PSY3007S Statistics Notes PDF
Document Details
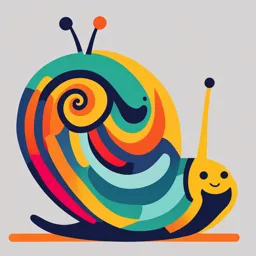
Uploaded by UnbeatableAlexandrite
UCT
Tags
Summary
These are notes on statistics, covering fundamental concepts and statistical tests. Topics include basic principles of statistics, probability, sampling distributions, and different tests. The document is likely for an undergraduate course focused on statistical analysis
Full Transcript
**Disclaimer**: Attention, dear reader. These notes have been lovingly crafted for students in dire need, and they demand the utmost respect. By unlocking the mystical wisdom held within these pages, you are granted exclusive access to an academic wonderland. But beware! By purchasing and utilizing...
**Disclaimer**: Attention, dear reader. These notes have been lovingly crafted for students in dire need, and they demand the utmost respect. By unlocking the mystical wisdom held within these pages, you are granted exclusive access to an academic wonderland. But beware! By purchasing and utilizing these notes, you solemnly swear to not share or resell them. Failure to uphold this sacred trust will unleash a storm of catastrophic consequences upon you. Expect pigeons to target you as their favourite perch, raining blessing upon your shoulder each time you pass Sarah Baartman Hall. Your eduroam connection will become elusive, and the hunt for a library plug point will be a never-ending quest. Your student card will mysteriously vanish, only to reappear the day after you buy a replacement. Legal repercussions, as well as eternal group projects with procrastinators will haunt your academic journey. No one will talk to you at parties, and your UCT shuttles will always run late. So, venture forth, intrepid seekers of knowledge, and let these notes illuminate your path. Just remember, they are strictly for your eyes only. Let the pursuit of academic greatness begin! PSY3007S Statistics =================== - Stats \> not intuitive, based on principles - Must understand fundamental rules \> normal distribution, standard normal dist, sampling dist of mean, central limit theorem, standard error Fundamental concepts in Statistics - Aim of statistics \> estimate population characteristic - work out probabilities so that you can make inferences about characteristics in the population - making inferences about population characteristics - estimates of what you think might exist in popn at random - mean = estimate - frequency count = estimate - thus make meaning of data - don't always have access to whole population \> make use of samples - samples give us means/measure - samples create degree of error in estimate - Acceptable amount of error defined by standard error - To find standard error \> must find a distribution of mean - [standard error] how much we expect a mean will vary across samples of a population - if estimate falls within standard amount of variation \> more or less similar to popn - if estimate far outside of margin of standard error \> then significant - standard error = standard deviation of the sample means - smaller sample = more error - residual / standard error = (sample mean -- popn mean)/error = test statistic (Chi, T, F) - is what you see different for what you expect to see when accounting for error? - Test statistic = what you expect to see under condition of randomnessText, letter Description automatically generated - How we calculate the residual and error will depend, in part on the type of data we have - acceptable amount of error defined by standard error - to find standard error we must go back to sampling distribution of mean - rejection region \> very small possibility that what you see is actually part of your null distribution - small probability \> reject null distribution, more than likely part of another (alternative) distribution - significance testing \> what is the probability that the null is correct? - Null hypothesis \> sample is same as population at large - Alternative hypothesis \> whatever change/difference in data is a function of the independent variable Chi-squared ----------- **Classification** - Statistical analyses requires that data be organized into four scales of measurement - Discrete - Nominal/categorical \> labels; no rank (religion) - Ordinal \> categories are ranked or ordered (likert scale) - Continuous - Interval \> true quantitative measure, no true zero (temperature) - Ratio \> physical properties which have a true zero value (age, length) - Types of variable \> determines the kind of statistical test to use  - Inference test tree - Chi-squared classification - Chi-squared is primarily used for nominal/categorical data (although also ordinal -- see insomnia study in textbook) - Classification is useful when it allows researchers to group data into *exhaustive* and *mutually exclusive* categories - [Exhaustive] encompasses all the members of a population - [Mutually exclusive] no member of the population can belong to more than one category Contingency tables - When data are classified with respect to two or more categorical variables, the data form a contingency table - AKA **r** x **c** table (row x column) - Contingency tables also help us understand the two types of Chi-square test - Goodness of fit -- unidimensional - Test of contingency - multidimensional - Examples - Multidimensional table - Table consists of 6 cells, number in each cell is a frequency/count - Unidimensional table - Suitable for 'goodness of fit' Chi-square test Chi-squared: x^2^ - The chi-squared test analyzes frequency data - Thus, data must be categorical (note: ordinal is also suitable) - Assumptions that underlie the test: - **M**utually exclusive classification - **E**xhaustive categories - **I**ndependence of observations (i.e. each count should be independent of another) - Two kinds of chi-square tests: - Goodness of fit -- useful to test a single dimension of data - Contingency -- useful to test whether two variables are associated (i.e. are they contingent on each other?) ### L1 Goodness of fit - Working out goodness of fit - Depends on what pattern you *expect* (i.e. *E*) - Should each category have about the same number of observations? - Do you expect an uneven distribution due to prior research? - eg. Handedness -- know what proportion of Right-handed vs Left-handed - Decision making steps in inferential statistics - Set up research hypothesis (H~1~) - Set up null hypothesis (H~0~) - Choose a significance level (a) - Calculate the sample statistic (e.g. *t*-value) - Calculate probability from hypothetical sampling distribution (*p-*value) - Decide if result is representative of hypothetical distribution: - If unlikely (*p \ dependent or independent? - Chi squared test of contingency aka test of independence aka two way chi-square - Changes \> how you calculate expected values and how you calculate degrees of freedom - Two-Way Chi-Square - Asks whether observed frequencies reflect the independence of two qualitative variables. - Compares the actual observed frequencies of some phenomenon (in our sample) with the frequencies we would expect if there were no relationship at all between the two variables in the larger (sampled) popn - Two variables are independent if knowledge of the value of one variable provides no information about the value of another variable. Steps of Test of Contingency 1. Construct table with row and column totals (observed frequencies) 2. Calculate expected frequencies Multiply the associated row total by the associated column total and divide by the grand total = [(row total)\*(column total)]\ grand total 3. Set Significance level (a = 0.5)\ Find Degrees of freedom = (number of columns -1) (number of rows-1) = (c-1)(r-1) 4. Calculate test statistic by adding all into formula 5. Calculate critical x^2^ using table (or in excel =CHIDIST(x, deg freedom) 6. Interpretation Decide 6\. **Interpretation** Table for critical values of x^2^ ###  ###  ### L3 Analysis of residuals Isolating the source of association - A significant χ² tells us that there *is* *an association between* the variables, however it does not tell us *where* the significance comes from - We need to determine in which cell(s) the significance is located - To locate the source of the significance we compute the *residual.* Analysis of residuals - A residual in a contingency table is the deviation of the observed from the expected frequency: - Residual = O - E - However, the size of the deviation is related to the size of the sample (i.e., cells with the largest expected values have the largest residuals) - Sample size can make residual seem quite big - The residual therefore needs to be *standardized* in order to control for sample/cell size Standardized residual - [Standardized residual] is a residual that controls for the size of the expected cell by dividing by the root of the expected frequency - Thus: - e = standardized residual - The standardized residual indicates each cell's relative contribution to the significance of the χ² value - Standardized residuals vs z-scores - Standard normal distribution: mean = 0, stdev = 1 - standardized residuals: stdev less than 1, so it underestimates the size of the variance. - Therefore, we calculate *adjusted residual* Adjusted Residual \ [\$\$d = \\frac{\\mathbb{e}}{\\sqrt{\\left( 1 - \\frac{n\_{\\text{row}}}{n\_{\\text{total}}} \\right)\\left( 1 - \\frac{n\_{\\text{column}}}{n\_{\\text{total}}} \\right)}}\$\$]{.math.display}\ - *e* = standard residual - n*~row~* = row total - n~*total*~ = grand total - n*~col~* = column total - The adjusted residuals are interpreted in the same way as z-scores. - The critical value for *z (z~crit~)* when *α =* 0.05 is 1.96 (or -1.96). - Thus, any adjusted residual larger than 1.96 (or smaller than -1.96) is statistically significant. Notes on interpretation - Significance should be interpreted in terms of what was *expected*. - A positive (standard/adjusted) residual means the observed value is (significantly) more than expected. - A negative (standard/adjusted) residual means the observed value is (significantly) less than expected #### (Tredoux & Durrheim, 2018) Ch19 Distribution-free tests - [parametric tests] statistical tests that require assumptions about parameters or their estimation are know L4 Hypothesis testing and T-tests --------------------------------- Definition of t-tests - Samples are only *estimates* of populations. - Sample means are subject to some degree of *error*. - The Central Limit Theorem is a theory about the properties of samples... (revise!) - [Central limit theorem] Each sample mean is an estimate of its relative population mean. But differs from the population mean by an amount indicated by the *standard error* - [t-test] allows us to compare the means for two distributions by taking into account the standard error. Hypothesis testing procedure - Set up research hypothesis (H~1~: decide if directional or not) - Set up null hypothesis (H~0~) - Choose a significance level (α) - Measure particular outcome (t - sample statistic) - Calculate probability from hypothetical sampling distribution (p) - Decide if outcome is representative of hypothetical distribution: - If unlikely (*p \* α), the result is inconclusive Decision making confidence - Decision-making conventions in statistics help us determine where **randomness** ends and an **effect** begins - conventions help us determine whether our observations are random (i.e., part of the hypothetical / null distribution) or the function of our independent variable (and therefore part of an alternative distribution) - How do we decide what is random and what is an effect? - We use alpha - [Alpha (α)] is a confidence level - It tells you the level of confidence you can have in your decision. - At a 95% confidence level, you accept a 5% chance of being wrong - At a 99% confidence level, you accept a 1% chance of being wrong - Actual percentage is somewhat arbitrary, consensus that these are acceptable levels - Which percentage we choose is dependent on whether we are more comfortable making type one or type two errors Type I & II errors - Alpha (α) and Beta (β) - **α** = **Type I Error**: Probability of rejecting H~0~ when it is true - **β = Type II Error**: Probability of accepting H~0~ when it is false Diagram Description automatically generated - red is hypothetical null - blue is hypothetical alternative distribution Decision making errors - decision making errors - [**https://www.youtube.com/watch?v=BJZpx7Mdde4**](https://www.youtube.com/watch?v=eoYYFVVcMlM) - [**https://www.youtube.com/watch?v=eoYYFVVcMlM**](https://www.youtube.com/watch?v=eoYYFVVcMlM) - Note that *α* is the significance level, so you can control the risk of a Type I error by choosing a more stringent level of *α.* - [Significance level] the probability with which we are willing to reject H~0~ when it is, in fact, correct. - So, if the significance level is.05 (*α =.05*) there is a.05 chance of a Type I error - A more stringent level of *α (e.g., 0.01*) reduces the chance of a Type I error. - However, that will increase the risk of a Type II error, i.e., *β* increases as *α* decreases. - This is the most important aspect of the significance level: - *α* determines the likelihood of making a wrong decision to reject the null hypothesis - You should choose *α* before starting the research ### One sample tests - A one-sample t-test is used to compare a sample to a known population. - However, unlike z-tests, the population variance is unknown (use sample variance) ### Two sample tests - Two related samples - Observations are not independent - Paired or repeated observations of the same sampling units - Two independent samples - Compare two distributions that are independent of one another **Assumptions** - The assumption of **normality** - It is assumed that the samples we are comparing come from populations which are normally distributed - Assumption of **independence** - We assume that the samples we are comparing do not influence each other's scores in any way (except for repeated measures t-tests - Assumption of **homogeneity of variance** - We assume that our samples have variances which are not very different (by a factor less than 4). - To calculate this, use the formula: - k = s~1~^2^ / s~2~^2^ - k=the ratio of the largest to smallest variances - s~1~^2^ = the larger variance - s~2~^2^ = the smaller variance **Independent sample t-tests** - Independent t-tests are used when two samples are compared. - The two samples are separate from one another and are independent. - That is, the two samples are comprised of different subjects or participants. - Sample sizes can be unequal. - Degrees of freedom when using pooled variance = n1 + n2 -- 2 - Degrees of freedom when using unequal variance = calculate n1-1 and n2-2  **Repeated Measure t-tests (dependent samples t-test)** - The repeated measures t-test is used to compare two samples which are not independent from one another. - The samples that are compared are comprised of the same subjects or participants (e.g., pre- and post- scores). - If your assumption of independence is violated, you can use this t-test. #### (Tredoux & Durrheim, 2018) Ch9 Analysis of variance -------------------- ### L6 One-way ANOVA (Ch 14, 15) **Logic of ANOVA** - T-tests vs ANOVA  - Example - 1.)Providing Cognitive Behavioural therapy; 2.) Providing Psychodynamic therapy; 3.) Lindt chocolate therapy; 4.) Exercise regime - T test cannot simultaneously test for differences between groups - Could do series of t-test but \> multiple comparisons problem - Must do 6 t-tests \> familywise error rate problem Familywise error - There is always uncertainty regarding statistical decisions - If the significance level (α) is 0.05, we have a 5% chance of making the wrong decision in rejecting the null hypothesis (type 1 error) - [Familywise error rate] the probability of rejecting at least one null hypothesis when it is true - in a set/family of comparisons - The familywise error rate allows us to estimate the probability of making at least 1 type 1 error in a family of comparisons. - FWER formula - We can demonstrate the FWER formula in the following steps: - Probability of making a type I error = α - P (type 1 error) = α - P (***Not*** making a type 1 error) = 1 -- α - P (***Not*** making a type 1 error in ***k*** tests) = (1 - α)^k^ - P (Making ***at least 1*** type 1 error in *k* tests) = 1 - (1 - α)^k^ - Familywise error rate formula = 1 -- (1 -- α)^k^ - - Helps us explain how much error we expect with many comparisons - FWER and multiple comparisons - where α is your significance level - and k is the number of significance tests you do - in previous example, 6 t tests so FWER = 1 -- (1 -- 0.05)^6^ = 1 - 0.735 = 0.265 - So here, we have a 27% chance of making a mistake -- finding at least 1 comparison significant when it really isn't - **↑Comparisons/tests = ↑ Type 1 Error rate** - Anova provides different approach - In 10 000 comparisons, up to 500 type one errors **ANOVA concepts and terminology** - ANOVA \> analysis of variance - Omnibus test -- does all the comparisons in one go - ANOVA can tell you whether there is a significant effect - Doesn't tell you precisely where the significant difference lies - Therefore, it is called an effect, rather than a difference (t-tests) - **Assumptions of ANOVA** - Certain requirements are made of the data, otherwise ANOVA may be inappropriate - [For the simple 1-way ANOVA (i.e., single Independent Variable):] - Normality - Homogeneity of variance - Difference between data set with smallest and largest variance must not be larger than factor of four - Independence of observations - ANOVA is relatively robust to minor violations of these assumptions but never violate assumption no. 3 -  - Sample variance becomes mean squares - Thus Text, letter Description automatically generated Error (random) variance VS systematic variance - [Error variance] the random variance in sample means (see, sampling distribution of the mean, CLT, standard error) - [sta] the variance in the sample that is *due to the action of the independent variable* (IV) - ANOVA is used to isolate the systematic variance Systematic variance - All the scores in each sample were identical - à Thus, no within-group variance - à Thus, no error variance in this example. - à Any difference between the groups is due to *systematic variance*. - à Due to variance between the groups. - Therefore, all the variance can be explained by the effect of the **independent variable** -- i.e. different types of treatment give different result - NOTE, in the example: - Error variance = found *within* the sample groups - Systematic variance = found *between* the sample groups Separating systematic variance - Unfortunately, real life is not this clear-cut -- there will always be some variance within the groups due to natural random fluctuation/differences. - We cannot separate systematic variance out and study it alone. - It is not possible to measure *pure* systematic variance. - There is always error mixed up with our estimate of systematic variance. - WITHIN GROUPS variance à error variance - BETWEEN GROUPS variance à error & systematic variance - ANOVA compares the two types of variance (i.e., within-group and between-group variance) to establish whether systematic variance is present. - If the variance BETWEEN groups (error + systematic) is much larger than WITHIN groups (error), clearly something other than error variance is accounting for the difference between groups ANOVA terminology - Variation -- spread / dispersion of scores around the mean - Variance -- average spread / dispersion - Variation in ANOVA measured with: - SSgroup - SSerror - Variance in ANOVA measures with: - MSgroup - MSerror -  - Note: SS = sum of squared differences from the mean. - SS is a measure of variation, not variance. Sum of squares -- formulas - Text, letter Description automatically generated - If you have two, you can work out the last one by subtraction - x = an individual score/observation - x̅ = group mean - X̅gm = grand mean - n = number of data points per group - df = n-1 (i.e., number of data points per group -- 1) - s^2^ = group variance Variance in ANOVA -  - MSgroup aka MSeffect - MS~error~ = average within-group variation - MS~group~ = average between-group variation - Thus, - MS~error~ = SS~error~ / *df*~error~ - Ms~group~ = SS~group~ / *df*~group~ Degrees of freedom - df~TOTAL~ = N -- 1 - where N is the total number of participants - df~error~ = k(n-1) - where k is the number of groups - n is the number of observations per group - df~group~ = k -- 1 the F ratio - The tests statistic in ANOVA is the F-statistic - F= MS~group~/Ms~error~ - Also called the F ratio - You can see that the bigger MS~group~ is compared to Ms~error~ the bigger F will be. - A significant F means we have a significant amount of systematic variance present -- hence we have a significant [effect.] - Compare to 0.05 ANOVA table ### L6 One-way ANOVA examples **Example** **1** - We compare three groups to see if they are significantly different from one another - We gather 5 scores from each group - Totalling 15 scores - Calculate grand mean and means for each individual group - Grand mean = 4.73 - Mean of group one = 4.2 - Mean of group two = 7.4 - Mean of group three = 2.6 - Calculating degrees of freedom - *df*~total~ = N -- 1 - dftotal = 15-1 = 14 - *df*~group~ = k - 1 (k = number of groups) - dfgroup = 3-1 = 2 - df~error~ = df~total~ - df~group~ - dferror = 14 -- 2 = 12 - *df~total~ = df*~error~ + *df*~group~ - Calculate sum of squares (SS) - SS Total =  - Take every score (*x*) and subtract it from the grand mean (GM). Then square the deviations. Summate the deviations to calculate SS total. - =(5-4.73)²+(4-4.73)²+(3-4.73)²+(6-4.73)²+(3-4.73)²+(6-4.73)²+(8-4.73)²+(9-4.73)²+(6-4.73)²+(8-4.73)²+(4-4.73)²+(3-4.73)²+(1-4.73)²+(2-4.73)²+(3-4.73)² - SS Total = 78.9 - SS Group = - To calculate SS group, we need the sample size (n), the individual means of each group ([\$\\overline{x}\$]{.math.inline}) and the grand mean ([\$\\overline{X}\$]{.math.inline}) - =5\*(4.2-4.73)²+5\*(7.4-4.73)²+5\*(2.6-4.73)² - SS group = 59.73 - SS Error =  - To calculate SS error, we need degrees of freedom (df, i.e. n-1) and variance ([*s*^2^)]{.math.inline} for each individual group. - Thus: 4\*1.7+4\*1.8+4\*1.3 = **19.2** - **OR** - SS error = 78.93- 59.73 = **19.2** - Calculating mean of squares -  - Insert scores into table Table, calendar Description automatically generated - Now calculate F ratio - F = MS group / MS error = 29.87/1.6 =18.67 - This is our F statistic - We now need to decide whether it is significant or not - Consult F distribution tables  - Critical F - Critical F is 3.89 according to F distribution table - If our calculated F (18,67) is larger than our critical F (3.89), we reject the null hypothesis - Therefore, there is a significant effect (i.e. one or more means are significantly different from another) **Example 2** - Table, calendar Description automatically generated - calculate the grand mean, and means for each individual group - Grand mean = 4.2 - Mean of placebo = 3 - Mean of low dose = 6.2 - Mean of high dose = 3.4 - Calculate the degrees of freedom - *df*~total~ = N - 1 - *df*~group~ = k - 1 (k = number of groups) - df~error~ = df~total~ - df~group~ - *df~total~ = df*~error~ + *df*~group~ - dftotal = 15-1 = 14 - dfgroup = 3-1 = 2 - dferror = 14 -- 2 = 12 - Calculate sum of squares (SS) -  - Take every score and subtract it from the grand mean. Then square the deviations. Summate the deviations to calculate SS total. - =(3-4.2)²+(2-4.2)²+(3-4.2)²+(5-4.2)²+(3-4.2)²+(7-4.2)²+(9-4.2)²+(6-4.2)²+(4-4.2)²+(5-4.2)²+(4-4.2)²+(3-4.2)²+(2-4.2)²+(4-4.2)²+(4-4.2)² - SS total = 54.4 - - To calculate SS group, we need the sample size, the individual means of each group and the grand mean - =5\*(3-4.2)²+5\*(6.2-4.2)²+5\*(3.4-4.2)² - SS group = 30.4 -  **General Steps** 1. Calculate the grand mean, and the means for each individual group 2. Calculate the degrees of freedom - *df*~total~ = N -- 1 - *df*~group~ = k -- 1 - (k = number of groups) - df~error~ = df~total~ - df~group~ - *df~total~ = df*~error~ + *df*~group~ 3. Calculate sum of squares (SS) - SStotal - Diagram Description automatically generated - To calculate SStotal, take every score and subtract it from the grand mean. Then square the deviations. Summate the deviations to calculate SS total - Do for each cell \> (x-x-)\^2 \> SUM(all range) - OR =VAR(entire sample)\*(N-1) - SSgroup -  - To calculate SSgroup, we need the sample size, indiv means fo each group, and grand mean - SSerror - -  4. Calculating the mean of squares (MS) - -  5. Insert scores into table - - P = FDIST(f, dfgroup, dferror) 6. Calculate F ratio - F = MSgroup/MSerror - This is our F statistic \> must decide whether it is significant or not - Consult F distribution table or given critical value #### (Tredoux & Durrheim, 2018) Ch14 #### (Tredoux & Durrheim, 2018) Ch15 Factorial ANOVA --------------- ### 27SEP L8 Factorial ANOVA introduction - Exam \> wont have to do calculations, only interpretations - ANOVA vs Factorial ANOVA - Simple one-way ANOVA recap - i.e. We have a single independent variable with multiple levels - E.g. We're interested in the effect of different forms of therapy on depression -- therapy is the independent variable but has multiple levels (e.g. CBT, psychodynamic, person-centred therapy) - Factorial ANOVA - Has more than 1 independent variable - For example, we could look at the impact that **type of therapy** AND **anti-depressant medication** has on treating depression - We could have 2 independent variables, e.g.: - **Type of therapy** (CBT, psychodynamic therapy and chocolate therapy), and - Anti-depressant **medication** (present or absent) - This would form a 3 x 2 design - One-way ANOVA could only answer if different therapies impact differently on depression -- 1 independent variable with 3 levels (CBT, psychodynamic therapy and chocolate therapy) Recap - Variables \> factorial ANOVA has more than one independent variable - Designs \> data presented in a factorial table - Benefits \> these designs are more complex (realistic), more economical, and allow us to capture interatctions - Effects \> main effects (differences betw marginal means) and interaction effects (differences between cell means) - Assumptions \> normality, homogeneity of variance Factors - Independent variables aka factors -  - Factor A has two levels - Factor B has 3 levels - 2x3 ANOVA design - Therefore 6 cells/groups of data to compare - Can also compare marginal means Factorial Designs - Factorial designs can have more than 2 independent variables - For example, we could introduce the variable, 'gender', to the previous example -- look at the effect of gender, therapy and anti-depressant on depression - If we had 3 IVs, we would have a 2 x 2 x 3 Factorial ANOVA design - Designs can become very complex and require large sample sizes - This can become hard to conceptualise and interpret - We will only cover designs that have 2 independent variables Benefits of factorial design - It allows us to capture more complexity - It's particularly helpful in studying social and psychological phenomena - Psychological phenomena are very rarely only affected by one thing or one independent variable - Factorial ANOVA allows us to examine the interaction of variables - It is economical -- we can test multiple hypotheses simultaneously Interaction and main effects - [Main effect] Compares marginal means for single IV/ Factor - Is there a difference across the levels of 1 factor? - Ignores the other IV; Looks at differences across marginal means - - [Interaction] Compares the cell means with each other -- looks at interaction of all IV's/ Factors - Interaction is present if the effects of one IV are dependent on the level of the other IV - This would occur if the (pattern of) therapy results differed for those on medication versus those not on medication - [It is necessary to look at 3 effects:] - Is there an effect for therapy? - Is there an effect for medication? - Is there an interaction between therapy and medication? Statistical hypotheses - **Factorial ANOVA tests 3 sets of hypotheses:** - 1\) Null Hypothesis: All Factor A (main) means are equal - 2\) Null Hypothesis: All Factor B (main) means are equal - 3\) Null Hypothesis: There is no interaction between Factor A and Factor B Logic of factorial ANOVA - **Analysis of variance** - In the simple 1-way design, the variance was split into between groups variance (systematic variance + error) and within groups variance (error) - Factorial ANOVA begins the same - SS~TOTAL~ split into SS~GROUPS~ and SS~ERROR~ - SS~GROUPS~ then further split into SS~A~, SS~B~ & SS~AB~ (Variation due to factor A, variation due to factor B, and variation due to the interaction of A and B) - *Note: the interaction is usually expressed as A\*B or AB* Partitioning variance in factorial ANOVA - Each main effect has the same variation (SS) and variance (MS) as if you would be running a one-way ANOVA on each, but the F for each effect is larger in Factorial ANOVA because... - factorial analysis reduces Mserror in accounting for the variance due to more than one effect and the interaction - error variance \> unexplained variance ### 30SEP L9 Factorial ANOVA Assumptions of factorial ANOVA 1. Normality - When the distribution is normal, the mean is the appropriate measure of central tendency -- ANOVA looks at means; hence normality is important - Relatively robust to violations of assumption - **BUT** not appropriate if you have both unequal cell sizes (i.e. number of subjects per cell) and distributions skewed in different directions 2. Homogeneity of variance - Again relatively robust to violations of assumption - **UNLESS** you have unequal cell sizes - Inspect outcome of Levene's test (If significant, variances are not equal) 3. Independence of observation - Can control for at the design stage of research Levene's example -  - Looking for insignificant p value (\>0.5) Factorial ANOVA example - We want to examine the effects of watching TV on **aggressive behaviour** in children. - We expose 3 groups of children to 3 different types of TV program: - one that is **non-violent** - one that features **Kung-Fu fighting** - one that features **domestic violence** - We also set up 2 different lengths of exposure time: - **2 hours** or - **6 hours** - Descriptive stats - means - Table Description automatically generated - Factorial ANOVA table - Relationships on the summary table: - Exactly the same as for the simple 1-Way ANOVA - Total degrees freedom = n-1 - Only new thing is that df~AB~ = df~A~\*df~B~ - Each main effect has the same variation (SS) and variance (MS) as with a one-way ANOVA - Factorial ANOVA reduces the MSerror Interpretation of the factorial ANOVA table - The main effects and the interaction effect are both sig. - **Rule:** - If the interaction is significant, interpret this first - If there is \> then interpretation of main effects needs caution - If the interaction is not significant, interpret main effects first - Main effects: indicates the differences between the marginal means of each factor (or IV). - **Exposure**: 2hrs mean is significantly different from the 6hrs mean - Only do further testing if there are 3 or more levels (eg not for exposure because only 2 levels) - **Type of violence**: There is at least one significant difference among the types of violence means. - must do further testing because there are 3 levels - Interaction effect: reflects differences between the cell means. - It indicates that the pattern of differences in aggression scores across types of violence (non-violent, Kung Fu, domestic violence) is different within each of the two exposure conditions. Interpreting main effects - NB: Interpret main effects with caution when the interaction effect is significant. - Main effects: - **Exposure**: 2hrs mean is significantly different from the 6hrs mean - Exposure only has two levels, so we can interpret the effect by comparing the means - The 6hr group (*M* = 5.6) scored significantly higher on aggression than the 2hr group (*M* = 2.8). - **Type of violence**: There is at least one significant difference among the types of violence means. - Post hoc tests - **Multiple comparisons** - Omnibus tests like ANOVA can only tell us when a significant effect is present, but it can not tell us where the effect lies (i.e., which means are different). - Post-hoc tests can help us identify the source of the significance. - Modified *t*-tests that control for FWER - Tukey's Honestly Significantly Difference test (HSD) - Tukey's HSD - Always come back to descriptive stats - Interpreting interaction effects - An interaction occurs when the effects of one independent variable depend upon the level of the other independent variable - The factors interact with each other in determining the values of the dependent variable - Tools to interpret interaction effects - **Cell mean plots** (visual pattern of interaction) - **Simple effects analysis** (one-way anova's; effect of an independent variable (e.g., type of violence (all 3 levels)) at one level of a second independent variable (e.g., 2 hrs (one level)). - Mini anovas across the different interactions - Note: Simple effects analysis is not a post hoc test. It is an a priori test (guided by theory), whereas post hoc is posteriori and requires adjusting for multiple comparisons. Steps in interpreting an interaction - **Cell mean plots** \> where do variables appear to interact? - This plot suggests that at 6hrs viewing Domestic Violence has a marked effect - While this view suggests the same regarding Domestic Violence - Note that there are 2 views of the same data - They both clearly show the interaction: - different types of TV program have different impacts on aggression depending on the length of exposure to the programs. - For example, it looks like domestic violence has a strong effect, but only at the longer level of exposure - Types of interactions - Ordinal \> Have simple effects that are in the same direction - *On the cell mean plot, the lines do not cross* - Disordinal \> Have simple effects that are in opposite directions - *On the cell mean plot, the lines cross* - When the lines cross on the cell mean plot à they represent opposing effects à one line shows increasing means, while the other shows decreasing means - For this reason, when you have a disordinal interaction, be cautious about interpreting main effects -- **the main effects don't reveal NB underlying differences** - No interaction \> *Parallel lines on the cell mean plot indicate that there is no interaction* - **Simple effects analysis**: - Your cell mean plots will help you describe interactions. But you need to run simple effects analyses to determine where the significance within the interaction comes from: - One way ANOVAs across each level of your IVs - Example: 2 x 3 table -  A screenshot of a computer Description automatically generated -  - Simple effects Table Description automatically generated - Six hour is significant (0.001 \< 0.05) - UNLESS TOLD OTHERWISE ALPHA IS 0.05 - Type of violence did have an effect on aggression at six hours - Type of violence did not have an effect on aggression at two hours - Kung fu does not have a significant effect across exposure levels - Only DV has significant effect across the two and six hour exposure levels Writing up your analysis - State the statistical hypotheses - Report descriptive statistics - Report on checks of assumptions - Report the overall F test - Interpret your analysis of the interaction -- where this is\ significant the interaction is more important; the interpretation of the main effects must make sense in terms of the interaction - Interpret your analysis of the main effects #### (Tredoux & Durrheim, 2018) Ch16 Factorial ANOVA --------------- Exam Structure - MCQs (15)-- theory, concepts, and applications. - Applied exercises - Similar in format to questions and exercises in tutorials - Four parts: chi-square (20), t-tests (10), ANOVA (15), Factorial ANOVA (15 - Formula sheet will be provided - Guidelines for submitting answers on Vula: - All answers, three decimals (including scientific notation, e.g., 5.486005E-07 should be submitted as 5.486E-07). - Accepted characters note. - "Next", "Previous", and "Save". Revision guidelines - Need to be able to state research hypotheses (for all types of tests) - Know your assumptions for each test. - Know how to apply all your formulas! - Understand Type I (& Type II errors). - Need to be able to interpret results... - Chi-square: Know how to interpret the direction of association - Look to adjusted residuals (significant if z value is above +1.96 or lower than -1.96) - T-tests: Decide if your hypothesis is 1 or 2-tailed - Directional or non directional - ANOVA: Know the limitations of what you can interpret... - Without post hoc test \> cannot decide where significance comes from ### Chi-squared - Categorical data - Assumptions: - Mutually exclusive - Exhaustive - Independent - Kinds - Goodness of fit (one-way chi-square) -- testing whether data fits expected pattern - One independent variable, check if difference between expected and observed is significance - Contingency (2/more categorical variables) -- are 2/more variables associated - Formula: - Contingency table - To find p-value - EXCEL: CHIDIST (value, df) - **Post-hoc:** - To isolate sources of association: - Calculate standardised residuals - Then *adjust* your residuals (into z-scores)  - Then p (using NORMSDIST function) ### T-tests - Continuous data - Formula - **Assumptions** - Normality - Homogeneity of variance (k ratio) -- calculate! - Independence - **Decision-making errors:** Type I and Type II - **NB: Decide if 1/2-tailed** - Three types - **One-sample T-test:** - Calculate t - P-value: use TDIST function - **Independent samples T-test** - Compare variances (k-ratio) - P-value: use TTEST Function - **Paired samples/Repeated measures T-test** - Calculate t (using difference scores) - OR Use TTEST function ### ANOVA - T-tests vs ANOVA - What is difference, why is it meaningful? - Multiple comparisons problem - Familywise Error Rate (↑Comparisons=↑Overall Type 1 Error rate) - ANOVA = different approach - Omnibus test - Indicates significant effect - Assumptions: - homogeneity of variance, normality, and independence - Variance = average distance of scores from their center - s^2^ = - This formula & idea of variance forms basis of ANOVA - SS = sum of squares = numerator of formula; i.e., - df = degrees of freedom = denominator; i.e., n-1 - MS = mean squares = variance; i.e., the whole formula s^2^ = - Error (random) variance vs systematic variance - Error variance refers to the random variance in sample means (...unexplained variance) - Systematic variance refers to the variance in the sample that **is due to the action of the independent variable (IV)** - ANOVA is used to isolate the systematic variance ANOVA table  - Larger F value = more significant (F\>1) Calculating ANOVA - **Relationships in the summary table (One-way ANOVA)** - SS~TOTAL~ = SS~EFFECT~ + SS~ERROR~ - df~TOTAL~ = df~EFFECT~ + df~ERROR~ - MS = SS/df Formulas for calculating SS Text, letter Description automatically generated Degrees of freedom - df~TOTAL~ = N -- 1 - where N is the total number of participants - df~ERROR~ = k(n-1) - where k is the number of groups - df~EFFECT~ = k -- 1 Formulae for calculating MS  ### Factorial ANOVA - maybe cell means plot, will mostly just have to interpret - 2/more independent variables - Main effects and interaction effects - Must be able to state research hypotheses (x 3 H0) - SS~TOTAL~ split into SS~GROUPS~ and SS~ERROR~ - SS~GROUPS~ then further split into SS~A~, SS~B~ & SS~AB~ (Variance due to factor A, variance due to factor B, and variance due to the interaction of A and B) - Partitioning variability in Factorial ANOVA - Must be able to understand/interpret statistical outputs - (factorial) ANOVA table - Levene's test \> what it is, how to work out whether there is homogeneity of variance - Tukey's vs simple effects - Tukeys is adjusted t test - Simple effects is adjusted anova - Must know when to use them - To interpret interaction: - Look at cell mean plots - Ordinal/disordinal - Simple effects - Equivalent to ANOVA - Look at effect of one independent variable within another independent variable - To interpret main effects: - Look at marginal means - Marginal means are the main effects - Post hoc tests -- Tukey's HSD - Use tukeys when there are more than two variables to determine which is significant - Compares different means with each other - Levenes \> you want your p value to be higher than.05 because you want to accept hypothesis that variances are equal