PSYC212 L9: Descriptive and Inferential Statistics PDF
Document Details
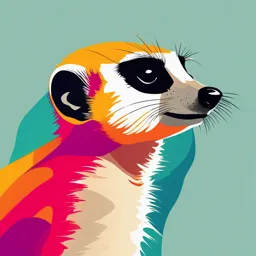
Uploaded by NavigableNonagon
Tags
Summary
This document covers descriptive and inferential statistics for a psychology course. It discusses measures of central tendency, distribution shapes, measures of variability, and how to present descriptive statistics. The document includes examples of different types of statistical analysis.
Full Transcript
Chapter 12: Descriptiv e Statistics Chapter 13: Inferential Statistics Measures of Central Tendency Point around which scores in the distribution tend to cluster Aka, What is the OVERALL response? Where did the data cluster around? 3 measures of central tendency: a) Mean: average -mos...
Chapter 12: Descriptiv e Statistics Chapter 13: Inferential Statistics Measures of Central Tendency Point around which scores in the distribution tend to cluster Aka, What is the OVERALL response? Where did the data cluster around? 3 measures of central tendency: a) Mean: average -most common method b) Median: middle score such that half the scores are greater and half the scores are less than this value -done after ranking your data c) Mode: most frequent score in a distribution; can be used on categorical data Distribution of a variable The way in which scores are dispersed across levels of that variable What is the variability around that central tendency? This can affect our interpretation of results. Can use frequency tables or histograms Distribution Shapes 1) Number of peaks: a) Unimodal: one peak with tails that taper in either direction of that peak b) Bimodal: two distinct peaks -less common, but still happens! e.g. Rule-switching behaviour in 3-year old children 3 year olds have insufficient executive functioning in that they cannot switch to the new rules as quickly as the 4 year olds. Distribution Shapes 2) Symmetry: Symmetrical or skewed? if data is not skewed, it doesn’t matter. If it IS skewed, it does! Outlier: extreme scores that is much higher or lower than the majority of scores in the distribution -can represent unique cases in the population Measures of Variability Extent to which scores vary or spread around their central tendency Spread: How tightly do they cluster around the central measurement? Observations: 1. mean, median, and mode are the same in both graphs 2. Less variability in first, more variability in second 3. Range of scores is different in both graphs. More Measures of Variability 3 common measures of variability 1) Range: difference between highest and lowest scores; easy to understand, but can be misleading if there are outliers 2) Standard deviation: average distance between the scores and mean OR E.g. SD = 1.69; scores differ from the mean by about 1.69 units Measures of Variability 3) Percentile Ranks: refers to the percentage of scores in the distribution that are lower than that score e.g., Interpretation of 85th percentile = 85% of the participants of the same test scored below you. OR, 15% of the participants of the same test scored above you. Measures of Variability 4) z-score: how far a raw score is from the mean in terms of standard deviation Important in defining outliers: ± 3 SD from the mea Describing statistical relationships Example: Treatment and control group The chart below shows an average, but it is only a snapshot in time. If you were to take the data again, how much variability and noise is there? This data is insufficient for telling you what is going on. In academic peer-reviewed papers, you must always include a measure of variability. Each group can be described by its mean and SD Common ways of presenting descriptive results Statistical values are usually reported to two decimal places M = 34.56, SD = 2.27 Bar graphs, line graphs, and tables Box and whisker plots Boxplot cover the interquartile interval where 50% of the data are found Line is the measure of central tendency Whiskers indicate the minimum and maximum It also captures Box and whisker plots APA Bar graph and line graphs servations: r graphs = mean looks similar e graphs = variability around the mean are very erent between 1st and 4th line bars show there is not a significant meaningful crease across one score to another APA Tables Observations: Some basic null hypothesis tests: The t-test 1. One-sample t-test: compare a mean against some value of comparison e.g., Is the class average mean IQ score different(statistically significantly) from 100? Some basic null hypothesis tests: The t-test 2. Dependent-samples t-test (or paired-sample t-test) where you have 2 means: To compare means for the same sample tested at two different occasions or conditions e.g. dosage of medication at diff times of day for the same individuals Some basic null hypothesis tests: The t-test 3. Independent samples t-test: To compare means from 2 different samples e.g., normal hearing group vs cochlear implant group, Compare their mean scores on a song identifying test Some basic null hypothesis tests: The Analysis of Variance (ANOVA) Used to compare means from more than 2 samples 1. Between-subjects ANOVA: compare means from different samples Clue: look at how your participants are assigned! e.g., 1st, 2nd, and 3rd graders tested as 3 separate groups. Some basic null hypothesis tests: The Analysis of Variance (ANOVA) 2. Within-subjects ANOVA (or repeated-measures ANOVA) More than 2 independent variables e.g., 1 cup, 2 cups, and 3 cups of coffee effects on the same people are tested Some basic null hypothesis tests: The Analysis of Variance (ANOVA) 3. Factorial ANOVA: used to test multiple independent variables (factors); used to understand the effect of each independent variable AND their interaction Factors can be the same type or mixed: e.g. Two-way ANOVA: -within-within subjects ANOVA -between-between subjects ANOVA -between-within (mixed) ANOVA -e.g. cell phone users and non users, and usage at day and at night