RES002 Week 1 - Preliminary Lesson - Lecture 1.1: Statistics PDF
Document Details
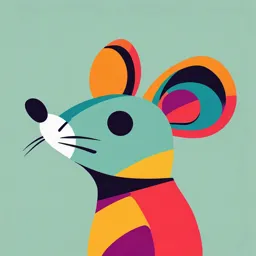
Uploaded by LikableHyperbolic
N. L. Dalmia Institute of Management Studies and Research
Joseph Elmer Noval
Tags
Summary
This document is a lecture on statistics, specifically focusing on descriptive and inferential statistics. It covers topics such as measures of central tendency (mean, median, mode), variability (range, variance, standard deviation), hypothesis testing, confidence intervals, and regression analysis. The document also highlights the importance of using appropriate statistical methods.
Full Transcript
RES002 TERM 1, 4TH YEAR WEEK 1 - PRELIM LESSON Joseph Elmer Noval LECTURE 1.1: STATISTICS INFERENTIAL STATISTICS TOPIC OUTLINE...
RES002 TERM 1, 4TH YEAR WEEK 1 - PRELIM LESSON Joseph Elmer Noval LECTURE 1.1: STATISTICS INFERENTIAL STATISTICS TOPIC OUTLINE Overview - Inferential statistics allow us to make predictions I. Statistics or inferences about a population based on a a. Definition II. Descriptive Statistics sample. a. Overview - They help in hypothesis testing, estimating b. Measure of Central Tendency c. Measures of Variability population parameters, and making predictions. III. Inferential Statistics - Examples include regression analysis, ANOVA, a. Overview and chi-square tests. b. Hypothesis Testing c. Confidence Intervals d. Regression Analysis Hypothesis Testing - Hypothesis testing is a method for testing a claim What is Statistics? or hypothesis about a parameter in a population. - Statistics is a branch of mathematics dealing with Null hypothesis (H0): A statement of no effect or data collection, analysis, interpretation, and no difference. presentation. It helps us make sense of numerical Alternative hypothesis (H1): A statement that data and draw conclusions. contradicts the null hypothesis. P-value: The probability of observing the data if DESCRIPTIVE STATISTICS the null hypothesis is true. Confidence Intervals Overview - Confidence intervals estimate the range within - Descriptive statistics summarize and describe the which a population parameter lies, based on a features of a dataset. sample statistic. - They provide simple summaries about the sample - They are expressed as a percentage (e.g., 95% and the measures. confidence interval). - The interval has an upper and lower bound, Measures of Central Tendency indicating the range of plausible values. - Central tendency describes the center of a dataset. Regression Analysis Mean: The average of all data points. - Regression analysis assesses the relationship Median: The middle value in a list of numbers. between variables. Mode: The most frequently occurring value(s). - It helps in understanding how the typical value of - Which measure of central tendency do you think the dependent variable changes when any one of is most affected by outliers? the independent variables is varied. - Linear regression is the most common form. Measures of Variability Variability gives us an idea of the spread or Choosing the Right Statistical Method dispersion of our data. - The choice between descriptive and inferential Range: The difference between the highest and statistics depends on the research question. lowest values. - Descriptive statistics are used when you want to Variance: The average of the squared describe the data. differences from the mean. - Inferential statistics are used when you want to Standard Deviation: A measure of the amount of make predictions or test hypotheses. variation or dispersion - What factors might influence your choice of statistical method? 1 Importance of Sample Size - Sample size affects the accuracy of both A Taxonomy of Statistics descriptive and inferential statistics. - Larger samples tend to give more reliable results. - However, larger samples require more resources to collect and analyze. - How might you determine the appropriate sample size for a study? Assumptions and Limitations - Both descriptive and inferential statistics have assumptions that must be met for valid results. - For example, many inferential statistics assume normal distribution of data. - Violating these assumptions can lead to incorrect conclusions. Conclusion and Discussion - Today, we've learned about the roles of descriptive and inferential statistics in research. - Descriptive statistics help us summarize data, Statistical Description of Data while inferential statistics help us make Statistics describes a numeric set of data by its predictions. Center - Both are crucial for understanding and Variability interpreting data in various fields. Shape Statistics describes a categorical set of data by Frequency, percentage or proportion of each category LECTURE 1.2: BASICS OF STATISTICS Some Definitions Variable - any characteristic of an individual or TOPIC OUTLINE entity. A variable can take different values for I. Basic of Statistics different individuals. Variables can be categorical a. Definitions II. Data Presentation or quantitative. Per S. S. Stevens… a) Graphical Nominal - Categorical variables with no inherent b) Numerical order or ranking sequence such as names or III. Methods of Center Measurement IV. Methods of Variability Measurement classes (e.g., gender). Value may be a numerical, but without numerical value (e.g., I, II, Definition: Science of collection, presentation, III). The only operation that can be applied to analysis, and reasonable interpretation of data. Nominal variables is enumeration. - Statistics presents a rigorous scientific method Ordinal - Variables with an inherent rank or for gaining insight into data. For example, order, e.g. mild, moderate, severe. Can be suppose we measure the weight of 100 patients compared for equality, or greater or less, but not in a study. With so many measurements, simply how much greater or less. looking at the data fails to provide an informative Interval - Values of the variable are ordered as account. However statistics can give an instant in Ordinal, and additionally, differences between overall picture of data based on graphical values are meaningful, however, the scale is not presentation or numerical summarization absolutely anchored. Calendar dates and irrespective of the number of data points. temperatures on the Fahrenheit scale are Besides data summarization, another important examples. Addition and subtraction, but not task of statistics is to make inference and predict multiplication and division are meaningful relations of variables. operations. 2 Ratio - Variables with all properties of Interval If a histogram is skewed to the left (also plus an absolute, non-arbitrary zero point, e.g. known as negatively skewed), it means that the age, weight, temperature (Kelvin). Addition, bulk of the data values are concentrated on the subtraction, multiplication, and division are all higher end of the distribution, with a tail extending meaningful operations. towards the lower values. Here's a breakdown of what this implies: Frequency Distribution Consider a data set of 26 children of ages 1-6 Shape: The histogram has a longer tail on the left years. Then the frequency distribution of variable side. Most of the data points (frequencies) are ‘age’ can be tabulated as follows: grouped towards the right side (higher values), with fewer data points as you move leftwards (lower values). Cumulative Frequency Cumulative frequency of data in previous page Center: The mean of the data is generally less than the median. The center of the data might still be closer to the right, where most of the data points are located, but the mean is pulled leftward due to the tail. Spread: The data has a wider spread towards the lower values. However, the frequency decreases as you move leftward, indicating that lower values are less common but present in the data set. Implications: A left-skewed histogram suggests that while most individuals/items have higher DATA PRESENTATION values (e.g., older ages, higher scores), there are a few cases with significantly lower values (e.g., - Two types of statistical presentation of data - younger ages, lower scores). This can indicate the graphical and numerical. presence of a lower-bound constraint in the data. GRAPHICAL PRESENTATION Examples: A left-skewed distribution might be We look for the overall pattern and for seen in situations like income distribution in a striking deviations from that pattern. Over all wealthy neighborhood (where most incomes are pattern usually described by shape, center, and high, with a few lower incomes) or the age spread of the data. An individual value that falls distribution of a senior community (where most outside the overall pattern is called an outlier. residents are older, with a few younger ones). - Bar diagram and Pie charts are used for In summary, a left-skewed histogram categorical variables. indicates that the majority of the data is - Histogram, stem and leaf and Box-plot are used concentrated at higher values, with a few lower for numerical variable. values pulling the tail of the distribution to the left. 3 If a histogram is skewed to the right (also dispersion (e.g., average distance from the mean) known as positively skewed), it indicates that the to indicate how well the central value characterizes bulk of the data values are concentrated on the the data as a whole. lower end of the distribution, with a tail extending - To understand how well a central value towards the higher values. Here's what this characterizes a set of observations, let us consider implies: the following two sets of data: A: 30, 50, 70 Shape: The histogram has a longer tail on the B: 40, 50, 60 right side. Most of the data points (frequencies) The mean of both two data sets is 50. But, are grouped towards the left side (lower values), the distance of the observations from the mean in with fewer data points as you move rightwards data set A is larger than in the data set B. Thus, (higher values). the mean of data set B is a better representation of the data set than is the case for set A. Center: The mean of the data is generally greater than the median. The center of the data might be METHODS OF CENTER MEASUREMENT closer to the left, where most of the data points are located, but the mean is pulled rightward due to Commonly used methods are mean, median, the tail. mode, geometric mean etc. Spread: The data has a wider spread towards the Mean: Summing up all the observations and higher values. However, the frequency decreases dividing by the number of observations. Mean of as you move rightward, indicating that higher 20, 30, 40 is (20+30+40)/3 = 30. values are less common but present in the data set. Median:The middle value in an ordered sequence of observations. That is, to find the median we Implications: A right-skewed histogram suggests need to order the data set and then find the middle that while most individuals/items have lower value. In case of an even number of observations values (e.g., younger ages, lower incomes), there the average of the two middle most values is the are a few cases with significantly higher values median. For example, to find the median of {9, 3, (e.g., older ages, higher incomes). This can 6, 7, 5}, we first sort the data giving {3, 5, 6, 7, 9}, indicate the presence of an upper-bound then choose the middle value 6. constraint in the data. If the number of observations is even, e.g., {9, 3, 6, 7, 5, 2}, then the median is the average of Examples: A right-skewed distribution might be the two middle values from the sorted sequence, seen in situations like income distribution in a in this case, (5 + 6) / 2 = 5.5. low-income area (where most incomes are low, with a few higher incomes) or age at retirement Mode: The value that is observed most frequently. (where most people retire around a certain age, The mode is undefined for sequences in which no but a few work much longer). observation is repeated. In summary, a right-skewed histogram indicates that the majority of the data is concentrated at lower values, with a few higher METHODS OF VARIABILITY MEASUREMENT values pulling the tail of the distribution to the right. Variability (or dispersion) measures the amount NUMERICAL PRESENTATION of scatter in a dataset. Commonly used methods: range, variance, A fundamental concept in summary standard deviation, coefficient of variation etc. statistics is that of a central value for a set of observations and the extent to which the central Range: The difference between the largest and value characterises the whole set of data. the smallest observations. The range of 10, 5, 2, Measures of central value such as the mean or 100 is (100-2)=98. It’s a crude measure of median must be coupled with measures of data variability. 4 LECTURE 2: SAMPLING Variance: The variance of a set of observations is the average of the squares of the deviations of the observations from their mean. TOPIC OUTLINE I. Sampling Standard Deviation: Square root of the variance. a. Definition b. Purpose c. Reason Quartiles: Data can be divided into four regions II. Sampling Process that cover the total range of observed values. Cut a. Defining Population of Interest. b. Sampling Frame points for these regions are known as quartiles. c. Sampling Technique In notations, quartiles of a data is the d. Determine the Sample Size ((n+1)/4)qth observation of the data, where q is the desired quartile and n is the number of observations of data Sampling The first quartile (Q1) is the first 25% of the - is the process of selecting a small number of data. The second quartile (Q2) is between the 25th elements from a larger defined target group and 50th percentage points in the data. The upper (Population) of elements such that the information bound of Q2 is the median. The third quartile (Q3) gathered from the small group will allow is the 25% of the data lying between the median judgments to be made about the larger groups. and the 75% cut point in the data. - is the act, process, or technique of selecting a Q1 is the median of the first half of the suitable sample, or a representative part of a ordered observations and Q3 is the median of the population for the purpose of determining second half of the ordered observations. parameters or characteristics of the whole population. Inter-quartile Range: Difference between Q3 and Q1. Inter-quartile range of the previous example is Purpose Of Sampling … 61- 40=21. The middle half of the ordered data lie - To draw conclusions about populations from between 40 and 61. samples. which enables us to determine a population's characteristics by directly observing Deciles: If data is ordered and divided into 10 only a portion (or sample) of the population. We parts, then cut points are called Deciles obtain a sample rather than a complete enumeration (a census ) of the population for Percentiles: If data is ordered and divided into many reasons. 100 parts, then cut points are called Percentiles. 25th percentile is the Q1, 50th percentile is the 6 MAIN REASONS FOR SAMPLING Median (Q2) and the 75th percentile of the data is Economy- taking a sample requires fewer Q3. resources than a census. In notations, percentiles of a data is the Timeliness- a sample may provide you with ((n+1)/100)p th observation of the data, where p is needed information quickly. the desired percentile and n is the number of The large size of many populations- many observations of data. populations about which inferences must be made are quite large Coefficient of Variation: The standard deviation Inaccessibility of some of the population- of data divided by it’s mean. It is usually expressed There are some populations that are so difficult to in percent. get access to that only a sample can be used. Destructiveness of the observation- sometimes the very act of observing the desired characteristic of a unit of the population destroys it for the intended use. Accuracy- A sample may be more accurate than a census. A sloppily conducted census can 5 provide less reliable information than a carefully - Population of interest is entirely dependent obtained sample. on Management Problem, Research Problems, and Research Design. Important terminologies Some Bases for Defining Population: Population- The population refers to the entire Geographic Area (Pakistan, Punjab, Banking group of people, events or things of interest that sector, Our Institute etc.) the researcher wishes to investigate. Demographics (Gender, Age, Color, Height Element- An element is the single member of the etc.) population. Census is a count of all elements in Usage/Lifestyle the human population. Awareness Sample- is a subset of the population. It comprises some members from it. A sample is 2. Sampling Frame thus a subgroup or subset of the population. By - A list of population elements (people, studying the sample, the researcher should be companies, houses, cities, etc.) from which able to draw conclusions that are generalizable to units to be sampled can be selected. the population of interest. - Difficult to get an accurate list. Sampling Unit- The sample unit is the element - Sample frame error occurs when certain or the set of elements that is available for elements of the population are accidentally selection in some stage of the sampling process. omitted or not included on the list. Subject- is a single member of the sample just as an element is a single member of the population. 3. SAMPLING METHOD/TECHNIQUES/TYPE Representative of Sampling Choosing the right sample cannot be over emphasized. If we choose the sample in a scientific way, we can be reasonably sure that sample statistics (Mean, Standard Deviation, (S) Variation in the sample ) and population parameters (Mean (u), Standard Deviation, Variation in the sample ) are close to each others. What is a Good Sample? Accurate: absence of bias Precise estimate: sampling error Probability sampling Sampling error is any type of bias that is - is one that gives every member of the population attributed to mistakes in either a sample or a known chance of being selected. sample size. All are selected randomly. Simple random sampling - anyone SAMPLING PROCESS Systematic sampling Stratified sampling- different groups (ages) Proportionate Cluster sampling- different areas (cities) Simple Random Sampling - is a method of probability sampling in which every unit has an equal non zero chance of being selected - Each element in the population has a known and equal probability of selection. - This implies that every element is selected independently of every other element. 1. Defining Population of Interest. 6 Systematic Random Sampling Judgmental Sampling - is a method of probability sampling in which the - is a form of convenience sampling in which the defined target population is ordered and the population elements are selected based on the sample is selected according to position using a judgment of the researcher. skip interval. - Test markets - Engineers selected in industrial marketing Stratified Random Sampling research - is a method of probability sampling in which the - Expert witnesses used in court population is divided into different subgroups and samples are selected from each Quota Sampling - may be viewed as two-stage restricted Cluster Sampling judgmental sampling. - The target population is first divided into mutually 1. The first stage consists of developing control exclusive and collectively exhaustive categories, or quotas, of population elements. subpopulations, or clusters. 2. In the second stage, sample elements are selected - Then a random sample of clusters is selected, based on convenience or judgment. based on a probability sampling technique. Snowball Sampling - For each selected cluster, either all the elements - an initial group of respondents is selected, usually are included in the sample (one-stage) or a at random. sample of elements is drawn probabilistically - After being interviewed, these respondents are (two-stage). asked to identify others who belong to the target - Elements within a cluster should be as population of interest. heterogeneous as possible, but clusters - Subsequent respondents are selected based on themselves should be as homogeneous as the referrals. possible. Ideally, each cluster should be a small-scale representation of the population. Factors to be considered in Research Design - In probability proportionate to size sampling, the clusters are sampled with probability proportional to size. In the second stage, the probability of selecting a sampling unit in a selected cluster varies inversely with the size of the cluster. Nonprobability Sampling - is an arbitrary grouping that limits the use of some statistical tests. It is not selected randomly. 4. Determining Sample Size Classifications of Nonprobability Sampling - How many completed questionnaires do we Convenience Sampling need to have a representative sample? Judgment Sampling - Generally the larger the better, but that takes Quota Sampling more time and money. Snowball Sampling Answer depends on: How different or dispersed the population is. Convenience sampling Desired level of confidence. - attempts to obtain a sample of convenient Desired degree of accuracy. elements. Often, respondents are selected because they happen to be in the right place at In conclusion, it can be said that using a sample in the right time. research saves mainly on money and time, if a - Use of students, and members of social suitable sampling strategy is used, appropriate organizations sample size selected and necessary precautions - Mail intercept interviews without qualifying the taken to reduce sampling and measurement respondents. errors, then a sample should yield valid and - "people on the street" interviews reliable information. 7 - It could be processed further into the standard LECTURE 3: MEASURES OF CENTRAL distribution. TENDENCY - It is unbiased/meaning it always gives us the population mean μ Disadvantages TOPIC OUTLINE - It may be some distance from the majority of II. Measures of Central Tendency observations a. Definitions - Can be misleading b. Mean c. Median - It is approximated for grouped data d. Mode - Sometimes the figure obtained is not anywhere in the distribution. - Can give fractional values even for ungrouped Introduction data - One of the most important objectives of statistical analysis is to get one single value that describes the characteristic of the entire mass of data. Median- the median conveys the notion of being - Such a value is called the central value or an the middle most value with in the data distribution average or the expected value of the variable. - The word average is commonly used in day to day Advantages/disadvantages of the median conversation Advantages: - Average is defined as attempt to find a single - Simple to calculate; figure to describe whole of figures - It is representative of entire distribution; - It is unique and representative of an actual Objectives of averaging figure in the distribution; - To get single value that describes the Disadvantages: characteristic of the entire group - It cannot be subjected to further processing - Measures of central value, by condensing the mass of data in one single, enable us to get a Mode- the Mode is the most common value in a bird's eye view of the entire data given range of data MEASURES OF CENTRAL TENDENCY Advantages/disadvantages of mode - Measures of central tendency are measures of the Advantages: location of the middle or the center of a - It is simple distribution. - Useful for qualitative data say the most - There are a number of measures of central handsome man; tendency and these include; mean, the median, Disadvantages: the mode - Cannot be called unbiased - A good Measure of Central tendency should have - Cannot be used to reconstruct the distribution the following characteristics: - Can not be further processed It should be easy to calculate and understand - Some distributions are bimodal It should be unique and exist at all times It should consider all observations It should not be affected by extreme values It should be suitable for further mathematical manipulation Mean- this is the summation of all observations divided by the number of observations in the sample Mean advantages/disadvantages Advantages - It summarizes the entire distribution 8