Research Design Notes PDF
Document Details
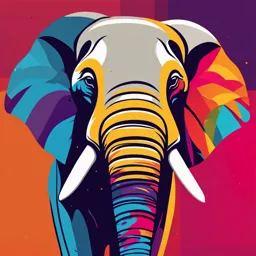
Uploaded by ResponsiveArcticTundra7823
Stellenbosch University
K Fouché
Tags
Related
- Field Methods in Psychology PDF
- Field Methods in Psychology PDF
- Practical Research 2 PDF
- Quantitative Notes - Research Methods in Psychology II - University of Cape Town PDF
- Psychology 243 Notes - Understanding Quantitative & Qualitative Research
- Psychology 2103 Quantitative Research Methods Fall 2024 PDF
Summary
This document outlines the basics of research design, including the research process, developing a research question, running a psychological research study, open science practices, measuring variables, confounding variables, different research designs (experimental, correlational, qualitative), and developing hypotheses. It covers various aspects of quantitative and qualitative research methods.
Full Transcript
K Fouché 25953621 Chapter 1: The basics of research design The research process ❖ When conducting a research project, be as methodological and as structured as possible. Developing a research question - The 1st thing you must do. W...
K Fouché 25953621 Chapter 1: The basics of research design The research process ❖ When conducting a research project, be as methodological and as structured as possible. Developing a research question - The 1st thing you must do. What is it you want to find out? - Must be very familiar with previous research. - Read the most recently published research → if not, you’ll have gaps in your knowledge. - Think critically about the research you review. - Identify and consider the limitations of previous research and your research. - Justify why your research is necessary. - When you have a clear idea of the previous research and your research: o create a hypothesis – your predicted findings based on the research you reviewed (only with quantitative studies). Justified by the previous research → consistent with previous research findings. Justified by your critique of the previous research → when you predict contrary research findings. Running a psychological research study - Apply for ethical approval when your research aims are clearly defined. - After ethical approval is successful → run your study and collect data. - After data is collected → use the appropriate statistical tool to discover whether the data supports the hypothesis or not. - The final thing to do is to write up the study. Open science and psychological research - Open science practices improve the quality and rigour of scientific research. o Starting point – the replication crisis (research findings are failing to be replicated). o Why should we be able to replicate findings? So that other researchers can run studies and find the same results. - Some researchers adopted questionable research practices o There’s a huge pressure on researchers to publish and report statistically significant results. - 3 problematic practices: Sample sizes → Very small = low power. HARKing → Developing a hypothesis after analysing the data/research is completed. → Basing the hypothesis on findings (analysed data). → Comes from = Hypothesizing After the Results are Known. P-hacking → Hacking data to get the p-value that indicated a significant result. → Analysing data repeatedly in different ways until a statistically significant finding is found. - The outcome of questionable research practices: o File drawer problem – studies with robust methodologies that weren’t replicating previous findings weren’t published. K Fouché 25953621 - Pre-registration – submitting a research proposal to the journal with a clear review of the previous literature and the hypothesis, methods, and analysis strategy fully planned out. o If research is accepted by the journal → research will be published whether the findings are significant or not. o Judgement of the quality of the research paper shifts to the clarity and rigour of the design and away from the findings. o Increases transparency in scientific research. - Key motivation in the open science movement → increasing transparency around scientific research. o Researchers share their data and analysis tools (may expose p-hacking). o Sharing research papers before they are submitted or published (researchers receive feedback and we see research that would previously have gone into the file drawer). Measuring variables in psychological research - Variable – something you can measure and manipulate that provides data for you to analyse. o Examples of manipulating a variable: Manipulate the length of time given to participants to learn a list. Showing participants positive or negative emotional stimuli. - Before measuring the variables → operationalize all variables you intend to measure. o Operationalize – clearly define what you intend to measure. o The same variable can be operationalized in different ways. - Collected data in qualitative data → mainly words. - Collected data in quantitative data → numeric. o 4 types of quantitative data: Nominal data Nominates people into categories. (Also called categorical data) No determined order between categories – no logical order/hierarchy. Distance between categories is always the same. Discrete (clear boundary) and mutually exclusive – can only belong to 1 category at a time. Extensive – includes all possible categories for the variable. Ordinal data Continuous, exists on a continuum. Includes all the categories of nominal data, but it is hierarchal (has an order). Distance between categories isn’t always the same (different distances). Data may exist in a defined order (1st, 2nd, 3rd...) but distance between them may differ (95%, 90%, 79%). Thus, there can be big and small gaps between data points. Interval data Continuous, exists on a continuum BUT always the same distance between data. Thus → includes the aspects of nominal and ordinal data but there are equal differences between categories. Example → temperature (1C is the same amount of temperature, regardless of where you look on the scale). Negative values are possible (-5C). K Fouché 25953621 Ratio data Continuous, exists on a continuum. (Difficult to find this type of data in social Distance between categories is always the same. sciences) No negative values. Most frequently collected type of data. Thus → includes the aspects of nominal, ordinal and interval data AND absolute zero (anxiety levels may be low but you can’t live without it). Examples → age prenatal = absolute 0, intelligence, reaction times, accuracy. Confounding variables in psychological research - It’s unlikely that chosen variables can account for the whole phenomenon you’re investigating. - Confounding / control variables / covariables – unmeasured variables that may influence the findings of a research study. Different designs for different research questions 1) The basics of the experimental design (quantitative) - Measure quantitative variables and look at differences between separate groups of participants or conditions. - More than 1 independent variable can be manipulated within an experimental design. - Independent variable – defines the group that you want to compare. o Always a nominal variable as it defines belonging to a certain group o What you’ll be COMPARING. o Example → dividing participants into separate groups based on whether they like chocolate or not because you’re interested in how happiness differs depending on dietary choices. - Dependent variable – always continuous variables that vary according to the independent variable. o What you’ll be MEASURING. o Example → after identifying the independent variables, you give participants a questionnaire to measure their happiness. K Fouché 25953621 2) The basics of the correlational design (quantitative) - The aim is to look at the relationship between two continuous variables. - Both variables must be continuous (If scores on variable 1 increase, scores on variable 2 increase/decrease). - There are 3 types of correlations: Positive correlation → When 1 variable increases, the 2nd variable increases. → 1 variable causes an increase in the other variable. Negative correlation → When 1 variable increases, the 2nd variable decreases. → 1 variable causes a decrease in the other variable. No correlation → There is no relationship between the variables. → The variables do not affect each other. 3) The basics of the qualitative design - The collected data is usually text-based. - Data is collected through questionaries, interviews, or focus groups with participants OR from existing sources such as online discussion forums or media reports. - The overall aim of analysing qualitative data: o Identifying the key themes or categories within the dataset. Developing a hypothesis in quantitative research - Hypothesis will differ depending on whether you will conduct an experimental or correlational study. - The types of hypotheses: Null hypothesis → Predicts that no effects will occur. Alternative/experimental hypothesis → Predicts that effects will be found. → Tends to be the research question that you ask. - Different types of alternative hypotheses: One-tailed hypothesis → Predicts the direction of your finding/you determine the direction of the effect. → Experimental example = clearly states which group has a higher happiness score. → Correlational example = predicts a relationship between 2 variables and specifies whether the relationship is positive or negative. Two-tailed hypothesis → Predicts that an effect occurs but doesn’t specify the direction of the finding. K Fouché 25953621 → Experimental example = predicts that the 2 groups differ in happiness but doesn’t specify what group has the highest happiness score. → Correlational example = predicts a relationship between 2 variables but doesn’t specify whether the relationship is positive or negative. - In an experimental research design → frame the hypothesis around the differences you may or may not find. - In a correlational research design → frame the hypothesis around the relationship between the 2 variables. Validity and reliability in psychological research - Research won’t be valid if it isn’t reliable. - THUS → “You can’t have validity if you don’t have reliability”. - Reliability is necessary but not sufficient → something must be reliable before it can be valid; if it isn’t reliable, it isn’t valid. - Both validity and reliability are contextual → something valid and reliable in Amerika won't necessarily be valid and reliable in South Africa. Validity When you measure what you want to measure. Reliability Consistently measuring what you want to measure. Something is reliable when it consistently does what it is supposed to do and what you expect it to do. Reliability is consistency! Different types of validity in psychological research 1) Construct validity - Ensuring that you are measuring what you think you are measuring. - operationalize your variables to ensure that it is clearly defined. - Different aspects of construct validity (with measuring one’s numeracy as an example): Content validity Are you measuring all possible aspects of it? Measuring all possible aspects of a variable. Example = Are you measuring all types of numeracy or only one part of it? Convergent validity Measuring variables that are correlated with our measure. Example = asking students for their maths grades. You might expect it to be positively correlated with numeracy if the numeracy measure is valid. Divergent validity Measuring variables that aren’t expected to be correlated with our measure. K Fouché 25953621 Very difficult to measure in practice, thus less considered as content and convergent validity. Example = asking students for their English grades when measuring numeracy. If it is highly correlated, it is rather a measure of overall academic ability than numeracy. 2) Internal validity - Is the study designed so other factors can’t explain the results? - Focused on how you design your study to ensure that there aren’t any sources of bias that might influence your findings. - Example = when measuring the mathematical skills of SU students, the design and findings of the study won't be valid if most of the sample includes students from the science faculty (sample bias). 3) External validity - You must be able to extrapolate the findings of the research to the wider population, findings shouldn’t only be relatable/applicable to the smaller sample. - It must be possible to generalize the findings of the research. - Easiest demonstration → different researchers in different labs replicating your design and finding the same results. 4) Ecological validity - Applicable in studies where the design attempts to simulate a real-world scenario. - More specific form of external validity where the aim is to extrapolate from the lab to the real world. - Designing the study to be as close to the actual scenario as possible. Different types of reliability in psychological research 1) Inter-rater consistency - When multiple people are coding and scoring data, they must do it in the same way. - Are all the items measuring the same thing? - If people code data differently → no reliability between the different codes. - Particularly relevant for qualitative research. 2) Test-retest consistency - When you measure the same variable at 2 different time points, the score must be very similar. - Example = when taking a numeracy test twice, 5 days apart, the results must be similar. 3) Internal consistency - Measures with multiple data points that all contribute to measuring 1 thing must give similar scores. - Different items/data points within a measure must be responded to similarly. - concerns about internal consistency arise particularly in questionnaires. Sampling, validity, and reliability - when conducting research, you choose a sample that will reflect the wider population. - Ways in which participants are recruited/selected can influence your ability to extrapolate your findings. - If the sample doesn’t reflect the wider population → findings aren’t valid and lacks reliability. K Fouché 25953621 - Ideal way of recruitment → random selection o Difficult to achieve, therefore most studies use: ▪ Volunteer sampling = participants respond to an advert and volunteer to participate. ▪ Opportunity sampling = researchers approach potential participants. ▪ When using volunteer and opportunity sampling → consider whether the sampling causes your findings to be less generalizable. Ethics in psychological research - All psychological research must adhere to the ethical guidelines of the British Psychological Society (BPS) or the American Psychological Association (APA), regardless of the chosen research design. - 5 types of ethics must be adhered to: 1) Informed consent and debriefing - Participants must give explicit consent to participate. o You get this by asking them to sign the consent form. ▪ But be aware of their willingness to participate and potential changes to this in the study. o Extremely important to ensure that their consent is fully informed → they must know what their participation will involve so that they can make an informed decision whether they want to participate or not. They must know the following: Roughly your expectations of them. Duration of the study. Location of the study. Potential risks to them. o Be careful of biasing participants’ performance by giving too much information of the study. - After the study is completed → debrief participants of the study’s purposes. o They must know the following: ▪ More information on what you asked them to do. ▪ Why you asked them to do it ▪ Your expected findings. ▪ Any potential ethical concerns with your study. o A debrief can be verbal but → it’s better to give a verbal debrief in addition to a written debrief. 2) Deception K Fouché 25953621 - Sometimes it is necessary but put as many safeguards in place as possible in your research design to protect participants. - When a well-considered debrief is invaluable (it might influence the findings and responses), do the following: o Explain to participants why deception is necessary. o Explain why the research is thought to be important enough to use of active deception. o Give advice if they experience lasting stress because of their participation. - There are 2 types of deception: Passive deception You don’t lie to participants but you don’t tell them about an aspect of your design in case it influences their responses. Example → when testing numeracy skills, I don’t tell participants that the questions get harder as they go on because I’m interested in when they decide to stop answering the questions. If I told them that it gets harder as they go on, it might’ve influenced their responses; thus, I didn’t include this aspect of the design in the informed consent. Active deception You outright lie about the aims of the study and what you expect the (Most of the time, this is where a well-considered participants to do. Example → I told participants that I’ll be testing their numeracy skills. While debrief might be invaluable) they are in the waiting room, one participant (that is an undercover researcher) collapses. The study doesn’t have anything to do with numeracy skills; I want to measure how people react in emergency situations. - Why would researchers actively deceive participants? o Hathorne effect → people act differently when they know they’re being watched. 3) Protecting participants from harm - Don’t expose participants to any type of physical or psychological harm. - If there’s any risk of harm → mention it in the participant information they read before consenting to participate in the study. - Participants may not realize the negative impact of their participation on them until later, therefore debriefing is essential in this situation. o Mention the following in the debriefing: ▪ Raise the potential lasting harm. ▪ Provide places participants can go to for support. 4) Right to withdraw - Participants have the right to withdraw at any time. o Make this clear in the consent form. - Inform participants how to indicate that they wish to withdraw and that they can withdraw without facing penalties or costs. - If you used any incentive for their participation, they must still receive it even if they withdrew early from the study. 5) Anonymity and confidentiality - Anonymity – any identifying information isn’t collected and stored with the data participants provide. When you have no way of telling who said what. - Confidentiality – when you must use identifying information, it must be securely stored and no one except the research team may access the data. When you can link a response to a certain person but don’t link it to protect the person’s confidentiality. K Fouché 25953621 - All collected personal data must be stored and handled according to the EU General Data Protection Regulation (GDPR). - The point of sharing data → others can replicate your analysis and try out other ways of analysing the data. o It isn’t necessary to share the entire dataset but only the variables used in the analysis. o No identifying information should be shared = easy to maintain anonymity. o If there’s a chance that the data will be shared → include it in the consent form Working with vulnerable groups - Examples of vulnerable groups include children or participants drawn from clinical populations. o In a school environment, you must make it very clear that the children can say no within this specific context (they are in a context where they must follow all rules and obey to the demands of adults). - Double-check each ethical standard and ensure that there are safeguards in place to protect participants. Applying for ethical approval - You require 3 documents when applying: o Participant information sheet o Consent form o Debriefing sheet - Submit these 3 documents for review. - Submit a summary of the rationale for your study and an outline of the methods to be used. - Sometimes → submit any materials that will be used (questionnaires, etc). - The chair of the committee will: o decide whether to approve the application o recommend revisions that must be made before ethical approval can be granted o reject the application. - When writing up a study, your work should be entirely your own. K Fouché 25953621 Writing about psychological research - Writing up a research report is the final part of the research process. - The structure and style → very standardized, determined by the standards of the APA. Title Should be concise and specific as possible to give readers a clear idea of the aims of the study 10 – 15 words. Abstract Summary of the entire paper. Includes an overview of the rationale, key methodological points, main findings, and brief mention of the implications. 1 – 2 sentences to summarize each of the 4 key sections of the research report. 150 – 250 words. Introduction Where the previous relevant research is reviewed and used to justify the need for your research. Funnel-shaped: o start with a broad introductory paragraph to set the context and clarify the need for your research. o Work through the previous research, getting narrower and more focused as you work towards explaining your research design. o Show your critical thinking skills. o The final paragraph should provide the rationale and present your hypothesis. Methods 4 subsections: o Participants (whom you are studying). o Materials (stimuli/questionnaires/instruments used). o Procedure (what you got participants to do). o Design and analysis (the type of research design used and how the data will be analysed). When writing this part → think of what other researchers must know to replicate your design. K Fouché 25953621 Results Factual account of the analysis conducted. Avoid going into an interpretation of your findings. There are APA standards for how statistics must be represented. Discussion 4 key elements: o Start with a summary of the results and consider whether the hypothesis is supported or not. o Relate your findings to previous research. ▪ Consistent → explain how your findings help improve the understanding of the psychological processes being investigated. ▪ Contradict explain how your findings explain the contradiction. o Consider the strengths and limitations of your study. ▪ Pick 2-3 more substantive issues and consider them in detail. ▪ Explain the issue and bring in wider research to support your ideas before suggesting how the design can be improved and how this might change the findings. o Consider future potential research directions. References Include sources that you have both read and cited within your report. and References must be represented in alphabetical order and APA format. appendices Appendices aren’t usually necessary unless you developed your own materials for your study and it isn’t possible to clearly describe these in the methods section (includes informed consent form ant any other materials). K Fouché 25953621 Chapter 2: Questionnaire design Questionnaires and research designs - Provides invaluable data within any research design. - Frequently used in correlational research designs. - Also used in more complex regression research designs → used in a predictive model. o Using a creativity questionnaire to predict a person’s annual income. o Looking at the big five personality traits to predict a person’s creativity. - Qualitative data can also be collected → ask open-ended questions. o Design a questionnaire in such a way that all main questions are open-ended. - Questionaries can include a mix of: o closed questions with predefined answers (yes/no or true/false) to collect quantitative data. o Open-ended questions to collect qualitative data. - Also used in research projects where the collected data from the questionnaire isn’t a core part of the research design. o Collecting demographic information – age, gender, address, education, sex, etc. - Can collect data that can be used as a covariable in your research design. o Covariable – not the main focus of the study but explains some of the variance in the dataset. What do you want to measure, and how many things? - Before designing a questionnaire → operationalize what it is you want to measure. o Include how broadly or narrowly you want to measure it. o Consider whether you want to measure one single variable of something or if there are various different aspects of it you want to measure separately. - 2 ways to design a questionnaire that has multiple scales: o Design every scale in a very deliberate way to measure different aspects of something. o Exploratory approach → design 1 big questionnaire, analyse the data and see if any separate scales emerge from the measure. o The approach you take depends on how much previous research exists on the topic of interest. - In qualitative research designs → more likely to use questions to explore a topic than to measure variables. o Helpful to consider if there are multiple aspects of the topic and to use previous literature to work this out. Do you really need to design a questionnaire from scratch? - Recommended to use an existing measure/questionnaire. o Why? → if there is published research using a well-validated questionnaire, it is preferred to use an existing measure. - Developing and validating a questionnaire takes a long time and is lots of work. - Is it okey to adapt existing measures? o Ideally no → measure is already validated in its current form. ▪ Any changes may risk reducing the measure’s validity. o If you adapt it → make it clear in the Methods section and state exactly how you adapted it. K Fouché 25953621 Designing a questionnaire from scratch Get a clear idea of what it is you want to measure and consider if you want to measure multiple things. Operationalize your variables. Decide what the things are that you are measuring. Review existing research on the topic If there’s an existing measure, it is best you use it. (If and explore existing measures. not, the following steps remain). Gather information to help you in This may come from previous research, existing developing your measure. measures, or from interviewing participants. Preliminary data – initial collection and review of data, either published research or newly collected data, that inform the development of a new questionnaire. Develop the questionnaire, its items and Also ask additional questions to establish its individual questions. participants’ backgrounds and demographic information. Pilot your questionnaire when you have Ask a small number of participants to complete it a full draft. and give feedback. After receiving feedback, consider If you made many amendments to the whether revisions are needed and revise questionnaire, pilot it again. your questionnaire. K Fouché 25953621 Writing the question - Be as consistent as possible throughout. - Pick a certain way of asking something and a certain way participants can respond (especially for quantitative research). o They respond better when there’s consistency in what you ask them to do. - Before any writing → plan out the ways you want to elicit responses. Open vs closed questions - One isn’t better than the other → it depends on the information you want to know. - Combination of both can be required in a mixed methodology approach. o Select the style of question that best addresses your research question. o Don’t swap too much → start with one style and then switch to the other. Open-ended question Participants provide unconstrained written responses. Elicits the data needed in qualitative research designs. Closed question Give participants a predetermined set of responses from which they must select the most appropriate response. Produces the data needed in quantitative research designs. Questions vs items - When using closed responses gotten from closed questions: o Think of whether you want to ask particular questions or ask them to respond to items (statements). - Think of the type of data you want to collect and whether questions or items are more suitable. o It influences the types of responses that participants give. - Be as consistent as possible in your style of data collection. Hints and tips for writing good questions - Be specific with your wording. - Don’t include double negatives in questions as they’re difficult to disentangle = data a participant provides may be meaningless. - Be careful of including two issues in one question – preferably, stick with one issue per question. K Fouché 25953621 - For open questions → ensure that the question’s wording encourage participants to give detailed responses. o Tip → if the question can be answered with yes/no = the question won’t elicit a rich response. Creating the responses - Think about what you want from the participants, choose the most suitable method for responding and stick to the method as consistently as possible. Responses to open-ended questions - No set responses → but think about the questionnaire layout and the space you provide the participants to respond. - The amount of space you leave for a response guides participants as to how long of a response you want. Types of closed responses - There are 3 types of closed responses to closed questions: o Categorical responses o Rank-ordered responses o Likert-scale responses Categorical responses - Questions have predefined categorical responses. Participants tick the right box to indicate which category they belong to. - Generates categorical/nominal data. - Ensure that all participants can tick one of your responses. o Solution → provide an “other” option. - Potential problem when you’re using categorical responses to collect data that could be measured as continuous data. o Example → age (problematic = 0-10, 10-20, 20-30 = overlapping categories). o Categories shouldn’t overlap. o Gaps between categories must be the same (correct = 0-9, 10-19, 20-29). K Fouché 25953621 Rank-ordered responses - Giving participants multiple responses, asking them to rank the responses in a determined order (for example, in order of importance). - Generates ordinal data. - Ensure that you are clear about how participants should complete their rank ordering. - To maintain validity → ensure all participants respond in the same way. Likert-scale responses - Responses are set on a scale and participants must select the response on the scale that represents their answer the most. - Consistency is important → try using only one system of responding and use it throughout the questionnaire. - If you must use different Likert-scale responses → group them together so participants don’t have to switch between different styles of responding. How many points should my Likert scale have? - No strict rule determining the number of responses. - Scales must be ordered symmetrically → equal number of positive and negative responses. - When you have an odd number of Likert options participants must pick from → include a neutral option. o Must be in the centre of the scale with the same number of positive and negative options on both sides. Does my Likert scale need a neutral option? - No but consider whether the neutral response is a legitimate response you’re interested in or whether the research topic requires participants to place their responses clearly within positive and negative responses. - Consider the ethical implications of excluding a neutral option. o Controversial or potentially upsetting questions → unethical to force participants to respond in a non-neutral way. Codes and scores for Likert responses. - After establishing the Likert-scale responses → think about how you’ll transform responses into quantitative data. K Fouché 25953621 - Different coding systems can have a big impact on descriptive statistics (mean, minimum and maximum scores). - When using, or adapting, an established questionnaire → use the same coding → to compare your findings with other published research using the same scale. Are Likert-scales parametric or non-parametric? - Parametric analysis – the preferable way of analysing data. o Certain assumptions of the data should be met to analyse it parametrically. - Consider whether the scores being analysed are taken from a single Likert-scale item or whether the scores are calculated from many Likert-scale items. o Taken from 1 item → collected numbers are limited in range – non-parametric analysis is most suitable. o Collected from many items → parametric analysis is most suitable. Acquiescence bias and negative/reverse scoring - Acquiescence bias – the tendency to agree with things. o May distort the data → participant can give any positive response more often than they should. - Resolve this using negative or reverse-scored items. o Have some items where strongly agreeing is good (example – high levels of creativity) and where strongly agreeing is bad (example – low levels of creativity). - Not all questionnaires use negatively scored items – but it should be considered. - Have a similar number of positively and negatively scored items in a questionnaire → present them in a random and unpredictable order → ensure that participants read every item properly before responding. - Use tick boxes instead of showing participants the numbers you’ll use for scoring. o They won’t see what you consider high and low scores. o They’ll be able to respond in a less bias way. K Fouché 25953621 Practical hints and tips for designing a good questionnaire - Make the questionnaire as simple and easy as possible for participants to complete. - Questionnaires must be clear and professional in style. - Don’t proofread the questionnaire too many times. - No typos or grammatical errors → looks unprofessional and impacts participants’ understanding. o Participants may provide low quality data. - Pilot your questionnaire before using it in your study. o You can check on the wording of questions, responses, layout and how easily participants can complete the questionnaire. Ethical considerations in questionnaire design - All the usual ethical considerations apply. - Consider how you will conduct research ethically if your questionnaire includes topics participants may find upsetting or intrusive. o Ideally → avoid asking such questions. o Sometimes they’re necessary to allow us to explore our research questions. K Fouché 25953621 Validity and reliability in questionnaire design - One type of reliability particularly relevant to questionnaire design: o internal consistency – relates to how consistent scores are in a scale. ▪ Example → Looking at creativity scales, imagine each scale contains 10 items and that every 10 items are telling us something about creativity. For example, one pair of 10 items tells us something about spatial creativity → if 1 or more items don’t actually reflect spatial creativity, the reliability of the scale is reduced. ▪ Can be statistically analysed using Cronbach’s alpha – tells us about the internal consistency of items within scales. Low level of internal consistency → Look at statistics for each individual item within a scale to determine which item/items lack reliability. Consider removing/rewriting any questions that lack internal consistency. Analysing questionnaire data Analysis of qualitative data from a questionnaire - If you used paper questionnaires → type participants’ open answers word for word. - If you used online questionnaires → no transcription is needed. - Techniques used for analysing open questionnaire data: Content analysis Used to convert open data into quantitative data by systematically coding it into categories (converts data into numerical data). Example → ask participants “what helps you be creative?” o Code the content of participants’ answers based on whether it mentioned the following: social support, time, motivation, etc. o Choose categories using previous literature/theory or form preliminary analysis of the data. o For each category, set up a variable in SPSS where you code answers as yes, it did include that category (1) or no, it didn’t (0). ▪ Turns the open data into frequency data → allows you to report the number and percentage of participants for each category. o You can also statistically analyse data using chi-squared analysis. Thematic analysis Look for repeated patterns of meaning within open data and identify qualitative themes. You can identify a central theme or a set of core themes with subthemes within these. Can cover participants’ answers to multiple open questions which were designed to explore different aspects of a overall topic. Creating scores from a questionnaire - There are 3 ways we can extract scores from this specific questionnaire: o Look at the entire questionnaire/ → combining all 30 scores for a single overall measure. o Look at each scale separately/using scale scores → combining the responses to the 10 items within each scale to get 3 measures. o Look at each item separately (in this case 30 items = 30 pieces of data for each participant). - In most cases, researchers use → a single measure (combine all 30 scores into 1 measure) or scale scores (combine each set of 10 items to get 3 measures). K Fouché 25953621 - 2 ways of combining the item scores together to create summary variables: o Sum → add up the item scores. o Mean → calculate the average score across the items. o The choice you make will heavily influence your descriptive analysis: ▪ Changes the numbers you interpret but doesn’t change the findings from your study. ▪ Therefore → any significant differences/correlations in the dataset will be the same regardless of whether you use summed or average scale scores. Determining scales within a questionnaire - Factor analysis – the statistical method often used to analyse questionnaire data. o Identifies groups of items where responses are highly correlated with each other. o Groups highly correlated items together to form a factor. - If participants respond very similar to a set of items → items represent the same underlying thing/variable. - Factor analysis detects patterns of responding, then place items within a single factor that represents something (for example, verbal creativity). K Fouché 25953621 Chapter 14: Correlational design An important distinction between experimental and correlational design: - Experimental → manipulate certain variables (independent) and see if it affects the other variable (dependent). - Correlational → don’t manipulate any variable, we measure existing variables – look for relationships. What does a correlation really show us? - Simplest analysis = look at the linear relationship between 2 continuous variables. - Linear relationship – a relationship between 2 variables where the change in scores are consistent across the full range of scores. o Tells us how scores in 1 variable may systematically change as scores on another changes. o Analyse linear relationships using Pearson’s correlation (parametric analysis). - The 3 different types of relationships: o Positive → scores on 1 variable increases as scores on the other variable increases. o Negative → scores on 1 variable decreases as scores on the other variable increases o No relationship → no correlation, variables doesn’t influence each other. - When a correlation is calculated: o You calculate a r statistic. ▪ Runs from -1 (perfect negative correlation) to +1 (perfect positive correlation). K Fouché 25953621 What variables can be used in a correlational study? - Only continuous variables can be correlated. o Both variables must be measured so that they provide a wide range of values. - Categorical/nominal variables aren’t suitable for correlational analysis. Reasons: o Categories don’t have a certain order → you can place categories in any order and create any correlational finding. o Best to analyse categorical/nominal data using ANOVA. ▪ ANOVA (analysis of variance) = method of analysis looking for differences between groups or conditions. o If your categorical variable only has 2 groups → can’t include it in correlational analysis BUT can analyse it using regression models. Correlation does not imply causation - Causation/causal relationship – the relationship between 2 variables where there is evidence that 1 variable has a direct, causal effect on the 2nd variable. o Even if there’s a significant correlation between 2 continuous variables → doesn’t mean higher/lower scores on 1 variable causes higher/lower scores on the other variable. - Can’t determine the potential casual direction – which variable causes the other variable to change -of this relationship with correlational designs. Confounding and control variables in correlational designs - Even if 2 variables are highly correlated, there might be unmeasured variables that might explain some of the variance in the relationship. o Confounding variables – - If we know of confounding variables before running the study → we can measure and use them as control variables in a certain type of correlational analysis: o Partial correlation – measure of the correlation between 2 variables after considering the variance explained by the 3rd control variable (the confounding variable that now has been noted). ▪ Partial correlation does the following: Removes any variance in the dataset that is explained by the control variable. Looks at the partial correlation between the 2 variables of interest. K Fouché 25953621 Designing correlational studies with more than two variables: Regression analysis - Correlational analysis allows you to look at the relationship between only 2 variables. - Regression analysis allows you to include many variables in your analysis. o Use these variables to create a predictive model where you use different predictor variables to predict a single outcome variable. ▪ Predictor variable – the variable in regression models used to predict the outcome variable. ▪ Outcome variable - the variable in a regression analysis that you want to predict using the predictor variable. How is regression analysis like a cake? - Circle diagram → represents all the variance in the dataset. - Purpose of analysis → determine how much of the variance can be explained by the relationships between variables AND how much is leftover residual/random variance. - Model variance = line of the best fit – straight line fitted to a scatterplot in regression analysis that best describes the relationship between the predictor and outcome variables. o Textbook definition → straight line characterising the strength of a correlation, mostly seen in scatterplots. o The stronger the relationship, the better the variance. - Scatterplots – graphs used to present correlational data. - Residual variance = unexplained variance. o Comes from how far the raw data points sit from the line of the best fit. - The left dataset has a bigger r value (.90) and the right dataset has a smaller r value of.74. - You can convert r values into a number shows the amount if variance that is explained by the model and the amount of leftover, unexplained residual variance. - Squaring the r value( 𝑟 2 ) → determine the variance portion that is explained by the model. - Multiply the 𝑟 2 by 100 → gives the % of the variance that is explained by the model. K Fouché 25953621 What kinds of variables can we use as predictor variables? - Simple correlational analysis → continuous variables. - Regression model → either continuous or binary categorical variables. o Binary categorical variable – defines which of only 2 groups a participants belongs to. ▪ Example → whether a person is innocent or guilty, whether a person is diagnosed with depression or not, whether a person has psychology or sociology. o categorical variable only has 2 groups → include it as a predictor in regression analysis. o Binary predictors must always be coded so that one group is 1 and the other group is 0. o Why can binary variables be used as predictors? ▪ Doesn’t change the analysis if we code the 2 groups the other way around. The change when coding the other way around remains the same in magnitude and only changes direction. o Which group you code as 1 and 0 determines how you interpret findings. - Why can’t you include a binary variable in correlational analysis? o Multiple regression model → use multiple variables to predict an outcome variable, so it’s helpful to include more predictors than just continuous predictors. o Correlational analysis model → only analyse 2 variables. ▪ If 1 variable is continuous and the other is binary, you are seeing whether the 2 groups differ on the continuous variable. If you do this → run an independent t test. Must the outcome variable always be continuous? - Depends on the type of regression analysis. - Multiple regression → analysis based on whether there’s a linear relationship between the predictor and outcome variables. o When you graph the relationship, you’ll be able to explain it with a single straight line. o Can have many continuous or binary predictor variables BUT the outcome variables must always be a continuous variable. K Fouché 25953621 - Logistic regression → form of regression where the outcome variable is a binary, categorical variable. o Trying to predict which of 2 categories participants belong to. o Can have many predictors variables, either continuous or variable. Multiple regression Predictor variables → continuous or binary. Outcome variables → always continuous. Logistic regression Predictor variables → continuous or categorical. Outcome variables → always binary and categorical. How do we deal with confounding variables in regression analysis? - In correlational analysis → run a partial correlation. - In multiple regression → run a hierarchal regression analysis – calculates the total amount of variance in the dataset. o The correlational version of ANCOVA. o Variance then divided into model variance, control variance and unexplained variance. - Regression determines how much of the variability can be explained by the control variable. Effect sizes in correlational and regression analysis - Correlational analysis → the r value is an effect size. - Regression analysis → most often used is the 𝑟 2 statistic. o Tells you about the model variance (the amount of variance that is explained) in the dataset. o Rather describing the effect as small, medium or large effect, you’ll describe it in terms of the amount of explained variance. K Fouché 25953621 How can we use correlational analysis to understand large datasets? - Factor analysis – method of analysis used to reduce a large number of variables down to a smaller number of variables that will summarize the entire dataset. o Groups manifest variables by looking at correlations between individual variables. o Highly correlated manifest variables are then grouped to form the latent variables. - Manifest variables – measured variables that are analysed using factor analysis to identify underlying latent variables. o the original measured variables (the items on the questionnaire). o Then reduced down to latent variables. - Latent variables – an unmeasured variable that is identified through shared variance in measured variables in a factor analysis. o Variable that each provide a single score that represents the underlying latent variable. - Known as “the questionnaire method of analysis” BUT can be used in many research areas. Dealing with assumptions in a correlational design - Assumption 1 → All continuous variables must be (roughly) normally distributed. - Assumption 2 → relationships between variables are linear. o Relationship is best described by a straight line and not a curved line. - Non-linear relationship – scores on 1 variable increases while scores on another variable may increase/decrease in a variable way. o Relationship between the variables differs across a range of scores. K Fouché 25953621 - Most correlational analyses assumes a linear relationship → forces linear description onto dataset. o Solution → create scatterplots and fit the lines to them ▪ Example → if you try to describe the relationship of the example on the right with a straight line, the line will be flat = interpret the findings as showing no linear correlation. - When data isn’t normally distributed, transformations can be used to make them normal. For instance: o Calculate the square root of the variable. o Compute logarithmic (log) transformation. - Simpler solution to transforming data → use a non-parametric method of analysis. For instance: o Spearman’s correlation = non-parametric correlational analysis. Validity and Reliability in correlational designs - The usual rules must be considered to ensure that the study is robust - Correlational studies often rely on data collected from questionnaires → test-retest reliability and internal consistency is very important. Test-retest reliability - Will you get the same or very similar scores if a participant completed a measure twice, at different time points? - Analysed by: o Looking at the correlation between the scores that were measured at different time points in a sample of participants. ▪ More highly correlated = more similar scores = better test-retest reliability of the measure. - Pearson Analysis → r = +1 is a perfect positive correlation and a r = -1 is perfect negative correlation. o Perfect positive correlation (r = +1) → exact same scores were recorded at the different time points. o Many confounding variables may cause scores to be slightly different. o No expectations of a perfect correlation, but expectations of the r value being.7 or higher. Internal consistency - Are all the items within a scale or factor providing similar scores and measuring the same thing? o If so → participants must get very similar scores across all the items in a scale or factor. o The scores within a scale or factor must be highly correlated. Calculating Cronbach’s alpha - Cronbach’s alpha – the statistic that tells you about internal consistency. o Makes split-half comparisons → items within a scale are randomly split into 2 halves and the correlation between the 2 halves is calculated. ▪ Similar scores between the 2 halves = highly correlated. - Calculates all possible split-half comparisons and calculates an overall summary score. - Runs from 0 – 1 → higher values = high levels of internal consistency. o We want an alpha of.7 or higher. K Fouché 25953621 Ethical considerations in correlational design - Must adhere to the APA or BPS guidelines for ethical research. - Sensitive topics → ensure that participants are protected against any potential harm, whether physical or psychological. o Put safeguards in place. o Get informed consent. o Let them know that they’ll partake in a study where the sensitive topic will be mentioned = no forms of deception. o Ensure them in the informed consent that their data is treated with confidentiality and anonymity. o Ensure participants that they can withdraw from the study at any time. o Questionnaires → ensure them that they may skip individual questions so they don’t feel obliged to complete the upsetting questions. o Debriefing is essential. o Provide participants with places where they can get support if needed. K Fouché 25953621 Chapter 5: Experimental design The basics of experimental design - Manipulate something and see whether the manipulation has an effect on another variable. Independent variables and dependent variables - Independent variable (IV) – a manipulated variable within an experimental design. o Must be a categorical variable (nominal). o Example → deciding whether participants receive treatment or no treatment (control group). - Dependent variable (DV) – data collected that are expected to differ according to the independent variable. o Must be a continuous variable (ordinal, interval, ratio). o Example → seeing if the 2 groups differ on some measure of chocolate addiction. Independent and repeated designs Independent measures design Experimental design where independent groups of participants are compared. Different participants are recruited for each of the conditions. Also known as between, unrelated and unpaired design. Repeated measures design Experimental design where the same participants repeat the study under multiple conditions. Only have 1 group of participants repeatedly taking part in the study under different conditions. Longitudinal and cross-sectional design - When you are doing developmental or lifespan research. Longitudinal design An experimental design with repeated measures where the same participants are tested multiple times over a long period of time. Example of a repeated measures design. Phases of testing are very widely spaced over months/years. May be impractical when wanting to explore changes over a very long time. Cross-sectional design An experimental design that collects data from participants at a single point and then compares groups (compares with longitudinal). Example of an independent measures design. Compares separate groups of participants, with each group being a different age. K Fouché 25953621 Pure experimental vs quasi-experimental approach - Pure experimental → randomly allocating participants to conditions/groups. - Quasi-experimental → comparing participants based on whether they’re part of one condition or the other and not randomly allocating them into conditions. o Example → we cannot determine whether people like chocolate or not. Which type of experimental design should we use? - Ideally → pure experimental design and allocate participants randomly into conditions. o If not possible → quasi-experimental design. - Might be obvious whether you should use independent IV or repeated IV measures. o If not → consider the advantages and disadvantages of each design. ▪ If there’s no clear way to divide the conditions into independent variables, run the study as a repeated measures design. - Carryover effects – an effect that influences scores in another condition. o 2 types: Fatigue effect Participants’ performance deteriorates over time or across conditions because of tiredness, boredom, etc. Practice effect Participants’ performance improves over time or across conditions because of repeatedly completing the measures and learning how to improve their performance. The simplest experimental design: comparing 2 groups or conditions Understanding variance in experimental designs - There will ALWAYS be variability in all collected data. o Variability – the spread of raw data points. - Experimental (between-groups) variance: o The variability that we created through our experimental IV manipulation. o The variability between our groups/conditions. - Random (within-groups) variance: o The variability within each of the groups/conditions we cannot explain. K Fouché 25953621 Why variance is like a cake - Statistical analysis quantifies the amount of experimental variance and random variance in the dataset. - The experimental variance is the same in both labs. o Average craving for chocolate is the same in both labs. - The random variance is different between the two labs. o Lab 1 = very little random variance – variance is better explained by experimental manipulation than random variance. o Lab 2 = far more random variance – statistical analysis will show no significant difference between the 2 groups in terms of chocolate craving. - Statistical analysis focuses on how consistent the effect is in each condition/group. Comparing 3 or more conditions Familywise error - Statistically comparing 2 variables → run a t-test. o Can’t use a t-test to measure 3 or more variables. - With each test of statistical significance → 5% chance of making a Type 1 error. o Type 1 error – incorrect rejection of a correct null hypothesis (false positive). o 5% chance of finding a significant difference in our sample that doesn’t really exist in the population. o For each t-test you do, there’s a 5% chance of committing a Type 1 error. ▪ Example → if you run 3 t-tests, there’s a 15% chance of finding a significant difference in the sample that doesn’t exist in the population. - Familywise error – increase in Type 1 error with increasing numbers of analyses conducted on the same dataset. o Solution → run an analysis of variance – ANOVA. o ANOVA – method of analysis that looks for differences between groups/conditions. o Considers the amount of experimental and random variance within a dataset by looking at all conditions at the same time. ▪ Determines whether the variance is best explained by: Experimental variability – differences between the 3 conditions. Random variance – variability in scores within each condition. ▪ Finding is called the main effect = tells you whether there’s a difference across all the conditions within an IV. ▪ When you have a main effect → run further analyses to see where the differences come from. K Fouché 25953621 Contrasts – statistical analysis to compare conditions and break down significant main effects in an ANOVA. Manipulating more than 1 IV: factorial designs - Manipulating more than 1 IV at a time. - Can have many IVs but will likely only see 2, 3, or 4 IVs being manipulated. - Factorial designs are described as two-way (2 IVs), etc. o Two-way factorial experimental design – an experimental design where 2 independent variables are manipulated. - Describing factorial designs: o 2*3 independent measures factorial design ▪ 2 → the 1st IV has two conditions. ▪ 3 → the 2nd IV has 3 conditions. ▪ Only 2 IVs were manipulated because only 2 numbers are shown (2 and 3). o Both IVs are independent measures → independent measures design. o Both IVs are repeated measures → repeated factorial design. o 1 IV is independent and the other is repeated → mixed factorial design. What is a main effect, and what is an interaction? - Use factorial ANOVA when analysing factorial experimental designs. - Two-way ANOVA has 3 separate findings: o Main effect of IV1 → tells you if IV 1’s conditions will differ significantly when ignoring any possible effects of IV2. o Main effect of IV 2 → o Interaction between IV1 and IV2 → tells you if differences across one IV differ depending on the other IV. K Fouché 25953621 Understanding the different ways interactions may look - Statistics are always needed to back the information on graphs up. - Example → When measuring chocolate addiction, you have a two-way factorial design: o 2 (IV1 – control vs mindfulness/experimental) *2 (IV2 – measuring immediately after eating chocolate vs measuring 6 months later). o 2*2 has 3 possible findings: - Left graph → change in scores for IV2 didn’t vary depending on IV1. o No significant interaction o The control group and the mindfulness group = increased from immediately after finishing the treatment to 6 months later. - Middle graph → crossover effect (the typical interaction) o Significant interaction because the change across time differs according to the treatment condition. - Right graph → there is an interaction. o Control group starts with slightly higher levels and this doesn’t change from immediately after treatment to 6 months later. o Mindfulness group had slightly weaker levels immediately after treatment finished, but then the levels further decreased over 6 months. K Fouché 25953621 - Therefore: o If lines are parallel → unlikely to have significant interaction. o If lines aren’t parallel → difference in 1 IV varies according to the other IV. - When adding a 3rd treatment condition, it is a 3*2 factorial design. o 3 (IV1 – control vs mindfulness vs cold turkey) *2 (immediately after vs 6 months later). - Left graph → lines are roughly parallel. o Change in chocolate cravings is similar across all 3 treatment types, but not identical. - Middle and right graph → lines aren’t parallel o Change in chocolate cravings is different across the 3 different groups. What does a factorial ANOVA look like? - Analysis is based on understanding where the variance in the dataset comes from: o The experimental differences between conditions. o The random variance within conditions. - Variance cake with more slices – there are 4 slices of variance: o 1 for each main effect. o 1 for the interaction. o 1 for the residual variance within the dataset we can’t explain. K Fouché 25953621 Developing a hypothesis - Two-tailed – you predict a difference with no directional prediction. - One-tailed – you predict which condition will have significantly higher scores. - In the Introduction, must be based on previous research you have reviewed. o One-tailed → If the research showed that scores in 1 condition are significantly higher. o Open-ended two- tailed → Inconsistent findings or the manipulation in use hasn’t been considered before. - Must frame how you discuss your findings in your Discussion. How many hypotheses do I need? - Depends on the type of experimental design in use. o Manipulating 1 IV → one overarching hypothesis. o Manipulating 2 IVs in a factorial design → at least 3 different hypotheses: ▪ 1 hypothesis for IV1. ▪ 1 hypothesis for IV2. ▪ 1 hypothesis for the interaction between IV1 and IV2. All 3 hypotheses don’t have to be either one-tailed or two-tailed. Dealing with assumptions when analysing data collected in experiments. The 4 assumptions of parametric analysis: - 2 comes from the way the study is designed: o Data must be independent – each participant’s data shouldn’t be influenced by any other participant. o The dependent variable (DV) must be at the interval or ratio level. - 2 comes from after we collected the data: o Data must be roughly normally distributed. o There must be homogeneity of variance across groups. - Aim to always use parametric analysis. Normally distributed data Independent measures design → separately look at the data distribution for each condition. o Non-parametric design if any condition isn’t normally distributed. K Fouché 25953621 Repeated measures design → look at whether the different scores are normally distributed. (Histograms and the Kolmogorov Smirnov test – can use either or both). Homogeneity of variance Definition – when the variability in scores across independent conditions is comparable. Each group must have a similar variability. o Amount of variance must be similar in each condition. Only an issue in independent measures design. - Right graph → variances are different between the groups. o Makes it difficult to draw on your analysis and conclusions. o Control group – cravings are quite consistent. o Mindfulness group – cravings vary widely. - In a repeated measures design, the same participants take part over and over again, so the variability is likely to be more consistent across conditions. o Don’t look at homogeneity across variance, we look at sphericity. Confounding variables in experimental designs - May explain some of the variability in the dataset, but we aren’t necessarily interested in it. Designing an experiment to deal with confounding variables Randomising participants - Independent measures design → decide which participants are allocated to each group. o Allocation should be done randomly so that any confounding variables are randomly spread across the conditions. Selecting participants and setting recruitment/exclusion criteria - Set recruitment criteria if a confound is unlikely to affect a large proportion of your sample. o You select only a certain type of participant (avoid any participant where the confound might be an issue). ▪ Example → you aren’t selecting diabetic participants in the chocolate addiction study because having them in the study will influence the findings. - Exclusion criterion – participant characteristics that mean they can’t be included in the study. o Include it when advertising the study and mention it in Methods. K Fouché 25953621 Matching participants - Alternative approach when wanting to compare groups in independent measures designs, but a confound is likely to affect a larger portion of the sample. - Matching participants across conditions on variables that may be confounds. o Get information from participants at the very beginning of the study to help with allocation. - Balance the number of participants across the condition. o Example → if there’s 6 participants with the confound, place 2 in each of the 3 treatment groups. - Takes away from the aim of random allocation. o Aim to randomly allocate participants after the confound has been considered. ▪ Example → allocate 2 participants to each of the group BUT randomly choose which 2 goes to which group. - After data collection, run statistical analysis. o May run ANOVA to see if the nr of addictions differ significantly across the 3 groups. ▪ Successful matching → nr of addictions doesn’t differ across the 3 groups (ANOVA isn’t significant). - Mention in Methods and include ANOVA to show that it has been effective. Counterbalancing - If the design has a repeated measures component, an aspect of the DV must be repeated for each participant. - The 2nd DV measure might not be as accurate as the 1st measure. - Solution → 2 comparable versions of the same questionnaire (different questions still aiming to measure the same thing). o Creates a 2nd issue – 1 version might be more sensible to detecting the DV. ▪ Solution → counterbalancing and randomisation - 1st time point – 50% of participants completes questionnaire version 1 and 50% completes questionnaire version 2. - 2nd time point – switch. o Randomly allocate participants to each of the version orders. Analysing strategies - Deal with confounds by measuring them and then controlling for them within a particular type of analysis. - Confound explains much of the variance cake → no longer know if our division of the variance into experimental and random variance is correct. - Confound takes away from random variance → a finding can become more significant. K Fouché 25953621 - Run ANCOVA to statistically control for a confound. - ANCOVA (analysis of covariance) – method of analysis that looks for differences between groups/conditions while controlling for the variability explained by a measured control variable. How do I know which confounds to consider in the design, and which way should I deal with them? - Decisions must be theoretically justified by previous research. - Note the confounds that previous researchers controlled for and how they controlled for them. - Consider unmeasured confounds in Discussion. - Include a justification in Introduction when including confounds in the study. o Minimum nr – no more than 1 or 2. - Set exclusion criteria. - Match participants. - Include the nr of addictions each participant has as a covariable in ANCOVA. Validity and reliability in experimental designs - Validity – you’re confident that what you are measuring/manipulating is what you intend to. - Reliability – measuring/manipulating it consistently. - The 2 important elements in experimental design (repeated measures design): o Internal validity o Test-retest reliability Internal validity in experiments - Design and run the experiment in such a way that no other variables explain the findings. Carryover effects and randomisation - Repeated measures design → carryover effects. - Carryover effects - scores increase because of practice or decrease because of fatigue. o Threats of internal validity in repeated measures design – want to be sure that any score changes are because of your manipulation, not because of carryover effects. - Independent measures design → randomly allocate participants to each condition to ensure internal validity. o Any bias = possible that any significant differences between conditions are due to factors other than your manipulation. o If participants withdrew from the study, consider whether it threatens the internal validity. Experimenter and participant biases - Experimenter – treat all participants exactly the same. - Participants may act biased – help the researcher find the “right” answer or act differently if they know they’re in the treatment group rather than the control group. - Solution → run experiment as a double-blind study. o Double-blind study – neither the participant nor the researcher know which condition the participant has been allocated to. Test-retest reliability in repeated measures design K Fouché 25953621 - When collecting data twice using the same measure and nothing major has changed between the 2 times, the scores are roughly similar. - How to test it: o Must have a 2nd set of participants that completes the questionnaire twice (a month between the 2 times). ▪ Nothing major happened between 2 time points = scores will be similar. Ethics in experiments - Must follow the ethical guidelines of the BPS and any relevant institutional guidelines. - 3 aspects of research design that are particularly relevant: o Informed consent. o Avoiding deception. o Protecting participants from harm. Informed consent in experiments - Before participants partake in the study, they must be fully aware of what they are signing up for, what they’ll do, how long they’ll do it for, etc. - Give information in an objective way. - Avoid using suggestions of potential findings. - Let participants generally know of the aims if the study and avoid letting them know of the specific predictions. Deception in experiments - Passive deception – not telling participants which condition they’ve been allocated to. - Active deception – tell something different/lie to participants about what they’ll actually do. - Ideally → avoid using deception. o Passive deception is quite acceptable as long as participants are fully debriefed about the entire design of the study. o When using active deception: ▪ Get ethical approval from an independent ethics committee. ▪ Consider safeguards that might make active deception more acceptable. ▪ More detailed debriefing. ▪ 2nd stage of consent after debriefing (initial consent wasn’t actually informed). Protecting participants from harm - Potential risks for participants in the treatment group (treatment isn’t effective) and in the control group (may suffer from not receiving the new treatment). - Get ethical approval from an appropriate ethics committee. - Be clear to participants about any potential risks. - Carefully debrief participants. - Give advice on where to go to get support if participants experienced any negative effects. Open science and experimental studies - The replication crisis started the open science movement. o Open science – movement in scientific research that opposes questionable research practices and promotes more rigorous and open practices. K Fouché 25953621 - File drawer problem – Not significant findings are rarely published and therefore failures to replicate tend to end up in the file drawer rather than the journals. - Issues arose because researchers adopted questionable research practices which led to the adoption of open science practices intended to improve the quality and rigour of scientific research. o Questionable research practices – intentional or unintentional research practices that bias research towards finding significant results. - Biggest change → we share research plans and data rather than just the final paper. o Other researchers can explore the data, consider alternative analyses, and draw on alternative conclusions. - Key practices of the open science framework: o At the very beginning of the research process, have a clear experimental plan that includes your analysis strategy. o Base your predictions on previous research, not on your own findings (HARKing). o Plan your analysis strategy clearly before collecting data. o If findings aren’t significant, be confident in considering the reasons why this occurred in your Discussion. K Fouché 25953621 Chapter 22: Designing interviews and focus groups When might interviews and focus groups be used? - Mostly used in qualitative or mixed methodologies research designs. - Used to get quantitative data or to lay the ground for quantitative studies. Primary data collection - When aiming to explore a topic. o 1:1 interviews for example. - Collect open data – participants giving free responses o Example → participants describing their feelings in-depth. - Closed open data – participants choose from a set of possible answers. o Example → participants choose the answer on the questionnaire that best describes their feelings. - Can be collected for only 1 part of the study. o Pluralism – the use of more than 1 qualitative approach in the same study. - Used to collect data for quantitative analysis o Example → content analysis. Open data Qualitative data. Not limited by measurement scales. Not driven by experimental manipulation. Possibilities are left open. Closed data Quantitative data. Limited by measurement scales. Driven by experimental manipulation. Type and format of the data is pre-determined. Exploring talk in action - Interviews and focus groups are used in studies that investigate talk and language in action, for example discourse analysis. Examples: o Individual interviews → explore how patients frame their experiences of being diagnosed with depression and the language they use to describe their symptoms. o Focus groups → explore societal discourses around mental illness by having the group talk about different mental health conditions. - Used for the exploration of meanings. Exploring experiences and processes - Interviews and focus groups are useful because of the achievable level of depth. Hypothesis and research question generation - Useful for creating hypotheses and research questions to be examined in a further study. K Fouché 25953621 Measure development - Used to assist the development or adaption of a quantitative measure by generating, refining or validating items. Understanding findings - Used to further understand some initial research findings, especially when the findings were unexpected or contradictory. Choosing between interviews and focus groups Level of structure - Interviews can be: Structured interviews Set questions. Collects closed, quantitative data. Semi-structured Flexible questions. interviews Collects open data that can be analysed quantitatively (content analysis) or qualitatively. Feels like a natural conversation. Interviewers have a set of questions. o How questions are phrased and when they are asked is flexible. ▪ Example → if question 1 links with question 5, interviewers skip questions 2,3 and 4 and return to them later. Most commonly used. Unstructured interviews No predetermined questions. Collects open data that can be analysed quantitatively (content analysis) or qualitatively. Requires the highest skill level for interviewers. No set questions or set order to ask them. Guided by participants’ words and by the themes of the moment. Create questions as you go and phrase them appropriately and sensitively. - Focus groups are most similar to semi-structured interviews. K Fouché 25953621 Depth vs breath, and other pros and cons - Interviews are great for achieving depth. - Focus groups are great for capturing breath. - Interviews: o Depth can be achieved because only 1 person is questioned and the interviewer has much control over the direction of the conversation. o Participants feel comfortable enough to disclose more than they would in a focus group. o Participants can see how interviewees use talk in interaction. o More time-consuming and costly to conduct. o Participants can describe group dynamics but there’s no opportunity to see how these play out. - Focus groups: o Better breath is achieved because more participants are included at once. o Quicker and cheaper than interviews → cover many participants in the same amount of time it takes to do 2 interviews. o Absolute limit in 1 focus group → 10 participants. o The researcher is the moderator or facilitator → steers the conversation rather than directing every aspect. ▪ Moderator/facilitator – the researcher running the group discussion. o More like a genuine social interaction → opportunity to observe talk in interaction in more realistic conversations. ▪ Can observe interpersonal processes and group dynamics. ▪ Group dynamics can distract from the discussion of the topic. o More difficult to transcribe focus group data. Participant behaviour as an additional source of the data - May convey how they feel about a topic by their tone of voice or body language. - Keep record of focus group’s emotional tone → may not be in the written transcript. - Focus groups reveal much about social interaction. o Issues may emerge and researchers see how participants handle it in the language they use and how they speak to each other. K Fouché 25953621 Sampling Considering your participant group - Semi-structured and unstructured interviews and focus groups → people willing to talk about their feelings. - May be difficult for people to talk about a sensitive topic or a topic that’s important to them. - Language and communication skills → barrier to opening up in the required way. o May be too challenging for people with difficulties in communication abilities to express themselves verbally. - Solutions: o Give participants choices. It may prevent particular groups from being excluded. ▪ Choice of how they take part (either a focus group or interview / interview or questionnaire). ▪ Choice to take part remotely (through a phone or email). Choosing your participants - Depends on the research questions. - Set inclusion and exclusion criteria. - The identity of each participant matters and is important. - You may only be able to include a certain number of people because of practical constraints. Sampling for focus groups - Homogenous group – A focus group in which participants share key features (shared characteristics or experiences connected to the subject). o Participants share the same background before the study. o Get more detail about people’s views with the shared characteristics. o Example → participants working for the same company. - Heterogenous group – a focus group in which participants are different (no shared characteristics or experiences connected to the subject). o Participants come from different backgrounds. o Example → see if there was a consensus between people’s views participating. - Pre-existing group – a focus group in which participants know each other. o More comfortable to open up and disclose information. o Example → students on the same course at the same university, friends or colleagues. - New group – a focus group where participants have never met before. o Harder for them to open up BUT you gain a broader range of perspectives. o Students who are on courses at different universities and have never met before. - Concerned group – a focus group in which the subject matter of the study is important to participants. o May be keener to participate and share views. - Naïve group – a focus group in which participants have no particular connection to the subject matter. K Fouché 25953621 Designing interview/focus group schedules - Schedule – list of questions and other texts used in interviews or focus groups. o Can also be called an agenda or guide. - Designing a structured interview → similar to designing a questionnaire. Planning and reviewing of semi-structured interviews - Open-ended questions are written in advance to encourage participants to talk about relevant concerns. - Questions put in a logical order but it doesn’t have to be asked in this order. - Non-prescriptive schedule → may skip questions if covered elsewhere and may add questions spontaneously to explore a topic. - may need more than 1 set of questions depending on the research questions. o Might have different questions for different participant groups. - Define the aims of each course of interviews/focus groups to determine which questions need to be covered. Number and order of questions - Number of questions decided by weighing up coverage vs time available. o You’ll not want to rush participants and the process. ▪ Consider if any demographic items can be asked in a separate questionnaire. - Must have a natural flow to the question topics: o Start with introductory questions (easy and helps to build a rapport of the participant). ▪ Focus groups → questions that everyone answers in turn. o In-depth and sensitive questions in the middle (participants are more comfortable and open to answer). o Don’t put important questions last (main topics must be covered before time runs out). o Good to end with less sensitive questions at the end – ends on a neutral tone. - Allow time at the end for the participants to say more or ask questions. K Fouché 25953621 Types of questions - Semi-structured schedules have main questions (each with follow-up prompts and probes). Main questions - Open-ended questions. - Must encourage participants to open up about an aspect of a topic. - Reflects the research aims and the qualitative approach. - Descriptive questions – ask participants to give a general account of something. o More appropriate in interviews/focus groups where the aim is gathering data to use with content analysis to be analysed quantitatively later. - Evaluative questions – ask participants how they feel about something. o More appropriate in interviews/focus groups where the aim is gaining in-depth insight into people’s experiences (grounded theo