Quantitative Research Designs and Sampling Procedure PDF
Document Details
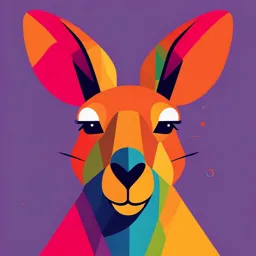
Uploaded by SpontaneousGrossular4509
Tags
Summary
This document provides a comprehensive overview of quantitative research designs and sampling procedures. It explains various research designs, their applications, and considerations. The document details different types of designs, including experimental, quasi-experimental, and non-experimental approaches, which are crucial for conducting reliable and valid research.
Full Transcript
Quantitative Research Designs and Sampling Procedure A good research requires a good design. The use of an appropriate design minimizes the occurrence of errors in the conduct of the study and in the conclusions drawn. A wrong choice of design puts at risk the validity and the reli...
Quantitative Research Designs and Sampling Procedure A good research requires a good design. The use of an appropriate design minimizes the occurrence of errors in the conduct of the study and in the conclusions drawn. A wrong choice of design puts at risk the validity and the reliability of the study. When this happens, it is quite difficult to find real answers to a research question, because there could be some hypotheses that can explain the occurrence of a problem. The selection of an appropriate study design can minimize possible errors by maximizing reliability and validity of data. Lesson 1: Research Design Research Design refers to an overall strategy that a researcher uses to logically and coherently integrate the various components of a study. A research design is the “blue print” of the study. It guides the collection, measurement and analysis of data. It is a plan or course of action which the research follows in order to answer the research question/s or solve the research problem. The design becomes the basis for determining what data will be collected, and how they will be analyzed and interpreted. Commonly Used Research Designs (Campbell and Stanley, 1968, Parel et al., 1985, Fisher, et al., 1994) The choice of a research design depends on the objectives of the study. These designs can be classified into: non- or pre-experimental designs, and experimental and quasi-experimental designs. Non/Pre-Experimental Designs True Experimental Design Posttest Only Design or After-Only Survey Pretest-Posttest Control group Design Pretest-Posttest Design or Before-After Posttest Only Control Group Design Survey Static Group Comparison Quasi-Experimental Design Non-Equivalent Control Group Design Time Series Design A. Non-Experimental or Pre-Experimental Designs Non-experimental designs are appropriate for collecting descriptive information about a population or subjects of a study. They are appropriate for descriptive studies like profile studies, exploratory studies, and for doing small case studies. They are also ideal for diagnostic studies or situation analysis. They are not recommended for evaluation studies intended to determine the effect or impact of a certain intervention or treatment. a. Posttest Only Design or After-Only Survey or One shot Survey - Data are collected only once - This design is used when the study objective is to describe a situation/condition of a study population as it exists, or determine/describe the characteristics of a population/respondents. There is no baseline data - This design is cheap and easy to conduct, but results cannot be conclusive in terms of causality or effect of an intervention. It is however, recommended for evaluation studies that intend to measure the effect of a program intervention, like training b. Pretest-Posttest Design - Used when the study wants to know the chance in characteristics of the study population in a given area - A survey, observation or testing is conducted before an intervention is introduced. After a period of time the survey, observation or testing is repeated and the results of the pretest and the posttest are compared to determine change/s. - For example, if a researcher wants to know if an information campaign against drug/substance abuse in a certain city has reduced drug use in the area after the campaign, a survey before and after the campaign can be conducted. No “control” area (area where no campaign is conducted), however, was surveyed. With the absence of a control area, this design cannot be considered as an experimental design. Any reduction in drug use over time, cannot be solely/conclusively attributed to the intervention campaign c. Static Group Comparison - In this design, there are two groups involved, the experimental and the control group. - The experimental group (O₁) received or is exposed to the intervention or treatment (X). This is followed by a measurement, the result of which is compared to the result of the measurement/observation from a control group (O₂) that did not received intervention. - The random process, however, was not used in the assignment of subjects to the experimental and control groups. - The problem with this design is the validity threat of selection and mortality is possible that the two groups differ greatly on the basis of the main variable of the study (selection) or some subjects in the experimental group may die out and be lost to follow-up or second observation/testing (mortality) B. True Experimental Designs In true experimental designs, subjects are randomly assigned to the experimental group and the control group to achieve pre-intervention /treatment equality of the two groups. With well-defined and properly selected experimental and control groups, validity threats are avoided. Before researcher decides on an alternative design, the feasibility of using true experimental designs must first be considered. The two most frequently used true experimental designs are the pretest-posttest control group design and the posttest control group design. 1. Pretest-Posttest Control Group Design - In the pretest-posttest control group design, the experimental group is exposed to or covered by an intervention or treatment, for example, a training or a new strategy, while the control group is left alone or given another kind of treatment. - Before the intervention/treatment is introduced to the experimental group, a survey/observation/testing is conducted for both experimental group and control group using the same device/instrument. - The pre-intervention survey/observation/test serves as pretest and the data collected serve as baseline data. After the introduction of the intervention in the experimental group or area, an evaluation survey/observation/testing is conducted in both experimental group/area and the control group/area, using the same instrument used in both during the pretest. The results serve as the posttest/endline data. - The baseline (pretest) and endline (posttest) data are compared. If the change in the “impact/effect indicator/s” or dependent variable/s is significantly better in the experimental area/group than the change in the control area/group, then the intervention is considered effective. If not, then the intervention is said to have had no effect 2. Posttest Only Control Group Design - The Posttest Only Control Group design is also used to determine the effects of an intervention or treatment introduced to a group of subjects (people/objects). As in the pretest-posttest control group design, at least two groups or areas with virtually the same characteristics are chosen and randomly assigned to the control and experimental group. - The experimental group or area is exposed to or covered by an intervention/treatment, while the control group is left alone. No pretest/preintervention study is conducted. The experimental and the control groups are assumed to have similar characteristics at the start of the study. After the introduction of an intervention in the experimental group or area, an evaluation survey/observation/testing is conducted in both experimental and the control groups or areas using the same “fair” instrument. - The data gathered from the experimental and control groups and compared. If the experimental group or area shows significantly better results than the control area/group with respect to the “impact/effect indicator/s”, the dependent variable/s, the intervention or treatment is considered effective, if not, then, the intervention is not effective C. Quasi-Experimental Designs In field studies, it is very difficult to meet the random assignment criterion of a true experimental design. In this situation, a quasi-experimental design is recommended. Quasi-experimental designs are nearly the same as true experimental designs, except that the former does not have restrictions as random assignment 1. Non-Equivalent Control Group Design - In field research, it is possible to compare an experimental group with a similar but not necessarily equivalent group. The two groups need only to have “collective similarity,” which means that they should have more or less the same characteristics in terms of aspects which are relevant to the study. For example, if one wants to determine the impact of an educational campaign on school attendance of children, the experimental and the control areas should have more or less the same socioeconomic characteristics, because these factors may also affect school attendance. - As in the pretest-posttest control group design, the intervention or treatment is introduced to the experimental group, but withheld from the control group. Before the introduction of the intervention, a survey/observation/testing is conducted in both the experimental group (O₁)and the control group (0₂). - After the introduction of the intervention to the experimental group, another observation/testing (posttest) is conducted to both groups (O₃ and O₄). The pretest can be used to determine whether the two groups have truly “collective similarity” at the start of the experiment. The results of the two posttests will also be compared. - The intervention is effective if the change in the impact/effect indicators in the experimental group is significantly higher/better than the change in the impact/effect indicators in the control group. If not, then the intervention/treatment cannot be considered effective. This design is a good one for evaluating training programs, and other community interventions. 2. Time Series Design - The time series design is similar to the non-experimental posttest design except that, it has repeated observations/measures before and after the intervention. Before the introduction of the intervention/treatment, a measurement/observation with respect to the impact/effect indicators will be conducted several times at a regular interval, say, every 30 days and then after the intervention in the same time interval as the first. - The same measuring instrument should be used at all times. The result or pattern of the observations or testing in the first set of measurements will be compared with that in the series of measurement after the intervention. - If the post-intervention series, then the intervention can be considered effective. However, if the pre- intervention and post intervention results or pattern of the same, or the post intervention result is not significantly better than the pre-intervention, then the intervention cannot be considered effective Selecting a Study Design In selecting a study design, it is important to consider ethical issues and the balancing of technical issues against practical and administrative issues. 1. Ethical Issues- the researcher must make sure that the use of a particular design does not endanger the respondent’s life, will not result in the violation of people’s rights and dignity or in a denial of services that otherwise would be available. The research should not involve unethical procedures. It is important therefore, that informed consent is secured from the respondents or subjects before they are involved in a study. 2. Practical and administrative issues- every research requires sufficient funds, competent personnel and adequate facilities, but these may not always be available. Most often funds are limited, time is inadequate, and qualified personnel are few. These issues often affect the choice of a good design. Limited resources often result in the adoption of a less ideal design. 3. Technical issues - the use of appropriate or ideal design helps minimize possible errors. It is important therefore, that technical aspects be given serious consideration. Whenever possible, the following should be done: a) Experimental and control groups should be randomly assigned b) When random assignment is not possible, try to find a comparison group that is nearly equivalent to the experimental group. c) When neither randomly assigned control group nor a similar comparison group is available, try using time series design that can provide information on trends before and after a program intervention. d) If time series cannot be used, try to obtain baseline information that can be compared against post program information (pretest-posttest) e) If baseline (pretest) information is unavailable, bear in mind that the type of analysis that you can use. f) Always keep in mind the issue of validity. Are your measurements true? Do they measure what they intend to measure? Guidelines for a Good Research Design 1. A “good” research design is an ethical design. 2. A “good” research design is capable of obtaining the most reliable and valid data given all possible constraints. 3. A “good” research design is capable of collecting the needed data measuring whatever it is that happens in the field. 4. A “good” research design helps an investigator avoid making erroneous conclusions. Writing the Research Design Part of your Research Sample: Research Design This study attempted to determine the Family Relationship Status and Academic Performance of Senior High School GAS students enrolled in the Senior High School Program at Passi National High School for school year 2018-2019 This study used the Posttest Only Design or After-Only Survey or One-shot Survey where data are collected only once. This design is used when the study objective is to describe a situation/condition of a study population as it exists, or determine/describe the characteristics of a population/respondents. Lesson 2: Sampling Procedures and the Sample The population or universe in statistics includes all members of a defined group that researchers are studying or collecting information on for data driven decisions. Topic population is a group or set (may be things, plants, animals or people) from which generalization can be made while respondent population are the individuals whose responses will serve as basis in making generalization about the topic population. Target population, on the other hand, is the group or set of items or individuals from which representative information is desired. Sample – a part or proportion of the population, a subset of a population that is used to represent the entire group as a whole. Sampling – the process of getting information from a proper subset of population. (Faltado et al, 2016). The fundamental purpose of all sampling plans is to describe the population characteristics through the values obtained from a sample as accurately as possible. It is therefore evident that if one were to draw conclusions based on a small sample then the sample must imitate the behavior or characteristics of the original population as closely as possible. Sampling Errors – differences between what is present in a population and what is present in a sample. The larger the sample size, the smaller the level of error. This is simply because as the sample becomes closer to reaching the size of the total population, the more likely it is to accurately capture all of the characteristics of the population. Reasons for Sampling Sampling is a research technique which is necessary and advantageous, particularly in terms of labor and cost. In carrying out a study, the use of population or complete enumeration (census) of target respondents is very difficult and costly. The following are reasons why sampling is necessary: 1. The research project can be done easier and faster. This is because only a part of the entire population is studied; since much fewer respondents are involved, data collection can be done more smoothly and completed more quickly. 2. More comprehensive information can be obtained from a sample. Being much fewer in number than the entire population, a sample can be thoroughly studied; the study is better managed, hence, more relevant information could be gathered. 3. Research results could be more valid and reliable. Since only a sample is being dealt with, errors incurred in the collection and processing of data could be minimized or completely eliminated. 4. It is cheaper, hence, save money. Principles of Sampling Among the many research activities the researcher does, the selection of the sampling design may be considered as the most critical in the sampling process. To do this, the underlying principles must be fully understood and followed by the researcher. These principles include: representativeness, randomness and adequacy of the sample. 1. Representativeness of Sample - it means that the sample or sampling units drawn exhibit the general characteristics of interest of the target population. Taking more respondents from “extremes” (the most and the least) and as a part of the sample, for instance, would likely yield misleading information and lead to wrong conclusions. The term extreme in this case refers to sampling elements which are atypical in the population. This could potentially ruin the accuracy of our data. 2. Randomness of Sample - a characteristic of sampling which allows all units of the population an equal chance to become part of the sample. Hand-picked samples preferred by the researcher himself are not random samples. Basically, this violates a sampling principle. If not employed properly, this can lead to wrong conclusions. Randomization is the most basic process researchers use to attain a more or less homogenous sample which is a very important attribute or the use a faulty randomization procedure in the selection of samples can samples can similarly result to erroneous data. 3. Adequacy of Sample - The size of a sample is not just any number the researcher wishes to use in his study. It must be sufficient enough to be able to represent the population from where it is taken. Inadequate sample may affect representativeness. This is the very reason why researchers are obliged to use a certain formula to determine the correct sample size. This is the best way to do to make sure that the sample size is enough to represent a certain population. Sample Size There are various formulas for calculating the required sample size based upon whether the data collected is to be of a categorical or quantitative nature. Before you can calculate a sample size, you need to determine a few things about the target population and the sample you need: 1. Population Size – how many total people fit your demographic? For instance, if you want to know about mothers living in the Philippines, your population sized would be the total number of mothers living in the Philippines. Don’t worry if you are unsure about this number. It is common for the population to be unknown or approximated. 2. Margin of Error (Confidence Interval) – no sample will be perfect, so you need to decide how much error to allow. It is the plus-or-minus figure usually reported in a newspaper or television opinion poll results. For example, if you use a confidence interval of 4 and 47% of your sample picks an answer you can be “sure” that if you had asked the question of the entire relevant population between 43% (47-4) and 51% (47+4) would have picked that answer. 3. Confidence Level – tells you how sure you can be. It is expressed as a percentage and represents how often the true percentage of the population who would pick an answer lies within the confidence interval. The 95% confidence level means you can be 95% certain; the 99% confidence level means you can be 99% certain. Most researchers use the 95% confidence level. 4. Standards of Deviation – how much variance do you expect in your responses? Since the actual survey has not been administered yet, the safe decision is to use.5 which is the most forgiving number and ensures that your sample will be large enough. 5. If you take a population sample, you must use a formula to figure out what sample size you need to take. Knowing the population can help you determine the sample size. You can use Slovin’s formula to figure out what sample size you need to take. The formula is: n = N/ (1 + Ne2) where: n = number of samples N = total population E – Error tolerance Sample Problem: Use Slovin’s formula to find out what sample of a population of 1,000 people you need to take for a survey on their soft drinks preferences. Step 1: Figure out what you want your confidence level to be. For example, you might want a confidence level of 95% (which will give you a margin of error of 0.05), or you might need better accuracy at the 98% confidence level (which produces a margin of error of 0.02). Step 2: Plug your data into the formula. In this example, you use a 95 percent confidence level with a population size of 1,000. n = N/(1+N e2) = 1,000/(1+1000*0.05) n = 1,000/1+1,000 x 0.0025 n = 1,000/1+2.5 n = 1,000/3.5 n=285.714 or 286 Step 3: Round your answer to a whole number (because you can’t sample a fraction of a person or thing) 285.714286 = 286 Four Ways of Determining the Sample Size of Your Study (Barrot, 2017) Heuristics – a term normally used in qualitative studies as a research approach that utilizes introspection. In quantitative research, it refers to the rule of thumb for the sample size used in a study. Below are the suggested sample sizes in the different quantitative research (Lunenberg and Irby, 2008). The survey indicated in the table refers to a research design and not to a research instrument. Research Design Number of Participants Survey 800 Correlational 100 to 200 Ex Post Facto 30+ Experimental 30 or more Literature Review – Read similar studies and check the sample size that they used. These studies can serve as a reference in proving the validity of the sample size that you plan to use. Formulas - you may use Slovin’s formula or the formula of Lynch, et.al. (1974) Table - another way of determining the sample size is by the use of a prepared table with a pre- determined/computed values based on population. In the following table, the approximate sample size for a particular universe is already calculated based on certain proportions and confidence level. (Source: Appendix to Sampling and Statistics Handbook for Survey in Education, Research Division of the National Education Association. Washington D.C. 1965) Population Size Sample Size for Permissible Error (Proportion).05.04.03.02.01 100 79 86 91 96 99 200 132 150 168 185 196 300 168 200 234 267 291 400 196 240 291 343 284 500 217 273 340 414 475 Sampling Techniques (Faltado et.al, 2016) The basic distinction in modern sampling theory is between probability and the non-probability sampling. Probability Sampling Probability Sampling refers to a sampling technique in which samples are obtained using some objective chance mechanism, thus involving randomization. They require the use of a sampling frame (a list/map of all the sampling units in the population). The probabilities of selection are known. They allow drawing of valid generalizations about the universe/population whose sampling error can be ascertained. The definition of a probability sample does not require equal probabilities of inclusion in the sample for all elements in the population. Probability sampling is the only approach that makes possible representative sampling plans. It makes possible for the investigators to estimate the extent to which the findings based on their sample are likely to differ in what they would have found by studying the population. The use of probability sampling enables the investigator to specify the size of the sample that they will need if they want to have the given degree of certainty that their sample findings do not differ by more than a specified amount from those that a study of a whole population would yield. Commonly used Probability Sampling Techniques 1. Simple Random Sampling the basic probability sampling design, which the sample is selected by a process that does not only give each element in the population a chance of being included in the sample but also makes the selection of every possible combination of the desired number of cases equally likely. The sample is selected in one of two ways: by means of a table of random numbers or by using the lottery technique or the fish bowl technique. This requires two stops a. Obtain a complete list of the population b. Randomly select individuals from that list for the sample 2. Systematic Random Sampling affected by drawing units at regular intervals from a list. The starting point or the first units to be taken is a random choice. It differs from one simple random sampling where each member of the population is not chosen independently. Once the first member has been selected, all the other members of the random sample are automatically determined. The population list in the systematic sampling must be in random order. The participants are selected from a list based on their order in the population or on a predetermined interval. This interval is obtained by dividing the population size by the sample size for the study. For example, you need a sample size of four participants from a population of 12 individuals. Using systematic sampling, you may decide to select every third person in the list. Take note that you are not required to begin with selecting person # 3. You may begin with any of the first three individuals (person 1, 2, 0r 3). Succeeding samples, however, should be consistently selected using the specified nth value (i.e. every third). 3. Stratified Random Sampling similar to simple random sampling in that the members of the population can all be selected by chance. However, in the stratified sampling, the population is divided into subgroups (strata). The samples are then selected from these subgroups, and not from the population as a whole. The stratified sampling technique is best used when the characteristics of the subgroups may influence the variables being measured. This means that the data obtained can be also group into different classes, creating more nuances in the analysis. However, it must be made sure that all subgroups are represented in the sample adequately. As shown in the illustration on page 11, the 12 individuals are divided first into three subgroups. Through one of the methods discussed earlier, it has also been determined beforehand that there will be a total sample size of four participants. The number of members per subgroup is then divided by the total population size as follows: Green subgroup: 3/12 = 0.25 (25%) Blue subgroup: 3/12 = 0.25 (25%) Red subgroup: 6/12 = 0.5.(50%) The result is the percentage of the members per subgroup who will be included in the sample. This percentage is then multiplied by the total sample size of four as follows: Green subgroup: 25% x 4 = 1 participant Blue subgroup: 25% x 4 = 1 participant Red subgroup: 50% x 4 = 2 participants This leads to the computation of the final number of participants per subgroup – one each from the blue and green subgroups and two from the red subgroup. This makes up the final sample for the study. 4. Cluster Sampling Similar to stratified sampling, cluster sampling involves the grouping of the population into subgroups or clusters. However, this clusters are not created based on specific characteristics or traits; thus they are heterogenous. In practice, the most common clusters are in terms of geographic units (region, province, town, barangay) or occupational groupings (teachers, farmers, vendors, fisherman). Specific participants are not selected from each subgroup/cluster. Instead two whole subgroups or clusters are selected to meet the planned sample size of four participants. One problem with using this type of random sampling is the possible inadequate representation of each type of characteristic among the population. To overcome inadequacy, a large number of samples should be used from different subgroups or clusters. It is a technique in which the unit of sampling is not the individual but the naturally occurring group of individuals. The technique is used when it is more convenient to select individuals from a defined population. It considers a universe divided into N mutually exclusive sub-groups called clusters. A random sample of n clusters is selected and their elements are completely enumerated. It is administratively convenient to implement and its main advantage is saving time and money. 5. Multi-Stage Sampling In this method, the sample is drawn in two or more stages by using several techniques we have already discussed. This is accomplished by dividing the whole study population into sub-populations which decreases in the number of elements as the phases of sampling proceeds. For instance, the study covers the whole region of five provinces involving all sustenance fishermen as the target population. Since the study is so large, the first stage of sampling may be drawing by random the provinces included in the study; within the province, the sample municipalities would be drawn as the second stage; then the barangays in the sample municipalities as the third stage; and finally, the respondent fishermen from each sample barangays as the fourth stage. This is an example of a four-stage multi- stage sampling technique. At each stage, a random, systematic or stratified random sampling technique may be employed. Non-Probability Sampling This is a technique when there is no way of estimating the probability that each element has of being included in the sample and no assurance that every element has a chance of being included. 1. Accidental Sample is one which the investigator simply reaches out and takes the case that are at hand, continuing the process until the sample reaches a designated size. This is one of the most common techniques of sampling. This is also known as “the man on the street” interviews conducted frequently by television news program to get quick reading of public opinion. In clinical practice, clients who are available to medical people are usually their sample. In research, samples are usually those who simply volunteer for the study. The problem here is the lack of evidence that they are the representatives of the population you are interested in generalizing. 2. Purposive Sampling or Judgment Sampling used when practical consideration prevents the use of probability sampling. Since sampling errors and biases cannot be computed for non-probability samples, purposive or judgmental sampling should be limited to situation like when the probable errors are not serious and when probability sampling is practically impossible. In purposive sampling, the researcher just picks out “typical cases from the entire population in which he may be interested. The researcher relies again so heavily on his judgment as to who must compose the sample. The use of key informants may be resorted also in this case. Data from judgmental samples at best suggest or indicate conclusions but in general they cannot be used as the basis of statistical testing procedures. In purposive sampling, you sample with a purpose in mind. Usually, you seek with one or more specific predefined groups. 3. Quota Sampling the non-randomized samples are just picked out in mass from a population which is merely based on the best judgment of the researcher himself; the group exhibits characteristics which area “replica” of the population under study. Here, sampling units are taken as a whole rather than individually, thus the term quota. In quota sampling, you select people non-randomly according to some fixed quota. There are two types of quota sampling: proportional and non-proportional. a. Proportional Quota Sampling - you want to represent the major characteristics of the population by sampling a proportional amount of each. For example, if you know the population has 70% women and 30% men, and that you want a total sample size of 100, you will continue sampling until you get those percentages and then you will stop. So, if you’ve already got the 70 women for you sample, but not the 30% men, you will continue to sample men but even if legitimate women respondents come along, you will not sample them because you have already met your “quota”. The problem here is that you have to decide the specific characteristics on which you will base the quota. Will it be by gender, age, education, race, religion, etc. b. Non-proportional quota sampling is a bit less restrictive. In this technique, you specify the minimum number of sampled units you want in each category. You will not be concerned with having numbers that match the proportions in the population. Instead, you simply want to have enough to assure that you will be able to talk about even the small groups in the population. This technique is the non-probabilistic analogue of stratified random sampling It is usually used to assure that smaller groups are adequately represented in your sample.