Module 2 Notes 2 PDF
Document Details
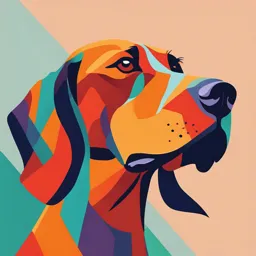
Uploaded by ReadableArlington
University of Kansas
Tags
Summary
These notes cover module 2 on data management and its importance in business analytics. They discuss the purpose, difficulties, lifecycle stages, and sources of data. The notes also detail how businesses can use data for monetization.
Full Transcript
Module 2 TOC Data Management........................................................................................................................2 Purpose...................................................................................................................................2 Dif...
Module 2 TOC Data Management........................................................................................................................2 Purpose...................................................................................................................................2 Difficulties...............................................................................................................................2 Need to easily process and convert data................................................................................2 Constant need to store data effectively..................................................................................2 Demand to continually optimize IT agility and costs................................................................3 Lack of data insight...............................................................................................................3 Difficulty maintaining data-management performance levels.................................................3 Challenges complying with changing data requirements........................................................3 5 Stages of the Data Lifecycle.......................................................................................................3 Creation...............................................................................................................................4 Storage.................................................................................................................................4 Usage...................................................................................................................................4 Archival................................................................................................................................4 Destruction..........................................................................................................................4 Data Sources...............................................................................................................................4 Data Use Examples..................................................................................................................5 Data monetization.......................................................................................................................5 How Businesses Can Monetize Data.........................................................................................6 Improving Internal Business Processes and Decisions............................................................6 Wrapping Information Around Core Products and Services.....................................................6 Selling Information Offerings to New and Existing Markets......................................................7 Data Management Purpose Data management is essential for successful business analytics and using data in an organization. The large volume, variety, and velocity (big data) of business data requires an effective business plan and processes for such tasks as gathering, storing, controlling access, archiving, and deleting data while it is within a business. Data management involves the processes used to effectively achieve these data-centric tasks. The goal of data management is to help those authorized persons and entities access the data they need within the organizational policy and legal parameters. Then, those individuals are able to conduct business analytics to support decision-making and maximize the value of the data for the organization. Review the flashcards familiarize yourself with key data terms related to data management, data monetization, and the data lifecycle. Hover over each card to reveal the definition. Difficulties According to recent research from PwC and Iron Mountain (White, 2015), only a small percentage of companies reported effective data management practices. Overall, 43 percent of companies surveyed “obtain little tangible benefit from their information,” while 23 percent “derive no benefit whatsoever,” according to the study. Businesses might be investing significant money into data capture, but then drop the ball when it comes time to actually use that data. When businesses attempt to effectively manage or control data, many challenges arise. Further complicating matters is the amount of data, data that is scattered in data silos across the organization, and data classification and regulation requirements. Database corporation Oracle has identified as number of challenges related to data management. Open each panel to reveal more information about each difficulty. Need to easily process and convert data Collecting and identifying the data itself doesn’t provide any value—the organization needs to process it. If it takes a lot of time and effort to convert the data into what they need for analysis, that analysis won’t happen. As a result, the potential value of that data is lost. Constant need to store data effectively In the new world of data management, organizations store data in multiple systems, including data warehouses and unstructured data lakes that store any data in any format in a single repository. An organization’s data scientists need a way to quickly and easily transform data from its original format into the shape, format, or model they need it to be in for a wide array of analyses. Demand to continually optimize IT agility and costs With the availability of cloud data management systems, organizations can now choose whether keep and analyze data in on-premises environments, in the cloud, or in a hybrid mixture of the two. IT organizations need to evaluate the level of identicality between on-premises and cloud environments in order to maintain maximum IT agility and lower costs. Lack of data insight Data from an increasing number and variety of sources such as sensors, smart devices, social media, and video cameras is being collected and stored. But none of that data is useful if the organization doesn’t know what data it has, where it is, and how to use it. Data management solutions need scale and performance to deliver meaningful insights in a timely manner. Difficulty maintaining data-management performance levels Organizations are capturing, storing, and using more data all the time. To maintain peak response times across this expanding tier, organizations need to continuously monitor the type of questions the database is answering and change the indexes as the queries change—without affecting performance. Challenges complying with changing data requirements Compliance regulations are complex and multi-jurisdictional, and they change constantly. Organizations need to be able to easily review their data and identify anything that falls under new or modified requirements. In particular, personally identifiable information (PII) must be detected, tracked, and monitored for compliance with increasingly strict global privacy regulations. Business analytics professionals play an important role in data management throughout their organization. They influence policy, decide on data requirements, enforce, practice, and maintain appropriate levels of data access to maintain data confidentiality and integrity, and have an important function in the determination of how long to keep organizational data. 5 Stages of the Data Lifecycle Businesses use such frameworks as the data lifecycle to ensure they have effective data management processes in place to meet organizational goals. As discussed in Module 1, according to WhatIs.Com, the “data lifecycle is the sequence of stages that a particular unit of data goes through from its initial generation or capture to its eventual archival and/or deletion at the end of its useful life” (Kirvan & Wigmore, 2023). The data lifecycle helps organizations manage the flow of data throughout its lifecycle—from initial creation through destruction. Creation The first phase of the data lifecycle is the creation/capture of data. This can include acquiring data from external sources (e.g. from governments or databrokers), creating data (e.g. creating data through apps, surveys, or Internet sites), or using shared data from business supply chain partners or customers Storage As data is collected, it must be stored in databases that support access requirements and provide security protection. Usage Within this stage, data can be viewed, processed, modified, and saved. All uses of data should create an audit trail. Where appropriate, this data can be made available to those outside the business (e.g. supply chain partners, customers, etc.). Archival Data is moved from operational/production systems and stored in case it is required again. Destruction Once it is determined the data is no longer valuable, the costs of the data outweigh the benefits, or based on legal regulations, data is purged from businesses. This may involve the simple deletion or secure destruction (the equivalent of throwing papers in a trashcan or using a crosscut shredder). Data Sources The first stage of the data lifecycle is the most important for data management and business analytics. This is where the data required to be analyzed for a business problem or decision is determined. Of course, if data is not gathered or created, it is data that does not need to be managed. The sources for data include: Internal Sources: Internal sources of data include data that is generated by business processes such as finance and accounting, supply chain systems, Internet of Things devices, human resources systems, and so on. Much of this data is tactical data used for day-to-day operations. The data silos we discussed in Module 1 can make this data difficult to access or unknown to business analytics professionals. Government External Sources: Federal, state, and local governments collect huge amounts of data that can be collected and used by private businesses. An example is data.gov, which provides access to datasets published by agencies across the federal government such as Census Bureau data, agricultural data, weather data, banking and economic data, and healthcare data. Private External Data Sources: These include data brokers. A data broker is a company that collects data from a variety of sources (e.g. governments, mobile phone apps, Internet sites…), processes it, and packages it to sell to individuals or companies. Data Use Examples Companies use external data for a variety of reasons. The following examples come from a Deloitte analysis: A credit union personalized marketing offers for members based on customer profiles developed with the aid of external data including consumer demographic data, social media data, and geolocation data. A digital media company used external data to better predict and improve employee retention rates. Examples include postings with the aid of external data: job websites, government economic and labor sources, and social media data. A logistics company uses external data to predict disruptions to clients' supply chains with the aid of external data, including social media data, online news postings, and data from suppliers. An agricultural giant launched a new service to help farmers predict and optimize crop yields with the aid of external data including geolocation data, weather data, and Internet of Things data. A grocer uses external data to improve demand forecasting and reduce stock issues with the aid of external data, including weather data, data from suppliers, and economic data and forecasts. Data monetization To effectively use and manage data as well as to advocate for business analytics processes within the business, leadership must understand the value of data. In our “What’s Your Data Worth” article, we can see how Microsoft paid $26.2 billion for LinkedIn and the Caesar’s Casino Group’s high-roller data (in their Total Rewards customer loyalty program) was valued at $1 billion in their bankruptcy proceedings. These two valuations were primarily based on the value of the existing data (and the future value of the analytics that could be done on this data). This provides insight into the value of data for organizations. Understanding and creating value from data is probably the most important aspect of business analytics initiatives in business. Data monetization involves the creation of that value from data for a business. In the following video, Dr. Salmans discusses the concept of data monetization and its potential for businesses. How Businesses Can Monetize Data The reality is, despite data and analytics hype and expectations, most organizations are not successfully monetizing their data. Businesses can monetize data utilizing three different approaches: 1. Improving internal business processes and decisions 2. Wrapping information around core products and services 3. Selling information offerings to new and existing markets We will now take a look at each approach in more detail. Improving Internal Business Processes and Decisions Organizations can generate value from their data and business analytics by increasing the effectiveness or efficiency of business processes. This might involve providing more insight in a particular area or to better inform business decisions or sharing data internally across business work centers. The following provides an example of improving internal business processes and decisions: Microsoft provides a real-life example of improving internal business products. The company created a new, integrated customer system that could produce 360-degree views of Microsoft’s relationships with corporate customers, including what those customers bought, what issues they encountered, and how the company engaged with them. The new system saved 10 to 15 minutes per sales opportunity by eliminating the need for Microsoft salespeople to manually search for and prepare data. The system also helped sales executives more accurately manage their pipelines; it used predictive analytics and machine learning to compute the likelihood of a successful sales engagement based on data that the salesperson provided about an opportunity. Wrapping Information Around Core Products and Services Organizations can look at existing services or processes and add additional data- and analytics-based context to provide more insight to customers. The following provide examples of data wrapping: FedEx Corp. was an early exemplar of wrapping when it introduced online package tracking as a free service. Capital One offers free credit scores. Selling Information Offerings to New and Existing Markets As organizations gain better understanding of the value of their data and analytics, they can sell this information to others directly, a direct way of gaining value. The following provide examples of selling information offerings: McKinsey: A business provides access to its business analytics and research behind a paywall Royal Dutch Shell: Licensing oil and gas exploration data to other companies in the industry American Express: Selling transaction data to merchants and business partners, enabling them to analyze customer spending patterns and improve their marketing strategies