Regression Analysis in Marketing PDF
Document Details
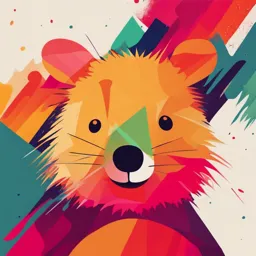
Uploaded by LuxuriantHyena
جامعة أم القرى
Tags
Summary
This document provides an introduction to regression analysis in marketing. It covers the definition, basic equation, purpose, and different types of regression, including simple and multiple linear regression, and logistic regression. It also details the application of regression in marketing for predicting sales and understanding customer satisfaction drivers.
Full Transcript
Introduction to Regression Analysis in Marketing What is Regression Analysis? Definition of regression: A statistical method to understand the relationship between variables. Basic equation: Y = β0 + β1X1 +... + βnXn + ε Purpose: Predict and explain changes in a depend...
Introduction to Regression Analysis in Marketing What is Regression Analysis? Definition of regression: A statistical method to understand the relationship between variables. Basic equation: Y = β0 + β1X1 +... + βnXn + ε Purpose: Predict and explain changes in a dependent variable. Why Use Regression Analysis? Explore relationships between variables. Make predictions based on trends. Improve decision-making based on data-driven insights. Types of Regression Linear (Simple) Regression: Relationship between two continuous variables. Multiple Regression: Involves more than one independent variable. Logistic Regression: Used for categorical dependent variables. Linear vs. Multiple Regression Simple Regression: Y = β0 + β1X Multiple Regression: Y = β0 + β1X1 + β2X2 +... + βnXn Sales = β0 + ad spending + e Sales = β0 + ad spend + price + quality + e Simple example: Sales as a function of advertising spend (linear) vs. sales as a function of advertising, price, and product quality (multiple). Application of Regression in Marketing Predicting sales based on ad spend, promotions, and pricing. Understanding customer satisfaction drivers. Estimating the impact of new marketing strategies. Marketing Example 1: Predicting Sales Predicting future sales based on marketing spend and market conditions. Use historical data to inform budget allocations. Marketing Example 2: Customer Satisfaction Analyzing survey data to determine the factors most impacting customer satisfaction. Helps companies focus on key drivers like product quality, customer service, etc. Marketing Example 3: Price Optimization Finding the optimal price point for products by analyzing demand at di erent price levels. Multiple regression can assess the impact of price along with other factors like promotions. Data Requirements for Regression Types of data: numerical (e.g., sales) for the dependent (outcome, predicted) variable for simple and multiple regression, categorical (e.g., product type) dependent variable for logistic regression. Importance of clean, complete datasets. Assumptions: Linearity, independence, homoscedasticity, no multicollinearity. Conducting a Regression Analysis (Step-by-Step) 1. Define the problem. 2. Collect and clean the data. 3. Choose the right regression model. 4. Fit the model to the data. 5. Evaluate model performance. Fitting the Model Using statistical software (e.g., Excel, SPSS, Python). Steps: load data, define variables, run regression. Output: regression coe icients, R-squared value, p-values. Evaluating Model Performance R-squared: How well the independent variables explain the variation in the dependent variable. Adjusted R-squared: Accounts for the number of predictors in the model. P-values: Determine the statistical significance of the predictors. Interpreting Regression Coe icients Each coe icient represents the impact of an independent variable on the dependent variable. Positive vs. negative relationships. The magnitude of coe icients tells the strength of the e ect. Example of Interpretation Example: "For every $1,000 spent on advertising, sales increase by 5 units." Discuss statistical significance (p-value). Mention the role of the intercept (β0). Common Pitfalls Multicollinearity: When predictors are highly correlated. Overfitting: Too many predictors can make the model unreliable. Violating assumptions: Can lead to biased results. Tools for Regression Analysis Software options: Excel, SPSS, R, Python, etc. Comparison of ease-of-use and functionality. Example: Running a regression in Excel. Conclusion Regression is a powerful tool in marketing for prediction and decision-making. Essential to ensure proper data and model selection. Interpretation drives actionable insights.