Preference Measurement Explicit PDF
Document Details
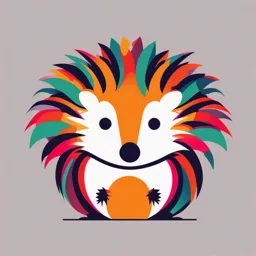
Uploaded by ConciseTrust
Tags
Summary
This document discusses various methods for measuring customer preferences, including explicit and implicit response questionnaires, behavioral or empirical data, and techniques like rating scales for importance measurement. It covers different approaches and provides examples.
Full Transcript
Key steps in Value Creation Forecasting primary demand evolution Exploratory research for customer decision factor identification Customer preference measurement Optimal product and price specification Preference Measurement Explicit 1 Understanding the decision making process of customers We want to...
Key steps in Value Creation Forecasting primary demand evolution Exploratory research for customer decision factor identification Customer preference measurement Optimal product and price specification Preference Measurement Explicit 1 Understanding the decision making process of customers We want to know: Which attributes are important? Which attribute-levels are more preferred? How does the customer put these together to make a choice? Consider the tablet computers category with these attributes and levels: Brand: Apple, Samsung, Lenovo Screen Size: 7 inches, 8 inches, 10 inches, 12 inches Price: $200, $350, $500 Andy Beth Chip Dave Within-attribute Preference Levels -------------------------------------------------------BrAp BrSm BrLn | Sc7 Sc8 Sc10 Sc12 | Pr200 Pr350 Pr500 7 2 1 | 1 2 6 7 | 7 2 1 7 1 5 | 7 5 2 1 | 7 4 1 1 6 7 | 5 6 7 1 | 7 3 1 1 7 5 | 1 7 6 2 | 7 5 1 Preference Measurement Explicit 2 | Across Attribute | Importances | ---------------| Brand ScrSz Price | 1 2 7 | 6 2 2 | 1 7 2 | 1 5 4 Why would we like to know the within-attribute preference levels and the across attribute importances? Market Share Forecasting: knowing these for a sample of consumers allows us to predict the likely share (and profits) for a candidate product. Optimal product-formulation/value-creation: We can then consider various candidate products, compute the share or profit for each and pick the best candidate Example: We can compute which of the following scenarios which would lead to a higher profit for Lenovo: Competing offerings: Apple 10inch $500 Apple 8inch $250 Samsung 7inch $200 Samsung 10inch $450 Samsung 12inch $550 Lenovo offerings: This? Lenovo 12inch $600 and Lenovo 10inch $400 Or This? Lenovo 12inch $550 and Lenovo 10inch $450 Preference Measurement Explicit 3 We want to know: Which attributes are important? Which attribute-levels are more preferred? How does the customer put these together to make a choice? For the first two, there are three distinct categories of approaches: Explicit Response Questionnaires Implicit Response Questionnaires Behavioral or Empirical Data Preference Measurement Explicit 4 Explicit Response Approach: Ask for importance valuations (or attribute-level preferences) directly, rather than infer them through preference ordering of products. Some leading alternatives: Rating scales (on 5-point or 7-point scale) Kano Method Rank Ordering Paired Comparisons Maximum Difference Scaling Constant Sum scale Pairwise Constant Sum Scale Pairwise Partial Profile Choice Probabilities Earlier methods are used to eliminate less important attributes. Later methods are used for refinement. Preference Measurement Explicit 5 Rating Scale for Importance Measurement Please indicate how important each of these aspects of a casual dining restaurant is to you. Use the scale across the top of this list to describe how important each aspect is to you. RANDOMISE ORDER. Not at all Somewhat Very important important Important Prompt greeting [ ] [ ] [ ] Overall cleanliness [ ] [ ] [ ] Comfortable environment [ ] [ ] [ ] Server attentiveness [ ] [ ] [ ] Server friendliness [ ] [ ] [ ] Pace of meal [ ] [ ] [ ] Taste of food [ ] [ ] [ ] Cuisine type [ ] [ ] [ ] Receive bill in timely manner [ ] [ ] [ ] Reasonable prices [ ] [ ] [ ] Extremely Critically important important [ ] [ ] [ ] [ ] [ ] [ ] [ ] [ ] [ ] [ ] [ ] [ ] [ ] [ ] [ ] [ ] [ ] [ ] [ ] [ ] Preference Measurement Explicit 6 Interpreting Scale Responses Consider the following patter of responses: Prompt greeting Overall cleanliness Comfortable environment... Not at all Somewhat Very important important Important [x] [ ] [ ] [ ] [ ] [ ] [ ] [x] [ ] Extremely Critically important important [ ] [ ] [x] [ ] [ ] [ ] Do you interpret this as meaning that to this customer... “Overall cleanliness” is three times as important as “Comfortable environment”? (Ratio) The increase in importance from “Comfortable environment” to “Overall cleanliness” is twice as much as the increase in importance from “Prompt greeting” to “Comfortable environment”? (Interval) That “Comfortable environment” is more important than “Prompt greeting” and that “Overall cleanliness” is more important than “Comfortable environment”? (Ordinal) Preference Measurement Explicit 7 Rating Scale for measuring preference strengths within an attribute level Indicate your overall preference for each of the cuisine types listed in the left column by checking one of the following categories: Indian Chinese Mexican strongly dislike [ ] [ ] [ ] [ [ [ ] ] ] like nor dislike [ ] [ ] [ ] [ [ [ ] ] ] Preference Measurement Explicit 8 strongly like [ ] [ ] [ ] Interpreting the scale responses Suppose we observe the following set of response Indian Chinese Mexican strongly dislike [X ] [ ] [ ] [ ] [X ] [ ] like nor dislike [ ] [ ] [ ] [ ] [ ] [X ] strongly like [ ] [ ] [ ] Do you interpret this as meaning that this customer believes... Chinese is twice as good as Indian? (Ratio) Mexican is three times better than Indian as Chinese is than Indian? (Interval) Is Chinese better than Indian? Mexican than Chinese ? (Ordinal) Preference Measurement Explicit 9 Why do need to worry about scale type? A scale’s interpretations/types dictates what kinds of analytics we can do with the data that we collect? How do we summarize data that we believe come from... Ratio-interpreted scales? Interval-interpreted scales? Ordinal-interpreted scales? Preference Measurement Explicit 10 Kano Method Data Collection Two questions for each need: one positively framed and the other negatively framed – “The rear tire is easy to change. How do you feel?” – “The rear tire is not easy to change. How do you feel?” – Five response options ∗ ∗ ∗ ∗ ∗ I highly like it that way It somewhat like it that way I am neutral I somewhat dislike it that way I highly dislike it that way Preference Measurement Explicit 11 Result of Negative Question Like Smw Like Neutral Result of Positive Question Like Smw Like Neutral Smw Dislike Dislike Preference Measurement Explicit 12 Smw Dislike Dislike Rank Ordering Please indicate how important each of these aspects of a casual dining restaurant is to you. Rank the aspects by placing a 1 beside the aspect you think is most important for you, a 2 beside the aspect you think is second-most important, and so on. RANDOMISE ORDER. Prompt greeting Overall cleanliness Comfortable environment Server attentiveness Server friendliness Pace of meal Taste of food Temperature of food Receive bill in timely manner Reasonable prices Rank ___ ___ ___ ___ ___ ___ ___ ___ ___ ___ Preference Measurement Explicit 13 Paired Comparisons We would like to understand how important various aspects of a casual dining restaurant are to to you. In each of the following pairs, which aspect do you think is more important? Please check one aspect within each pair. Comfortable environment Pace of meal Receive bill in timely manner... ___ ___ ___ OR OR OR Server attentiveness Receive bill in timely manner Overall cleanliness ___ ___ ___ How exactly would we infer the relative importances after collecting such information from customers? Preference Measurement Explicit 14 Maximum Difference Scaling Please indicate how important each of these aspects of a casual dining restaurant is to you. In each of the following sets of items, please pick the ONE item you consider MOST important and the ONE item you consider least important. Set1 Temperature of food Server friendliness Server attentiveness Prompt greeting Set3 Overall cleanliness Server attentiveness Temperature of food Prompt greeting... ___ ___ ___ ___ Set2 Server attentiveness Taste of food Server friendliness Overall cleanliness ___ ___ ___ ___ ___ ___ ___ ___ Set4 Prompt greeting Comfortable environment Temperature of food Overall cleanliness ___ ___ ___ ___ Preference Measurement Explicit 15 Evaluating Importance in Maximum Difference scaling Simple Analysis: Raw Importance of X = (#Best)−(#Worst) Total Number of Sets in which X appears +1 Standardized Importance = Raw 2 Preference Measurement Explicit 16 SET ----------4 7 8 9 3 6 8 10 2 5 9 10 1 8 9 10 4 5 6 10 3 5 7 9 2 6 7 8 1 5 6 7 2 3 7 10 2 4 6 9 3 4 5 8 1 2 3 4 1 4 7 10 1 3 6 9 1 2 5 8 Best 4 6 9 8 4 7 6 6 7 4 4 4 4 6 8 Worst 9 10 5 10 5 5 2 5 10 2 5 1 10 1 5 a #b #w 1 0 2 2 0 2 3 0 0 4 6 0 5 0 6 6 4 0 7 2 0 8 2 0 9 1 1 10 0 4 Analysis d #s raw -2 6 -0.33 -2 6 -0.33 0 6 0.00 6 6 1.00 -6 6 -1.00 4 6 0.67 2 6 0.33 2 6 0.33 0 6 0.00 -4 6 -0.67 std 0.33 0.33 0.50 1.00 0.00 0.83 0.67 0.67 0.50 0.17 Preference Measurement Explicit 17 Design Issues in Maximum Difference Scaling How many items per set? Suppose I is the number of items per set. As I increases, the number of pairs of orderings increases but cognitive load also increases. Accuracy as a function of I has an inverted U shape. Optimal I is 4 or 5. How many sets? Minimum of the two numbers below: – Statistical Consideration: Suppose C is the desired number of times of coverage, A is the number of attributes, I is the number of items per set. The minimal number of sets is C×A I – Psychological Consideration: The number of sets that can be fit into the target number of minutes. Once we have number of items per set and the number of sets, how do we decided what items go into the various sets? Using the methodology of “Balanced Incomplete Block Designs”. Preference Measurement Explicit 18 More Complex Analysis for Unbalanced Sets: Suppose out of A, B, C, D, customer picks D as best and B as worst. Let a, b, c, d be the true underlying importances of A, B, C, D. We model the probability of the customer’s picks as a function of the true importances, and use these probabilities in likelihood-based estimation. Preference Measurement Explicit 19 One way to model the probability. Use the logit probability for picking any particular pair out of all possible pairs, assuming the utility for each pair as the difference in importances between the two attributes in the pair. Out of A, B, C, D, customer picks D as best and B as worst. Numerator: exp (d − b) Denominator: exp(a − b) + exp(a − c) + exp(a − d)+ exp(b − a) + exp(b − c) + exp(b − d)+ exp(c − a) + exp(c − b) + exp(c − d)+ exp(d − a) + exp(d − b) + exp(d − c) Preference Measurement Explicit 20 Another way to model the probability: Out of A, B, C, D, customer picks D as best and B as worst. Consider this is as implying that the complete importance ordering as instantiated in the mind of the customer is either D, A, C, B or D, C, A, B. If the complete importance ordering instantiated is D, A, C, B, its probability by the exploded logit model is exp(d) exp(a) exp(c) exp(d) + exp(a) + exp(c) + exp(b) exp(a) + exp(c) + exp(b) exp(c) + exp(b) Similarly, if the complete importance ordering instantiated is D, C, A, B, its probability is: exp(c) exp(a) exp(d) exp(d) + exp(c) + exp(a) + exp(b) exp(c) + exp(a) + exp(b) exp(a) + exp(b) The overall probability of picking D as best and B as worst is the sum of the two expressions above. Preference Measurement Explicit 21 Constant Sum Scale Please indicate how important each of these aspects of a casual dining restaurant is to you. To do this, please distribute 100 points across these aspects according to how important each is to you. You can give as many or as few points as you like to each aspect, but the total number of points you assign must be 100. RANDOMISE ORDER, MAKE SURE TOTAL SUMS TO 100. Prompt greeting Overall cleanliness Comfortable environment Server attentiveness Server friendliness Pace of meal Taste of food Temperature of food Receive bill in timely manner Reasonable prices ___ ___ ___ ___ ___ ___ ___ ___ ___ ___ Preference Measurement Explicit 22 Pairwise Constant Sum Scale We would like to understand the relative importances of aspects of restaurants that you consider when deciding your overall preference for a restaurant. Listed below are several pairs of aspects. Within each pair, distribute 100 points across the two aspects according to how important each aspect is is to you. You can give as many or as few points as you like to each aspect, but the total number of points you assign must be 100. RANDOMISE ORDER, MAKE SURE TOTAL SUMS TO 100. Comfortable environment Pace of meal Receive bill in timely manner... ___ Server attentiveness ___ Receive bill in timely manner ___ Overall cleanliness ___ ___ ___ TOTAL 100 100 100 Preference Measurement Explicit 23 Pairwise Partial Profile Choice Probabilities This is a relatively low cognitive load and accurate way to get ratio-scaled measures of attribute importances. We present questions of the following form to consumers: What is the probability of choosing alternative A over B? Alternative A: Battery Life 6 hrs, Price USD 100 Alternative B: Battery Life 8 hrs, Price USD 150 Suppose the consumer responds “0.6”. Then we take this to imply that Importance(Price) 0.6 = 1−0.6 , or Importance(Battery Life) 0.6 = 0.4054 logImportance(Price) − logImportance(Battery Life) = log 1−0.6 In general, we present questions of the following form: What is the probability of choosing alternative A over B? Alternative A: Best level of Attribute i, Worst level of Attribute j Alternative B: Best level of Attribute j, Worst level of Attribute i Suppose the consumer responds with pij. Then we take this to imply that pij log Importance(Attribute i) − logImportance(Attribute j) = log 1−p , or ij Li − Lj = log pij 1−pij , denoting Log Importance of attribute a as La. Preference Measurement Explicit 24 Data presentation: While not necessary, the set of pairs should preferably be obtained via R by running find.BIB(A, C*A/2, 2) where A is the number of C is the desired number of times of coverage, and A is the number of attributes. Data collection: Ask for the choice probability for each pair as described on the previous slide. Example: Pair ID 1 2 3 4 5 i j 1 3 1 2 4 2 4 5 3 5 probability response 40 25 45 70 65 log odds ratio -0.405 -1.099 -0.201 0.847 0.619 Log Importance Difference = = = = = L1 L3 L1 L2 L4 - L2 L4 L5 L3 L5 Preference Measurement Explicit 25 (1) Create a regression dataset with one row per pair of attributes presented. The y value for a row is the corresponding log odds ratio. The X values for each row are all zero except that there is a 1 for attribute i of the pair and a −1 for attribute j of the pair. (2) Take the importance of the first attribute to be 1. This makes L1 = 0, which allows us to ignore the first column of X matrix. Run the regression without an intercept to obtain the log importances of the rest of the attributes. Pair ID 1 2 3 4 5 X1 X2 X3 X4 X5 1 0 1 0 0 -1 0 0 1 0 0 1 0 -1 0 0 -1 0 0 1 0 0 -1 0 -1 y -0.405 -1.099 -0.201 0.847 0.619 Preference Measurement Explicit 26 Running the regression gives the coefficients to be: 0.4376, -0.3768, 0.7548, 0.1684 attribute 1 2 3 4 5 log importance 0 0.4376 -0.3768 0.7548 0.1684 exp(log( importance)) 1.000 1.549 0.686 2.127 1.183 -----6.545=sum normalized importance (sum to 100) 15.3 23.7 10.5 32.5 18.1 Preference Measurement Explicit 27 Recommended strategy for measuring importances when you have a large number of attributes: Start with a full set of attributes and do a first pass at reducing the set by using rating scales Reduce the attribute set further by using Maxdiff scaling. If needed, reduce further and get more refined scale properties by using the Pairwise Partial Profile Choice approach. If needed, refine further via Conjoint Analysis Preference Measurement Explicit 28 Recommended strategy for measuring the preference strengths for various attribute levels within an attribute: Focus on the more important attributes. The differences in preference strengths for various attribute-levels within the less important attributes do not matter much. Within each attribute that is being considered further: – Ask the respondent to rate the different levels of each attribute – Rescale the ratings so that the most preferred attribute-level has a rating of some fixed number like 7, and the least preferred attribute-level has a rating of fixed number like 1. This ensures that all the all the attributes have an equal spread of preference levels. If there are many attribute-levels for a certain attribute (like the Brand attribute), then use the more elaborate methods like the MaxDiff approach. Preference Measurement Explicit 29 A key deficiency of stated importance methods as commonly executed: The importance of an attribute depends on the range or set of values the attribute can take. For better results, mention the range or set of values explicitly and phrase as “how important it is to get your most preferred level” and optionally add as qualifier “relative to getting the least preferred level” “ relative to average preferred level” Preference Measurement Explicit 30