Statistics for Marketing Notebook PDF
Document Details
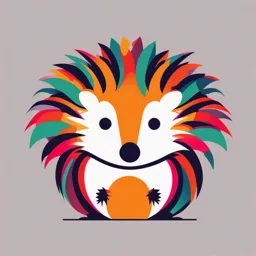
Uploaded by FearlessLoyalty
Tags
Summary
This document is a notebook containing information on a range of statistical concepts for marketing analytics, including mean, median, mode, range, standard deviation, z-scores, bias, frequency tables, contingency tables, correlation, and experimental design. It also covers simple linear regression, cluster analysis, and time series analysis.
Full Transcript
Statistics for Marketing Measures of Central Tendency Review 4 Mean 4 Median 5 Mode 6 Measures of Dispersion Review 8 Range 8 Standard Deviation 9 Z-Scores 10 Being Accurate: Avoiding Bias 12 Survey Bias 13 Culture Bias 13 Confirmation Bias 14 Observation Bias 14 Selection...
Statistics for Marketing Measures of Central Tendency Review 4 Mean 4 Median 5 Mode 6 Measures of Dispersion Review 8 Range 8 Standard Deviation 9 Z-Scores 10 Being Accurate: Avoiding Bias 12 Survey Bias 13 Culture Bias 13 Confirmation Bias 14 Observation Bias 14 Selection Bias 14 Frequency, Contingency, and Scatterplots Review 15 Frequency 15 Frequency Tables 15 Contingency Tables 17 Correlation 20 Scatter Plots 20 Correlation Coefficient 22 Experimental Design Review 25 Questioning 25 Hypothesis 26 1 Required Variables 27 Choosing a Measurement Approach 27 Selecting an Analysis 28 Hypothesis Testing in Spreadsheet Review 29 More on Hypothesis Testing 29 P-Values 30 Confidence Intervals 31 Hypothesis Testing and A/B Tests 34 Testing Hypotheses 34 The Null Hypothesis (H0) and The Alternative Hypothesis (H1) 34 A/B Tests 35 False Positives and False Negatives Review 36 False Negatives – Type II Errors 37 Simple Linear Regression Review 37 The Purpose of SLR 37 Variable Requirements for SLR 38 Data Assumptions for SLR 38 SLR In Action 39 Cluster Analysis Review 41 The Purpose of Cluster Analysis 41 Variable Requirements for Cluster Analysis 41 Data Assumptions for Cluster Analysis 41 Cluster Analysis In Action 43 Time Series Analysis Review 44 The Purpose of Time Series Analysis 45 Variable Requirements for Time Series Analysis 45 Data Assumptions for Time Series Analysis 45 2 Time Series Analysis In Action 46 Choosing a Model Review 48 Purpose 48 Variable Requirements 48 Data Assumptions 48 Which Model Fits Your Analysis? 49 3 Measures of Central Tendency Review Descriptive statistics, such as measures of central tendency, give you basic information about the data that you or your company have gathered, so you can better understand your audience, your sales, and your marketing efforts. Measures of central tendency include the mean, median, and mode of a set of data values. In this reading, let’s review what these measures of central tendency are and how to calculate them both by hand and in a spreadsheet. Measures of central tendency allow you to find the middle of a dataset. This middle—whether it be the mean, the median, or the mode—is a quick and easy way to summarize a dataset in a single number. Knowing the mean, median, or mode of a dataset can help marketers understand customer behavior, sales frequency, and other key metrics quickly and easily. Mean The mean of a dataset is the average value of that data. For data gathered over time, mean is best used when your data is recorded regularly—like every day, week, or month—so that you can consider measurements like daily averages (e.g., average number of website visits per day). For data characterizing grouped values, like the different ages within a group of customers, the mean is best used when there are no significant outliers (values that are unusually high or unusually low relative to the other values in the group). To find a mean, add up every value in your set and divide that sum by the number of values: You can always do this calculation yourself but, for data that you have collected in a spreadsheet, you can have your spreadsheet calculate the mean for you. This is particularly useful for a large set of values! Spreadsheet software usually has a function that calculates a mean. In Excel and in Google Sheets, functions like these are implemented by entering a formula specifying the values that the function is performing the calculation upon. So, for the function that calculates a mean, we can enter the formula =AVERAGE(Ax:Ay) into the cell where we want the calculated mean to be shown (Ax is the spreadsheet cell containing the first in a range of values and Ay is the cell containing the last value in the range). Simply type the =AVERAGE(Ax:Ay) formula into another 4 cell of your spreadsheet and the mean of the values in the range of cells, Ax through Ay, will be displayed. Here is an example calculating the mean for a set of ten customer ages. In this example, all the ages added together equals 325 years. Since there are ten customer ages being added together, the mean for that group of customers is 325/10 = 32.5 years of age. The spreadsheet above illustrates how the =AVERAGE(Ax:Ay) formula was used in Excel to find this mean. The ten customer age values are located in cells C3 through C12 so, when you enter the formula =AVERAGE(C3:C12) into cell F8, the mean of those values is displayed in cell F8. Median If you have significant outliers in a group of data values, it is best to use the median for the middle value of your dataset, rather than the mean. Median is the number that is in the exact middle of your dataset after you sort all the numbers from smallest to largest. For example, if you have a dataset of five values sorted from smallest to largest, the median would be the third value in the list of sorted values, because the third value is located in the exact middle of the list. Notice that you get a median by simply sorting and finding it—no need to calculate! However, if there is an even number of entries you will need to take the average of the two numbers in the middle. So, if you had a dataset of eight values sorted from smallest to largest, the median would be the average of the fourth and fifth values. Of course, the larger the set of values you find the median for, the harder it will be to sort so many numbers from smallest to largest. Fortunately, spreadsheets can help you with this task, too. 5 Spreadsheet software again usually has a function to find the median. In Excel and in Google Sheets, that function is implemented with the =MEDIAN(Ax:Ay) formula, where Ax is the spreadsheet cell containing the first in a range of values and Ay is the cell containing the last value in the range. Simply type the =MEDIAN(Ax:Ay) formula into the cell of your spreadsheet where you want the median to be shown, and the median of the values in that range of cells will be displayed. Here is an example for calculating the median of our set of ten customer ages: This spreadsheet illustrates how the =MEDIAN(Ax:Ay) formula was used in Excel to find the median. Again, the ten customer age values are located in cells C3 through C12 so, when you enter the formula =MEDIAN(C3:C12) into cell F9, the median of those values is displayed in cell F9. Notice how the =MEDIAN function lets you display the median of the dataset without having to rearrange the data in your sheet. You don’t have to sort the values from smallest to largest—the =MEDIAN function does all that for you, in the background, so the data in your sheet can remain unchanged! Mode Finally, our last measure of central tendency is mode. The mode of a dataset is simply the value that occurs most frequently. Mode is particularly useful when you are looking at frequencies of categories, like which products sold the most, or which types of services customers signed up for the most. Again, spreadsheet software usually has a function that finds the mode of a dataset. In Excel and in Google Sheets, that function is implemented with the =MODE(Ax:Ay) formula, where Ax is the 6 spreadsheet cell containing the first in a range of values and Ay is the cell containing the last value in the range. Simply type the =MODE(Ax:Ay) formula into the cell of your spreadsheet where you want the mode to be shown, into another cell of your spreadsheet, and the mode of the values in that range of cells will be displayed. This spreadsheet illustrates how the =MODE(Ax:Ay) formula was used in Excel to find the mode of our customer ages. Again the ten customer age values are located in cells C3 through C12 so, when you enter the formula =MODE(C3:C12) into cell F10, the mode of those values is displayed in cell F10. In this case, the only value that occurs more than once is the age 25. Since that age occurs most frequently in our list of customer ages, 25 is the mode of this list. Even though the idea of mode is quite simple, it can also be difficult to find when you have a large dataset. Imagine trying to determine the most frequent value in a list of a thousand numbers! This is another time when your spreadsheet software may help you. You should be aware that it’s possible for there to be multiple modes in a dataset. This happens when there is more than one value that occurs with the highest frequency. For example, here is a set of numbers that has two modes: {5, 3, 3, 1, 4, 5}. In this case, 5 and 3 are both modes in the set, because they both occur twice and no other value occurs more than once. When a dataset has two modes, we call it “bimodal.” Of course, you could have even more than two modes in a dataset. We refer to a dataset with more than two modes as “multimodal.” It’s important to know that the =MODE(Ax:Ay) formula will report only one of the modes for a bimodal or multimodal set. Specifically, it will report the first mode that occurs when reading the values in order. So, if we ran the =MODE(Ax:Ay) formula on our example set, {5, 3, 3, 1, 4, 5}, our spreadsheet would report 5 as the mode of this set because 5 is the first of the modal values to occur in the set. 7 These measures of central tendency can be valuable tools for analyzing marketing data. You are now ready to use them yourself and know how to let your spreadsheet software help you out! Measures of Dispersion Review Measures of dispersion can be important tools for understanding your marketing data. In marketing analytics, we use these tools to describe the variance within a dataset. In this context, variance refers to a number that expresses how “spread out” our data points are from the mean of the dataset. So, measures of dispersion are the tools we use to describe how much the values within our dataset deviate from the mean value of that set. In marketing analytics, variance is used in many different ways. It is an important part of risk analysis because if there is a high degree of variance in your data, you cannot accurately predict an outcome from that data. Consequently, actions based on those predictions pose a higher risk of being wrong. Variance is an important indication of data reliability. In general, it helps you determine how much you can trust what your data seems to say. Additionally, variance can help marketing analysts make decisions about who might be a reasonable target audience, or judge if a targeted campaign was successful in reaching its audience. Simply put, measures of dispersion can help you calibrate marketing efforts to improve your chances of hitting your targets! Range There are several ways to express variance, or how spread out your data is. The simplest way is by calculating the range of your data. Range is just the difference between the highest value in your dataset and the lowest value. You calculate the range of a dataset by subtracting the minimum value in the set from the maximum value: From this calculation you can see how a large range reflects a higher degree of variance, because it shows a bigger spread between the highest and lowest values in the set. These values will be spread further from the mean than in cases where the range is smaller and less spread out. The degree of variance tells you how accurate the mean value is likely to be as a prediction of future values. So, if your dataset has a small range (with less deviation from the mean), then the mean is probably a good indication of future values for that set. But if the set has a large range, then there’s a greater chance that future values will not be close to the mean. Recall our sample dataset for customer ages that we used in our earlier reading to calculate a mean: 8 If you look closely at the different ages represented here, you’ll notice that the highest age is 50 and the lowest age is 19. So, the range of ages for this dataset is 50 - 19 = 31 years. What do you think: Does this seem like a high variance or a low one? Standard Deviation While range is a quick and easy way to determine variance in a dataset, standard deviation is a more precise measure of dispersion. It is more precise because it gives you a specific range of values that captures a specific percentage of your dataset. For example, standard deviation can be used to analyze a set of sales figures and tell you that, based on past marketing efforts, 68% of your customers are likely to spend between $18 and $21. Standard deviation does this by breaking your dataset into standardized blocks, based on percent difference from the mean. The calculation for standard deviation—which we’ll let our spreadsheets do for us—determines what values capture 34% of our dataset, on each side of the mean value. For example, let’s say we have another dataset of customer ages that shows a mean value of 50 years old. Then, let’s say the standard deviation of this set is calculated to be 5 years. This indicates that 34% of our customers are between the ages of 45 and 50 (because 50 - 5 = 45 years), and another 34% of our customers are between the ages of 50 and 55 (because 50 + 5 = 55 years). It also indicates that 68% of our customers (34% + 34%) are between the ages 45 and 55, and that these customers are evenly spread out around the mean of our dataset! 9 As mentioned, standard deviation is a calculated value that most spreadsheet software will have a formula for. In Excel and Google Sheets, the formula for standard deviation is =STDEV(A1:A2). Simply type the =STDEV(A1:A2) formula into another cell of your spreadsheet and the standard deviation for values in the range of cells will be displayed. Once you know the standard deviation for your dataset, it can be used to determine variance based on a normal distribution curve, or “bell curve,” which estimates that the next standard deviation (i.e., “two standard deviations”) will capture an additional 13.6% of data values on each side of the mean. This shows that, in normal cases, about 95% of your data (68% for the first standard deviation, plus this additional 27.2% for the second standard deviation) should fall within the range of two standard deviations. Looking at our example dataset of customer ages, where we assumed a standard deviation of 5 years and a mean of 50 years, if we use this measure to evaluate two standard deviations, we can determine that 95% of our customers should fall within the range of ages 40-60 years old. This method can be carried on to show that three standard deviations will cover ages 35-65 years, which the “bell curve” shows us will capture 99% of our typical customers. This also suggests that any customer ages outside of this range would represent a statistical outlier (an unusually high or unusually low age) compared to our set of typical customers. Z-Scores A z-score uses the concept of standard deviation to indicate precisely how far a certain value is from the mean of a dataset and, most importantly, how it compares to the observed distribution of values within that set. Here is the formula for calculating a z-score: 10 “Value,” in this formula, is the value that you are calculating the z-score for and you may notice how the z-score just interprets that value’s distance from the mean as a number of standard deviations. Put another way, the z-score of a value just tells you how many standard deviations away from the mean that value lies. For example, if we go back to the dataset of customer ages in our discussion of standard deviation, we might compute a z-score for the age 37. Recall how we said that the mean value for that set was 50 years old and the standard deviation was determined to be 5 years. With this information we can compute the z-score for age 37: This score tells us that the age 37 lies 2.6 standard deviations away from the mean age of 50, and the fact that it is a negative number just indicates that it lies 2.6 standard deviations below the mean. What makes this score valuable is that we can use it to determine precisely what percentage of our typical customers are an age greater than and less than 37! We can find this with the help of a z-score table, like this one: Just look up the z-score value in your z-score table and it will show you a percentage. In our example, we look at the value 2.6 and find the percentage .9953 associated with it. (Note: The numbers across the top of the table just represent additional increments of z-score. For example, 11 the next column over from our circled percentage would be for a z-score of 2.61 instead of 2.6.) This tells us that our value, age 37, is further from the mean age 50 than 99.53% of the typical customer population. And that indicates that a customer aged 37 would be pretty atypical for our population. It also indicates that if I want to attract 37-year-old customers, I will probably want to try something different from what I’ve been doing to attract my current customer population! Notice how the z-score table only tabulates percentages for scores up to 3.09. That’s because any score beyond that will represent a value further from the mean than 100% of the typical population (since 3.09 already deviates further than 99.99% of that population). This tells you that any value with a z-score higher than 3.09 is a statistical outlier and should occur only rarely in your typical population. Of course, the further beyond 3.09 a value’s z-score goes, the more unusual that value is. Being Accurate: Avoiding Bias You may recall from earlier lessons how marketing analytics relies almost exclusively on sampling. This is because the evaluation questions that drive your marketing studies are typically focused on understanding the interests, behaviors, and purchasing preferences of a specific population—such as a population of typical customers. However, the logistics and cost of understanding the interests, behaviors, and purchasing preferences of a population increases as the size of that population increases and, usually, it’s simply impossible to survey every possible customer who might walk through your door! Luckily, a sound marketing analysis does not require information on every member of the target population. All you need is a sample, which is a carefully selected subset of the population that is intended to represent the whole. Sample: Carefully selected subset of a population meant to represent the group as a whole But how carefully you select your sample population is an essential consideration, because your marketing analysis will only be accurate if your sample data accurately represents your whole population. This is where the problem of bias comes into play for every marketing analyst. Bias refers to a flaw in experimental design or the data collection process, which leads to results that don’t accurately represent your target population. This means bias in your studies leads to inaccurate results in your marketing analyses. It’s important to be aware of the different ways bias may enter your studies, so that you can avoid them. There are many different kinds of bias but, in this lesson, we cover just a few that you are most likely to run into: ● ● ● ● ● Survey bias Culture bias Confirmation bias Observation bias Selection bias 12 Let’s have a closer look at these five common biases. Survey Bias Survey bias involves several things you might do, usually by accident, that can skew the results of a survey. One example of survey bias is order bias. The order in which you give questions or possible answers presents an inherent bias. For example, people are more likely to select the first answer on a list than any other answer. Also, if you ask a question specifically about if they like your company at the start of a survey, it will be fresh in their mind that they just said they liked it, and they will be more likely to select your company over other companies in later questions. Luckily, most survey software today knows about this bias and has options to deal with it, like randomizing the order of questions or answers. Another type of survey bias involves leading questions. Leading questions are exactly what they sound like: they are questions worded in a way that leads people to a desired answer. An easy example is asking someone to compare two products. Imagine someone asking you: “Would you prefer this delightful product A or that disgusting product B?” The wording of the question clearly tries to influence the outcome. (Of course I will prefer something “delightful” over something “disgusting!”) This kind of bias can be avoided by reading through your questions carefully to make sure you aren’t accidentally leading the reader to a specific outcome. Also consider the survey bias known as recall bias. Recall bias happens due to the fact that people often can’t remember events from the past or they’ll omit details upon recall. This means that they may report events or memories incorrectly when asked on a survey. The occurrence of recall bias depends a lot on your participant’s ability and willingness to report accurately about past experiences. There isn’t a lot you can do to correct for their inability or unwillingness to do so. However, you can avoid questions that ask your survey participant to write down something they remember from the past. If you must ask questions about the past, keep them short, specific, and multiple choice. Culture Bias We all look at the world using the standards that we were taught in our own culture. This may not be an issue if you are only advertising locally, and appealing to people who share your own cultural perspective. But when you start to advertise to other cities, states, or countries, cultural bias quickly becomes a problem. To accurately represent your target population, you need to “meet them where they are,” and present your survey in a way that appeals to the cultural perspective you are surveying. There are a couple of things you can do to adjust for this, including: 13 ● ● ● Making sure the people giving the surveys are from the local culture, or are at least very familiar with it. Making sure the tests are translated for intent and not just a direct translation. Using localization groups to make sure the data is appropriate for that area. Confirmation Bias Humans are wired to believe the things they expect to be true, even if their expectation may not be accurate. Put another way, the human brain seems to actively seek out things that prove its hypotheses right. Even subconsciously, your brain will pay more attention to things that fit your expectations and interpret your results in a more favorable light, and ignore things that don’t agree with your viewpoint. This natural tendency leads to the phenomenon we know as confirmation bias. For example, if you go out looking specifically for evidence that your company is the best in the world, you will probably find something that “proves” it, whether it’s actually true or not. Confirmation bias is a problem for analysts in any field and there is no easy way around it, since your brain is actively trying to “help” you prove what you want to be true. That said, try to be as objective as possible when you conduct a survey. Never go into your survey with a mindset that you are trying to prove one side or another. Keep an open mind and approach your work with curiosity and a healthy skepticism. Observation Bias People tend to act differently when they know they are being watched. This fact creates a problem for survey-takers known as confirmation bias. Obvious signs of being observed, like setting up a tripod in the room to record everything or having people in the back of the room writing things on clipboards, will make your participant feel self-conscious and often will skew your results. For example, if someone is being watched, they may give answers they think they should give, and not their true answers. To avoid this, try taking a less formal approach. Also, if you have any sensitive information to ask, let participants fill out a form in private and let them know that their data will be anonymized. By taking steps like these, your participant will feel more comfortable expressing their true feelings and opinions. Selection Bias As we noted above, the goal in sampling is to collect a sample that is a good representation of the general population. This means that the way you select your sample of survey participants is very important. You always need to ensure that you are not accidentally favoring some subsets of the general population over other subsets. When your selection of participants does favor subsets of the population, this leads to a survey bias known as selection bias. Selection bias happens when the sample is selected in such a way that it no longer represents the whole target population. The 14 best way to avoid this is to make sure you use the random sampling techniques we learned earlier in this course, when we looked at the various forms of good sampling techniques. You now have a better idea of what bias is in general, as well as a few common biases to watch out for. We’ve also suggested some ways to avoid these biases in your surveys. That said, there are literally dozens of different kinds of bias, so if you are looking at your data and suspect that you have introduced bias somewhere, double check your work and consider having someone else look it over for you. Remember, results run on a biased dataset are worse than no results at all. For a more extensive list of common statistical biases, we encourage you to look over the Centre for Evidence-Based Medicine’s “Catalogue of Bias” Frequency, Contingency, and Scatterplots Review Frequency Frequency is a fundamental part of descriptive statistics and is one of the tools you will use regularly as a marketing analyst. It tells you how often values occur in your data set. Frequency can give you quick and actionable insight into how your data divides into categories. Frequency: the number of times a value occurs in a data set For example, to determine whether gender is a factor for how your marketing appeals to potential customers, you may want to know how many of your actual customers are male and how many are female. Frequency Tables Frequency tables are often used in marketing analytics when the variables in question (like gender) are broken into categories (like male and female). Frequency tables give you a way to visualize the number of times each value in a set is recorded and is a great way to describe categorical data. Even numerical variables, like customer ages, can be categorized if you break them into chunks, like age groups. Let’s look at a basic frequency table that answers the question: How many customers subscribe to a service we offer? Suppose we have data for our customer population that assigns each customer to one of three possible subscriber status categories: active, expired, and 15 nonsubscriber. Our frequency table simply counts the number of customers that fall into each of these three categories and tabulates the frequencies (the total number of instances for each category) like this: At a glance, this very simple table gives a lot of information. For example, because there are so many more non-subscribers than subscribers, it suggests to us that the sales funnel that turns customers into subscribers may need improvement! It also tells us that more customers have let their subscription expire than have kept it active, which could indicate that they were not satisfied with the subscription. Whatever causes customers not to renew their subscription also may be the same thing that keeps customers from subscribing in the first place. These are red flags that need to be investigated further—and we discovered all of this from a simple frequency table. The procedure for creating a frequency table is straightforward. Once you’ve determined a set of relevant categories to analyze for a particular dataset, it is simply a matter of counting the number of occurrences that fall into those categories. You can do this yourself, by hand, going through every line of your data and counting occurrences. But this becomes increasingly difficult and tedious for very large datasets, with hundreds or thousands of lines of data! Fortunately, we can rely on our spreadsheet software to help us out again. Spreadsheet software usually has a function that will comb through your data and count the number of occurrences for a specified category value. In Excel and in Google Sheets, that formula is represented by =COUNTIF(A:A,“Value”), where A:A is the spreadsheet column containing the lines of data you want to comb through, and Value is the category value or word that you want to count. Simply type the =COUNTIF(A:A,“Value”) formula into another cell of your spreadsheet and the number of times the value occurs will be displayed. Here is an example that counts the number of times the three categories—Active, Expired, and nonsubscriber—occur in the column of data that is labelled “Subscriber Status” (column A of the sheet). 16 In this example, we used the =COUNTIF function to count the number of nonsubscribers in our dataset by entering =COUNTIF(A:A,“Nonsubscriber”) into cell G3 of our sheet. This combs through all the values in column A of the sheet, which contains the data for “Subscriber Status,” and counts the number of times the value “Nonsubscriber” occurs in that column. The number of occurrences is then displayed in cell G3. Note that there are in fact 500 lines of data in column A of this sheet (even though our picture only shows 23 of them), and our =COUNTIF function combs through all of them looking for occurrences of the specified value. Thankfully we don’t have to comb through those 500 lines of data by hand! Also notice that we have counts for the occurrences of “Active,” in cell E3 of this sheet, and of “Expired,” in cell F3. Can you figure out exactly how the =COUNTIF function was used to display those counts in those cells? This is a good example of how you can use the =COUNTIF function in Excel and Google Sheets to create frequency tables. Contingency Tables Contingency tables are a slightly more advanced form of frequency table and can give you a lot of information about your data. If you use them carefully, you can answer very specific questions 17 about your audience, and their behaviors, and use those answers to improve your marketing efforts. But what are contingency tables exactly? They are a type of frequency table that allows you to look at multiple categorical variables at the same time. This will give you a more fine-grained view of your data. Here’s an example: This table is similar to the basic frequency table we looked at above, but this time we are looking at two sets of categorical variables—subscription status and gender—rather than looking only at subscription status. This means we have a dataset of customers who are identified by subscription status and gender, and our contingency table counts the number of customers who fall into each combination of categories, such as male customers who are nonsubscribers, or female customers whose subscriptions have expired. The example data shown in the contingency table above might prove interesting for our marketing analysis: notice how male customers, though fewer in number, are more likely to subscribe but also much more likely to let their subscription expire. We also see that there are more female customers, but they are less likely to subscribe. If they do subscribe, however, they are more likely to keep their subscriptions active. This tells us that the sales funnel that turns customers into subscribers should focus more on female customers, while campaigns for getting customers to renew subscriptions should focus more on male customers. You can understand from these relatively simple examples how much actionable information may be presented by frequency tables, especially as you investigate multiple combinations of data with contingency tables! The procedure for creating contingency tables is basically the same as that for a simple frequency table: decide which categories you want to determine frequencies for and start counting. The only difference is that now you are counting occurrences of value combinations (like subscription status and gender) rather than occurrences of a single value (like gender alone). For data that is collected into a spreadsheet, this means you will have to comb through multiple columns of data—for example, one column containing the subscription status data and another containing gender data—and count each combination of values. This is another task that spreadsheet software can do for you. In Excel and in Google Sheets, the task is accomplished by the =COUNTIFS(A:A,“Value1”, B:B,“Value2”) formula, where A:A is the first spreadsheet column containing the data you want to comb through, and Value1 is the first category value or word that you are looking for; and B:B is another spreadsheet column containing the additional data you want to comb through, with Value2 as the second category value or word that you are looking for. The =COUNTIFS formula will count the number of times it finds a row of data with Value1 in 18 column A along with Value2 in column B. Simply type the =COUNTIFS(A:A,“Value1”, B:B,“Value2”) formula into a cell of your spreadsheet and it will display the number of times that combination of values occurs! Have another look at the contingency table above. Can you tell which cell is defined with the formula =COUNTIFS(A:A,“Expired”, B:B,“Female”)? Answer: It’s the cell that displays the number 8. Contingency tables are not limited to two variables. In fact, you can add as many variables as you like to a contingency table, but the tables can quickly get complicated and harder to read. Let’s look at the previous example again but, this time, also consider whether age is a factor. Here’s a spreadsheet with the contingency table that captures all three of the categories we now want to consider: subscription status, gender, and age. This sheet illustrates how we can use the =COUNTIFS formula to count combinations of the three variables—just add the third column and value inside the parentheses of the formula. So, in this example, we counted all the occurrences of male customers, between ages 21-30, with expired subscriptions and displayed that count in cell I5 by entering the =COUNTIFS(A:A,“Expired”, B:B,“Male”,C:C,“21-30”) formula into that cell. You can notice with this example how your contingency table will become more complicated with every additional variable you add to your combination, so that you’ll have to include significantly more cells of frequency data, which means more =COUNTIFS formulas to display your frequency counts. In this case, we have to enter thirty different =COUNTIFS formulas to capture all the frequency data we’re looking for. 19 Correlation Besides frequency, correlation is another measure that is often used in marketing analytics to evaluate potential connections between variables. Specifically, when we evaluate the correlation between two variables, we are measuring how much those two variables are related to one another. Correlation: how two variables are related For example, if you are working on an e-commerce website, you may want to know whether there is a correlation between how long someone stays on a page and how likely they are to buy something from that page. Or you may wonder: Is there a correlation between how many web pages a customer visits and how much they end up spending? Scatter Plots A key tool for visualizing the correlation between two variables is a scatter plot. A scatter plot is a simple visualization that compares two numerical variables by using a dot to represent each data point. These data points are determined by treating corresponding values of the two variables as coordinates on a graph. Here’s an example: 20 This is a scatter plot that compares the number of purchases a customer makes with the amount the customer spends per purchase. The dots form a rough line that is sloping from the upper left-hand corner to the lower right. This shows that, in general, the more purchases a customer makes, the less they spend each time. Note, we are not saying that one of these numbers is causing the other; we are only saying that there is a relationship between them, and as one changes so does the other. The plot suggests a fairly clear correlation between the two variables, amount of purchase and number of purchases, because there is a clear trend in the way the data points cluster around a line that slopes from the upper left to the lower right. This is not always the case. Sometimes there is no relationship between two variables. When that’s the case, there will be no line on the chart and the dots will be scattered at random. Here’s an example: In this case there is no trend in the plotting of the data points. The dots are scattered all over the graph. This is because there is no correlation between the plotted variables. Here’s one more example of a scatter plot that shows a correlation: 21 This scatter plot is similar to the first scatter plot above, that compared the amount of purchases with the number of purchases, in that it also shows a clear trend in the way the data points cluster around a line. But in this case the trend slopes from the lower left to the upper right. This is the opposite direction from what we saw in the first scatter plot above. This difference is described as negative correlation (in the first case) versus positive correlation (in this second case). When a scatter plot shows a trend line that goes from the upper left to the lower right, this shows a negative correlation, because as one variable increases, the other decreases. When a scatter plot shows a trend line that goes from the lower left to the upper right, it is a positive correlation, because as one variable increases, so does the other. And, as we saw above, when a scatter plot shows no trend at all because the data points are scattered at random, then there is no correlation at all. Correlation Coefficient Besides distinguishing between positive and negative correlation, we also measure correlation by degrees. What that means is that we have a way of saying precisely how much correlation exists between two variables. We can do this by calculating a correlation coefficient for our dataset. The correlation coefficient is a numerical value, ranging between -1 and 1, where -1 represents a high degree of negative correlation and 1 represents a high degree of positive correlation. Of course, there is the 0 point in between, which represents no correlation in the data! Here’s a typical correlation coefficient scale used to describe the amount of correlation indicated by a calculated coefficient: 22 There are many different scales, but a safe guide is that anything below -0.7 or above 0.7 is high correlation. Anything below -0.5 or above 0.5 is a medium correlation, below -0.3 or above 0.3 is low correlation, and anything between -0.3 and 0.3 is no correlation. So how can you come up with a correlation coefficient for a dataset? Once again, your spreadsheet software will usually have a formula you can use! In Excel and Google Sheets, the formula that will calculate a correlation coefficient for you is the =CORREL(A:A,B:B) formula. In this formula, A:A is a spreadsheet column that contains the data for the first variable you are comparing, and B:B is a column containing the data for the second variable. Here’s an example of this formula in action: 23 In this spreadsheet, the =CORREL formula in cell D3 compares “Number of Clicks” (column A) and “Number of Items Purchased” (column B) and calculates a correlation coefficient of 0.883. What does this number say to you? Is there high, medium, low, or no correlation between number of clicks and number of items purchased? If there is correlation, is it positive or negative correlation? Can you visualize what a scatter plot might look like for this data set? These are all questions we’ve learned to answer in this reading! 24 Experimental Design Review Sometimes data will simply get handed to you, but sometimes you will have to perform a study to gather data that answers specific business questions you have been given. The process of designing a study is a little less technical and more conceptual than many of the topics covered so far in this course, but let’s frame things as a set of practical skills and knowledge that can help you on your journey to becoming a marketing analyst. Experimental Design is the process of planning out your study and must be done before doing anything else. This reading walks you through five basic steps, which you can apply to the design of any kind of study. The five steps involve: 1. 2. 3. 4. 5. Questioning Hypothesis Required Variables Choosing a Measurement Approach Selecting an Analysis Questioning All studies start with a question! We study things to find answers to questions that interest us. In this course, we’ve referred to the questions that interest marketing analysts as “evaluation questions.” Evaluation questions come from many sources. Sometimes you get these questions from your manager or directors as they evaluate business needs, or even from a board. Occasionally you will get a question directly from a stakeholder or client. You may notice an anomaly in the data you are using for something else, which may cause you to want to know more about the data. Finally, as a marketer, you’re probably already thinking of every possible question you could ask about your marketing efforts and audience to help your company. But what makes a good evaluation question? First of all, it helps to be as specific as possible. Big questions are okay as a start, but you should break them down into smaller specific questions that you can actually answer. “Big picture” questions are hard to answer. For example, asking “Will business be good next year?” is a very difficult question to answer. By contrast, the following questions lead to similar insights but are answered much more feasibly: “How many new customers will our Facebook ads bring in next year, based on projections from this year?” Or, 25 “Is our conversion rate better this year than last year?” The more specific the question, the easier it is to find an answer. It’s also important to keep your marketing goals in focus. If your manager or a stakeholder asks a question, you should always do your best to answer it. Take time to listen and understand what kinds of questions your manager, directors, or clients care about and focus on those. Also, be proactive to ask your own evaluation questions when you want to discover more information about your data. When thinking up an evaluation question, you should also remain clear about what data you have access to. Know whether you can gather more information, or if your company wants you to stick strictly with a single dataset. If you can gather information, find out what kind. If you are using a pre-made data set, know what variables you have. There is nothing to be gained from asking questions that your data can’t answer. To summarize: When formulating the question to be answered by your study, remember to be specific, keep the goal in mind, and know your resources. Hypothesis Once you have a specific idea of the question you want your study to answer, you will formulate a hypothesis for your study to test. A hypothesis is a proposed explanation based on the limited information you have already as a starting point for further investigation. This doesn’t have to be a formal research hypothesis, but there are a few tips that will help you write a clear hypothesis. A clear hypothesis will have three parts that address three specific questions your tests will answer: Three Parts of a Clear Hypothesis: ● ● ● What will change? How will it change? What will cause the change? For example, we might form a hypothesis that says: “Sales will increase with a higher marketing budget.” In this case, sales are the variable we have identified as “what is going to change.” Our hypothesis also addresses “how sales change” by anticipating that sales will increase. Finally, our hypothesis addresses the question of “what will cause sales to increase?”: a higher marketing budget. Remember: When forming the hypothesis of your study, state what will change, how it will change, and what will cause that change. 26 Required Variables Determining required variables is the step in the experimental design process where you sit down and figure out exactly what information you will need to answer your question and test your hypothesis. First and foremost, you will need to determine the independent and dependent variables in your study. Recall from previous lessons how, when you conduct an experiment or test, you will typically manipulate one set of variables to see how changes in those variables influence changes measured in some other set of variables. To take an example from our earlier lessons, we might test whether the color scheme of a website influences the number of sales completed on the site. In that case, we might create two versions of our website—a version with one color scheme and a second version with another color—then count the number of completed sales for each version. So, to test whether color influences sales, we are manipulating color and measuring the effect on sales. In a test like this, we call the variable that we manipulate an independent variable. The variable whose effect we measure is called a dependent variable. In our color influence test, the independent variable would be the website’s color scheme and the dependent variable would be the number of sales. Besides independent and dependent variables, you may need to record data for some other variables that help provide context for your study. For example, you might also need to know the day, the time, or some other variable, if you think that additional information might impact your results or your ability to analyze those results. Required variables can take some thought. Before you start running your study, you want to make sure you’ve anticipated what data will be needed to fully understand and analyze your results. Choosing a Measurement Approach Once you’ve determined what variables you require data for, you next will need to determine how to acquire that data! There are many different types of experimental set-ups, or measurement approaches, you might choose from, but usually you want to keep things as simple as possible. The approach described above, for our study of color influence on sales, is a good example of a simple measurement approach (often called an “A/B Test”) where we just present our independent variable in two options (options A and B) and measure the difference in the dependent variable between those two options. The more complicated an experiment is, the greater the chance that something will go wrong. This is why simpler is usually better. That said, most companies will have a few different kinds of studies they already do, which give you possibilities to choose from. If you are in doubt about what type of measurement approach to use, don’t hesitate to ask another marketing analyst which type of study they think would be most appropriate for acquiring your required variables! 27 Selecting an Analysis Understanding the different types of analyses you might perform with your marketing data, once you’ve gathered it, is a more technical aspect of marketing analytics that we will delve into later in this course. But, when it comes to experimental design, it’s important to recognize that each analysis has specific requirements, and you need to make sure you will have all the data you need to meet those requirements. This means the desired analysis may affect what you determine to be your required variables and what measurement approach you choose. You will learn about specific analyses and their requirements in later lessons. For now, be aware that your familiarity with those different analyses will be an important factor in how you design your studies! 28 Hypothesis Testing in Spreadsheet Review More on Hypothesis Testing We have said that, in hypothesis testing, we are looking for an answer to the question, “Is there a difference?” But, in statistics, the answer to this question is not quite as simple as it may sound. What we are looking for is not whether there is just any difference between two measurements, but whether there is a statistically significant difference. A simple example may help explain: Suppose you are trying to determine whether a coin-flip is fair by determining whether you are using a “fair coin.” With a fair coin there should be no statistically significant difference between the likelihood of getting a heads versus getting a tails. Let’s say we flip our coin 100 times to test the hypothesis that the coin is fair (i.e., that there will be no difference between the number of tails versus heads), and we find that our coin flip comes up heads 48 times and tails 52 times. There is a difference in the number of tails versus heads, but is it a statistically significant difference? Probably not. It is well within the realm of possibility that a fair coin will come up with heads or tails, a few times more than the other for every 100 flips. So, this relatively small difference of 52 versus 48 is not enough of a difference to reach the conclusion that we have an unfair coin. By contrast, suppose our flips come up heads 32 times and tails 68 times. That’s a pretty big difference! It’s highly unlikely that a fair coin will come up tails 36 more times than heads, over a span of 100 flips. That much difference is a statistically significant difference that should lead us to suspect an unfair coin. 29 P-Values So how do you go about determining whether there is a statistically significant difference in your data? One way this is done in data analytics is by determining a p-value for your analysis. Technically, a p-value represents the probability that a difference between two data measurements was just a matter of random chance (the way 52 tails versus 48 heads might be), rather than being a statistically significant difference (the way 68 tails versus 32 heads would be). Put another way, a very low p-value represents a low probability that a difference is a matter of random chance and, therefore, a high probability of statistical significance. This p-value probability is usually written in decimal form, and often it is calculated for you by the software you use to run your analyses (such as spreadsheet software, like Excel, or data visualization software, like Tableau). There are also ways to calculate a p-value for yourself, such as the T-test method that we will learn later in this course. For now, it’s important to understand what you are seeing when you are presented with a p-value: it is a percentage probability that the measured difference in your data set might occur as a matter of chance. So, for example, if you run an analysis and find that your analysis reflects a p-value = 0.04, this tells you that there is a 4% chance that a measured difference would occur as a matter of chance and is not the kind of statistically significant difference that you are looking for when you are running your hypothesis test. In other words, a p-value = 0.04 tells you that, when your hypothesis test finds a difference and, therefore, tells you to accept the “alternative hypothesis” (i.e., H1, which says there is a significant difference between your two comparison groups) there’s a 4% chance that you’re incorrect in that conclusion. In practical terms, this means that if you act on that conclusion, there’s a 4% risk that you will not see the results you’re expecting. This leaves open a question about how much risk is too much risk in hypothesis testing. In terms of p-value, this amounts to a question of what would be a good p-value to look for in your analyses. The answer to that question is determined largely by considerations outside your data analysis—for example, considerations like how open your company is to changing their current website from red to blue, or how much of an increase in sales they need to see for it to be worth doing so. Generally speaking, these considerations are determined by the person, or persons, who pose the evaluation question for your analysis. Their question usually comes with a certain amount of risk tolerance they want you to factor into your analysis. This tolerance is often presented as a percentage of accuracy expressed by a value called alpha (𝛂). This alpha value determines the boundary for an acceptable p-value in your analysis. You always want a p-value that is less than or equal to the specified alpha. For example, your stakeholder may specify an alpha of 2.5% (𝛂 = 0.025), which means they are only willing to act on a hypothesis that is likely to prove incorrect no more than 2.5% of the time (i.e., they want 30 to be 97.5% confident that there’s a real difference between the options being compared). In effect, this tells you that your evaluation of significant difference should be based on a p-value less than or equal to 0.025. With this in mind, we can note that a standard alpha assumption is 5% (𝛂 = 0.05). So, typically, your analyses will assume that a p-value = 0.05 is an acceptable standard of significant difference. Confidence Intervals Finally, now that we have raised the subject of error risk and hypothesis confidence, we should consider the concept of confidence intervals. Confidence intervals establish a range of precision for the calculations you make in your analyses. Specifically, confidence intervals are a span of values around your calculated value within which you can have a specific degree of confidence that your observed results will match your calculation. Take our coin flip experiment again. For a fair coin, the number of times a flip of that coin will come up tails would be about 50 times out of 100 flips (50%). But it’s not likely to be exactly 50 times. It would not be unusual for the fair coin to come up tails 52 times, or even 41 times. That’s to say, even though the flips will come up tails 50 times on average, that doesn’t allow us to expect with 100% certainty that it will come up tails 50 times for every 100 flips. However, if the coin is fair, it turns out that I can be 95% certain that it will come up tails somewhere between 40 and 60 times! We know this because we know what the probability distribution is for a fair coin and, from this, we can calculate “confidence intervals” for the fair coin (very much like how we are able to calculate the standard deviation of a known probability distribution). In this case we’re saying that the 95% Confidence Interval for flipping tails with a fair coin is 10 flips out of 100—i.e., there is a 95% chance that tails will come up within 10 flips of the statistical mean of 50 flips (95% Confidence Interval = 10). And, because 40 flips keeps us within 10 flips of 50 (on the lower side) and 60 flips keeps us within 10 flips of 50 (on the higher side), the 95% Confidence Interval = 10 tells us that we can be 95% confident that our fair coin will come up tails somewhere between 40 to 60 times. Notice that we are pegging our degree of confidence on the calculable probabilities: I’m 95% confident that my fair coin will come up tails 40 to 60 times out of 100 because the probability distribution for a fair coin tells me that this happens 95% of the time! Confidence intervals, like our 95% Confidence Interval above, are calculated based on the probability distribution and size of the data samples we want to analyze. But, you may not always be calculating a 95% Confidence Interval—we can calculate for any degree of confidence we want. It all depends on a degree of variance that you are asked to look for in your analysis. Once again, as with p-values, you will be working with a specified alpha value (𝛂) that sets the degree of variance around your calculated value that your stakeholder is willing to tolerate. The percentage of your confidence interval (e.g., the “95%” in “95% Confidence Interval”) is just alpha subtracted from %100. 31 For example, let’s say your analysis of data reveals a tendency toward a specific value (e.g., your analysis of past sales data shows an average $200 profit per week). But, you and your stakeholders know that this past tendency doesn’t guarantee that you’ll hit that exact value every week—there will be some amount of variance around that value (i.e., the actual profit for a given week is likely to fall somewhere on either side of your average $200 weekly profit). For a clearer idea of what to expect for a specific week, your stakeholders ask you for the range of values that they can be 97.5 % confident in; that is, they want to know the range that includes 97.5% of the typical values. For example, your sales manager wants to know the range of profit around your $200 average they can expect the weekly profit to fall into, 97.5% of the time. In that case, your stakeholders are accepting a 2.5% variance from your calculated value and you would be working with an 𝛂 = 0.025, which calls for a 97.5% Confidence Interval. In this way, the percentage of your Confidence Interval is determined by your alpha value, or amount of tolerable variance, such that a 95% Confidence Interval will be based on a 5%alpha value (𝛂 = 0.05), a 97.5% Confidence Interval will be based on a 2.5%alpha value (𝛂 = 0.025), and so on. With this in mind, we can note again that a standard alpha assumption is 5%. So, typically, your analyses will assume an 𝛂 = 0.05 and look for a 95% Confidence Interval. Now you have all the information you need to understand how to calculate a confidence interval. Here is the formula for calculating a confidence interval in an Excel or Google Sheets spreadsheet: In this formula, 𝛂 is the decimal alpha value described above; “SampleSize” is the number of values in your sample, which you’ve learned to compute with the =COUNT function; and, “StandardDeviation” is the standard deviation of that sample, which you’ve learned to compute with the =STDEV function. Note that this formula will give you the confidence interval with a percentage of 1 - 𝛂 (e.g., if 𝛂 = 0.03, the formula would give you the 1 - 0.03 = 0.97 = 97% Confidence Interval). Here’s an example of our confidence interval formula in action: 32 In this spreadsheet, we are given a sample of weekly sales data for a website, which shows that we are averaging $120.25 of sales per week. We can calculate the size of our sample with the =COUNT formula in cell H3, and the standard deviation with the =STDEV formula in cell H4. We assume the typical alpha assumption of 5% (𝛂 = 0.05) so that we can calculate the 95% Confidence Interval for our sales data. We display this confidence interval in cell H6. With that 95% Confidence Interval = $7.26, we can tell our stakeholder that our data shows a 95% likelihood that next week’s sales will fall somewhere in the range between $112.99 and $127.51! (Remember: Lower end of range = Mean Value - 95% Confidence Interval, and higher end of range = Mean Value + 95% Con