Module D: Service Capacity PDF
Document Details
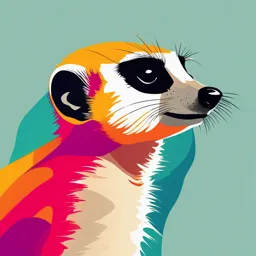
Uploaded by DiplomaticJadeite1956
Bentley University
Prof. Jalili
Tags
Summary
This document appears to be lecture notes or study guide for a course on service operations management, covering topics such as service capacity, demand and supply, variability, queuing systems, and the newsvendor problem. It is structured as a table of contents and includes diagrams and examples for different types of service configurations.
Full Transcript
Prof. Jalili Service Operations Management MG316 Module D: Service Capacity Table of Contents Module D: Service Capacity..........................
Prof. Jalili Service Operations Management MG316 Module D: Service Capacity Table of Contents Module D: Service Capacity........................................................................................................... 1 Two Sides of a Business: Demand and Supply.............................................................................. 3 Demand...................................................................................................................................................... 3 Service Capacity........................................................................................................................................ 3 Understanding Variability in Service Operations.......................................................................... 5 Customer-Induced Variability.................................................................................................................. 5 Operations-Induced Variability................................................................................................................ 6 Two General Ways to Match Demand & Supply in Services......................................................... 8 “Chase Demand” Strategy........................................................................................................................ 8 “Level Capacity” Strategy......................................................................................................................... 9 Understanding Waiting Lines......................................................................................................... 9 Essential Features of Queuing Systems...................................................................................... 11 Calling Population................................................................................................................................... 11 Arrival Process......................................................................................................................................... 12 Queuing Configurations.......................................................................................................................... 15 Queuing Discipline.................................................................................................................................. 16 Service Process....................................................................................................................................... 16 Common Queuing Systems.......................................................................................................... 20 Queuing System Analysis............................................................................................................. 20 Designing an optimal queuing system......................................................................................... 23 Key Criteria for Capacity Planning............................................................................................... 23 Queuing Systems Practice Problems.......................................................................................... 23 Demand Management: Differential Pricing................................................................................. 24 Newsvendor Problem................................................................................................................... 26 Building blocks of Newsvendor Problem............................................................................................... 26 Newsvendor Solution.............................................................................................................................. 27 Managerial Insights on the Newsvendor Problem...................................................................... 28 1 Prof. Jalili Service Operations Management MG316 What is Yield Management?......................................................................................................... 29 Why is Yield Management so useful in the Service Industry?.................................................... 30 What do “Newsvendor”, “Overbooking” and “Advance-Selling” have in common?.............. 31 Yield Management Practice problems........................................................................................ 32 2 Prof. Jalili Service Operations Management MG316 Two Sides of a Business: Demand and Supply Understanding the dynamics of demand and supply is fundamental in the realm of service operations. Demand Demand refers to the number of customers seeking to access the service over a specific period. For example, in a restaurant, demand refers to the number of customers seeking to dine there over a specific period. To effectively respond to the fluctuating nature of demand, service operations managers rely heavily on demand forecasting and demand management. Demand forecasting involves predicting future customer demand based on historical data, trends, and external factors, such as holidays or local events. Accurate forecasting allows managers to allocate resources, such as staff and inventory, efficiently. On the other hand, demand management refers to the strategies used to influence or control demand to match the service capacity. This might include offering promotions during low-demand periods or implementing reservation systems during peak hours to prevent service delays. Later in this module, we will dive into “differential pricing” as common tactic to influence and shape demand, Service Capacity Supply, often termed as service capacity, is the number of customers that can be served or the number of service transactions that can be completed within a defined unit of time. Let’s review the capacity metrics from Module B and learn a few new terms: Design Capacity refers to theoretical maximum output under perfect conditions. For example, a restaurant is designed to seat 100 customers at full capacity. Effective Capacity refers to the output rate that can be expected under real-world conditions, considering factors like staff availability & equipment downtime. For example, due to staff limitations and kitchen efficiency, the restaurant effectively can serve only up to 80 customers at a time. 3 Prof. Jalili Service Operations Management MG316 Fixed Capacity refers to the level of output that cannot be easily changed or adjusted, often due to physical or technical limitations. For example, a conference room has a fixed capacity of 30 people due to fire safety regulations. Variable Capacity refers to the level of output that can be adjusted or scaled to meet changing demand, often through the use of technology or flexible arrangements. For example, an online webinar platform can host anywhere from 100 to 1000 participants, adjusting server capacity based on the number of registered attendees. Frontstage Capacity refers to the visible functions and interactions that occur directly between the service provider and the customer. For example, a retail store has 5 cashiers available for customer checkout, representing its frontstage capacity. Backstage Capacity refers to the behind-the-scenes functions and activities that support the visible (frontstage) operations but do not directly involve customer interaction. For example, the same retail store has 10 staff working on inventory, restocking, and pricing, which constitutes its backstage capacity. Backstage Frontstage Fixed (Long-Term) Kitchen Equipment Seating Area Capacity Storage Facilities Point of Sale Systems Variable (Short- Kitchen Staff Serving Staff. Term) Capacity Prep Stations Special Event Areas Capacity Utilization is a metric that shows how effectively a service operation uses its capacity to produce output. For example, a call center designed to handle 500 calls per hour (i.e., service capacity) but only receiving 400 calls has a capacity utilization of 80% (= 400/500 * 100%). 4 Prof. Jalili Service Operations Management MG316 Understanding Variability in Service Operations In service operations, variability refers to the inherent fluctuations and differences that can impact the performance, quality, and efficiency of services. Variability in service operations can arise from two main sources: customer-induced variability (demand side) and operations- induced variability (supply side) Customer-Induced Variability Variability induced by the customers can be categorized into five reasons: Arrival of customers Requests made by customers Capability of customers with respect to their expected involvement Effort customers are willing to exert Subjective Preference of customers for how service should be delivered Subective Arrival Request Capability Effort Preference The logic of the sequence is based on the typical, chronological stages in a customer service experience. Customers tend to arrive at a service location, make a request, engage with the experience based on levels of capability and effort, and then assess the experience through the lens of their subjective preferences. Customers passively involved in service production introduce arrival and request variability. Customers who actively participate in production can also be sources of capability and effort variability. Subjective preference variability is introduced by customers in all service encounters. How to address these variabilities? Generally, there are two strategies: either accommodate variability or find ways to reduce it. Accommodation strategy involves adjusting the service operation to cater to the variability, rather than trying to alter the variability itself. It is about being flexible and adapting the service to meet the diverse needs and expectations of customers. In contrast, the Reduction strategy aims to minimize or eliminate variability by standardizing the service process. This could involve setting specific guidelines or requirements for customers to follow, thus making the service delivery more predictable and easier to manage. 5 Prof. Jalili Service Operations Management MG316 Here’s an expanded breakdown of these variabilities and the corresponding examples strategies: Variability Variability Strategies: Accommodation Reduction Provide generous staffing to Require reservations to manage Arrival Variability handle peak times. customer flow Adapt to different customer Target customers based on their Capability Variability skill levels. capability to interact with the service. Cross-train employees to Limit the service breadth to reduce the Request Variability handle diverse requests. variation in requests. Do the work for customers to Reward customers for increased effort Effort Variability ease their effort. in the service process. Persuade customers to adjust their Diagnose expectations and Preference Variability expectations to align with the service adapt the service accordingly. offering. Operations-Induced Variability In service operations, variability is often influenced by several key factors, known as the Six M's. Each of these elements can introduce unpredictability in service quality and capacity, affecting overall performance. Man (People): Staff availability, skill, and performance create variability in service capacity. Understaffing or high turnover leads to longer wait times and less efficient service, particularly during peak hours. For example, if a restaurant has fewer servers during a busy dinner rush, customers will experience longer wait times. Machine (Equipment): Service reliability depends on technology and equipment. Malfunctioning tools, such as a broken grill, directly impact service delivery, slowing down preparation and limiting capacity. For instance, 6 Prof. Jalili Service Operations Management MG316 when a restaurant's POS system goes down, order processing slows, leading to delays in service. Method (Processes): Inconsistent procedures or workflows, such as deviations from standard food preparation or table turnover methods, lead to variability in service speed and quality. For example, if different chefs follow different recipes for the same dish, customers may receive inconsistent meal quality. Material: Variability in the supply chain—such as delays or poor-quality ingredients— affects service capacity. Lack of key ingredients can result in menu limitations, reducing customer satisfaction. For instance, if a shipment of fresh seafood is delayed, a restaurant may be unable to serve popular dishes. Mother Nature: Environmental factors like weather impact service operations. Outdoor seating capacity can vary based on weather conditions, causing shifts in service availability. For example, a rainstorm can force a restaurant to close its outdoor seating, reducing its overall capacity. Measurement: Inconsistent performance tracking or lack of feedback mechanisms can cause operational inefficiencies, as service issues may go undetected and unresolved. For instance, without proper tracking, a restaurant may not realize that certain menu items consistently take longer to prepare, impacting service speed. 7 Prof. Jalili Service Operations Management MG316 Two General Ways to Match Demand & Supply in Services In service operations, two common capacity management strategies are “chase demand” and “level capacity”. These strategies address how a service provider adjusts its capacity to match customer demand, but they come with trade-offs in terms of cost, flexibility, and customer experience. “Chase Demand” Strategy With a chase demand strategy, the service provider adjusts its capacity to match the demand fluctuations. This could involve hiring temporary staff, adjusting working hours, or using part-time employees to handle peak demand periods. Here are six tactics under this strategy: Chase Demand Description Example Risk Tactics Call centers increase Work Shift Align staff schedules Frequent changes may staff during product Scheduling with demand peaks. lower employee morale. launches. Supermarkets use self- Increase Customer Involve customers to Over-reliance can harm checkout kiosks during Participation reduce staff workload. service quality. busy times. Movable walls in Modify physical Higher maintenance costs conference centers Adjustable Capacity capacity based on and potential limits on optimize space demand. flexibility. utilization. Share resources to Food trucks sharing Potential conflicts over Sharing Capacity maximize utilization. commissary kitchens. resource allocation. Use part-time staff to Part-timers may lack full- Retailers hire extra staff Part-time Employees manage demand time employee during holidays. surges. commitment. Train staff for multiple Restaurant staff handle Increased training costs Crosstrain roles to increase different tasks as and potential loss of Employees flexibility. needed. specialization. Question: What are some trade-offs and operational challenges of chasing demand? 8 Prof. Jalili Service Operations Management MG316 “Level Capacity” Strategy A level capacity strategy keeps capacity constant regardless of demand fluctuations. In this case, the service provider maintains a steady workforce and operational level even when demand changes. Here are five tactics under this strategy: Level Capacity Description Example Pros/Risks Tactics Pro: Improves capacity Aligning fixed capacity Hotels segment business utilization. Segmenting with demand segments vs. leisure travelers; Risk: Misjudgment in Demand for accurate airlines use class segments can lead to forecasting. categories. inefficient capacity use. Restaurants offer "happy Pro: Smoothens demand Price reductions to Offering Price hour" specials; gyms offer and increases off-peak use. attract customers Incentives lower membership rates Risk: Overuse can lower during off-peak periods. for off-peak hours. perceived service value. Pro: Reduces idle time, Retail stores offer early- Promotions to drive balances demand. Promoting Off- morning sales; museums business during slower Risk: May not attract Peak Demand host special weekday periods. enough off-peak exhibits. customers. Pro: Diversifies revenue, Developing Add services to boost Spas offer yoga classes; optimizes resources. Complementary demand during off-peak cafes host book readings Risk: May attract customers Services times. or art events. who hurt core business. Provides visibility into Pro: Predictable demand, Restaurants use Reservation demand and reduces idle capacity. reservations; airlines Systems and compensates for Risk: Overbooking can hurt overbook flights to Overbooking cancellations with customer satisfaction if maximize capacity use. overbooking. mishandled. Question: What are some trade-offs and operational challenges of “level capacity”? Understanding Waiting Lines Waiting lines, or queues, form when demand for a service exceeds the system’s capacity to serve customers promptly. This often results from variability in either demand or service time. For instance, restaurants experience rush hours when customer traffic spikes unpredictably, while services like healthcare tend to have variable appointment durations, creating extended wait times. 9 Prof. Jalili Service Operations Management MG316 A queue is simply a line of waiting flow units (customers, products, etc.) that require service from one or more servers. Queues can be physical, like people waiting to check out at IKEA, or virtual, such as when customers are in a digital line for concert tickets. Queuing systems take many forms. In some cases, multiple customers are served simultaneously, like when passengers board a bus or elevator. In other cases, servers travel to the customer, as with a mobile mechanic or food delivery service. Services may also consist of multiple stages/phases. At a theme park, for example, visitors may queue first to buy tickets and then again for each ride, extending the total waiting experience. Additionally, some customers may receive priority over others. In airports, first-class passengers and those with special needs often board ahead of others, creating a tiered queuing system. Waiting has costs, both for customers and for firms. For customers, waiting often leads to feelings of boredom, frustration, or anxiety. Consider the DMV, where customers may wonder if they’ve chosen the fastest line or are being treated fairly. This feeling of "empty time" can make waiting seem even longer. Firms, on the other hand, face the risk of losing sales when customers abandon their purchases due to long waits. A crowded waiting area in a doctor's office, for example, can negatively impact other customers’ experiences and their overall satisfaction with the service. To reduce the negative impact of waiting, businesses can adopt several key strategies to improve the customer experience: 1. Manage Expectations: Set clear expectations from the start, like Disney World’s practice of displaying wait times, helping customers plan and mentally prepare. 2. Create Comfort: Enhance the waiting environment, such as Starbucks offering Wi- Fi and comfortable seating to make waiting more enjoyable. 3. Engage Immediately: Provide interaction early on, like restaurants handing out menus right away to reduce the mental burden of waiting. 4. Signal Progress: Use indicators like digital displays at takeout restaurants to show order status, reducing anxiety and helping customers feel reassured. 5. Communicate Transparently: Offer wait-time updates and options, as ride-sharing apps do with estimated arrival times, to reduce uncertainty and frustration. 6. Serve with Empathy: Show respect for the customer’s time by acknowledging delays, as pharmacies often do, to turn potential frustration into a positive experience. 10 Prof. Jalili Service Operations Management MG316 Essential Features of Queuing Systems Each queuing system is comprised of five components: (1) calling population (2) arrival process (3) queue configuration (4) queue discipline (5) service process Services obtain customers from a calling population. The rate at which customers arrive to the process determined by the arrival process. If servers are idle, then the customer is attended immediately; otherwise, the customer is diverted to a queue, which can have various configurations. When a server does become available, a customer then is selected from the queue and service begins. The policy governing the selection is known as the queue discipline. The service process can consist of no servers (i.e., self-service), one or more servers, or complex arrangements of servers in series or in parallel. After the service has been rendered, the customer departs the facility. At that time, the customer either might rejoin the calling population for future return or exit with no intention of returning. Calling Population In service systems, the calling population refers to the pool of potential customers who might request service. This population can be classified in two key ways: finite or infinite, and homogeneous or heterogeneous: Finite vs. Infinite Calling Population 1. Finite Calling Population: The number of potential customers is limited, meaning the system serves a specific, restricted group. For example, a corporate IT helpdesk only caters to the company’s employees. If the company has 500 employees, the helpdesk knows its total potential customer base and can allocate resources (technicians, service stations) accordingly. Another example is a small repair shop that serves only customers with warranty- covered items or a medical clinic with 20 available beds. 2. Infinite Calling Population: The number of potential customers greatly exceeds the system’s capacity. This creates more unpredictability in customer arrivals and often requires scalable services. For example, a supermarket or Disney theme park, where customers from a large, unrestricted population visit without a fixed limit. The service system must be prepared for fluctuating demand, especially during peak times like holidays or sales events. 11 Prof. Jalili Service Operations Management MG316 Homogeneous vs. Heterogeneous Calling Population 1. Homogeneous Calling Population: All customers share similar service needs. For example, a specialty dental clinic that only handles patients needing braces. The clinic can design its services around one type of procedure, simplifying the queuing process. 2. Heterogeneous Calling Population: Customers have varying service needs, requiring different treatment and expectations. For example, a movie theater attracts families, couples, and groups of friends, all with different expectations for seating and movie preferences. Similarly, a travel agency may serve luxury travelers, backpackers, and family vacationers, each expecting a tailored service. Managing this diversity in a queue system requires flexibility and planning. Arrival Process The arrival process describes how customers or flow units enter a service system. A key factor in managing queues effectively is understanding how often arrivals occur and whether these arrivals follow a predictable pattern or not. The primary concept used to describe this is inter-arrival time, which refers to the time between consecutive customer arrivals. Arrival Rate and Inter-Arrival Times: Two Sides of the Same Coin The arrival rate and inter-arrival times are closely related concepts in queuing systems, representing two perspectives on the same coin. The arrival rate is the number of customers (or flow units) that arrive at a service system over a given period, while inter-arrival time refers to the time between consecutive arrivals. For example, if the arrival rate at a coffee shop is 12 customers per hour, the average inter-arrival time is 5 minutes (60 minutes divided by 12). These two measures are just different ways of understanding how customers enter the service system. The choice of perspective depends on what aspect of the process you’re focused on—managing overall volume (arrival rate) or spacing between arrivals (inter-arrival time). 12 Prof. Jalili Service Operations Management MG316 Deterministic Arrivals and Constant Inter-Arrival Time In systems with scheduled arrivals, customers arrive at predictable, regular intervals, resulting in a constant inter-arrival time. These arrivals can be planned and managed efficiently because the service system knows exactly when the next customer will show up. At a dental clinic, patients are scheduled for appointments every 30 minutes. This creates a constant inter-arrival time, where the staff can predict exactly when the next patient will arrive and plan resources accordingly. In this case, the arrival rate is 2 patients per hour, and the inter-arrival time remains fixed at 30 minutes. Scheduled arrivals allow for precise staffing and resource allocation. Since there’s no variability in inter-arrival times, the service provider can focus on maintaining efficiency without worrying about sudden changes in demand. Random Arrivals and Variable Inter-Arrival Time In systems with random arrivals, customers show up unpredictably, causing variation in the inter-arrival times. These fluctuations make it difficult to predict when the next customer will arrive. Random arrivals are common in environments where there is no control over when customers decide to use the service. At a gas station, customers arrive randomly throughout the day. One customer might arrive after a few seconds, while the next might arrive several minutes later. This results in variable inter-arrival times that can range from seconds to minutes, depending on the flow of traffic or other factors. The average arrival rate might be 20 customers per hour, but the exact times between arrivals are inconsistent. This unpredictability makes resource planning more challenging. The service system must be flexible enough to handle sudden spikes in demand, such as having additional staff on hand or using self- service options during busy periods. Statistical modeling helps capture the random arrivals and the variation in inter-arrival times. Two key metrics are used: the arrival rate (λ), which is the number of arrivals within a specific time period, and the inter-arrival time (1/λ), which is the time between two successive arrivals. Let’s look at common real-world examples. 13 Prof. Jalili Service Operations Management MG316 Carwash: At a carwash, the arrival rate (λ) might be 10 cars per hour, which means, on average, one car arrives every 6 minutes. This average inter-arrival time (1/λ) helps in predicting how often a new car will enter the queue. Call Center: In a call center, the arrival rate (λ) could be 3 calls per minute. This translates to an inter-arrival time of 20 seconds (1/λ) between successive calls, helping managers allocate the right number of agents to handle the incoming calls efficiently. Home Appliances Needing Repair: For a home appliance repair service, the arrival rate (λ) might be 4 maintenance requests per season. The corresponding inter-arrival time would be around 13 weeks between two maintenance needs (1/λ). Understanding this helps with scheduling technicians and ordering spare parts in advance. Two key probability distributions are often used to model random arrivals and inter-arrival times: Poisson Distribution is commonly used to model the number of arrivals (λ) within a given time interval. For example, the number of cars arriving at a carwash in an hour or the number of calls received by a call center in a minute follows a Poisson distribution. Exponential Distribution models the inter-arrival time (1/λ), or the time between two successive arrivals. In the case of a call center, the time between the arrival of two calls would be modeled using an exponential distribution, as this process is memoryless and random. The following histogram shows a sample of 1000 inter-arrival times, resembling an exponential distribution with average inter-arrival time of 2 minutes. o 298 customers arrived within less than 0.7 minutes (i.e., the busiest time where customers arrived quickly one after another). o 195 customers arrived between 0.7 and 1.5 minutes after the previous customer. o 140 customers arrived between 1.5 and 2.2 minutes after the previous customer, and so on. Inter-Arrival Time (Exponential Distribution) 298 195 140 104 87 58 33 24 19 10 11 5 4 5 2 2 3 [0.0, 0.7] (0.7, 1.5] (1.5, 2.2] (2.2, 2.9] (2.9, 3.7] (3.7, 4.4] (4.4, 5.1] (5.1, 5.8] (5.8, 6.6] (6.6, 7.3] (7.3, 8.0] (8.0, 8.8] (8.8, 9.5] (9.5, 10.2] (10.2, 11.0] (11.0, 11.7] (11.7, 12.4] 14 Prof. Jalili Service Operations Management MG316 Queuing Configurations Queuing configuration refers to the structure of the queue system, including the number of queues, their locations, spatial requirements, and their effects on customer behavior. Single Line Multiple Lines Multiple Lines or a Single Line: Multiple Lines: In this system, each service point (e.g., cash register) has its own queue. Customers choose a line, which can lead to varying speeds. At a grocery store, each cashier has their own line. If you're in the fastest line, it's great, but if your line is slow, frustration can build. This queuing configuration works well when all servers handle similar tasks, but can feel unfair to customers stuck in slower lines. Single Line: Here, one line feeds into multiple service stations, ensuring a first-come, first- served system. At a bank or airport check-in, customers wait in a single queue that directs them to the next available agent. This queue configuration creates a sense of fairness since no one gets stuck in a slow line, and servers are evenly loaded. Customer Impatience and Behaviors Impatience in queues can lead to various behaviors: Balking: Customers might avoid joining the queue if it looks too long. Jockeying: Once in line, they may switch between queues to find the fastest one. Reneging: After waiting for a while, some may leave the line out of frustration. 15 Prof. Jalili Service Operations Management MG316 Queuing Discipline The queuing discipline is the policy that determines the order in which customers are served in a queue. Different queuing disciplines are used depending on the service context, and not all customers are treated equally in every system. Common Queuing Disciplines: 1. First-Come, First-Served (FCFS): This is the most common rule, where customers are served in the order they arrive. At a fast-food restaurant, customers are processed based on the time they enter the line. The first person to join the queue is the first person to be served. 2. Last-Come, First-Served (LCFS): In some systems, the last customer to arrive is served first. This typically happens when jobs are stacked. In a warehouse, the most recently stacked items might be retrieved first if they're more accessible. 3. Round-Robin Service: Customers share a server, with the server rotating between them, providing service in small intervals. A dentist moves back and forth between multiple patients, performing short tasks for each and alternating between them. 4. Priority-Based Service: Certain customers are given priority due to the urgency or value of their needs. In an emergency room, patients with life-threatening conditions are treated before those with minor injuries, regardless of arrival time. VIP customers at a luxury store may be served immediately, bypassing the general queue. 5. Preemptive Service: A server may interrupt current work to handle more urgent tasks, which can pause or delay regular service. A rush order at a manufacturing plant might preempt regular production schedules, requiring immediate attention over standard jobs. 6. Shortest Processing Time (SPT): Customers requiring the least amount of service time are prioritized, which helps minimize the overall time spent in the system. In a job shop, a small order might be processed before a larger one, even if the larger order arrived first. Service Process The service process in a queuing system defines how customers are served once they reach the front of the queue. It includes the number of servers, the arrangement of service points, and the nature of the service times. Let's explore the common configurations with relatable examples. Service Time Just like inter-arrival time refers to the time between customer arrivals, service time refers to the time it takes to serve each customer once they reach the front of the queue. Sometimes service time is referred to as inter-departure time. Both service time and inter-arrival time can be either constant or random, and this distinction has a significant impact on how queues are managed. 16 Prof. Jalili Service Operations Management MG316 1. Constant Service Time: When service time is constant, each customer requires the same amount of time to be served, making the process predictable and easier to manage. In an automated car wash, every car takes exactly 5 minutes to go through the system. 2. Random Service Time: In many service environments, service time is variable due to differences in customer needs or server performance. This adds unpredictability, similar to random inter-arrival times, and can lead to longer or shorter waits depending on how long each customer needs. At a hair salon, one customer might take 30 minutes for a simple haircut, while another may take an hour for a more complex treatment. Table below shows four possible combinations for constant/random nature of inter-arrival times and service times: Constant Inter-Arrival Time Random Inter-Arrival Time A factory with a machine-paced In a self-service car wash, Constant assembly line, products arrive at a steady customers arrive randomly, but Service rate and are processed within a set each car wash takes the same Time timeframe, creating a smooth flow. amount of time. In a doctor’s office with an appointment In a coffee shop, customers arrive Random system patients arrive at fixed intervals randomly, and their orders take Service (e.g., every 30 minutes), but each different amounts of time to Time appointment varies in length depending prepare. on the complexity of the patient's issue. Statistical modeling helps capture the variability in service times. Two key metrics are used: the service rate (μ), which is the number of customers that can be served within a specific time period, and the service time (1/μ), which is the time taken to serve each customer. Let's explore some real-world examples: Carwash: At a carwash, the service rate (μ) might be 10 cars per hour, meaning each car takes 6 minutes (1/μ) to go through the wash. This average service time helps the business determine how many cars can be processed in a given period. Call Center: In a call center, the service rate (μ) could be 20 calls per hour. This translates to an average service time of 3 minutes per call (1/μ), enabling managers to allocate agents based on call volumes and expected service duration. Home Appliance Repairs: For a home appliance repair service, the service rate (μ) might be 2 repairs per day, with each repair taking an average of 4 hours (1/μ). Knowing the service time helps schedule technicians effectively and manage their workload. The following histogram shows a sample of 1000 service times, resembling an exponential distribution with average service time of 1.5 minutes. 17 Prof. Jalili Service Operations Management MG316 290 services were completed within less than 0.6 minutes. 204 services were completed between 0.6 and 1.1 minutes. 165 services were completed between 1.1 and 1.7 minutes and so on. 5 services took more than 8.3 minutes. Can you see why? Service Time (Exponential Distribution) 350 300 290 250 200 204 150 165 100 93 90 50 43 35 21 19 10 9 4 6 0 6 1 1 0 1 1 0 0 0 0 1 0 [0.0, 0.6] (0.6, 1.1] (1.1, 1.7] (1.7, 2.2] (2.2, 2.8] (2.8, 3.3] (3.3, 3.9] (3.9, 4.4] (4.4, 5.0] (5.0, 5.5] (5.5, 6.1] (6.1, 6.6] (6.6, 7.2] (7.2, 7.7] (7.7, 8.3] (8.3, 8.8] (8.8, 9.4] (9.4, 9.9] (9.9, 10.5] (10.5, 11.0] (11.0, 11.6] (11.6, 12.1] (12.1, 12.7] (12.7, 13.2] (13.2, 13.8] Types of Service Process Configurations The following figure shows four configurations for the service process 18 Prof. Jalili Service Operations Management MG316 Single-Channel Multi-Channel In this system, there is only one Here, multiple servers work server available for all customers. simultaneously, each serving one Every customer must wait their customer at a time. For example, a turn for that single service point. supermarket with multiple checkout Single Phase For example, a toll booth with only counters. Each counter has its own one lane open. Every car must pass line, and customers are served at through this one booth, creating a different counters simultaneously. line behind it. This system has both multiple servers and multiple stages. Customers move In this configuration, the customer through different stages and are moves through multiple stages of served by various servers in each service, but there is still only one phase. For example, a hospital channel or line for all customers. Multi-Phase emergency room. Patients first check For example, a car wash where a in (first phase), then see a nurse for car goes through multiple triage (second phase), and finally phases—rinsing, soaping, and receive treatment from different drying—all in one lane. doctors in different rooms (third phase). Static vs. Dynamic Service Processes 1. Static Processes: These processes remain consistent over time and do not adjust to external factors. o Self-service: Customers complete the service themselves. Using an ATM to withdraw money or self-checkout counters at grocery stores. o Machine-paced: The rate of service is controlled by machines, maintaining a constant pace. Conveyor belts in factories or vending machines. 2. Dynamic Processes: These processes change based on factors like demand or resources. o Varying Service Rate: Service speed can change based on demand. Customer support hotlines may have longer wait times during peak hours, while restaurants may serve customers faster during off-peak times. o Opening and Closing Service Lanes: Adjusting the number of service points based on real-time demand. Supermarkets open more checkout lanes when there's a rush, and toll booths open more lanes during peak traffic hours 19 Prof. Jalili Service Operations Management MG316 Common Queuing Systems One of the most common types of queuing systems is the M/M/1 queue, which stands for a single-server system where both inter-arrival times and service times follow an exponential distribution (denoted by M, for "Markovian"). This system assumes random, memoryless arrivals and service rates, typical of many service environments like banks, call centers, or help desks. Other variations of this model include: M/M/c: Multiple servers (c) but still using exponential inter-arrival and service times. This model is more applicable in environments like hospitals or service desks where multiple employees serve customers. M/D/1: A single server with deterministic (constant) service times, which might be used in automated processes where service time is always the same, such as in certain manufacturing lines. M/G/1: A system with a single server, but the service time distribution is general, allowing for more variability in the time it takes to serve each customer. Queuing System Analysis Analyzing how a queuing system performs is essential for understanding its efficiency and effectiveness. Several key metrics help measure and track performance in any queuing system. λ: Average customer arrival rate 𝜇: Average service rate per one server c: number of servers λ/(c* 𝜇) = system utilization λ: Average customer arrival rate Lq : Average number of customers in line Wq: Average waiting time Lq = λ * Wq λ: Average customer arrival rate Ls : Average number of customers in service Ws: Average service time Ls = λ * Ws 20 Prof. Jalili Service Operations Management MG316 λ: Average customer arrival rate L: Average number of customers in the system (in queue + in service) W: Average time in the system (in queue + in service) L= λ * W px: Probability of exactly x customers in the system (in queue + in service). 21 Prof. Jalili Service Operations Management MG316 px Name of Probability of Number Space the Inter-Arrival Service exactly x queuing Times Times of Capacity Lq Wq Ls Ws Servers Limit customers in system the system (in queue + in service). Exponentially Exponentially 𝝀 𝝀 Lq 𝝀 1 𝝀 𝝀 " M/M/1 c=1 Unlimited × (1 − )( ) Distributed Distributed 𝝁 𝝁−𝜆 𝝀 𝝁 𝝁 𝝁 𝝁 Exponentially 𝝀 𝝀 Lq 𝝀 1 M/D/1 Constant c=1 Unlimited × Too complex Distributed 𝝁 𝟐( 𝝁 − 𝜆) 𝝀 𝝁 𝝁 𝝀 Read From 𝐿# 1 (1 − 𝝁) 𝝀 Exponentially Exponentially M/M/1/N c=1 N 1- p0 ( )" Distributed Distributed Excel 𝝀(1 − 𝑝$ ) 𝝁 𝝀 1 − ( 𝝁)$%& 𝝁 Exponentially Exponentially Read From Lq 𝝀 1 Read From M/M/c c>1 Unlimited Distributed Distributed Excel or Table 𝝀 𝝁 𝝁 Excel 22 Prof. Jalili Service Operations Management MG316 Designing an optimal queuing system Designing an optimal queuing system involves balancing customer satisfaction and operational efficiency. Determining the right number of servers is key to minimizing costs while avoiding long wait times that can hurt the customer experience. Businesses must decide between single or multiple lines and consider whether dedicated stations for special tasks would improve operations. Properly sized waiting spaces are also important to prevent overcrowding. The system should be cost-effective yet capable of timely service. Companies must manage the costs of waiting space, as well as the potential loss of business from customers who balk or renege, as unmanaged wait times can lead to reduced goodwill and disruption to business operations. Key Criteria for Capacity Planning Criteria 1: The sum of capacity cost and customer waiting costs should be minimized. o Example: A movie theater balances the cost of hiring ticket counter staff with minimizing wait times for customers purchasing tickets before showtime. The goal is to minimize the combined cost of hiring staff and the potential dissatisfaction of waiting customers. Criteria 2: Improve Wait Time (Wq). o Reducing the time customers spend waiting is essential for keeping them satisfied. o Example: An online retailer may implement more efficient order-processing systems to shorten the time customers wait for their deliveries. Criteria 3: Improve Service Level. o Service levels represent the speed and quality of service provided to customers. o Example: In a hospital emergency room, having enough staff on hand during peak times ensures patients are seen quickly, improving the service level and overall patient experience. Criteria 4: The probability of losing sales remains below a specific limit. o Businesses must ensure that the chance of losing customers due to long wait times stays within acceptable levels. o Example: An e-commerce website may track the percentage of customers who abandon their shopping carts if page loading times are too slow, aiming to reduce that abandonment rate to below 5%. Queuing Systems Practice Problems Check BrighSpace Module D for see exercises and solved examples of Queuing Systems. 23 Prof. Jalili Service Operations Management MG316 Demand Management: Differential Pricing Differential pricing, also known as price discrimination, is a pricing strategy in which businesses charge different prices for the same product or service to different customers or segments of customers. In first-degree price discrimination, each customer is charged the maximum price they are willing to pay. This is also called personalized or perfect price discrimination. Businesses assess the individual’s willingness to pay by analyzing personal data or negotiating directly. For example, a car dealership might negotiate a different price for the same vehicle depending on the customer's financial capacity or negotiation skills. The goal is to capture the entire consumer surplus by tailoring prices to each buyer. Professionals like lawyers and accountants may charge clients based on their specific circumstances. Wealthier clients or businesses may be charged more for the same service than smaller clients with less financial capability, reflecting a tailored pricing strategy to capture the maximum willingness to pay. In Second-degree price discrimination, customers are charged different prices based on the quantity they buy or the version of the product they choose. Here, bundling and versioning are common strategies. For example, a software company might offer basic, premium, and enterprise versions of their service, allowing customers to self-select the package that fits their needs and budget. Alternatively, customers may receive discounts for purchasing larger quantities. This form of pricing encourages customers to voluntarily choose the option that yields the most profit for the company, based on their usage or needs, without requiring the company to know each customer’s exact willingness to pay. See a variety of example tactics: 2nd degree price Description Examples discrimination tactics Quantity-Based Discounts for bulk purchases or "Buy one, get one free," discounts for Discrimination volume-based pricing. larger quantities. Versioning/Feature- Offering different product/service SaaS platforms offering basic, premium, Based Discrimination tiers based on features. and enterprise versions. Offering multiple Cable packages bundling channels, Bundling products/services together at a telecom bundles with different data/call discounted price. limits. Time-Based Price Prices vary based on the time of Early bird discounts, dynamic pricing in Discrimination purchase or service usage. hospitality, utility peak/off-peak rates. Pricing changes based on Ski resorts offering peak season rates Seasonal Pricing seasonality of demand. vs. off-season discounts. Third-degree price discrimination occurs when companies divide customers into distinct segments based on observable characteristics, such as age, profession, or location, and 24 Prof. Jalili Service Operations Management MG316 charge different prices to each group. For example, airlines often charge different prices for business and economy class, where each segment is clearly defined, and customers have limited influence over the price they pay based on the segment they fall into. Students or seniors might receive discounts in retail stores or movie theaters because these groups are seen as having lower willingness or ability to pay. In this case, prices are more rigid and dictated by the segment the customer belongs to, rather than through individual negotiation or choice. See a variety of example tactics: 3rd degree price Description Examples discrimination tactics Discounts for children, students, and Offering special pricing to Age-Based Discounts seniors at movie theaters or public specific age groups. transport. Membership-Based Offering lower prices to members Costco or Sam's Club membership Pricing who pay an annual fee. pricing. Pricing varies based on the user's Software companies charging different Geographic Pricing location. prices depending on the country. Occupation-Based Special pricing for certain Discounts for teachers, military Discounts professions. personnel, and healthcare workers. Pricing benefits for frequent Airlines' frequent flyer programs, hotel Loyalty Program Pricing customers. loyalty discounts. Charging different prices based Differing prices at hair salons for men Gender-Based Pricing on gender (controversial and and women (often criticized and being (Controversial) often discouraged). phased out in many regions). Cons of Differential Pricing Differential pricing comes with several notable drawbacks. First, it raises legal and ethical concerns, as businesses may face potential discrimination claims and regulatory scrutiny. Additionally, the cost of implementation is high, requiring substantial investment in technology and adding operational complexity. There is also the risk of negative customer perception, as differential pricing can be seen as unfair, potentially leading to brand damage and customer dissatisfaction. Competitively, the strategy may trigger price wars or encourage copycat tactics from other firms. Moreover, accurately segmenting the market for differential pricing can be challenging, risking over-complication. Finally, the approach relies on personal data, raising significant privacy and data security concerns. 25 Prof. Jalili Service Operations Management MG316 Newsvendor Problem How many newspapers The Newsvendor problem is a classic operations management should I stock? problem that addresses inventory decisions under demand uncertainty. Let’s look at an example: Alex runs a newsstand. Each newspaper costs him $1. He sells each newspaper for $3. At the end of the day, any unsold newspaper is worthless, and Alex throws them away. Alex needs to determine how many newspapers to order daily. Building blocks of Newsvendor Problem Demand Uncertainty: The primary challenge in the Newsvendor problem is predicting uncertain future demand. The demand is usually modeled as a random variable with a given probability distribution. Using past historical data and various demand forecasting techniques, such demand information can be modeled. For example, Alex believes the demand (D) for his newspapers is random with the following probability distribution: Demand (D) 35 40 45 50 55 60 >60 Probability 0.11 0.17 0.22 0.22 0.17 0.11 0 Cost Parameters: The second step in the Newsvendor problem is identifying the cost Alex must bear if he overstocks or understocks newspapers. a. Underage cost (Cu): This is the cost incurred when you don’t order enough inventory to meet demand. It's the cost of missing a sale or the opportunity cost due to lost revenue and unsatisfied customers. For example, Alex will lose profit of $3--$1=$2 per unit if a missed sales, then his Cu would be $2. b. Overage cost (Co): This is the cost incurred when you order more than what was demanded. It includes the cost of holding excess inventory, possible markdowns, or even disposal of unsold goods. In the case of Alex, Alex will have to bear the loss of his unit cost of $1, then Co would be $1. 26 Prof. Jalili Service Operations Management MG316 Decision and Objective: In the Newsvendor problem, the key decision is determining the optimal inventory level, denoted as Q∗. The objective is to minimize the total expected costs by balancing the risks of stock-outs (not enough inventory) with the costs of excess inventory (leftover stock). Newsvendor Solution Through a very elegant and relatively simple set of steps, a magical formula is born as the solution to the newsvendor problem. This formula says that Alex should stock enough inventory such that 𝑪𝒖 there’s a 𝑪 %𝑪 chance he’s going to meet demand. Such approach would achieve minimizing the 𝒖 𝒐 goals of total expected costs. Recall that the service level is the probability that the available inventory will meet or exceed the actual demand. It can be written as: Service Level=Pr(Demand ≤ 𝑪𝒖 Q). In other words, Alex should optimally provide Service level of 𝑪 %𝑪. 𝒖 𝒐 𝑪𝒖 Optimal Service Level = Pr (Demand ≤ Q∗)= 𝑪 𝒖 %𝑪𝒐 Let’s solve this problem for Alex: Step 1: Calculate the cumulative probabilities: Pr(D