Linear Regression Analysis with MI in R: Illustrative Example PDF
Document Details
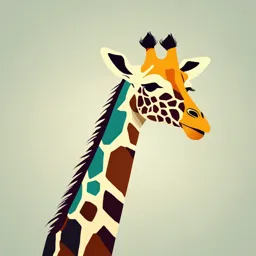
Uploaded by PraiseworthyHammeredDulcimer
null
null
Jose Barrera
Tags
Summary
This document provides an illustrative example of linear regression analysis with multiple imputation (MI) in R, focusing on estimating the mean testicular volume (tv) as a function of age (age) and region (reg), among boys 5 years or older. It meticulously explains the imputation process, customizing the methods, and analyzes missing data efficiently. The document covers statistical methods relevant to health sciences.
Full Transcript
Linear regression analysis with MI in R: illustrative example Data We will work with a random sample (10%) of 748 Dutch boys from the cross-sectional data used to construct the Dutch growth (height, weight, head circumference and puberty) references 1997.a Variables are: • age: Decimal age (0-21 yea...
Linear regression analysis with MI in R: illustrative example Data We will work with a random sample (10%) of 748 Dutch boys from the cross-sectional data used to construct the Dutch growth (height, weight, head circumference and puberty) references 1997.a Variables are: • age: Decimal age (0-21 years) • hgt: Height (cm) • wgt: Weight (kg) • bmi: Body mass index (kg/m2 ) • hc: Head circumference (cm) • gen: Genital Tanner stage (G1-G5) (https://en.wikipedia.org/wiki/Tanner_scale) • phb: Pubic hair (Tanner P1-P6) • tv: Testicular volume (ml) • reg: Region (north, east, west, south, city) We will use data for boys 5-year old or older. a For further details, ?mice::boys. Jose Barrera (ISGlobal & UAB) Statistics in Health Sciences, 2023/2024 17 / 33 Linear regression analysis with MI in R: illustrative example Aim Suppose we are interested in fitting a linear regression model to estimate the mean testicular volume (tv) as a function of age (age), adjusting by region (reg), among boys 5-year old or older. The following plot include only observations that are complete in age, tv and reg. . . Testicular volume (ml) 25 Region north east west south city 20 15 10 5 5 10 15 20 Age (decimal years) but we would like to use all observations. . . Jose Barrera (ISGlobal & UAB) Statistics in Health Sciences, 2023/2024 18 / 33 Linear regression analysis with MI in R: illustrative example Data in R > > > > > library(mice) ### ?boys # ("boys" data set is included in the "mice" package) ### We will work only with boys 5-year old or older: boys5 <- boys[boys$age >= 5, ] head(boys5) ## ## ## ## ## ## ## 2874 2883 2885 2891 2892 2932 age 5.196 5.286 5.292 5.333 5.338 5.533 hgt 111.1 128.7 109.3 112.9 123.0 116.3 wgt 20.9 30.6 19.6 19.1 28.1 20.2 bmi 16.93 18.47 16.40 14.98 18.57 14.93 hc 54.0 55.0 52.4 53.0 52.5 50.0 gen <NA> <NA> <NA> <NA> <NA> <NA> phb <NA> <NA> <NA> <NA> <NA> <NA> tv reg NA east NA east NA south NA south NA city NA west > summary(boys5) ## ## ## ## ## ## ## ## age Min. : 5.196 1st Qu.:11.545 Median :14.540 Mean :14.129 3rd Qu.:16.807 Max. :21.177 hgt Min. :109.3 1st Qu.:150.8 Median :170.3 Mean :165.4 3rd Qu.:180.7 Max. :198.0 NA's :3 Jose Barrera (ISGlobal & UAB) wgt Min. : 19.10 1st Qu.: 39.12 Median : 54.80 Mean : 54.35 3rd Qu.: 66.90 Max. :117.40 NA's :3 bmi Min. :13.69 1st Qu.:16.82 Median :18.77 Mean :19.18 3rd Qu.:20.89 Max. :31.74 NA's :3 hc Min. :48.20 1st Qu.:53.70 Median :55.50 Mean :55.39 3rd Qu.:57.00 Max. :65.00 NA's :36 Statistics in Health Sciences, 2023/2024 gen G1 : 56 G2 : 50 G3 : 22 G4 : 42 G5 : 75 NA's:212 phb P1 : 63 P2 : 40 P3 : 19 P4 : 32 P5 : 50 P6 : 41 NA's:212 tv Min. : 1.00 1st Qu.: 4.00 Median :12.00 Mean :11.89 3rd Qu.:20.00 Max. :25.00 NA's :231 reg north: 60 east :105 west :143 south:107 city : 42 19 / 33 Linear regression analysis with MI in R: illustrative example Exploration of missingness > ### percentage of complete cases: > 100 * mean(complete.cases(boys5)) ## [1] 48.7965 > ### percentage of cells with missing value: > 100 * mean(is.na(boys5)) ## [1] 17.01921 Jose Barrera (ISGlobal & UAB) Statistics in Health Sciences, 2023/2024 20 / 33 Linear regression analysis with MI in R: illustrative example “1” = observed; “0” = missing ## ## ## ## ## ## ## ## ## ## ## 223 19 1 1 176 34 1 1 1 age reg hgt wgt bmi hc gen phb tv 1 1 1 1 1 1 1 1 1 0 1 1 1 1 1 1 1 1 0 1 1 1 1 1 1 1 1 0 1 1 1 1 1 1 1 1 0 1 0 2 1 1 1 1 1 1 0 0 0 3 1 1 1 1 1 0 0 0 0 4 1 1 0 0 0 1 1 1 1 3 1 1 0 0 0 0 1 1 1 4 1 1 0 0 0 0 0 0 0 7 0 0 3 3 3 36 212 212 231 700 tv phb gen hc bmi wgt 223 0 19 1 1 1 1 2 176 3 34 4 1 3 1 4 1 7 0 Jose Barrera (ISGlobal & UAB) hgt age > mice::md.pattern(boys5, + rotate.names = TRUE) reg blue = observed; maroon = missing Missingness patterns 0 Statistics in Health Sciences, 2023/2024 3 3 3 36 212 212 231 700 21 / 33 Linear regression analysis with MI in R: illustrative example Setting the imputation process: initial setting First, we run the imputation model but with no iterations (i.e. no imputations are made), to get the default setting that R uses and modify it to our convenience: > ### ?mice > ini <- mice(boys5, maxit = 0) > ini ### note that age doesn't need to be imputed ## ## ## ## ## ## ## ## ## ## ## ## ## Class: mids Number of multiple imputations: 5 Imputation methods: age hgt wgt bmi hc gen phb "" "pmm" "pmm" "pmm" "pmm" "polr" "polr" PredictorMatrix: age hgt wgt bmi hc gen phb tv reg age 0 1 1 1 1 1 1 1 1 hgt 1 0 1 1 1 1 1 1 1 wgt 1 1 0 1 1 1 1 1 1 bmi 1 1 1 0 1 1 1 1 1 hc 1 1 1 1 0 1 1 1 1 gen 1 1 1 1 1 0 1 1 1 tv "pmm" reg "" See the help of the mice function for details on the available imputation methods. Jose Barrera (ISGlobal & UAB) Statistics in Health Sciences, 2023/2024 22 / 33 Linear regression analysis with MI in R: illustrative example Setting the imputation process: customizing the imputation method • The mice function in the mice package includes a number of possible imputation methods, depending on the metric of the variable to be imputed. See “Details” in ?mice. • We can customize the imputation method used for each imputed variable with the argument method. • A special case is one in which there are variables related in a deterministic way. For instance, in weight (kg) our data set, we need to make sure that data obey the relationship BMI = (height . It can be (cm)/100)2 done using passive imputation: > > > > ### Get the imputation method and modify: meth <- ini$method ### passive imputation for bmi: meth["bmi"] <- "~I(wgt / (hgt / 100)^2)" Passive imputation also affects the predictorMatrix argument (see next slide). Jose Barrera (ISGlobal & UAB) Statistics in Health Sciences, 2023/2024 23 / 33 Linear regression analysis with MI in R: illustrative example Setting the imputation process: customizing the predictors • The predictorMatrix is p ⇥ p, where p is the number of variables in the data set, containing 0/1 data specifying the set of predictors to be used for each target (i.e. to be imputed) variable. Each row corresponds to variable to be imputed. A value of 1 means that the column variable is used as a predictor for the row target variable. By default, all values are 1, except for the diagonal. • For instance, we can modify the predictorMatrix to avoid BMI being a predictor for height or weight: > > > > > ### Get the predictorMatrix and modify: pred <- ini$predictorMatrix ### We made sure to exclude bmi as a predictor for the imputation of hgt and wgt: pred[c("hgt", "wgt"), "bmi"] <- 0 pred ## ## ## ## ## ## ## ## ## ## age hgt wgt bmi hc gen phb tv reg age hgt wgt bmi hc gen phb tv reg 0 1 1 1 1 1 1 1 1 1 0 1 0 1 1 1 1 1 1 1 0 0 1 1 1 1 1 1 1 1 0 1 1 1 1 1 1 1 1 1 0 1 1 1 1 1 1 1 1 1 0 1 1 1 1 1 1 1 1 1 0 1 1 1 1 1 1 1 1 1 0 1 1 1 1 1 1 1 1 1 0 Jose Barrera (ISGlobal & UAB) Statistics in Health Sciences, 2023/2024 24 / 33 Linear regression analysis with MI in R: illustrative example Setting the imputation process: customizing the predictors (cont.) • Our data set could include variables that don’t have any role in the imputation process. An example is a identifier variable, say id. In that case, id should be neither imputed nor a predictor variable. Hence, we would need to set: > meth["id"] <- "" > pred["id"] <- "" # do not impute "id" # do not use "id" as a predictor for any variable • In addition, when MI is performed to then fit a regression model, as in this example, we should make sure that each of the variables included in the main analysis model (i.e. in the regression model of interest) is predictor for each of the other. Hence, in our example each of the variables tv, age and reg, should be predictor of the others in the imputation process. Jose Barrera (ISGlobal & UAB) Statistics in Health Sciences, 2023/2024 25 / 33 Linear regression analysis with MI in R: illustrative example Performing the imputations Now, we can perform MI using the modified methods and predictor matrix, and using 10 imputations: > > > > > + + + + + + ### number of imputed data sets: M <- 10 ### imputations: imps <- mice(data = boys5, m = M, method = meth, predictorMatrix = pred, maxit = 20, printFlag = FALSE, # set printFlag = TRUE until the analysis is definitive seed = 666) Jose Barrera (ISGlobal & UAB) Statistics in Health Sciences, 2023/2024 26 / 33 Linear regression analysis with MI in R: illustrative example Testicular volume (mean) Testicular volume (variance) > ### mean in the original data set: > mean(boys5$tv, na.rm = TRUE) > ### variance in the original data set: > var(boys5$tv, na.rm = TRUE) ## [1] 11.89381 ## [1] 63.88201 > ### mean in the first imputed data set: > imp1 <- mice::complete(data = imps, action = 1) > mean(imp1$tv) > ### variance in the first imputed data set: > imp1 <- mice::complete(data = imps, action = 1) > var(imp1$tv) ## [1] 12.29322 ## [1] 64.41384 > > > > > > > > > > > > ### pooled mean using all imputed data sets: library(miceadds) # withPool_MI # list of means from each imputed data set: means <- with(imps, mean(tv)) # pool means: withPool_MI(means) ## [1] 12.36608 Jose Barrera (ISGlobal & UAB) ### pooled variance using all imputed data sets: library(miceadds) # withPool_MI # list of variances from each imputed data set: vars <- with(imps, var(tv)) # pool variances: withPool_MI(vars) ## [1] 64.64265 Statistics in Health Sciences, 2023/2024 27 / 33 Linear regression analysis with MI in R: illustrative example Fitting the regression model in the original data set > modorig <- lm(tv ~ age + reg, data = boys5) > summary(modorig) ## ## ## ## ## ## ## ## ## ## ## ## ## ## ## ## ## ## ## ## ## ## ## Call: lm(formula = tv ~ age + reg, data = boys5) Residuals: Min 1Q -10.1672 -2.9345 Median -0.4986 3Q 2.4073 Max 10.2126 Coefficients: Estimate Std. Error t value Pr(>|t|) (Intercept) -21.87424 1.49505 -14.631 < 2e-16 *** age 2.15914 0.08892 24.282 < 2e-16 *** regeast 4.68260 0.94114 4.975 0.00000131 *** regwest 3.61709 0.91099 3.970 0.00009715 *** regsouth 3.98715 0.91632 4.351 0.00002072 *** regcity 2.49995 1.19229 2.097 0.0372 * --Signif. codes: 0 '***' 0.001 '**' 0.01 '*' 0.05 '.' 0.1 ' ' 1 Residual standard error: 4.179 on 220 degrees of freedom (231 observations deleted due to missingness) Multiple R-squared: 0.7327,Adjusted R-squared: 0.7266 F-statistic: 120.6 on 5 and 220 DF, p-value: < 2.2e-16 Jose Barrera (ISGlobal & UAB) Statistics in Health Sciences, 2023/2024 28 / 33 Linear regression analysis with MI in R: illustrative example Fitting the regression model in the 1st imputed data set > modimp1 <- lm(tv ~ age + reg, data = imp1) > summary(modimp1) ## ## ## ## ## ## ## ## ## ## ## ## ## ## ## ## ## ## ## ## ## ## Call: lm(formula = tv ~ age + reg, data = imp1) Residuals: Min 1Q -12.1793 -3.2220 Median -0.6139 3Q 3.1626 Max 10.4163 Coefficients: Estimate Std. Error t value Pr(>|t|) (Intercept) -17.25001 1.08139 -15.952 < 2e-16 *** age 1.84122 0.05875 31.338 < 2e-16 *** regeast 5.07733 0.74192 6.843 2.53e-11 *** regwest 3.30778 0.69899 4.732 2.98e-06 *** regsouth 4.05642 0.73325 5.532 5.37e-08 *** regcity 4.10041 0.91599 4.476 9.62e-06 *** --Signif. codes: 0 '***' 0.001 '**' 0.01 '*' 0.05 '.' 0.1 ' ' 1 Residual standard error: 4.519 on 451 degrees of freedom Multiple R-squared: 0.6864,Adjusted R-squared: 0.6829 F-statistic: 197.4 on 5 and 451 DF, p-value: < 2.2e-16 Jose Barrera (ISGlobal & UAB) Statistics in Health Sciences, 2023/2024 29 / 33 Linear regression analysis with MI in R: illustrative example Fitting the regression model in all imputed data sets and pooling results > modimp <- with(imps, lm(tv ~ age + reg)) > pooledest <- summary(pool(modimp), conf.int = TRUE) > pooledest ## ## ## ## ## ## ## term estimate std.error statistic df p.value 2.5 % 97.5 % 1 (Intercept) -17.596312 1.22893640 -14.318326 89.91460 6.424416e-25 -20.0378398 -15.154783 2 age 1.859907 0.07124406 26.106134 56.35211 3.684463e-33 1.7172076 2.002606 3 regeast 5.085049 0.80103130 6.348128 147.20107 2.549040e-09 3.5020424 6.668056 4 regwest 4.070209 0.88841177 4.581445 43.39399 3.876443e-05 2.2790266 5.861392 5 regsouth 4.099201 0.97790888 4.191802 34.83876 1.798018e-04 2.1136115 6.084790 6 regcity 3.063818 1.31680944 2.326698 26.52301 2.787490e-02 0.3596728 5.767963 Jose Barrera (ISGlobal & UAB) Statistics in Health Sciences, 2023/2024 30 / 33 Linear regression analysis with MI in R: illustrative example Comparison of results for the coefficient of interest 2.3 2.2 ^ βage 2.1 2.0 1.9 1.8 1.7 Original data set 1st imputed data set All imputed data sets (pooling) Data used in the main analysis (The R code to reproduce this plot is included in the file SHS_05_Missing_data.R) Jose Barrera (ISGlobal & UAB) Statistics in Health Sciences, 2023/2024 31 / 33 Linear regression analysis with MI in R Further details You can find additional explained examples and R code in the document Exercises_SHS-CDA_v0_4.pdf, section 3. Jose Barrera (ISGlobal & UAB) Statistics in Health Sciences, 2023/2024 32 / 33 References [1] DB. Rubin. Inference and missing data. Biometrika, 63(3):581–592, 1976. URL https://doi.org/10.2307/2335739. [2] SR. Seaman and IR. White. Review of inverse probability weighting for dealing with missing data. Statistical Methods in Medical Research, 22(3):278–295, 2013. URL https://doi.org/10.1177/0962280210395740. [3] DB. Rubin. Multiple Imputation for Nonresponse in Surveys. John Wiley & Sons, Inc., Hoboken, New Jersey, 1987. [4] MA. Klebanoff and SR. Cole. Use of multiple imputation in the epidemiologic literature. American Journal of Epidemiology, 168(4):355– 357, 2008. URL https://doi.org/10.1093/aje/kwn071. [5] JA. Sterne, IR. White, JB. Carlin, M. Spratt, P. Royston, MG. Kenward, AM. Wood, and JR. Carpenter. Multiple imputation for missing data in epidemiological and clinical research: potential and pitfalls. BMJ, 338:b2393, 2009. URL https://doi.org/10.1136/bmj.b2393. [6] MJ. Azur, EA. Stuart, C. Frangakis, and PJ. Leaf. Multiple imputation by chained equations: what is it and how does it work? International Journal of Methods in Psychiatric Research, 20(1):40–49, 2011. URL https://doi.org/10.1002/mpr.329. Jose Barrera (ISGlobal & UAB) Statistics in Health Sciences, 2023/2024 33 / 33