PSYC 4780 Midterm - Readings Compiled PDF
Document Details
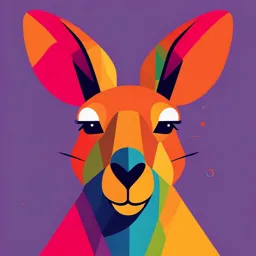
Uploaded by JovialObsidian6423
Tags
Summary
This document discusses open science and the replication crisis in psychology, focusing on topics like replication, reproducibility, and the scientific method.
Full Transcript
PSYC 4780 Midterm - Readings Compiled Week 1 Readings - Chapters 1 and 2 Chapter 1 - Introduction Open Science - reflects the idea that knowledge of all kinds should be openly accessible, transparent, rigorous, reproducible, repliable, accumulative, and inclusive - E.g., study preregistration,...
PSYC 4780 Midterm - Readings Compiled Week 1 Readings - Chapters 1 and 2 Chapter 1 - Introduction Open Science - reflects the idea that knowledge of all kinds should be openly accessible, transparent, rigorous, reproducible, repliable, accumulative, and inclusive - E.g., study preregistration, open materials and data, open access publishing - Open science can reform psychology because of transparency Chapter 2 - The Replication Crisis Replication = the cornerstone of science - To know whether or not a finding is real, it must be confirmed that the results can be found again Replicability - obtaining consistent results across studies aimed at answering the same scientific question, each of which has obtained its own data Contrasted with: Reproducibility - obtaining consistent results when the same data are analyzed using the same techniques To replicate: 1. Repeat your own/someone else’s experiment 2. Collect new data - do the original results hold on repeated tries? 3. Rerun the same analytical tests on your new data - do you get the same numbers? Scientific Method: 1. Observation 2. Question (about what has been observed) 3. Hypotheses 4. Experiments 5. Conclusion 6. Replication - only after multiple successful replications should a result be recognized as scientific knowledge Two main types of replication: 1. Exact Replication (direct replication) - exactly the same - Aim to exactly recreate an original study based on its research questions, hypotheses, methods, context, treatments, and analyses - E.g., dropping an apple - when repeating, apple would need to be exact weight, size, and dropped from the same height - Inform whether the original finding is a true effect or if it was a false positive (Type I error) - Allows us to test, redefine, or develop more robust theories 2. Conceptual Replication - tests the concept in a different way - Test the same research questions/hypotheses but use slightly different measures, treatments/analyses, OR assess whether results hold under different conditions (e.g., context or time) - E.g., dropping an apple - try dropping a different object from the same height, or the same object from a different height - Help us identify under which conditions the results hold - Allows us to assess the generalizability of theories + their boundary conditions (e.g., within a certain population or context) What Science Should Be Credible - be willing to have claims/discoveries scrutinized Trustworthy - results reported accurately, general public is able to trust findings Transparent - be crystal clear, methods/results reported in detail (allowing for independent replication and evaluation) Accessible - other researchers and the general public having easy access to scientific findings Inclusionary - science is diverse and inclusive, scientists from underrepresented groups have equal opportunities Collaborative - maximize the use of available resources, collaborative work (vs competitively) Self-correcting - based on accurate evidence in the pursuit of knowledge, errors corrected and explained AND normalized Replication Crisis - the finding and related shift in academic culture and thinking, that a large proportion of scientific studies do not replicate - Successful replication - when research is rerun and obtains the same result - Failed replication - when an attempted replication finds different or null findings Landmark events in the history of the replication crisis Event 1 - ‘Most published research findings are false’ - John Ioannidis (2005) - many of the findings in his field of medicine were false positives - concluding there is an effect when it does not exist - Main reasons: - Over reliance on small + underpowered sample sizes - Preoccupation with p-values to denote statistical significance - Flexibility in research design/analyses - Competition among researchers to produce positive results in fashionable areas Event 2 - Could Bem ‘feel the future’? - Daryl Bem (2011) wrote an article that evidenced precognition - people’s conscious awareness of future events can influence current ones - ‘Time reversed’ 4 well-established psychological effects = causal stimulus occurred after participants’ responses were recorded - Bem showed the number of words participants recalled in the first test could be predicted by a test they took afterwards - Memory recall in the first test was higher for words participants would see again the future = ‘retroactive facilitation of recall’ - Better at recalling words they saw again compared to those they didn’t - These results were unable to be replicated by multiple researchers BUT the same journal article who published Bem, rejected the replications - “The field of psychology did not care about replications that would question the reality of these effects” Event 3 - Stapel’s fraudulent fixings - One of Stapel’s widely publicized studies suggested that policymakers could fight racism/discrimination by cleaning up environments (e.g., train stations) - After being accused of academic misconduct, he attempted to replicate the train station study + could not find the location described in the paper - Fortunately, academic fraud is very rare Event 4 - Meta-research shines a light on questionable research practices - Meta-research is centered on how researchers do research - methods, reporting, reproducibility, evaluation, and incentives - Questionable Research Practices - exploit the gray area of scientific norms for collecting and analyzing data - Flexibility in data collection, analysis and reporting = researchers presenting ‘anything as significant’ - E.g., optional stopping - analyze the data as it is collected + stop when statistical significance is reached - E.g., p-hacking - perform many different unplanned tests, trying different variables when one doesn’t reach p <.05 - E.g., hypothesizing after results are known - Simmons found researchers could double false positive rates by using a single QRP, increasing to 60% when multiple were used - Simmons was able to show that people were statistically younger after listening to a Beatles song - proving time-reversed aging John et al. (2012) - self-report QRP study (n = 2000 researchers) - 60% of researchers failed to report all of their dependent measures - 50% engaged in optional stopping - 40% selectively reported experiments that had ‘worked’ - Researchers also reported their colleagues did all of these way more than they had - They believed these were defensible b/c they constituted academic norms of the time Event 5 - Psychology’s crisis of confidence - 2012 Pashler and Wagenmakers declare psychology is facing a ‘crisis of confidence’ in Perspectives on Psychological Science Event 6 - ‘Many labs’ unite - 2014: the first large-scale replication attempt in psychology is published, known as ‘Many Labs’ - Tested the replicability of 13 classic psychological findings across 12 countries and approx. 6300 participants - Selected based on: - Relatively short studies - Simple design - Easily conducted online - Studies included: ‘sunk cost fallacy’, influence of gain vs loss framing on risk-taking, sex differences in implicit attitudes towards math or arts - 77% of studies replicated the original findings - BUT researchers argued that several of these classic studies were already thought to be highly replicable - AND all this said was that there were at least 10 effects in the entirety of psychology that could be replicated Event 7 - The Open Science Collaboration - 2015: Open Science Collaboration (OSC) publishes the Reproducibility Project: Psychology - 270 researchers attempt to replicate 100 randomly selected findings - Confirmed the original designs - Increased sample sizes = statistical power - Registered their methods + analysis plans before collecting data - Only 36% successfully replicated with a p-value below 0.05 - Only 25% of social psych effects replicated vs 50% of cognitive psych effects - If the original effects were true, a minimum replication rate of 89% was expected - Among the studies that did replicate, the effect sizes decreased by approx. half compared to originals Event 8 - 1500 scientists lift the lid on reproducibility - 2016: Baker surveys 1500 scientists across scientific fields - 90% of respondents (chemistry, medicine, physics, engineering) agreed there was a crisis - 40% of scientists struggled to reproduce their own findings - 60% struggled to reproduce other’s findings - Over 85% of researchers in the field of chemistry failed to reproduce someone else’s results What does ‘failure to replicate’ mean? There are many reasons a replication attempt might fail + it is really difficult to determine the exact explanation A failed replication might suggest the original study was a false positive - detected evidence purely by chance - This is very likely considering older studies used very small sample sizes = low statistical power The replication attempt may have been a false negative (Type II error) - rejected evidence for an effect when it does exist - Might be due to a critical difference between original study + replication attempt - Might be due to methodological confounds, insufficient statistical power, potential moderating variables making the effect bigger/smaller context depending Are we really in a crisis? Redish et al. (2018) - not being able to replicate a study’s findings is not necessarily a failure/crisis, but opens doors to the underlying factors + limitations of an existing phenomenon - It is not an issue of ‘bad science’, but science needs time to reconcile conflicting findings Other researchers have renamed the crisis the ‘credibility’ and ‘transparency’ revolution - Highlights improvements in research (e.g., replication is essential, transparency is key) - Renewed focus on self-correction Chapter 3 - Causes of the Crisis Academic incentives reward quantity over quality - Scientists are supposed to be objective, rigorous, rational, and dispassionate advocates of knowledge - they are also human - with egos, career goals, and mortgages to pay - In addition to teaching + administrative duties = researchers need to publish a certain number of papers in “top tier” journals + attract large amounts of funding to build/sustain their research groups - Academic incentive structure - leads to a ‘publish or perish’ mentality (emphasize academic outputs for a successful career) - Creates a paradox between what is good for the scientist and what is good for science - Intrinsic motivations to carry out the best science < extrinsic motivations centered on quantity over quality - Baker (2016) - over 60% of researchers blame the replication crisis on pressure to publish - Frith (2020) - slow science - a refocus on quality over quantity, which would also drive down feelings of competition Bias, bias, and more bias Cognitive Biases - change + shape what we see and how we see it - Confirmation bias - tendency to search for or interpret information in a manner that supports our prior beliefs - People will think ‘what went wrong’ as opposed to ‘what bias or experimental error could have caused a false positive finding?’ - Apophenia - our natural predisposition to notice patterns in random data + our preference for positive/negative findings - This may result in searching for effects that might not exist - Hindsight bias – the tendency to see an event or finding as predictable only after it has occurred (e.g., Bem’s experiments on precognition) - Lead researchers to write up findings as though they ‘knew it all along’ = inaccurate representation of findings Publication Bias - the failure to publish research based on the direction or strength of the finding Biases are reinforced by what kind of research makes it into academic journals - Many peer reviewers + journal editors favor novel studies with positive findings over replication studies or null findings - Rationale: profitable journals want people to read their contents + get excited - File drawer problem - studies with null or inconclusive results are never published + have no findable documentation Traditional peer review process for publishing a journal article “Prejudice against the null hypothesis” = a body of literature that is: - Unrepresentative of the true number of completed theories - ‘Undead’ theories - popular, but have little basis in fact - Biased effect size estimates in meta-analyses Fanelli (2010) - 91.5% of psychology + psychiatry articles presented positive results - 5x higher than articles in space science (literal rocket scientists) Scheel (2021) - 96% of psychology articles reported positive results Why is this a problem? - Sample sizes in psychology are too low to detect typical effect sizes - This means psychologists are always testing true hypotheses OR something is wrong Citation bias - tendency to disproportionately cite positive vs null results - Common for failed replications - original articles continue to pick up many citations while their replication attempts are left under-recognized Psychology as an “aversion to null results” - distracting from the emphasis on falsification Questionable Research Practices (QRPs) Immense pressure to publish + academic incentive structures + publication bias = overlook the scientific method and work in ways that are detrimental - Questionable Research Practices - range of behaviors that researchers engage in to either intentionally or unintentionally distort their findings P-hacking or researcher degrees of freedom - describe the way in which a researcher can obtain a statistically significant result - Removing outliers after looking at their effect on the data - Measuring the same DV in several ways + only reporting the one that ‘worked’ - Running multiple unplanned analyses - ‘garden of forking paths’ - Optional stopping - where a researcher repeatedly analyzes their data during ongoing data collection + purposefully decided to stop when their p-value reaches a specific threshold (e.g., <.05) - Creates a ‘dance of the p-values’ = estimates of statistical significance become unreliable - NOT considered a QRP if specified in advance + resulting p-value is appropriately corrected (i.e., sequential sampling) Questionable because they are enacted AFTER looking at data, not a priori (before the fact) Common QRPs Hypothesizing After Results are Known (HARKing) - When a researcher changes their experimental hypothesis after looking at the direction of their results - Problem: results in exploratory research being presented as confirmatory - researchers who engage in HARKing always confirm their hypotheses with their results - Creates theories that are hard to eradicate + lead to unreplicable findings = lead other researchers to a dead end - Rubin (2017) - 43% of researchers admitted to having engaged in HARKing - Butler (2017) - QRPs in business studies were themed around playing with numbers, playing with models, and playing with hypotheses - Ironic paradox: to live up to the image of being a pure science, researchers find themselves committing impure actions QRPs are used by undergraduate students in their research - Krishna and Peter (2018) - students mostly engaged in: - Selective reporting - 28% - Excluding data after looking at the results - 15% - HARKing - 15% - This was predicted by their endorsement of QRPs + their perceived supervisors’ attitudes toward these practices - Motivation to write a good dissertation = negatively predicted use of QRPs - Students do not face the same pressures that their supervisors do - O’Boyle (2017) - from dissertation to journal article - the amount of non-significant results decreased, while statistically significant results increased - Researchers engage in QRPs to increase chances of publication = achievement goals predict QRP engagement Why are these questionable and not fraud? - Many researchers were not aware of the substantial impact QRPs could have on their data - Many practices were commonplace Statistically Significant p-values Null Hypothesis Statistical Testing (NHST) - frequentist approach used to test the probability that an observed effect significantly differs from the null hypothesis of no effect - Derived from the p-value - probability of the observed data occurring under the assumption that the null is true - p <.05 = statistically significant Problem - p-values have become the currency for publication Just because a p-value is statistically significant does not mean the hypothesis is true - Lindley’s paradox - highlights the arbitrary nature of the p <.05 threshold - in very large sample sizes, p-values around the.05 region can indicate support for the null hypothesis - Researchers can use QRPs to get a p-value of.05 = false positives - Some journals have banned p-values from their journals completely - Benjamin (2018) - argues a p-value of.05 is too low + a causal factor in studies not replicating - suggests lowering to p <.005 - Other researchers recommend reporting p-values alongside effect sizes and confidence intervals - Others have suggested researchers must justify the p-value they want to use A history of (too) small sample sizes Psychology has historically relied on small sample sizes that allowed researchers to maximize quantity at the cost of quality - Smaldina and McElreath (2016) = ‘natural selection of bad science’ - powerful incentives actively encourage + reward poor research design Just because an effect is significant with a small sample size does NOT mean it will be significant with a larger sample - Small sample sizes may lack statistical power - the long-run probability that a statistical test correctly rejects the null hypothesis if the alternative hypothesis is true - Higher % = greater power - Allows a researcher to balance Type I (false positive) and Type II (false negative) error rates - usually set to a minimum of 80% in psychology - 80% chance of detecting an effect if it exists + 20% of being a false negative To calculate statistical power, you need: 1. Effect size of interest 2. Significance criterion 3. Planned sample size Note: if you have 2 of these things, you can calculate the third (e.g., using effect size and significance to determine sample size needed) Many older studies in psychology have no mention of statistical power = inflated effect sizes - E.g., ego depletion - meta-analyses finding effect sizes of d =.62, while replication attempts found effects at least 6x smaller (d =.04 -.10) - Effect sizes in Open Science Collaboration = half the size of original studies Low statistical power undermines the purpose of research: - Reducing the chance of detecting a true effect when it exists - Reducing the likelihood that a statistically significant result actually reflects a true effect Note: just because a sample size is small, does NOT mean it lacks statistical power - It depends on the effect size of interest - If a researcher expects a large effect size = a smaller sample size might be sufficiently powered - Statistical power depends on the significance criterion, the effect size of interest, and the sample size used for a specific analysis Measurement Schemsurement In order to measure something = you must be able to define the construct - E.g., using intelligence tests to measure IQ - How do we know that IQ provides a good proxy for intelligence? Assess construct validity Construct validity - whether the measure ‘behaves’ in a way consistent with theoretical hypotheses - Some researchers argue an overlooked explanation of the replication crisis is our measures lacking construct validity - Flake (2017) - 35 articles in a prestigious journal - Of 433 unique measurement scales = 40% had no source, only 7% states that had been developed by the authors - 19% of those that had a reference were adapted in some way compared to the original = psychometric properties were unknown - Also found evidence of Questionable Measurement Practices (QMPs) - nondisclosure of validity when such estimates are found to be unsatisfactory Internal validity - how a study establishes a cause-and-effect relationship between the IV and DV - A replication attempt may fail if the original study suffered from a threat to validity that causes a spurious effect (i.e., high internal validity of the replication attempt) - OR if the replication researchers introduce something that did not exist in the original study External validity - whether the results of an original study can be generalized to other contexts/populations - Influence replication attempts if it is conducted on a different population from the original - OR if study materials are translated from a different languages + participants don’t understand requirements - E.g., Facial feedback hypothesis (pen-in-mouth task) - Strack et al. (1988) - participants reported feeling more amused when smiling vs pouting - Wagenmakers et al. (2016) - large scale replication, found no evidence of the effect - Strack (2016) - countered by presenting numerous problems pointing to threats to external validity (e.g., unlike the original study, participants in the replication were being recorded, which could influence self-consciousness) - Marsh et al. (2019) = found evidence for facial feedback effect when there was no video camera - Noah et al. (2018) = found that the effect diminished when participants were recorded - Coles et al. (2022) - adversarial collaboration (people from opposing viewpoints work together) found that emotion could be amplified by facial mimicry, but the pen-in-mouth task was inconclusive - Possibly because it could not reliably produce prototypical emotional facial expressions Measurement schemsurement - describes the lack of consideration for measurement validity in psychological science - If a study’s measures are not valid, than the findings + conclusions drawn from them cannot be either - When they are replicated = destined to fail before they begin Favoring novelty over replication Emphasis on positive + novel results = replications + negative results are a rarity Antonakis (2017) suggests that science suffers from: - ‘significosis’ - a disproportionate focus on significant findings - ‘neophilia’ - an extreme emphasis on novelty This idea is not new: - Sterling (1959) - researchers could repeatedly test a hypothesis in many different experiments until eventually, by chance = significant effect - Tukey (1969) - ‘confirmation comes from repetition, any attempt to avoid this statement leads to failure, and more probably to destruction.’ Makel et al. (2012) - out of 500 articles from top-tier journals, only 1.07% were replications - In 2014 - out of 100 education journals, only 0.13% were replications - In both psychology + education research = majority of replications tended to be ‘successful’ = publication bias Novelty over replication can also be seen in student research projects - E.g., undergraduate theses - often undertaken alone, minimal supervision, no funding, and very strict deadlines - Suffer the same issues as wider literature: underpowered, novel studies that yield a high rate of false positives - If these are selectively published = students are rewarded for being lucky rather than right Science has not been self-correcting Academic incentives + reputations = scientists are worried about correcting their mistakes - The time needed to continuously evaluate + correct past work becomes a barrier to new research/work Registered Replication Report of Srull and Wyer (1979) by McCarthy et al. (2018) - Original study - priming participants with aggression-related stimuli caused them to interpret ambiguous behavior as hostile - The effect size of the replication was considered negligible (d =.06) - One reason: statistics in the original study may have been incorrectly reported van der Zee et al. (2017) - describe ‘statistical heartburn’ attempting to reanalyze data for 4 articles from Cornell Food Lab - Identified over 150 inconsistencies in data reporting ‘Error detection’ researchers - receiving backlash on social media - Suggests that this work makes researchers uncomfortable Rather than a publication marking the ‘end’ of a particular project = researchers have a responsibility to always be on the lookout for errors - Intellectual humility in science - researchers ‘own’ the limitations of their work indefinitely - Corrections should be encouraged, articles should be retracted or ‘loss of confidence’ statements given Closed Science Major barrier to replication + reproducibility = lack of transparency + detail in previous studies - Researchers have approached scientific investigation as though it were a secret recipe Example of research practices under a system of ‘closed’ science: Under-reporting of methodological details in published articles - Research involves many decisions, and unless explained clearly, no one will know what the researcher did - E.g., estimates that between only 1 - 14 % of research articles share their materials Under-reporting generalizes to the availability of data and reporting of analyses - Decisions made about how to look at outliers, what to do if data does not meet certain assumptions, how to correct for multiple analyses - If researchers do not transparently report these plans = results can differ dramatically + will not reproduce - Unplanned decisions (made after viewing the data) = garden of forking paths ‘Many Analysts’ - Silberzahn (2018) - 61 analysts presented with the same question - 69% found a significant positive effect, 31% found a non-significant effect - None of the teams used the same analysis OR same variables Example of the garden of forking paths: Making methodological and analytical decisions based on RESULTS - Can lead to false positives Chapter 4 - Crisis Averted! Open Science Reform Open Science - umbrella term reflecting the idea that knowledge of all kinds should be openly accessible, transparent, rigorous, reproducible, and replicable. - Scrutinizing whose voices are represented in science to confront sources of bias - Used interchangeably with open research (all disciplines) and open scholarship (teaching and pedagogy) - Goal: to improve science Open research is facilitated by several processes: Preprints Paywall - people can only read the article if they are affiliated with an institution that funds a license OR they pay to read it themselves Open Access - unrestricted public availability of research products - authors pay a large amount of money to a journal ‘Article Processing Charge (APC)’ - Researchers often don’t have money to cover this cost There is a practice that bypasses the payment of open access licenses, allowing public sharing of research = preprint - a scientific document made available freely and legally outside of traditional publishers through an online repository Preprint - unpublished work that has not undergone peer review Postprint - final version of the work that will be published in an academic outlet Many preprint repositories have been around for decades, but are only now being adopted in psychology - E.g., arXiv (17 years old) - 2 million preprints in physics, math, and compsci - PsyArXiv - founded in 2016 Preprints support the notion that science is collectively owned + funded by the general public Researcher benefits of preprints: - Allowing them to gain feedback pre-publication, disseminate their research more quickly - A lot of time can pass between article submission and its actual publication - additional studies can be published, literature is outdated - Metrics from preprints + metrics from final published article = increase number of citations gained by up to 40% - Aids collaboration between researchers to comment on each others work Replication crisis benefits of preprints: - Other readers can evaluate the claims + spot any errors = aid self-correction because errors are identified before it's too late - Publication bias - allows researchers to share outputs that would be put in the file-drawer - Increase reliability of meta-analyses - Offer useful information to researchers who want to pursue a new line of research - Transparency - repositories have inbuilt version control = readers can see any changes to the original document - Becomes helpful after article has undergone many rounds of peer review - Reproducibility - a barrier to methodological/analytical transparency due to strict word count guidelines from journals - Using the internet as the primary source of articles removes printing/shipping costs - Freely available preprint servers allow authors to submit multiple documents Preregistration Need a practice that can distinguish between prediction and postdiction: Preregistration - the initiation of a time-stamped, non editable document of the research questions, hypotheses, and analysis plans before data collection and/or analysis. - Several pre registration registries with their own templates - structured workflow that asks researchers to answer several questions - Researchers are asked to clearly distinguish between confirmatory and exploratory analyses - Used for quantitative, qualitative, and designs that utilize secondary data clinicaltrials.gov - implemented in 2000: US Congress passes a law mandating all experimental drug trials be accessible to the general public - 2005: many medical journals require trial registration as a prerequisite for publication - Benefits: meeting ethical obligations, enhancing patient access to enrollment, preventing the duplication of trials for unsafe/ineffective treatments Center for Open Science (2013) - Open Science Framework (OSF) - Only 38 pre registrations on OSF in 2012 is now over 100,000 as of 2022 Benefits of pre registration: - Pre Registration is proposed to protect researchers against their cognitive biases - Helps restrain researcher degrees of freedom + mitigate QRPs (e.g., selective reporting, p-hacking) or make them more detectable - Prevent publication bias by making research more discoverable - Added transparency can increase the credibility of research - Allow others to evaluate whether a hypothesis can be falsified van den Akker (2021) - percentage of positive results reported from pre registered studies is around 65% - Compared to approx. 90% in standard (not pre registered) literature Kaplan and Irvin (2015) - before clinical trials were introduced, 57% reported the intervention was ineffective, which dropped to 8% after registration was implemented Limitations of pre registration: - Many pre registrations lack detail + specificity = difficult to analyze their effectiveness - Discrepancies between pre registrations and final publications are often undisclosed - May be due to misaligned incentives for pre registering OR lack of sufficient training - Without a standardized way of reporting discrepancies + many peer reviewers do not check pre registrations = introduce bias into research process Registered Reports We need a strategy that can ensure research is published regardless of the results Registered Reports - Moves peer review to a much earlier stage in the research process + allows researchers to embed study pre-registration within the article Traditional Publishing Model: - Conduct study - Write report - note pre-registration in the method section + provide a link to it - Submit article to journal = accepted or rejected Registered Reports - splits peer review process into 2 stages: Works on the premise that replicability + reproducibility need to focus on the research process itself NOT the outcomes - Researchers focus their efforts on designing important + methodologically sound research Stage 1 - Submit a proposal that includes an introduction, method, and analysis plan - Peer reviewers provide feedback BEFORE data collection/analysis starts - Researchers can be offered ‘in principle acceptance’ (IPA) - Journal commits to publishing the research regardless of the results - The study becomes pre registered automatically Stage 2 - Manuscript which knots the results + discussion section to the original proposal - Reviewers make sure researchers have stuck to their plans (or deviations were approved + reported) and results have been interpreted appropriately - Reviewers are not allowed to voice any concerns about any part of Stage 1 + not allowed to get upset about results The idea of Registered Reports were proposed in 1966 - This idea was formalized in 1976 by Martin Johnson in the European Journal of Parapsychology, but retired in 1992 + remained unknown Idea was resurrected in 2012 - Proposed by the editors of Cortex, Perspectives on Psychological Science, and formally offered in 2013 to these and Social Psychology Peer Community in Registered Reports - launched in April 2021 - Facilitate the peer review of Registered Report preprints - Removes the need to journals to envision a completely new way of publishing - Allows researchers to schedule the peer review process to overcome time constraints with Stage 1 acceptance Benefits of Registered Reports - Underpowered Research - Registered Reports have higher standards for acceptance than other journals, specifying high-powered designs + appropriate statistical analyses - Reduces chance of Type I and II errors - Take focus away from p-values - researchers are rewarded for methodology over results + asked to implement additional tests to assess the level of support for their hypotheses - Increase replicability + reproducibility - vetted protocols contain sufficient detail - Detaching authors from their results should limit QRPs - Make science more self-correcting - reviewers actually evaluate pre registration in Stage 1 + evaluate Stage 2 manuscript = spot deviation or errors before appearing in published literature - Mitigate publication bias + file drawer problem - positive, null, and inconclusive results are published - Incentivize replication - with a special category: Registered Replication Reports - Combine the results of numerous replications across independent labs Empirical Evidence for Registered Reports - Scheel (2021) - 95% of standard literature found support for a primary hypothesis vs 44% in Registered Reports - Note: researchers may be using Registered Reports to test riskier hypotheses - Will Registered Reports be less cited + judged as less important/creative? - Preliminary analysis of 70 Registered Reports found they are cited the same or at a slightly higher rate than traditional articles - Peer reviewers judge them as being higher in rigor, quality and detail, and comparable in creativity/importance - Registered Reports are more reproducible than standard articles - e.g., Obels (2020) of 62 reports, 58% were able to reproduce Transparency and Openness Promotion (TOP) guidelines - set of standards to aid the transparency + reproducibility of published research Open materials, code and data - Open materials - the public sharing of inputs to a research study, such as questionnaire items, stimulus materials, or experiment scripts - Open code - making the computer code, used in experimental programming and/or data analysis, freely and publicly available. - Open data - refers to making anonymized research data - the output - freely and publicly accessible for use by others without restrictions. All of these together can help make research ‘FAIR’ - Findable - Accessible - Interoperable - Reusable Note: making research ‘as open as possible, as closed as necessary’ - Following ethical guidelines, data protection laws, copyrights, etc. Benefits of open materials, code, and data: - Allow other researchers to ‘look under the hood’ of a research study - deter some QRPs and fraud, or make both more detectable - Eliminate closed science - foster comprehensive reporting of methodology + analyses - Make science more self-correcting - allow errors to be identified - Reproducibility + replicability - future-proof research with detailed documentation to be used in years to come Researcher benefits: - Associated with more citations - Lead to collaboration + public impact Researchers may perceive time as a large barrier to implementing these practices - we need to adopt slow science to ensure replicability, reproducibility, and credibility of the discipline Meta-research - the study of research itself - methods, reporting, reproducibility, evaluation, and incentives. - Example - informing us of how researchers have engaged in QRPs driven by incentive structures to provide one explanation for the replication crisis - Example - evaluating the effectiveness of open science initiatives such as Registered Reports and pre registration Helps create higher standards by developing + providing empirical evidence for the proposed reform initiatives. Big team science Big team science - open, large-scale collaboration between researchers, who work together to solve fundamental research questions + pool resources across different labs, institutions, disciplines, cultures and continents - E.g., Many Labs - 50 authors, 13 psychological effects, 36 independent samples, 6344 participants - E.g., Reproducibility Project: Psychology - 270 authors Psychological Science Accelerator (PSA) - created to facilitate generalizable + reproducible research to bridge the gap between truth about human behavior and our current understanding - 1400 researchers - 300 labs - 82 countries - E.g., effectiveness of emotion regulation - A cognitive reappraisal strategy in reducing negative emotions associated with COVID-19 in over 20,000 participants across 87 countries Students typically undertake individual research projects with limited resources - Solution: adopt a big team science approach to research training + education - E.g., Collaborative Replications and Education Project (CREP) - Undergrad students are taught best practices to conduct high-quality, direct replications of psychological research Benefits of Big Team Science: - Pooling available resources allows researchers to undertake more rigorous + reliable larger-scale studies than normally achievable - Overcome low statistical power = detect more reliable effects, reduce research waste - Reduce QRPs - embeds open science practices from the beginning e.g., pre registration and/or Registered Reports to improve transparency - Replication and Reproducibility - standardization of methods + the need for clear communication of research procedures across labs - ALSO through incentivizing replication - Self-correcting + future proof - adopting open materials, code, and data Most powerful benefit (unique from all other open science practices): - Ability to increase diversity + representation of participants and researchers - E.g., consortium approach to the undergraduate dissertation - Students with a range of diverse skills contribute to the project - Increases variety of expertise, voices, and ideas in science - help unearth ‘hidden curriculum’ around research (e.g., learning about publication process), enable more students to try out research - Science can become more equitable - resources can be shared Example - consortium approach to student research perspectives This also helps students who do not wish to pursue research - ‘Open scholarship’ = helps students to become consumers of research who evaluate sources critically + understand importance of transparency Open science communities Bottom-up, grassroots learning groups that discuss open science in an accessible + constructive manner - e.g., ReproducibiliTEA journal clubs - 140 institutions in 26 countries - Goal: creating a supportive + constructive community for researchers and students to keep up to date with research around reproducibility, research practice, social justice and inclusion, and other ideas for supporting science - e.g., Society for the Improvement of Psychological Science (SIPS) - Create a community for those wanting to improve methods + practices in psychology and beyond - Change incentive structures of academia = awards prizes to projects that improve research training + practices - Evaluate policies that aim to change research norms - e.g., Framework for Open and Reproducible Research Training (FORRT) - Goal: embed the teaching of open + reproducible practices into higher education - Using big team science approach - develops open educational resources (OERs) to reduce the labor associated with developing/implementing open scholarship content - Example - developed a bank of lesson plans, curated list of replications Challenges to open science 1. Overturn deep-rooted incentive structures in academic culture - Researchers need to be supported by their institutions, funders, publishers, and the government - E.g., badge system - developed by the Center for Open Science that attaches a visual icon to research articles to certify pre registration or sharing of its materials/data - E.g., some funders have mandated open access publications, recognize the benefits of preprints, partnered with journals to offer funding for Registered Reports 2. Ensuring equality, diversity, and representation - Open science needs to be about who is welcomed to the table - Address the historic lack of diversity and non-inclusive culture in research - i.e., psychological science was created for + drew on the experiences of white, affluent males - We need to diversify who defines open science and reorder traditional power dynamics - E.g., champion all voices equitably, consider the systemic marginalization of some individuals, work to dismantle pervasive hierarchies in academia Chapter 5 - A student’s guide to open science Bergnmann (2018) - see open science as a buffet - not learning all of these practices at once, but picking + choosing when you need them - Open science does not prescribe a set of rules, but is a collection of behaviors A recap of open science practices: Embedding open science in your research workflow: Platforms to facilitate open science: using the OSF Open Science Framework (OSF) - Developed by the Center for Open Science - goal is to increase openness, integrity and reproducibility in scholarly research - Users can upload preprints, initiate study pre registration and Registered Reports, share their materials, code, and data - Benefits: - Provides version control - Transparently documents project updates - Stores data under local legislation - Uses persistent + unique identifiers for citation Preprints Gold route - open access via a publisher, most still charging an Article Processing Charge (APC) Green route - authors self-archiving their work - Include preprint servers - allow researchers to upload work legally + for free - Kathawalla (2021) - article preprints are the lowest effort open science practice you can implement Decisions before you upload your work to a preprint server: 1. Choose a license for your work so others know how to use it - E.g., PsyArXiv - ‘CC0 1.0 Universal’ = public domain, ‘CC-BY’ = allows others to reuse your work as long as they give the original author credit 2. Check journal rules for ‘internet posting’ - Most publishers will let you post preprints before submission + final author version of the accepted article before it goes through proofing/editing - Most do not let you upload the publisher formatted version unless you’ve paid the APC for copyrights - Include information about the status of the preprint on the title page (e.g., submitted for publication) - allows people who discover your work to report on it appropriately 3. Check that your co-authors or supervisory team approve of the preprint before being submitted 4. Choose a preprint server that is hosted in a stable + public location (e.g., institutional or scholarly repository) Study preregistration Difference between pre registration vs submitting study to ethical review board = pre registration is uploaded to a verified repository (date stamped, permanent, and publically accessible) 1. Choose the most appropriate pre registration website based on the type of research you’ll be doing 2. Work through the template provided by the repository - for example: - Provide hypotheses, information about planned sample size + rules for stopping data collection, research materials, participant inclusion/exclusion criteria, data analysis strategy, etc. - Number each hypothesis + make sure they are not ambiguous - Check your analysis plan allows your to appropriately test these hypotheses - Be specific - Assume no prior knowledge of the reader - make descriptions very clear Imagine pre registration like a cake recipe - if one step is missing + a person tries to follow it, they will end up with a very different cake - You need to include every detail, including justifying your decisions Note: preregistration is a plan, not a prison - As you progress with your study, you may realize something isn’t working as planned + you have to change it = simply report it in your final manuscript (e.g., ‘deviations from pre registration’ table) Registered Reports Registered Reports are submitted for peer review before data collection and/or analysis - If the proposed study receives ‘in principle acceptance’ (IPA) = it is published regardless of its results - A reviewer cannot point out additional ‘flaws’ in the study upon viewing the results For researchers who want to pursue this publishing format formally: 1. Think carefully about the specificity of your proposed research questions + hypotheses, and then start to build robust methods + analyses that map onto these 2. Think about the feasibility of your sampling plan - including time or resource restraints 3. Think deeply about the validity of the proposed methods or analyses before submission, which can be guided by pilot data - Check that there are precise links between the research questions, hypotheses, sampling/analyses plants, and outline your interpretations in the case of different outcomes 4. Ensure you have the appropriate data quality checks (e.g., attention/manipulation checks) One of the main barriers = time - Registered Reports are arguably more efficient than pre registration b/c you’ve already written the intro + methods and have them approved - By gaining IPA for the study = prevent the need for multiple journal submissions (typical for traditional articles when reviewers decide they don’t like the results) - ALSO Peer Community in Registered Reports offers a scheduled review track - researchers submit a one-page ‘snapshot’ + request a specific time period for a review to be conducted Open materials, code, and data Open Materials - If you are using unoriginal materials = check copyrights before sharing - E.g., some materials are only available via researcher requests from original authors - If the materials cannot be shared for a valid reason - upload a ‘README’ file with an explanation + as much information as you can provide - If you are using your own materials = can the end user access them - E.g., is your script programmed in an open-source software (free) or a proprietary one (paid) - Release your materials under a Creative Commons license - allows others to reuse your work, whole ensuring you retain all the rights for it - Always adhere to ethical principles when sharing materials - E.g., if a person is identifiable in experimental stimuli, ensure everyone has consented to how the information will be used - ALSO explain how materials will be shared in your ethics application Open Code - Refers to code used in experimental scripts AND code used to analyze your data - E.g., SPSS is underpinned by computer code that can be shared (syntax) - this can be exported into text or Word files to be shared with others Open Data - Ethical principles - Include information in your ethics application about how data will be stored and shared, what will happen if a participant chooses to withdraw - Participant-facing ethical materials include information about open data - so participants can provide informed consent - Check where you are storing your data + if it adheres to data protection laws - What data should be shared? - Raw data (originally recorded) vs processed data (extracted/compiled to provide input for analyses) - Ensure participants cannot be re-identified via their shared data - ‘is my data anonymized to protect + preserve participants’ privacy?’ - It is almost always possible to share anonymized processed data - e.g., redact identifying information, such as demographics - ALSO creating a synthetic data set that de-identifies participants, while preserving statistical properties - Data management + organization - FAIR principles in mind when sharing data - Findable - use a recognized data archive in your field, provide a direct link to it in your research - Accessible - openly available, aim to convert proprietary files into open-source versions - Interoperable - is your data readable by both humans and machines? - Appropriate file name, well structured - Data codebook - data dictionary, provides a list + detailed annotation of all variables in the data set, what they refer to, how they were compiled - Reusable - attach a license to your data so others know how it can be reused Psychology Moving Forward Although conversations about replication concerns and open science are sweeping through the research community = they are lost in the teaching of psychology - Robust science requires robust training