the M estimates of the variance of ✓ or within imputation variance M 1 PM ˆ ✓ˆMI )2 is the variance of the estimates of ✓ or between imputation variance j=1 (✓j M 1 • V̂B captures the uncertainty of the imputations and inflates the error of the estimate accordingly. • In R, multiple imputation analysis can be performed using the mice package. • For further details: Klebanoff and Cole [4] , Sterne et al. [5] . Detailed explanation: Azur et al. [6] . Jose Barrera (ISGlobal & UAB) Statistics in Health Sciences, 2023/2024 16 / 33