PSCI LECTURE SLIDES PDF
Document Details
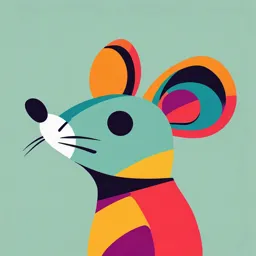
Uploaded by ConscientiousEvergreenForest1127
Carleton University
2024
Michael E. Campbell
Tags
Summary
These are lecture slides for a PSCI 2702(A) course on Quantitative Research Methods in Political Science at Carleton University, facilitated by Michael E. Campbell in 2024. Topics covered include course description, learning objectives, research methods, the scientific method, variables, levels of measurement, and examples of empirical research.
Full Transcript
Quantitative Research Methods in Political Science Lecture 1: Introduction to Quantitative Research Methods Course Instructor: Michael E. Campbell Course Number: PSCI 2702(A) Date: 09/05/2024 ...
Quantitative Research Methods in Political Science Lecture 1: Introduction to Quantitative Research Methods Course Instructor: Michael E. Campbell Course Number: PSCI 2702(A) Date: 09/05/2024 Course Description Purpose of course: to arm you with knowledge required to conduct research using quantitative research methods Overtime, you will be introduced to more complex techniques/formulas to analyze data A mixture of theory and practical knowledge (divided between lecture and tutorial) You will learn the systematic processes underpinning empirical research methods in political science Learning Objectives Learning-Outcomes 1. Understand the purpose and advantages of social scientific research 2. Comprehend foundational concepts and operations associated with empirical data analysis 3. Effectively interpret and evaluate data 4. Utilize various statistical techniques used for data analysis and hypothesis testing Format Lectures versus Tutorials Weekly Lectures: Thursdays from 9:35 to 11:25 Lectures: focused on foundational concepts, theories, and formulas Tutorials: Before or after Tutorials: focused on use of lecture, depending analytical software (SPSS) on group Office Hours and Communication E-mail TAs with Instructor: Michael Campbell Instructor Office Hours: TBA questions or Instructor E-mail Address: [email protected] concerns T.A.: Rohit Samaroo T.A. E-mail Address: [email protected] T.A.: Kaia Goodhope Cc Instructor on e- T.A. E-mail Address: [email protected] mail Course Materials Required Textbook Downloads Textbook: Healey, Joseph F. Christpher Donoghue, and Steven 1. SPSS Analytical Software Prus. 2023. Statistics: A Tool for Social Research, 5th ed. Toronto, ON: Cengage. 2. Varieties of Democracy Data Textbook is on reserve at library Links for each on Additional Readings: found on Brightspace ARES reserves via-Brightspace or Carleton Library Website Grading Breakdown Grading Breakdown Late Penalties 1. Tutorial Attendance (10%) No due date 5% late penalty / day 2. Assignment #1 (10%) 10 October (11:59PM) without valid reason for extension 3. Midterm Exam (25%) 17 October (in-class) 4. Assignment #2 (20%) Any assignment 5 December (11:59PM) submitted 7-days late will 5. Final Exam (35%) not be graded (without TBA (during exam period) valid reason for extension) Introduction to Quantitative Research Methods Research: “any process by which information is systematically and carefully gathered for the purpose of answering questions, examining ideas, or testing theories” (Healey, Donoghue, Prus 2023, 10). What are Quantitative research make use of statistical Quantitative analysis Research Statistics: “a set of mathematical techniques Methods? used by social scientists to organize and manipulate data for the purposes of answering questions and testing theories” (Healey, Donoghue, Prus 2023, 10). Think of statistics as tools that will tell you specific information about your data Systematically means that quantitative research follows a series of predetermined steps The Scientific Method The Scientific Method 1. Identify the problem (Dependent Variable) 2. Hypothesize a cause (Independent Variable) 3. Define the concepts (what are we talking about?) 4. Gather the empirical data (measurement) 5. Test the hypotheses 6. Reflect on theory 7. Publicize the results Components of The Scientific Method 8. Replicate the analysis were developed by several thinkers, but empirical science can be traced to Natural Vs. Social Sciences The Natural Sciences: The Social World: Studies natural Is unpredictable phenomena (physics, biology, chemistry) Subject to more ‘Noise’ Is predictable less ‘Noise’ Deals in Facts Less control than natural sciences The Social Sciences: Studies society and behavior Data are less Deals in Facts & Values valid/reliable Facts vs. Values Q1: At what temperature does sand turn Facts vs. to glass? Values Answer: Between 1700 °C and 2000 °C OR… Facts = What Is (Empirical/Objective) Q2: What was voter turnout in among Canadians who were eligible to vote in the 2021 Federal election? Values = What Ought to Be Answer: 17.2 million people (or roughly (Normative/Subjective) 62.6% of the eligible electorate) These are Questions of Fact What happens if are questions normative? Q3: Should/Ought sand turn into glass at 1700 °C to 2000 °C? Facts vs. Values Answer: Why even ask? (The results will never change because it Cont’d is based in the natural sciences…) Q4: Should/Ought we increase voter turnout? Answer: Requires us to make a value judgement – i.e., “what is an appropriate level of voter turnout?” (Based in the social sciences…) Value Judgement: a choice between things we believe are right or wrong Quantitative Research Methods = minimize personal opinions/biases as much as possible Opinions are empirically testable (do data support your beliefs?) Results of research should not reflect opinions, opinions should reflect results of research Goal of quantitative methods is to make the research process as objective as possible Will never be completely objective...because we must make Value Judgements e.g., conceptualization and operationalization, selecting variables to represent concepts, etc. Value Judgments The Role of Statistics in Social Science Theory: a statement about Research the relationship between phenomena The Wheel of Science Hypothesis: a statement about the relationship between variables Observations: what we see when we study our data Generalizations: summary patterns observed in our Source: W. Wallace. 1971. The Logic of Science in data concerning Sociology. Sourced from Healey, Donoghue, and Prus. 2023. expectations about Statistics a Tool for Social Research and Data Analysis. 5th Empirical Research Example Hypotheses: the higher the level of household internet access, the higher the level of voter turnout Theory: the more Observations: study informed an data and make electorate, the more observations (identify likely they are to relationships, participate politically patterns, etc.) Empirical Generalizations: in countries where there are more households with access to the internet, there tends to be higher levels of voter turnout The Value of Statistics in Political Science Good social science is both empirical and normative – because it deals in both facts and values Common argument (but wrong): ‘social science is not a science because science must be value free’ The better we answer questions, the better equipped we are to understand the world Quantitative methods are not better than qualitative methods, but provide reliable picture of reality A note on Methods vs. Methodology: Methods: tools we use to conduct research (think about different tools in a toolbox) Methodology a concern with the logical structure and procedures of scientific inquiry Therefore, methods are tools that researchers use to collect and analyze data, and methodologies are justifications made by the researcher for the use of these tools Variables and Levels of Measurement Introductio n to Variables Characteris Variables have three primary characteristics: tics of 1. Response categories must be mutually Variables exclusive 2. Response categories must be exhaustive 3. Response categories should be homogenous Mutual Exclusivity Response categories must Not Mutually Mutually Exclusive not overlap Exclusive 18-24 18-24 Each observation should 23-34 25-34 belong to only one 34-44 35-44 category 45-54 45-54 Above 54 Above 54 Example: a survey question asks you to Categories on the left overlap. This is corrected identify which age group by ensuring the categories do not overlap. you fall into Exhaustiveness A variable must Not Exhaustive Exhaustive encompass all possible Red Red categories or value Blue Blue If observations are left Green Green unclassified, variable is Yellow Yellow not exhaustive Other Example: a survey asks On the left, not all colors are accounted for. This respondents for their is corrected by adding “other” category. favorite color Homogeneity Categories should be Not Homogenous Homogenous consistent, measuring the Ford Ford same characteristics or attributes Suzuki Suzuki Honda Honda Categories represent the Tomato Other same underlying concept – to ensure consistency Other and comparability On the left, a tomato is unrelated and does not measure the same concept as other categories. Example: a survey asks This is corrected by eliminating that category. Discrete and Continuous Variables Discrete Variables Continuous Variables Variables whose basic Variables whose subunits can subunits cannot be divided be subdivided infinitely Will always be a whole Can have decimal points (but number may not) Example: the number of Example: time can be people living in a household measured in hours, minutes, (there won’t be 1.7 people seconds, etc. (10 minutes = living in a house) 600 seconds = 0.17 hours) Levels of Measurement Levels of Measurement Level of measurement of a variable: “The mathematical nature of variables under consideration” There are three levels of (Healey, Donoghue, and Prus 2023, 22). measurement: 1. Nominal (least precise) In other words, the level of 2. Ordinal measurement refers to the degree 3. Interval-Ratio (most of precision with which a variable precise) measures the empirical characteristic it is supposed to. We can determine the level of measurement by looking at a variable’s response categories. Nominal Level of Measurement Nominal variables classify observations into categories The categories are different, but cannot be more-or-less / higher-or-lower than another Therefore, the categories and cases cannot be ranked Categories can only be counted and compared Nominal Variable Example Religious Affiliation Ontario Area Codes Religion Type Frequncy Area Code Frequency Christianity 10 613 10 Judaism 22 753 22 Islam 15 683 15 Hinduism 19 437 19 Buddhism 7 365 7 Other 12 Other 12 Ordinal Level of Measurement A higher level of measurement than Question: How would you rate your shopping nominal level variables experience at the Carleton Bookstore? Response Frequency Contain scores or categories that Category can be ranked from high to low Very Good 10 Somewhat Good 25 Categories/cases can only be described as “more or less” or “higher or lower” Neither Good nor 7 Bad Often found in public opinion Somewhat Bad 27 surveys Very Bad 36 Cannot distinguish distance between Interval-Ratio Level of Measurement The highest and most precise level of measurement Variables measured at this level have equal intervals – i.e., scores are equidistant Example: income – difference between $1.00 and $2.00 is $1.00 Ratio level variables have a naturally occurring zero – interval variables do not All mathematical operations are possible with interval-ratio variables (addition, subtraction, division, etc.) Interval-Ratio Variable Examples Ratio Interval Respondent Hours Spent Day Temperature in Studying Per Celcius Week Monday 0 (temp. still exists Michael 0 (real absence of and can go lower) time) Tuesday 3 Brittany 1 Cenk 2 Wednesday 15 Tim 3 Thursday 7 Muhammad 4 Friday 2 Amanda 5 Hank 6 Saturday 3 Aisha 7 Sunday 1 Aki 8 Characteristics of Levels of Measurement Levels of Measurement Summary Level of Examples Measurement Mathematical Measurement Procedures Operations Permitted Nominal Gender, race, Classification into a) counting number religion, martial categories of cases in each status category of the variable b) Comparing sizes of categories Ordinal Socioeconomic Classification into All operations above, status, attitude and categories plus as well as opinion scales ranking of categories judgements of with respect to one “greater than” and another “less than” Interval Age, number of All of the above, plus All of the above, plus children, income description of all other distances between mathematical scores in terms of operations (addition, Quantitative Research Methods in Political Science Lecture 2: Conceptualization and Operationalization Course Instructor: Michael E. Campbell Course Number: PSCI 2702(A) Date: 09/12/2024 The Wheel of Science Source: W. Wallace. 1971. The Logic of Science in Sociology. Sourced from Healey, Donoghue, and Prus. 2023. Statistics a Tool for Social Research and Data Analysis. 5th ed. Causality is the idea that one thing causes another thing to happen In statistics, causation means Causation and variation in one variable causes variation in another variable Causal Think of it in terms of Cause and Relationships Effect When thinking about causation, consider: 1. Covariance of Phenomena 2. Temporality 3. Causal Mechanisms/Pathways 4. Non-Spurious Covariance Covariance and Temporality and Causal Pathways Covariance Temporality Temporality: the point in time The identification of a patterned at which the phenomena you relationship between phenomena are studying occur For instance, let’s say you have two For causation to occur, one variables each representing a thing must precede the other concept… in time These variables must vary together in Example: Smoking causes some way Cancer For example, as the values on one The act of smoking must variable increase or decrease, the precede the cancer diagnosis values on another variable must increase or decrease Causal Mechanisms Causal mechanisms can be understood as the pathways through which an outcome occurs Can also think of it in terms of the explanatory links between variables Example: Smoking causes Cancer – but how? Carcinogens inhaled from cigarettes alter the DNA in your lung cells resulting in the production of cancer cells This is based on the theory of carcinogens Visualize all of the causal mechanisms in a game of Mouse This is why we use theoretical Trap In lecture 1 we theorized that the more informed an electorate, the higher the level of political participation We used two variables to represent these concepts: (1) # of households with internet access; (2) voter turnout We hypothesized that in countries where more households Causal had internet access, the level of voter turnout would increase Mechanism But it is not necessarily internet access that is causing variation in the level of voter turnout… Example Instead, it might be that when more people have access to the internet, the more likely they are to have information about politicians’ policies Therefore, the causal mechanism is… More households with internet access more information about policies provided to constituents likelihood of voting increases Non-Spurious Covariance The presence of covariance does not necessarily mean causation Always remember, “correlation does not mean causation.” Just because two things co-occur, does not mean that one causes another For example, winter does not cause spring (even if it always precedes it in time) Therefore, always be sure that variance in one thing is the cause of variance in the other, and that it isn’t happening by random chance Causality and Variables (Independent and Dependent) Independent Variables Dependent Variables Represented by symbol X Represented by symbol Y Variation in the The dependent variable is independent variable is hypothesized to vary hypothesized to cause because of variation in variation in the dependent the independent variable variable Independent and Dependent Variables Cont’d Smokin Cance g r The The Independe Depende nt Variable IV will always nt (X) precede the DV in Variable (Y) Occurs at time Time 1 Occurs at Time XY 2 Confounding Variables Related to the idea of spurious covariance Example: you find covariance between high ice cream sales and more drownings Therefore, you might assume high ice cream sales cause more drownings But in reality, it is something else causing variation in both variables… Confounding Variables Cont’d In our example, it is the temperature which is affecting variation in both variables… X Ice Cream Sales High temperature causes more people to buy ice High Z cream and to go swimming Temp. The more people swim, the more likely they are to drown Y # of Spurious Relationship: Drownings when there is seeming Therefore, when a spurious relationship exists and is controlled for, the apparent relationship association between two between X and Y disappears variables, but another variable is the true source of variation in Confounding variables are represented by symbol Z each. Therefore, we must anticipate alternative Conceptualizati on and Operationalizat ion Conceptualization and Operationalization “Politics…is all about making choices” (Pollock III and Edwards 2020, 1). Concept: “an idea or mental construct that organizes, maps, and helps us to understand phenomena in the real world and make choices” (Pollock III and Edwards 2020, 1). In other words, concepts are abstractions… Concepts can be more or less complex – e.g., “globalization,” “power,” and “democratization” are all concepts Question: What is a political Party? (seems simple enough to answer) Example: ‘Political Party’ as a Concept Political Thinker Definition Edmund Burke (1770) “[A] party is a body of men united, for promoting by their joint endeavors the national interest, upon some particular principle in which they are all agreed.” Anthony Downs (1957) “[A] political party is a coalition of men seeking to control the governing apparatus by legal means. By coalition, we mean a group of individuals who have certain ends in common and cooperate with each other to achieve them.” V.O. Key, Jr. (1964) “A political party, at least on the American scene, tends to be a “group” of a peculiar sort…Within the body of voters as a whole, groups are formed of persons who regard themselves as party members…In another sense, a “party” may refer to the group of more or less professional workers…At times party denotes groups within the government….Often it refers to an entity which rolls into one the party-in the-electorate, the professional group, the party-in-the-legislature, and the party-in-the-government…In truth, this all encompassing usage has its legitimate application, for all the types of groups called party interact more or less closely and at times may be as one. Yet both analytically and operationally the term ‘party’ most of the time must refer to several types of group; and it is useful to keep relatively clear the meaning in which the term is used.” William Nisbet “[A] political party in the modern sense may be thought of as a relatively durable social formation which seeks offices or power Chambers (1967) in government, exhibits a structure or organization which links leaders at the centers of government to a significant popular following in the political arena and its local enclaves, and generates in-group perspectives or at least symbols of identification or loyalty.” Leon D. Epstein (1980) “[What] is meant by political party [is] any group, however loosely organized, seeking to elect government office holders under a given label.” Ronald Reagan (1984) “A political party isn’t a fraternity. It isn’t something like the tie you wear. You band together in a political party because of certain beliefs of what government should be” Robert Huckshorn “[A] political party is an autonomous group of citizens having the purpose of making nominations and contesting elections in (1984) hope of gaining control over governmental power through the capture of public offices and the organization of the government.” Joseph Schlesinger “A political party is a group organized to gain control of government in the name of the group by winning election to public (1991) office.” Even if a concept seems simple, it can nevertheless mean many different John Aldrich (1995) “Political parties can be seen as coalitions of elites to capture and use political office. [But] a political party is more than a Concepts Concepts can be more-or-less complex – e.g., “globalization,” “power,” and “democratization” are all broad concepts What does someone mean when they refer to a concept? It is hard to tell because concepts are ideas Example: you have a research question: “does globalization exacerbate climate change?” It is impossible to answer the question in this form, it is a conceptual question The concepts are too ambiguous for us to work with in these types of questions… Conceptual and Concrete Questions Conceptual Question: “a question expressed using ideas, is frequently unclear and is difficult to answer empirically” (Pollock III and Edwards 2020, 2). To answer a conceptual question requires the process of conceptualization and operationalization… This will transform concepts into concrete terms so that can be described and analyzed In so doing, you can develop a concrete question Concrete Question: “a question expressed using tangible properties, [which] can be answered empirically” (Pollock III and Edwards 2020, 2). Conceptual Definition: “Clearly describes the concept’s measurable properties and specifies the units of analysis (e.g., people, nations, states, and so on) to which the concept applies” (Pollock III and Edwards 2020, 2). They are more precise and clear expressions of Conceptual phenomena under review Definitions Can only be written once you have selected a set of properties that best represent the concept “Communicates the subjects to which the concept applies and suggests a measurement strategy” (Pollock III and Edwards 2020, 3). Operational Definition: “describes the instrument to be used in measuring the concept and putting a conceptual definition “into operation”” (Pollock III and Edwards 2020, 8). In other words, it indicates how we know if there is more or less of a phenomenon Operational Definitions An operational definition will “describe explicitly how a concept is to measured empirically” (Pollock III and Edwards 2020, 10). Example: you are measuring height. Operational definition: “Height” is defined by the number of feet/inches a person is tall.” The Process of Conceptualization and Operationalization Step 1 Step 2 Step 3 Develop Clarify the Conceptual Operational Concept Definition n Communic Dev Select ate ope essential necessary l de characteris definitional Dev tic(s) componen inst ts atio The first step of clarification is to identify the concepts concrete properties – i.e., a list of properties that best represent the concept Step 1: Properties have two characteristics: Clarify the 1. They must be concrete (i.e., they must be perceptible, meaning we must be able to observe them) Concept 2. They must vary (i.e., they must occur or not occur, or occur at different levels) Example: Does globalization exacerbate climate change? Begin by identifying the concepts in the conceptual question In this case: (1) globalization; and (2) climate change Example: Conceptual Clarification of “Globalization” Globalization has certain characteristics associated with it (a general understanding exists) You must identify these essential characteristics – which must be concrete and variable Identifying these characteristics will reduce conceptual ambiguity Can be identified using intuition or through research (use research in assignments!) Conceptual Clarification of “Globalization” Cont’d When you identify the characteristics, you then narrow them down to identify the most essential characteristic(s) International Monetary Fund (IMF) identifies four characteristics of “Globalization”: 1. Trade and Transactions 2. Capital Investment and Movement 3. Migration and the Movement of People 4. The dissemination of Knowledge Each of these are tangible properties, because we can perceive them (and measure them directly) Globalization – Essential Characteristics Not only do we need to select a characteristic that best represents the concept, but it must be consistent with the context of our research. And the data available to us. Selection requires that we make a value judgement! While multidimensional concepts exist, “as much as possible, you should define your concepts in clear, unidimensional terms” Globalization – Essential Characteristics (Pollock III and Edwards 2020, 7). Cont’d Justification for trade and transactions: they are legally binding and necessarily tie different countries closer together. We might then argue that higher levels of trade represent higher levels of transnational integration. Simultaneously, there may be justifications why the other characteristics may not represent our concept as well as trade… Globalization – Essential Characteristics Cont’d Capital and investment movements can increase without the presence of global institutions or regulations that guide and harmonize these activities on a global scale Globalization – Essential Characteristics Cont’d Migration of people across borders occurs within specific regions or between neighboring countries (e.g., in the European Union or in South America), but they can increase without impacting global migration trends. Globalization – Essential Characteristics Cont’d Knowledge dissemination can increase without being global if access is limited to certain regions, countries, or socio- economic groups. For instance, digital divides and disparities in education mean that increased knowledge flow might not be global in Globalization – Essential Characteristics scope. Cont’d Globalization – Essential Characteristics Cont’d Step 2: Develop Conceptual Definition Once you have identified your concept’s essential characteristic, you need to develop a conceptual definition Conceptual Definition Template (See: Pollock III and Edwards 2020, A conceptual definition must 7): communicate three things: 1. The variation within a measurable characteristic (or “The concept of ________ is defined as set of characteristics) the extent to which ________ exhibit the characteristics of ________.” 2. The subject or groups to which the concept applies (unit of analysis) 3. How the characteristic is to be measured The concept of (A) globalization is defined as Step 2 - the extent to which (B) countries exhibit the characteristics of (C) high levels of trade Develop and transactions Conceptual Components represent: Definition A. By stating that (A) globalization is defined by the extent to which, it restates the name of the broad idea Cont’d being conceptualized, but it also points to the existence of variation, meaning that it can exist in varying levels, or not at all B. By identifying (B) countries, we are identifying the subjects to whom the concept applies C. By stating (C) high levels of trade and transactions, we’re identifying the way that the concept can be measured The unit of analysis is the entity to which your concept applies, and the entity we want to describe and analyze There are two levels of analysis: 1. The Individual Level of Analysis Is oriented towards the study of individual political Unit of behavior At this level, we can learn something about individual preferences and attitudes about something in particular Analysis 2. The Aggregate Level of Analysis: Is a collection of individual entities For instance, countries are studied at the aggregate level, because they are aggregations of multiple entities Warning: be careful when applying aggregate level results to make inferences at the individual level – it risks an Ecological Fallacy Step 3: Develop Operational Definition (Operationalization) Operational definitions are extensions of conceptual definitions that explicitly state how the concept will be measured empirically For example, how would we know if trade was occurring at a high levels among countries? Example operational definition of Trade and Transactions: the total amount of imports and exports of a country, in millions of current year US dollars Operational definitions are almost always accompanied by an instrument Instruments are tools used to measure a specific concept, variable, or phenomenon in a systematic and reliable way Instrument Example Please respond to the following. On a scale of 1 to 10, with 10 being “Very High,” and 1 being “Very Low,” rate your shopping experience Instruments can be simple or complex depending at Sephora… on complexity of characteristic you are measuring 1 – Very Low 2 Example: You want to know peoples’ satisfaction 3 with shopping experience at Sephora 4 5 Operational definition: The shopping 6 experience at Sephora is defined as the overall 7 satisfaction of customers during their visit to the 8 store 9 10 – Very High To the right is an instrument that will measure ordinal level data Measurement Error It is important that instruments measure intended characteristics, as opposed to unintended characteristics You want to maximize the fit between the definition of the concept and the empirical measure of the concept Otherwise, your data may contain 1. Systematic Measurement Error (a.k.a. Measurement Bias) 2. Random Measurement Error These types of error disrupt the link between the concept and the empirical measure Systematic Measurement Error Systematic Measurement Error: “introduces consistent, chronic distortion into an empirical measurement…[by producing] Imagine if the above instrument added operational readings that consistently mismeasure the characteristic the or omitted a key trait. researcher is after” (Pollock III and Edwards 2020, 13). For example, what if it measured only the imports of a country and not the Occurs when instrument captures exports. unwanted traits, or omits necessary traits that artificially inflate or deflate It would provide data on trade that were what you are trying to measure consistently lower every time it was used. There would be inconsistencies between the observed and true values of trade. Systematic Measurement Error Visualized True mean value Observed mean with properly value of trade if defined instrument exports are that accounts for omitted from imports and instrument exports The presence of systematic measurement error will create bias in estimation on every single occasion because error is built into Is the result of random chance. Random measurement error introduces “haphazard chaotic distortion into an empirical measurement, producing inconsistent operational readings of a concept” (Pollock III and Edwards Random 2020, 13). Measureme Even if instrument is free from systematic error, nt Error there is a random chance the measurement will be wrong Example: you are measuring trade in a country whose main export is lumber, but there was a significant lumber shortage that year (resulting in lower observed values) Resolve by looking at mean value over time Validity and Reliability Reliability The validity ofValidity a measure is “the extent to which it records the true The reliability of a measure is “the extent value of the intended characteristic to which it is a consistent measure of a concept” (Pollock III and Edwards 2020, and does not measure unintended 16). characteristics” (Pollock III and Edwards 2020, 17). To be reliable, the measurement should give the same reading every time Even if reliability is lacking, we can assume that if we used the measure A measure can still have systematic error enough over time that the problem of and be reliable, but results must be random error would correct itself and consistent reflect the true value of the characteristic As random error increases, reliability is negatively affected because the results Thus, we can say validity is more will be more inconsistent important than reliability (but both are still important) Reliability and Validity Visualized Validity and Reliability Visualized Cont’d Reliable Results consistent Results consistent but do not across contexts and accurately the measure represent concept accurately Invalid Valid represents concept Results Results inconsistent inconsistent across contexts but and do not measure accurately accurately represents concept measure concept Unreliable Step 4: Variable Selection Example: Once you have your operational Does Globalization exacerbate Climate definition and instrument, you can begin Change? to collect data Globalization conceptualized as Trade Operationalized to measure countries’ The result will be a variable that imports / exports Therefore, I can select the following variable represents the characteristic(s) of the concept you were trying to measure If you are not collecting the data yourself, though, you can select a variable that best reflects your conceptual and operational definitions Source: Quality of Government (2022) Quantitative Research Methods in Political Science Lecture 3: Descriptive Statistics and Measures of Central Tendency and Dispersion Course Instructor: Michael E. Campbell Course Number: PSCI 2702(A) Date: 09/19/2024 Descriptive Statistics Statistics are used to summarize information about a variable or variables quickly and effectively Two types of descriptive statistics: Univariate Statistics: summarize or describe the distribution of a single variable Bivariate (or Multivariate) Statistics: summarize or describe the relationship between two or more variables Proportions and Percentages Proportions and Percentages Used to standardize raw data and compare parts of a whole Can be used to compare parts of a whole or groups of different sizes Standardization: to transform the unit of measurement so that it can be compared to other values on a common scale For example: Proportions always on a scale of 1.00 Percentages always on a scale of 100 Proportions The formula for proportions is: In this equation: f is the total number of all cases in any category n the number of cases in all categories Percentages The formula for percentages is: In this equation: f is the total number of all cases in any category n the number of cases in all categories Proportions and Percentages Example Most Popular Ways for Canadians to Celebrate St. Valentines Day Ways to Celebrate Frequency Proportion Percentage Going to a restaurant 312 0.3995 39.95 Romantic evening at 110 0.1408 14.08 home Giving a 92 0.1178 11.78 gift/card/flowers/chocol ate Going on a trip 22 0.0282 2.82 Going on out dancing 7 0.0090 0.90 Other 74 0.0947 9.47 Don’t Know 164 0.2100 21.00 Proportions and Percentages Example Cont’d Proportion of Percentage of “Romantic Evening at Home” “Romantic Evening at Home” Therefore… Therefore… “Out of 781 people surveyed, “Out of the 781 people surveyed, the approximately 14.08% of proportion who prefer celebrating St. respondents prefer a romantic Valentine’s Day with a romantic evening at home” evening at home is 0.1408” Comparing Groups of Different Sizes Example In the absence of a standardized statistic, it is very hard to compare relative group sizes because they the size of groups might be different… For instance, do more males or females pursue studies in business, Comparing Groups of Different Sizes Example Cont’d “Calculating percentages eliminates the difference in size of the two groups by standardizing both distributions on a base of 100” (Healey, Donoghue, and Prus 2023, 42). For instance, now we can see that a higher percentage of males (25.57%) study business, management, and Guidelines on Use of Proportions and Percentages 1. If you have fewer than 20 cases, report on frequencies With small number of cases, percentages can change drastically with only minor changes The higher the number of observations, the impact of each additional observation on proportions/percentages will be smaller 2. Always report the number of observations (n) along with proportions and percentages Allows the reader to judge the adequacy of sample size Helps to prevent use of misleading statistics 3. Proportions and percentages can be reported for variables at all three levels of measurement Ratios and Rates Ratios allow us to “compare the categories of a variable in terms of relative frequency” (Healey, Donoghue, Prus 2023, 45). We do not standardize when we calculate ratios (or rates) Ratios “tell us exactly how much one category outnumbers (or is outnumbered) by the other” (Healey, Ratios Donoghue, Prus 2023, 47). The formula for Ratio: Ratio = In this equation: f1 = the number of cases in the first category f2 = the number of cases in the second category Q: How would you describe your feelings towards the Sponsorship Scandal? Response Frequency Angry 3351 Not Angry 630 What is the ratio of Canadians who are angry about the Ratio Sponsorship Scandal to Canadians who are not angry? Example Ratio = Ratio = This means that “for every Canadian who is not angry about the Sponsorship Scandal, there are 5.32 Canadians who are angry about the scandal.” Ratios are often multiplied by some power of 10 (to eliminate decimal points) 5.32 x 100 = 532 Therefore, “for every 100 Canadians who are not angry Ratio about the Sponsorship Scandal, there are 532 Canadians who are angry about the scandal.” Example For greater clarity, comparison of units are usually Cont’d expressed Based on units of ones, the ratio of Canadians who think about the same as now or less would be expressed as: 5.32:1 Based on hundreds, this would be expressed as: 532:100 Rates The formula for rates is: Rate = In this equation: f actual is the number of actual occurrences of a phenomenon f possible is the number of possible occurrences of the phenomenon Are also multiplied by some power of 10 to eliminate decimal points A Country’s Death Rate is commonly used rate in research To determine death rate, divide the number of deaths (f actual) in a country by the total population (f possible) Rates In 2019, there was 284 082 deaths in Canada (f actual) Example Population of Canada was 37 590 000 (f possible) The death rate for Canada in 2019 would be: Rate = This leaves us with 0.006 (very small) Rates Example Cont’d To resolve, multiply by 1000 (common with death rates)… Rate = x 1000 Rate = 7.56 Therefore, for every 1000 Canadians, there were 7.56 deaths in 2019 Frequency Distributions Frequency distributions are different than instruments Instruments are measurement tools… Frequency distribution “is a table that summarizes the distribution of a variables values by reporting the number of cases contained in each category of the variable” (Healey, Donoghue, and Prus 2023, 49). Frequency distributions are a useful way of organizing and presenting data They are also one of the first steps in data analysis Frequency Distribution at Nominal Level Nominal-Level Variable Frequency Table (Employment Status) Employment Status Frequency Employed 36 Unemployed 14 Frequency Distribution at Nominal Level Cont’d Here is a distribution table reporting the types of electoral system in a country. Majoritarian systems are coded as 0.00 Proportional systems are coded as 1.00 Mixed systems are coded as 2.00 Other systems are coded as 3.00 See instrument on page 89- Frequency Distribution at Nominal Level Cont’d We see the same table with value labels now ( it is the exact same information as before) We see there are 40 observations (N) and 139 missing values Frequency column tells us the number of observations for each category Percent tells us percent of cases per category, but does not omit missing cases Valid percent column corrects for this Ordinal-Level Variable Frequency Frequency Table (Satisfaction Distributio with Meal) n at How Satisfied Were Frequenc Ordinal You with Your Meal? y Level Very satisfied 15 Somewhat satisfied 25 Somewhat dissatisfied 7 Very dissatisfied 3 Frequency Distribution at Ordinal Level Cont’d 179 Valid Cases (0 missing) Notice “Percent” and “Valid Percent” columns are now the same This is because there are no missing cases Public Sector Corrupt Exchanges are “Extremely Common” in 11 countries Public Sector Corrupt Exchanges are “Extremely Common” in 6.1% of countries Public Sector Corrupt Exchanges are “Extremely A lot of information presented here… 45 valid cases No repeating Frequency values, so each case equals 2.2% Distributio of valid cases n for Using cumulative percentage Interval- column, we can make statements Ratio like “In 20% of Level countries in our sample, voter turnout is 58.77% or less.” But these types of Frequency Distribution at Interval-Ratio Level Cont’d To make distribution table more manageable, researchers sometimes collapse data into groups… This is the same information as previous table…. Now, we have four categories 2.2% of countries in the sample have “Very Low” voter turnout (below 25.9%) 11.1% of countries in the sample have “Low” voter turnout (between 26 and 50.9%) Provide visual representation of data Graphs and Charts are often used by researchers to present their data in ways that are less confusing than just presenting statistics… Graphs and Charts These give us a general sense of the overall shape of the distribution These give us general sense about the way the data are dispersed Pie Chart Election Turnout 2% Very simple and 11% intuitive 36% Used when there are few categories 51% Rarely used in quantitative academic research Very Low Low High Very High Bar Chart Voter Turnout Categories of 60% variable along 51% horizonal axis 50% 40% 36% Frequencies (or 30% percentages) along vertical axis 20% 11% 10% Bar charts used 2% when there are four 0% or five categories Very Low Low High Very High Histogram Most appropriate for continuous interval-ratio data Categories of scores are contiguous (the bars touch each other) Here, rather than categories of voter turnout, we can use the original frequency distribution A line can be placed atop of to get a better sense of how the data are dispersed Measures of Central Tendency and Dispersion Measures of Central Tendency and Dispersion Measures of Central Tendency allow us to describe data so we can identify the typical or average case in Three Measures of Central Tendency: a distribution 1. Mode 2. Median They are statistics that help us 3. Mean summarize data so we can describe the most common scores, the middle case, or the average or all cases MCT statistics “reduce huge arrays combined of data to a single, easily understood number” (Healey, Donoghue, and Prus 2023, 80) Measures of Dispersion give us some idea about the level of heterogeneity (or how much variety) there is in a distribution Measures of Central Tendency and Dispersion Cont’d “For a full description of scores, measures The best Measures of Dispersion will: of central tendency must be paired with 1. use all the scores in a distribution, measures of dispersion” (Healey, meaning the statistic will be Donoghue, and Prus 2023, 80) computed using all the information that is available Measures of dispersion provide information 2. describe the average or typical about “the amount of variety, diversity, or deviation of the scores and give us an heterogeneity within a distribution of idea of how far the scores are from scores” (Healey, Donoghue, and Prus 2023, one another or from the center of the 80). distribution 3. increase in value as the distribution of scores becomes more diverse Data Dispersion Think about data dispersion in terms of homogeneity or heterogeneity of data The less spread out the data, the less dispersed it is, meaning it is more homogenous (See Essay Exam) The more spread out the data, the more dispersed it is, meaning it is heterogenous (See Multiple-Choice Exam) Please note that the average is the same in this example, despite differences in variability The Mode Is the most recurring value in a variable Example: you have a set of scores (8, 9, 9, 14, 17, 20). The mode is 9. The mode represents the variable’s largest category Is the only measure of central tendency that can be used with nominal data The mode in the above example is “Proportional” electoral systems Mode Limitations 1. Some distributions have no mode at all (no repeating values). Or some distributions have multiple modes (many repeating values). 2. With ordinal and interval-ratio data, the modal score “may not be central to the distribution as a whole” (Healey, Donoghue, and Prus 2023, 83) This suggests that the most common Although the mode is 93 in score may not be “typical” in identifying the above example, it does the center of a distribution not accurately convey the distribution, because the vast majority of students scored below that. Index of The IQV the only measure of dispersion that can be used with nominal level data Qualitative Variation The IQV is “the ratio of the amount of variation actually observed in a distribution of scores to (IQV) the maximum variation that could exist in a distribution” (Healey, Donoghue, and Prus 2023, 83). IQV ranges from 0.00 (no variation) to 1.00 (maximum variation) IQV Cont’d If everyone were indigenous (or non- indigenous) in Canada, the IQV would be 0.00 (no variation) If 50% of population were indigenous and 50% non-indigenous the IQV would be 1.00 (maximum variation) Looking at percentages in table, we see that indigenous population has been increasing over time IQV Cont’d The formula for the IQV is: In this equation: is the number of variable response categories is the sum of squared percentages of cases in the variable's response categories IQV for 1996: IQV A higher IQV means Cont’d = = 0.109 more dispersion in the data. IQV for 2006: As you see, the IQV = = 0.144 increases over time… IQV for 2016: Variation increases from 0.109 (10.9%) = = 0.185 to 0.185 (18.5%). Indigenous population is increasing. The Median The median represents the exact center score in a distribution Exactly half the cases will fall below the median, and half the cases above Example: Median household income in Ottawa is $88 000 This means that 50% of households make less than this, and 50% make more Median Cont’d Exam Score Frequency 58 2 72 3 Let’s say you have data on Exam Scores 75 1 from a class of 11 people… 79 2 To determine the median, you must order 80 1 the cases from lowest to highest (or highest 87 1 to lowest)…. 96 1 Total: 11 Median Cont’d Now that all scores are in order… Exam Score Frequency The median is exactly halfway between the 58 1 scores of the two middle cases 58 1 72 1 To identify the middle case, take the sample 72 1 size and add 1 then divide by 2 72 1 In this case it is (11+1)/2 = 6 75 1 79 1 In this example, the median is 75% (half the 79 1 cases are below it and half are above it) 80 1 87 1 But this was an odd number of cases… 96 1 What happens if you have an even number? Total: 11 Median Cont’d If you have an even number of cases, you Exam Score Frequency determine the median by adding the two 58 1 middle cases 72 1 To identify middle cases, divide N by 2 (this 72 1 gives you the first middle case) (10/2) = 5 72 1 Then, add 1 to that number (this gives you the 75 1 second middle case) (5+1) = 6 79 1 Therefore, the center scores here are 75 and 79 1 79 (there are four cases above and four cases 80 1 below them) 87 1 Add those numbers and divide by 2 96 1 Total: 10 (75+79) / 2 = 77 (the median is 77) The median can be used for interval Note: because the median requires scores to level variables, but is preferred for The range (and interquartile range) are measured of dispersion generally used for ordinal level data Range: “defined as the difference between or interval between the highest score (H) and the lowest score (L) in a distribution” (Healey, Donoghue, and Prus 2023, 88). Range The formula for Range: R=H–L -In this equation: -H is the highest score -L is the lowest score Range Example #1 Age of Students in a Class Age Frequency 18 2 R = 26 – 18 R=8 19 2 With this information we could say “The 20 1 age range of students in the course spans 21 2 8 years, with the youngest being 18 years old and the oldest being 26.” 22 1 24 1 However, the range is calculated using 26 1 only the highest and lowest scores and can be misleading if there is an outlier Range Example #2 Age of Students in a Let’s say that there was a retiree in the Classroom (with outlier) course, with an age of 65… Age Frequency R = 65 – 18 18 2 R = 47 19 2 As you can see, the range is now 20 1 misleading, suggesting the data are more 21 2 dispersed than they actually are 22 1 This is the result of the outlier (65) 33 1 Therefore, the range is limited in what it can 65 1 tell us abut the distribution of data Interquartile Range Quartiles represent the percentage of observations that fall within different segments of a dataset for the same variable The first quartile (Q1) is the first 25% of cases, the second is the second 25% of cases, and so on… There are four quartiles in total, each representing 25% of cases in a variable… Interquartile Range Cont’d The formula for the interquartile range is: In this equation: Q3 is the value of the third quartile Q1 is the value of the first quartile Interquartile Range Cont’d Q1 is the point below which 25% of the cases fall and above which 75% of the cases fall Q3 is the point below which 75% of the cases fall and above which 25% of the cases fall L represents the lowest score H represents the highest score Q is the range in the middle 50% of cases in a distribution It only uses 50% of cases, and will not be affected by outliers Interquartile Range Cont’d We know the range is 47 Age of Students in Class (with outlier) Now, you need to calculate Q Age Frequency (requiring you to identify Q1 and Q3) 18 1 18 1 To do this, find the median in the 19 1 ordered data 19 1 20 1 (n/2) + 1 – indicates cases 5th and 6th are center values 21 1 21 1 Median = (20+21)/2 = 20.5 22 1 33 1 Interquartile Range Cont’d You can now divide the data into 2 50% below 50% above sets the Median the Median Remember, 50% of cases fall above the median and 50% below (20.5) (20.5) Find the median for each set 18 21 Q1 = the median for the lowest half 18 21 of the data 19 22 Q3 = the median for the upper half of the data 19 33 Therefore… 20 65 Q1 = 19 Interquartile Range Cont’d Therefore, interquartile range is 3, as opposed to 47 Better than range, because it is not influenced by outliers Or Therefore, “the interquartile range is a more useful measure of dispersion than the range because it is not affected by extreme scores, or outliers” (Healey, Donoghue, and Prus 2023, 90) The formula for the mean is: The Mean (or In this equation: Arithmetic = the sample mean = the sum of scores Average) = the number of cases in the sample The sum of scores means that you add all of the scores in a distribution represents each individual score A Note on Notation If we were calculating for a population and not a sample, the notation would change The formula for the mean would be: In this equation: = the population mean = the sum of scores = the number of cases in the population Characteristics of the Mean 1. The first characteristic is that the sum of differences will always add up to 0 The mean is the center of the distribution Unlike median, it is the point around which all scores cancel out Symbolically, represented by: This means if we take each score from a distribution and subtract the mean from it, and all of those differences, the sum will always be 0 Characteristics of the Mean Cont’d Imagine you have 5 exam Sum of Differences scores in a sample: 65, 73, 77, 85, 90 65 65-78 = -13 73 73-78 = -5 77 77-78 = -1 85 85-78 = 7 90 90-78 = 12 = 390 As you see, the total negative differences will be exactly equal to the total positive differences Characteristics of the Mean Cont’d 2. The second characteristic is the least-squares principle Is expressed by the following statement: Suggests that the mean is the point in a distribution around which the variation of scores (as indicted by squared differences) is minimized In other words, if we square the differences between scores and add them together, the resultant sum will be less than the sum of squared differences between the scores and any other point in the distribution Least-Squares Principle Example Five exam sample scores: 65, 73, 77, 85, 90 We know the differences between these scores and the mean are -13, - 5, -1, 7, 12 If we square these differences and add them, we get a total of 388 If we use any number besides the mean, the result will always be higher Least-Squares Principle Cont’d 65 65-78 = -13 (-13)² = 169 65-77 = -12 = (-12)² = 144 73 73-78 = -5 (-5) ² = 25 73-77 = -4 = (-4)² = 16 77 77-78 = -1 (-1) ² = 1 77-77 = 0 = (0)² = 0 85 85-78 = 7 (7) ² = 49 85-77 = 64 = (64)² = 64 90 90-78 = 12 (12) ² = 144 90-77 = 169 = (169)² The least-squares principle tells us that the mean (or average) is =169 close to all of = 390the other scores than the other = measures 388 = 393 of central tendency Characteristics of the Mean Cont’d 3. Third characteristic is that every score in distribution affects the mean For example, take the same scores as previous example, but change the last score to 500… Neither the mode nor the median is affected by every score Now we have 65, 73, 77, 85, 500 The mean is calculated using all the The median is still 77 information available to us But now the mean is 400 This is an advantage as disadvantage The mean will always be pulled in the (because outliers can make the mean misleading) direction of outliers Symmetrical Distribution (Unskewed) The median and mean will only have the same value when the data are symmetrical Positive and Negative Skews When a distribution has some extremely high scores (high value outliers) the mean will always have a greater value than the median A Positive Skew When a distribution has extremely low scores (low value outliers) the mean is lower in value than the median A Negative Skew “A quick comparison of the median and the mean always tells you if a distribution is skewed and the direction of the skew” (Healey, Donoghue, and Prus 2023, 97) If you have a data that with a A Note on positive or negative skew, the median is a better measure of Mean and the central tendency to use Skew If the distribution is symmetrical, then the mean is the most appropriate measure of central tendency Computing the mean requires addition and division, therefore it should only be used with interval- ratio variables Variance and Standard Deviation Unlike range and interquartile range, variance and standard deviation use all of the scores in a distribution We must identify the distance Deviations smaller between each score in a distribution and the mean These distances are known as deviations (and deviations increase in size as the data become more heterogenic) Deviations larger If the scores are more homogenous, they will be clustered around the mean and the deviations will be smaller Using Deviations to Calculate Variance and Standard Deviation We can use deviations of scores to calculate useful statistics But we know the sum of deviation is always 0 So, we need to square the deviations However, the higher the number of cases, the higher the squared deviation value Scores Deviations Deviations Squared () () 65 65-78 = -13 (-13)² = 169 73 73-78 = -5 (-5) ² = 25 77 77-78 = -1 (-1) ² = 1 85 85-78 = 7 (7) ² = 49 90 90-78 = 12 (12) ² = 144 = 390 = 388 Variance Uses the squared deviations and divide by the number of cases – thereby standardizing distributions of different sizes Formula for variance: In this equation: is the score is the sample mean is the number of cases in a sample Standard Deviation To compute the standard deviation, you use the square root of the variance The formula for standard deviation is: In this equation: is the scores is the sample mean is the number of cases in a sample Standard Deviation Cont’d Our sum of squares was 388, with 5 cases in the sample. Therefore… The standard deviation is 8.81 Interpreting the Standard Deviation Standard deviation is a very important statistic (required for understanding Normal Curve) Is also an index of variability A larger standard deviation represents more dispersion, a smaller standard deviation represents less dispersion Has a low value of 0.00 (no variation in the data) Interpreting Standard Deviation Example Data represent daily temperatures for Calgary, Alberta, and Gander, Newfoundland and Labrador for the month Calgary Gander of January = -7.1 = -7.1 The Mean for each city is -7.1 Celsius = 4.5 =1.8 But standard deviation is higher in Calgary (why?) Because there is a greater level of variation in day-to-day temperature in Calgary than in Gander Calgary might have had some hotter and colder days, while Gander has Interpreting Standard Deviation Example Cont’d Think back to the exam type example… Here, the means are the same… But the standard deviation would be larger for the Multiple-Choice Exam, because the data are more dispersed (i.e., more people got scores that were higher or lower on the exam) Selecting Appropriate In general, select MCT and MD based on level of measurement Measures of But keep in mind how data are dispersed Central Tendency For example, mean and standard dev. are best for and interval data, unless outliers exist Dispersion In such cases, the median and interquartile range will give you more accurate description of data For this reason, researcher usually present more than one MCT and MD Quantitative Research Methods in Political Science Lecture 4: The Normal Curve and Z Scores Course Instructor: Michael E. Campbell Course Number: PSCI 2702 (A) Date: 09/26/2024 Quick Recap Lecture 1 Lecture 2 The role of statistics in the social Causality (or causal relationships) sciences The use of systematic processes Independent and dependent variables Difference between facts and values Conceptualization and operationalization The characteristics of variables Instruments and instrumentation Discrete vs. continuous Variables Systematic and random measurement Levels of measurement error (reliability and validity) Lecture 3 Descriptive and univariate statistics: Proportions, percentages, rates, ratios Measures of central tendency: Quick Mode, median, mean Recap Measures of dispersion: IQV, range, interquartile range, variance, standard Cont’d deviation Frequency distribution tables Graphs and charts: Pie, bar, histograms All of what we’ve looked at, in one way or another, serves as the foundation for the Normal Curve The Normal Curve Is a theoretical model used in statistics Can be used to precisely describe empirical distributions The Normal Curve: “a special kind of perfectly smooth frequency polygon that is unimodal (i.e., it has a single mode or peak) and symmetrical (unskewed) so that its mean, median, and mode are all exactly the same value” Healey, Donoghue, and Prus 2023, 126. The Normal Curve Cont’d The Normal Curve resembles the unskewed distribution from last lecture (Chapter 3) Is bell shaped (gaussian curve) and the tails extend into infinity Does not exist in nature – no distribution we observe in empirical reality will ever match this exactly Several variables (or data distributions) come close enough that we can assume data are The Normal Curve Cont’d Think of normal curve as a tool: 1. To make descriptive statements about empirical distributions 2. To be used in inferential statistics to generalize from sample to population Distances along the horizontal axis will always encompass same proportion of total area under the curve when measured in standard deviations The distances between any point and the mean cuts off the same proportion of the total area when measured in standard deviations The Normal Curve Example These data represent the IQ scores for children Hypothetical Distribution of IQ Scores and adults Among Children and Adults Children Adults In each case, the distributions are symmetrical (unskewed) = 100 = 100 Each has a sample (n) of 1000 = 20 = 10 The mean () is the same for each = 1000 = 1000 The standard deviations (s): 1. For children s = 20 2. For adults s = 10 The Normal Curve Example Cont’d Larger spread of data for children’s IQ scores because std. dev. is larger Two scales appear here: 1. IQ Units 2. Standard deviations from the mean Technically no difference between these scales Since the mean is 100: 1. One standard deviation above the mean for children is 120 2. One standard deviation below the mean for children is 80 Why? Because the standard deviation for The Normal Curve Example Cont’d The same logic applies for adult IQ scores Since the standard deviation is 10 for adults: 1. One standard deviation below the mean = 90 2. One standard deviation above the mean = 110 Area Under the Normal Curve Between Lies +/- 1 Std. Dev. 68.26% of area +/- 2 Std. Dev. 95.44% of area +/- 3 Std. Dev. 99.72% of area When measured in standard deviations, the distances along the horizontal axis on any normal curve will always encompass the same proportion of area under the curve Area Under the Normal Curve Cont’d 68.26 % Area Under the Normal Curve Cont’d Between Lies +/- 1.65 Std. Dev. 90% of area +/- 1.96 Std. Dev. 95% of area +/- 2.58 Std. Dev. 99% of area Social scientists tend to use whole number area values (90%, 95%, 99%) Useful for building confidence intervals (see textbook chapter 6) Can also be expressed in # of cases… For example, if you have 1000 cases 1. 683 cases +/- 1 std. dev. from mean Z scores are the way scores are expressed after they have been standardized to the theoretical normal curve Z Scores (Standard This means that if we want to find “the percentage of the total area (or number of cases) above, below, or between scores in an empirical distribution, we must first express the original Scores) scores in units of the standard deviation or convert them to Z scores, which are also called standard scores” (Healey, Donoghue, and Prus 2023, 129). Original units can be anything (weight, time, IQ scores, etc.) Z scores will always have the same values for mean and std. dev. Mean will always be 0 and standard deviation will always be 1 Computing Z Scores When we convert raw scores (e.g., in our case the IQ of an adult of child) into Z scores, we are changing the original units of measurement into Z scores This standardizes the normal curve to a distribution that has a mean of 0 and a standard deviation of 1 The formula for Z scores is: In this equation: is an individual score is the sample mean is the sample standard deviation Computing Z Scores Cont’d Let’s say you have 5 scores: 10, 20, 30, 40, 50 First, calculate the mean (see lecture 3 + textbook chapter 3): Computing Z Scores Cont’d Then calculate standard deviation (see lecture 3 + textbook chapter 3): Remember, to calculate this, you need to know the deviations (the distance of each score from the mean) Computing Z Scores Cont’d Take the sum of squared deviations and input them into the std. dev. formula… Therefore, the standard deviation is: Computing Z Scores Cont’d With this information, you can now compute the Z scores: Raw Scores: 10, 20, 30, 40, 50 s = 14.14 The five Z scores are: 1. -1.414 2. -0.707 3. 0.000 4. 0.707 5. 1.414 Positive and Negative Z Scores A positive Z score will fall to the right of the mean A negative Z score will fall to the left of the mean For instance, a positive Z score of +1.00 indicates that the score will fall exactly +1.00 standard deviation to the right of the mean Remember, when you convert the In our case, a positive Z score of 1.414 original scores to Z scores the nomal indicates that a score of 50 will fall 1.414 distribution takes on a mean of 0.00 and standard deviations to the right of the mean a standard deviation of 1.00 (see above) The Standard Normal Curve Table A Standard Normal Curve Table (or Z table) tells you the areas related to any Z score(s) Can be found in Appendix A of textbook (p.501 to 504 in 5th ed.) For our purposes right now, we can used abridged version… The Standard Normal Curve Table Cont’d There are three columns: 1. Column (a) shows Z score 2. Column (b) shows area between mean and Z 3. Column (c) shows area beyond the Z If we look at a Z score of 1.00 in this table, we see the area between Z and the mean is 0.3413 – why? When you calculate Z scores, you are standardizing a data point by expressing how many standard deviations it is away from the mean… 68.26% of all cases fall within 1.00 standard deviations from the mean… Therefore, the distance between a Z score of 1.00 and the mean is half of this – i.e., 0.3413 Answer 0.341 0.34 3 13 0.6826 Positive Z Score Example Let’s go back to the IQ of children Children’s IQ Scores = 100 Question: if a child has an IQ score of 130, how much of the = 20 area under the normal curve lies between the mean and this score? = 1000 Positive Z Score Example Cont’d First thing, convert raw score into Z score: Positive Z Score Example Cont’d We see that the area covered in column (b) of the standard normal curve table is 0.4332 Therefore, we