Book - Business Analytics - MRCET (1).pdf
Document Details
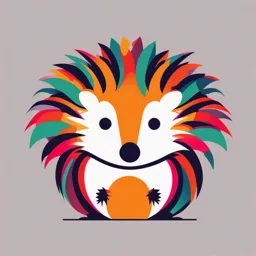
Uploaded by FineDialogue
Christ University
2023
Tags
Full Transcript
DIGITAL NOTES ON BUSINESS ANALYTICS BASICS B.TECH III YEAR – II SEM (2023-2024) DEPARTMENT OF AERONAUTICAL ENGINEERING MALLA REDDY COLLEGE OF ENGINEERING & TECHNOL...
DIGITAL NOTES ON BUSINESS ANALYTICS BASICS B.TECH III YEAR – II SEM (2023-2024) DEPARTMENT OF AERONAUTICAL ENGINEERING MALLA REDDY COLLEGE OF ENGINEERING & TECHNOLOGY (Autonomous Institution – UGC, Govt. of India) Recognized under 2(f) and 12 (B) of UGC ACT 1956 (Affiliated to JNTUH, Hyderabad, Approved by AICTE - Accredited by NBA & NAAC – ‘A’ Grade - ISO 9001:2015 Certified) Maisammaguda, Dhulapally (Post Via. Hakimpet), Secunderabad – 500100, Telangana State, India MALLA REDDY COLLEGE OF ENGINEERING AND TECHNOLOGY B.Tech -III Year-II Sem (ANE) L/T/P/C 3/-/-/3 BUSINESS ANALYTICS BASICS COURSE OBJECTIVES To help students in under standing how the managersuse business analytics for managerial decision making. LearningOutcome/s: The students will be familiar with the practices of analyzing and reporting the business data useful for the insights of business growth and development. Unit-I:Understanding Business Analytics Introduction: Meaning of Analytics - Evolution of Analytics - Need of Analytics - BusinessAnalysis vs. Business Analytics - Categorization of Analytical Models - Data Scientist vs. DataEngineervs. BusinessAnalyst-BusinessAnalytics inPractice-Types ofData-RoleofBusinessAnalyst. Unit-II:Dealing with Data and DataScience Data: Data Collection - Data Management - Big Data Management - Organization/Sources ofData-ImportanceofDataQuality-DealingwithMissingorIncompleteData-DataVisualization-Data Classification. DataScienceProjectLifeCycle:BusinessRequirement-DataAcquisition-DataPreparation - Hypothesis and Modeling - Evaluation and Interpretation - Deployment - Operations - Optimization-Applications forDataScience Unit-III:Data Mining and Machine Learning Data Mining: The Origins of Data Mining - Data Mining Tasks - OLAP and MultidimensionalDataAnalysis- Basic ConceptofAssociation AnalysisandCluster Analysis. Machine Learning: History and Evolution - AI Evolution - Statistics vs. Data Mining vs. DataAnalytics vs. Data Science - Supervised Learning - Unsupervised Learning - ReinforcementLearning-FrameworksforBuildingMachine Learning Systems. Unit-IV:Applications of Business Analytics Overview of Business Analytics Applications: Financial Analytics - Marketing Analytics - HRAnalytics - Supply Chain Analytics - Retail Industry - Sales Analytics - Web & Social MediaAnalytics-HealthcareAnalytics-EnergyAnalytics-TransportationAnalytics-LendingAnalytics -SportsAnalytics-Futureof Business Analytics. Unit-V:Ethical, Legal and Organizational Issues Issues&Challenges:BusinessAnalyticsImplementationChallenges-PrivacyandAnonymizaiton- HackingandInsider Threats - MakingCustomer Comfortable. REFERENCES: JamesREvans,BusinessAnalytics,GlobalEdition,PearsonEducation UDineshKumar, BusinessAnalytics, WileyIndiaPvt.Ltd.,NewDelhi GerKoole,AnIntroductiontoBusinessAnalytics,Lulu.com,2019 J.D.Camm,J.J.Cochran,M.J.Fry,J.W.Ohlmann,D.R.Anderson,D.J.Sweeney,T.A.Williams- Essentials ofBusiness Analytics,2e;Cengage Learning. VipinKumar,IntroductiontoDataMining,Pang- NingTan,MichaelSteinbach,PearsonEducationIndia BhimasankaramPochiraju,SridharSeshadri,EssentialsofBusinessAnalytics:AnIntroductiont othe Methodology anditsApplication, Springer UNIT 1 Understanding Business Analytics Introduction – Meaning of Analytics-Evolution of Analytics-Need of Analytics- Business Analytics vs. Business Analytics – Categorization of Analytical Models – Data Scientist vs. Data Engineer vs. Business Analyst – Business Analytics in practice- Types of Data- Role of Business Analyst. Introduction The word analytics has come into the foreground in last decade or so. The increase of the internet and information technology has made analytics very relevant in the current age. Analytics is a field which combines data, information technology, statistical analysis, quantitative methods and computer-based models into one. This all are combined to provide decision makers all the possible scenarios to make a well thought and researched decision. The computer-based model ensures that decision makers are able to see performance of decision under various scenarios. Meaning Business analytics (BA) is a set of disciplines and technologies for solving business problems using data analysis, statistical models and other quantitative methods. It involves an iterative, methodical exploration of an organization's data, with an emphasis on statistical analysis, to drive decision-making. At its core, business analytics involves a combination of the following: identifying new patterns and relationships with data mining; using quantitative and statistical analysis to design business models; conducting A/B and multi-variable testing based on findings; forecasting future business needs, performance, and industry trends with predictive modelling; and Communicating your findings in easy-to-digest reports to colleagues, management, and customers. Definition Business analytics (BA) refers to the skills, technologies, and practices for continuous iterative exploration and investigation of past business performance to gain insight and drive business planning. Business analytics focuses on developing new insights and understanding of business performance based on data and statistical methods. Business Analytics is the process of transforming data into insights to improve business decisions. Data management, data visualization, predictive modelling, data mining, forecasting simulation, and optimization are some of the tools used to create insights from data. Evolution of Business Analytics Business analytics has been existence since very long time and has evolved with availability of newer and better technologies. It has its roots in operations research, which was extensively used during World War II. Operations research was an analytical way to look at data to conduct military operations. Over a period of time, this technique started getting utilized for business. Here operation’s research evolved into management science. Again, basis for management science remained same as operation research in data, decision making models, etc. Analytics have been used in business since the management exercises were put into place by Frederick Winslow Taylor in the late 19th century. Henry Ford measured the time of each component in his newly established assembly line. But analytics began to command more attention in the late 1960s when computers were used in decision support systems. Since then, analytics have changed and formed with the development of enterprise resource planning (ERP) systems, data warehouses, and a large number of other software tools and processes. In later years the business analytics have exploded with the introduction of computers. This change has brought analytics to a whole new level and has brought about endless possibilities. As far as analytics has come in history, and what the current field of analytics is today, many people would never think that analytics started in the early 1900s with Mr. Ford himself. As the economies started developing and companies became more and more competitive, management science evolved into business intelligence, decision support systems and into PC software. Scope of Business Analytics Business analytics has a wide range of application and usages. It can be used for descriptive analysis in which data is utilized to understand past and present situation. This kind of descriptive analysis is used to asses’ current market position of the company and effectiveness of previous business decision. It is used for predictive analysis, which is typical used to asses’ previous business performance. Business analytics is also used for prescriptive analysis, which is utilized to formulate optimization techniques for stronger business performance. For example, business analytics is used to determine pricing of various products in a departmental store based past and present set of information. How business analytics works Before any data analysis takes place, BA starts with several foundational processes: Determine the business goal of the analysis. Select an analysis methodology. Get business data to support the analysis, often from various systems and sources. Cleanse and integrate data into a single repository, such as a data warehouse or data mart. Need/Importance of Business Analytics Business analytics is a methodology or tool to make a sound commercial decision. Hence it impacts functioning of the whole organization. Therefore, business analytics can help improve profitability of the business, increase market share and revenue and provide better return to a shareholder. Facilitates better understanding of available primary and secondary data, which again affect operational efficiency of several departments. Provides a competitive advantage to companies. In this digital age flow of information is almost equal to all the players. It is how this information is utilized makes the company competitive. Business analytics combines available data with various well thought models to improve business decisions. Converts available data into valuable information. This information can be presented in any required format, comfortable to the decision maker. For starters, business analytics is the tool your company needs to make accurate decisions. These decisions are likely to impact your entire organization as they help you to improve profitability, increase market share, and provide a greater return to potential shareholders. While some companies are unsure what to do with large amounts of data, business analytics works to combine this data with actionable insights to improve the decisions you make as a company Essentially, the four main ways business analytics is important, no matter the industry, are: Improves performance by giving your business a clear picture of what is and isn’t working Provides faster and more accurate decisions Minimizes risks as it helps a business make the right choices regarding consumer behaviour, trends, and performance Inspires change and innovation by answering questions about the consumer. Essentials of business analytics Business analytics has many use cases, but when it comes to commercial organizations, BA is typically used to: Analyze data from a variety of sources. This could be anything from cloud applications to marketing automation tools and CRM software. Use advanced analytics and statistics to find patterns within datasets. These patterns can help you predict trends in the future and access new insights about the consumer and their behaviour. Monitor KPIs and trends as they change in real-time. This makes it easy for businesses to not only have their data in one place but to also come to conclusions quickly and accurately. Support decisions based on the most current information. With BA providing such a vast amount of data that you can use to back up your decisions, you can be sure that you are fully informed for not one, but several different scenarios. Data for Analytics Business analytics uses data from three sources for construction of the business model. It uses business data such as annual reports, financial ratios, marketing research, etc. It uses the database which contains various computer files and information coming from data analysis. Benefits of implementing BA in your organization Apart from having applications in various arenas, following are the benefits of Business Analytics and its impact on business – Accurately transferring information Consequent improvement in efficiency Help portray Future Challenges Make Strategic decisions As a perfect blend of data science and analytics Reduction in Costs Improved Decisions Share information with a larger audience Ease in Sharing information with stakeholders Challenges Moreover, any technology is subject to its own set of problems and challenges. Following are the challenges in implementing business analytics in an organization. Lack of technical skills in employees Fuss over acceptance of BA by staff Data Security and Maintenance Integrity of Data Delivering relevant information in the given time Inability to address complex issues Costs involved in implementing BA Investment of staff time in implementation of BA Lack of a proper strategy to implement BA Business analytics can be possible only on large volume of data. It is sometime difficult obtain large volume of data and not question its integrity. Business analytics depends on sufficient volumes of high-quality data. The difficulty in ensuring data quality is integrating and reconciling data across different systems, and then deciding what subsets of data to make available. Previously, analytics was considered a type of after-the-fact method of forecasting consumer behaviour by examining the number of units sold in the last quarter or the last year. This type of data warehousing required a lot more storage space than it did speed. Now business analytics is becoming a tool that can influence the outcome of customer interactions. When a specific customer type is considering a purchase, an analytics- enabled enterprise can modify the sales pitch to appeal to that consumer. This means the storage space for all that data must react extremely fast to provide the necessary data in real-time. Application Business analytics has a wide range of application from customer relationship management, financial management, and marketing, supply-chain management, human- resource management, pricing and even in sports through team game strategies. In healthcare, business analysis can be used to operate and manage clinical information systems. It can transform medical data from a bewildering array of analytical methods into useful information. Data analysis can also be used to generate contemporary reporting systems which include the patient's latest key indicators, historical trends and reference values. Decision analytics: supports human decisions with visual analytics that the user models to reflect reasoning. Descriptive analytics: gains insight from historical data with reporting, scorecards, clustering etc. Predictive analytics: employs predictive modelling using statistical and machine learning techniques Prescriptive analytics: recommends decisions using optimization, simulation, etc. Behavioural analytics Cohort analysis Competitor analysis Cyber analytics Enterprise optimization Financial services analytics Fraud analytics Health care analytics Key Performance Indicators (KPI's) Marketing analytics Pricing analytics Retail sales analytics Risk & Credit analytics Supply chain analytics Talent analytics Telecommunications Transportation analytics Customer Journey Analytics Market Basket Analysis Business Analysis vs. Business Analytics The aim of business analytics is data and reporting—examining past business performance and forecasting future business performance. On the other hand, the business analysis focuses on functions and processes—determining business requirements and suggesting solutions. Business Analysis: Definition and Activities Business analysis is the practice of assisting firms in resolving their technical difficulties by understanding, defining, and solving those issues. The activities that are carried out while performing Business Analysis: Company analysis: Business analysis aims at figuring out the requirements of a firm in general and its strategic direction and determining the initiatives that will enable the business to address those strategic goals. Requirements planning and management: It focuses on planning the requirements of the development process, identifying what the top priority is for execution, and managing the changes. Requirements elicitation: It outlines techniques for collecting needs from relevant members of the project team. Requirements analysis and documentation: It explains how to establish and define the needs in detail to allow them to be effectively carried out by the team. Requirements communication: Business analysis explains methods to help stakeholders have a shared understanding of the needs and how they will be carried out. Solution assessment and validation: It also explains how a business analyst can execute a suggested solution, how to support the execution of a solution, and how to evaluate possible flaws in the implementation. Business analysis is performed by Functional Analysts, Systems Analysts, Business Analysts, and Business Requirements Analysts. Business Analytics: Definition and Its Applications Business analytics is also known as data analytics. It is a process of collecting, evaluating, and drawing valuable outcomes from the enormous amount of data available. Business analytics is widely used in the following applications: Finance Marketing HR CRM Manufacturing Banking and Credit Cards Business analytics is performed by Data Scientists and Data Analysts. Business Analysis vs. Business Analytics Most people believe that business analysis and analytics are the same, but they are not! The primary differences between business analysis and business analytics: Business Analysis It mainly aims at the methods and determining the business needs. It is employed to figure out the organizational needs and possible problems to have productive outcomes. Here, the tasks are carried out by Functional Analysts, Systems Analysts, and Business Analysts. Business, functional, and domain skills are needed to perform business analysis. The architectural domains for business analysis include enterprise architecture, process architecture, technology architecture, and organization architecture. Business Analytics It aims at data and reporting. It is widely practiced to reckon further stats and make decisions to bring improvements in the business. Here, the tasks are carried out by Data Scientists and Data Analysts. Mathematical, statistical, and programming skills are needed for executing business analytics. The architectural domains for business analytics include data architecture, technology architecture, and information architecture. Business Analysis vs. Analytics: Similarities Explained Business analysis and business analytics have some commonalities. They both: Examine and enhance businesses Determine solutions to issues Establish things based on the requirements Business analysis is a practice of identifying business requirements and figuring out solutions to specific business problems. This has a heavy overlap with the analysis of business needs to function normally and to enhance how they function. Sometimes, the solutions include a system’s development feature. It can also incorporate business change, process enhancement or strategic planning, and policy improvement. On the contrary, business analytics is all about the group of tools, techniques, and skills that help the investigation of previous business performance. It also aids to gain insights into future performance. In general, business analytics aims mostly at data and statistical analysis. Categorization of Analytical Models 4 Types of Business Analytics There are mainly four types of Business Analytics, each of these types are increasingly complex. They allow us to be closer to achieving real-time and future situation insight application. Each of these types of business analytics have been discussed below. 1. Descriptive Analytics 2. Diagnostic Analytics 3. Predictive Analytics 4. Prescriptive Analytics 1. Descriptive Analytics It summarizes an organisation’s existing data to understand what has happened in the past or is happening currently. Descriptive Analytics is the simplest form of analytics as it employs data aggregation and mining techniques. It makes data more accessible to members of an organisation such as the investors, shareholders, marketing executives, and sales managers. It can help identify strengths and weaknesses and provides an insight into customer behaviour too. This helps in forming strategies that can be developed in the area of targeted marketing. 2. Diagnostic Analytics This type of Analytics helps shift focus from past performance to the current events and determine which factors are influencing trends. To uncover the root cause of events, techniques such as data discovery, data mining and drill-down are employed. Diagnostic analytics makes use of probabilities, and likelihoods to understand why events may occur. Techniques such as sensitivity analysis and training algorithms are employed for classification and regression. 3. Predictive Analytics This type of Analytics is used to forecast the possibility of a future event with the help of statistical models and ML techniques. It builds on the result of descriptive analytics to devise models to extrapolate the likelihood of items. To run predictive analysis, Machine Learning experts are employed. They can achieve a higher level of accuracy than by business intelligence alone. One of the most common applications is sentiment analysis. Here, existing data collected from social media and is used to provide a comprehensive picture of an users opinion. This data is analysed to predict their sentiment (positive, neutral or negative). 4. Prescriptive Analytics Going a step beyond predictive analytics, it provides recommendations for the next best action to be taken. It suggests all favourable outcomes according to a specific course of action and also recommends the specific actions needed to deliver the most desired result. It mainly relies on two things, a strong feedback system and a constant iterative analysis. It learns the relation between actions and their outcomes. One common use of this type of analytics is to create recommendation systems. Business Analytics Tools Business Analytics tools help analysts to perform the tasks at hand and generate reports which may be easy for a layman to understand. These tools can be obtained from open source platforms, and enable business analysts to manage their insights in a comprehensive manner. They tend to be flexible and user-friendly. Various business analytics tools and techniques like. Python is very flexible and can also be used in web scripting. It is mainly applied when there is a need for integrating the data analyzed with a web application or the statistics is to be used in a database production. The I Python Notebook facilitates and makes it easy to work with Python and data. One can share notebooks with other people without necessarily telling them to install anything which reduces code organizing overhead SAS The tool has a user-friendly GUI and can churn through terabytes of data with ease. It comes with an extensive documentation and tutorial base which can help early learners get started seamlessly. R is open source software and is completely free to use making it easier for individual professionals or students starting out to learn. Graphical capabilities or data visualization is the strongest forte of R with R having access to packages like GGPlot, RGIS, Lattice, and GGVIS among others which provide superior graphical competency. Tableau is the most popular and advanced data visualization tool in the market. Story-telling and presenting data insights in a comprehensive way has become one of the trademarks of a competent business analyst Tableau is a great platform to develop customized visualizations in no time, thanks to the drop and drag features. Python, R, SAS, Excel, and Tableau have all got their unique places when it comes to usage. Data Scientist vs. Data Engineer vs. Data Analyst 1. Data scientists use their advanced statistical skills to help improve the models the data engineers implement and to put proper statistical rigour on the data discovery and analysis the customer is asking for. Companies extract data to analyze and gain insights about various trends and practices. In order to do so, they employ specialized data scientists who possess knowledge of statistical tools and programming skills. Moreover, a data scientist possesses knowledge of machine learning algorithms. However, Data Science is not a singular field. It is a quantitative field that shares its background with math, statistics and computer programming. With the help of data science, industries are qualified to make careful data-driven decisions. These algorithms are responsible for predicting future events. Therefore, data science can be thought of as an ocean that includes all the data operations like data extraction, data processing, data analysis and data prediction to gain necessary insights. A Data Scientist is required to perform responsibilities – Performing data pre-processing that involves data transformation as well as data cleaning. Using various machine learning tools to forecast and classify patterns in the data. Increasing the performance and accuracy of machine learning algorithms through fine-tuning and further performance optimization. Understanding the requirements of the company and formulating questions that needs to be addressed. Using robust storytelling tools to communicate results with the team members. For becoming a Data Scientist, you must have the following key skills – Should be proficient with Math and Statistics. Should be able to handle structured & unstructured information. In-depth knowledge of tools like R, Python and SAS. Well versed in various machine learning algorithms. Have knowledge of SQL(Structured Query Language) and NoSQL(Non Structured Query Language or not only SQL) Must be familiar with Big Data tools. Some of the tools that are used by Data Scientist are Web Scraping Data Analytics Machine Learning Reporting 2. A Data Engineer is a person who specializes in preparing data for analytical usage. Data Engineering also involves the development of platforms and architectures for data processing. In other words, a data engineer develops the foundation for various data operations. A Data Engineer is responsible for designing the format for data scientists and analysts to work on. Data Engineers have to work with both structured and unstructured data. Therefore, they need expertise in SQL and NoSQL databases both. Data Engineers allow data scientists to carry out their data operations. Data Engineers have to deal with Big Data where they engage in numerous operations like data cleaning, management, transformation, data deduplication etc. A Data Engineer is more experienced with core programming concepts and algorithms. The role of a data engineer also follows closely to that of a software engineer. This is because a data engineer is assigned to develop platforms and architecture that utilize guidelines of software development. For example, developing a cloud infrastructure to facilitate real-time analysis of data requires various development principles. Therefore, building an interface API is one of the job responsibilities of a data engineer. Tools used by Data Engineers Some of the tools that are used by Data Engineers are – Hadoop Apache Spark Kubernetes Java Yarn A Data Engineer is supposed to have the following responsibilities – Development, construction, and maintenance of data architectures. Conducting testing on large scale data platforms. Handling error logs and building robust data pipelines. Ability to handle raw and unstructured data. Provide recommendations for data improvement, quality, and efficiency of data. Ensure and support the data architecture utilized by data scientists and analysts. Development of data processes for data modelling, mining, and data production. Following are the key skills required to become a data engineer – Knowledge of programming tools like Python and Java. Solid Understanding of Operating Systems. Ability to develop scalable ETL packages. Should be well versed in SQL as well as NoSQL technologies like Cassandra and MongoDB. He should possess knowledge of data warehouse and big data technologies like Hadoop, Hive, Pig, and Spark. Should possess creative and out of the box thinking. 3. A Data Analyst is responsible for taking actionable that affect the current scope of the company. A data engineer is responsible for developing a platform those data analysts and data scientists work on. And, a data scientist is responsible for unearthing future insights from existing data and helping companies to make data-driven decisions. A data analyst does not directly participate in the decision-making process; rather, he helps indirectly through providing static insights about company performance. A data engineer is not responsible for decision making. And, a data scientist participates in the active decision-making process that affects the course of the company. A data analyst uses static modelling techniques that summarize the data through descriptive analysis. On the other hand, a data engineer is responsible for the development and maintenance of data pipelines. A data scientist uses dynamic techniques like Machine learning to gain insights about the future. Knowledge of machine learning is not important for data analysts. However, this is mandatory for data scientists. A data engineer need not require the knowledge of machine learning but he is required to have the knowledge of core computing concepts like programming and algorithms to build robust data systems. A data analyst only has to deal with structured data. However, both data scientists and data engineers deal with unstructured data as well. Data analyst and data scientists are both required to be proficient in data visualization. However, this is not required in the case of a data engineer. Both data scientists and analysts need not have knowledge of application development and working of the APIs. However, this is the most essential requirement for a data engineer. A Data Analyst has following responsibilities - Analyzing the data through descriptive statistics. Using database query languages to retrieve and manipulate information. Perform data filtering, cleaning and early stage transformation. Communicating results with the team using data visualization. Work with the management team to understand business requirements. In order to become a Data Analyst, you must possess the following skills – Should possess the strong mathematical aptitude Should be well versed with Excel, Oracle, and SQL. Possession of problem-solving attitude. Proficient in the communication of results to the team. Should have a strong suite of analytical skills. Some of the tools that are used by Data Analyst are Talend :Talend is one of the most powerful data analytics tools available in the market and is developed in the eclipse graphical development environment.... Qlik Sense.... Apache Spark.... Power BI.... ThoughtSpot.... RapidMiner.... Tableau Business Analyst Business analysts use data to form business insights and recommend changes in businesses and other organizations. Business analysts can identify issues in virtually any part of an organization, including IT processes, organizational structures, or staff development. As businesses seek to increase efficiency and reduce costs, business analytics has become an important component of their operations. Let’s take a closer look at what business analysts do and what it takes to get a job in business analysis. Business analysts identify business areas that can be improved to increase efficiency and strengthen business processes. They often work closely with others throughout the business hierarchy to communicate their findings and help implement changes. Tasks and duties can include: Identifying and prioritizing the organization's functional and technical needs and requirements Using SQL and Excel to analyze large data sets Compiling charts, tables, and other elements of data visualization Creating financial models to support business decisions Understanding business strategies, goals, and requirements Planning enterprise architecture (the structure of a business) Forecasting, budgeting, and performing both variance analysis and financial analysis Business analyst skills The key skills business analysts need are: Technical skills: These skills include stakeholder management, data modeling and knowledge of IT. Analytical skills: Business analysts have to analyze large amounts of data and other business processes to form ideas and fix problems. Communication: These professionals must communicate their ideas in an expressive way that is easy for the receiver to understand. Problem-solving: It is a business analyst’s primary responsibility to come up with solutions to an organization’s problems. Research skills: Thorough research must be conducted about new processes and software to present results that are effective. Business analyst responsibilities Analyzing and evaluating the current business processes a company has and identifying areas of improvement Researching and reviewing up-to-date business processes and new IT advancements to make systems more modern Presenting ideas and findings in meetings Training and coaching staff members Creating initiatives depending on the business’s requirements and needs Developing projects and monitoring project performance Collaborating with users and stakeholders Working closely with senior management, partners, clients and technicians Types of Data Qualitative vs. Quantitative Data 1. Quantitative data Quantitative data seems to be the easiest to explain. It answers key questions such as “how many, “how much” and “how often”. Quantitative data can be expressed as a number or can be quantified. Simply put, it can be measured by numerical variables. Quantitative data are easily amenable to statistical manipulation and can be represented by a wide variety of statistical types of graphs and charts such as line, bar graph, scatter plot, and etc. Examples of quantitative data: Scores on tests and exams e.g. 85, 67, 90 and etc. The weight of a person or a subject. Your shoe size. The temperature in a room. 2. Qualitative data Qualitative data can’t be expressed as a number and can’t be measured. Qualitative data consist of words, pictures, and symbols, not numbers. Qualitative data is also called categorical data because the information can be sorted by category, not by number. Qualitative data can answer questions such as “how this has happened” or and “why this has happened”. Examples of qualitative data: Colors e.g. the color of the sea Your favorite holiday destination such as Hawaii, New Zealand and etc. Names as John, Patricia.. Ethnicity such as American Indian, Asian, etc. Nominal vs. Ordinal Data 3. Nominal data Nominal data is used just for labelling variables, without any type of quantitative value. The name ‘nominal’ comes from the Latin word “nomen” which means ‘name’. The nominal data just name a thing without applying it to order. Actually, the nominal data could just be called “labels.” Examples of Nominal Data: Gender (Women, Men) Hair color (Blonde, Brown, Brunette, Red, etc.) Marital status (Married, Single, Widowed) Ethnicity (Hispanic, Asian) Eye color is a nominal variable having a few categories (Blue, Green, Brown) and there is no way to order these categories from highest to lowest. 4. Ordinal data Ordinal data shows where a number is in order. This is the crucial difference from nominal types of data. Ordinal data is data which is placed into some kind of order by their position on a scale. Ordinal data may indicate superiority. However, you cannot do arithmetic with ordinal numbers because they only show sequence. Ordinal variables are considered as “in between” qualitative and quantitative variables. In other words, the ordinal data is qualitative data for which the values are ordered. In comparison with nominal data, the second one is qualitative data for which the values cannot be placed in an ordered. We can also assign numbers to ordinal data to show their relative position. But we cannot do math with those numbers. For example: “first, second, third…etc.” Examples of Ordinal Data: The first, second and third person in a competition. Letter grades: A, B, C, and etc. When a company asks a customer to rate the sales experience on a scale of 1-10. Economic status: low, medium and high. Discrete vs. Continuous Data In statistics, marketing research, and data science, many decisions depend on whether the basic data is discrete or continuous. 5. Discrete data Discrete data is a count that involves only integers. The discrete values cannot be subdivided into parts. For example, the number of children in a class is discrete data. You can count whole individuals. You can’t count 1.5 kids. To put in other words, discrete data can take only certain values. The data variables cannot be divided into smaller parts. It has a limited number of possible values e.g. days of the month. Examples of discrete data: The number of students in a class. The number of workers in a company. The number of home runs in a baseball game. The number of test questions you answered correctly 6. Continuous data Continuous data is information that could be meaningfully divided into finer levels. It can be measured on a scale or continuum and can have almost any numeric value. For example, you can measure your height at very precise scales — meters, centimeters, millimeters and etc. You can record continuous data at so many different measurements – width, temperature, time, and etc. This is where the key difference from discrete types of data lies. The continuous variables can take any value between two numbers. For example, between 50 and 72 inches, there are literally millions of possible heights: 52.04762 inches, 69.948376 inches and etc. A good great rule for defining if a data is continuous or discrete is that if the point of measurement can be reduced in half and still make sense, the data is continuous. Examples of continuous data: The amount of time required to complete a project. The height of children. The square footage of a two-bedroom house. The speed of cars. Conclusion All of the different types of data have a critical place in statistics, research, and data science. Data types work great together to help organizations and businesses from all industries build successful data-driven decision-making process. Working in the data management area and having a good range of data science skills involves a deep understanding of various types of data and when to apply them. ROLES OF A BUSINESS ANALYST 1. BA LEVELS There are four levels that a business analyst in an organization comprises of: Strategic management: This is the analysis level, where a business analyst evaluates and calculates the strategic where about if a company. This is one of the most critical levels because unless the evaluation is done on the point, none of the further steps can work appropriately. Analysis of business model: This level has to do with evaluating policies that are currently being employed by the company. This not only enables us to implement what’s new but also helps in checking the previous ones. Designing the process: Like an artist creates his imagination, business analysts do that with their skills. The step includes modelling the business processes, which comes out to be designing and modelling. Analysis of technology: Technical systems need a thorough analysis too. This is something that, if not taken care of, leads to severe consequences. The key business analyst roles and responsibilities: What does a business needs: As a business analyst, it is his key responsibility to understand what stakeholders need and pass these requirements to the developers, and also give on the developer’s expectations to the stakeholders. A business analyst’s skill for this responsibility is the communication skills that can impress everyone across. While he transfers the information, he is the one who needs to put these in such words that make a difference. This responsibility is no doubt tome taking because he needs to listen and execute, which might seem easy, but only a skilled professional can handle all this. Conducting meetings with developing team and stakeholders: Business analysts are supposed to coordinate with both stakeholders and the development team whenever a new feature or update is added to a project. This may vary from project to project. This facilitates the collection of client feedback and the resolution of issues encountered by the development team when implementing new features. The business analyst role is to understand and explain the new feature updates to clients and take feedback for further development. Based on client feedback, Business Analyst instructs the development team to make amendments or continue as is. At times, the client requests an additional feature be added to a project, and the BA must determine whether or not it is feasible, and then assign resources if necessary to implement it. System possibilities: A business analyst might be considered one among those working in the software team, but their key responsibility Is not what the team does. He has to ensure that he figures out what a project needs. He is the one who leads the path to the goals. He might be the one who dreams of targets, but he is also the one who knows how to make those dreams a reality. Looking for the opportunities and grabbing them before they go is what a business analyst is good at. Present the company: He can be called the face of a business. A business analyst is responsible for putting a business’s thoughts and goals in front of the stakeholders. In short, he is the one who needs to impress the stakeholders with his presentation skills and the skill to present what the person on the other side is looking for and not what the company has in store for them. Present the details: A project brings with itself hundreds of minute details that might be left unseen. A business analyst is the one who is responsible for elaborating the project with the tiniest of the loopholes or hidden secrets. This is considered the most crucial role of a business analyst because unless the details are put across the stakeholders, they won’t take an interest, and unless they show the part, the project is likely to take a pause. Implementation of the project: After going through all the steps mentioned above, the next and the most important role of a business analyst in agile is to implement whatever has been planned. Execution is not easy unless the previous steps have been taken care of in a systemized fashion. Functional and non-functional requirements of a business: As an organization, the main goal is to receive an end product that is productive and gives a company a long time. The role of business analyst in it company is to take care of the business’s functional aspect, which includes the steps and ways to ensure the working of the project. Sideways he is also supposed to take care of the non-functional that comprise how a project or a business is supposed to work. Testing: The role of a business analyst is way longer than expected. Once the product is prepared, the next step is to test it among the users to know it’s working capacity and quality. The Business Analyst tests the prototype/interface by involving some clients and recording their experiences with the model that has been developed, according to the role description. Based on their feedback, Business Analyst intends to make some changes to the model that will make it even better. They conduct UAT (user acceptance test) to determine whether or not the prototype meets the requirements of the project under consideration. Decision making and problem-solving: The responsibilities of business analyst range from developing the required documents to making decisions in the most stringent circumstances, job role of business analyst is to do it all. Moreover, a business analyst is expected to be the one who tackles things most easily and calmly because he should also be good at problem-solving, even if that’s related to the stakeholders, employees, or the clients. Maintenance: Like they say that care is as essential as building something new. No matter how much human resources, energy, or finds you spend on a project, if the maintenance part is not taken care of properly or is neglected, it tends to spoil the entire hard work put across. What is the role of a business analyst here? Is it just limited to the maintenance of the clients or sales; it also has to ensure that the quality and the promised products are maintained throughout. Building a team: Everyone is born with varied skills. As a business analyst, the business analyst’s responsibility is to make the team with people possessing different skills required for the project. Not only the hiring but retaining them is as essential. A well united and skilled team can do wonders. The things that are required in a great section inside co combination, structuring, and skills. A good team tends to take the company to the heights of success. Presentation and Documentation of the Final Project: After the business project is completed, the Business Analyst must document the details of the project and share the project’s findings with the client. In most cases, BA roles and responsibilities include preparing reports and presenting the results of a project to key stakeholders and clients. During building the project, they must also record all of the lessons learned and challenges they encountered in a concise form. This step aids the business analyst in making better decisions in the future. CONCLUSION A business analyst might be another position in an organization but its roles and responsibilities play a vital role in an organization’s success. While he needs to be a good orator, he should possess the quality of bringing people closers to his team and across. His roles are not limited to a specific step in project management. He is required one overstep till the end. From the initial stages of evaluation to the maintenance, a company needs a business analyst’s skill. UNIT-II Dealing with Data and Data Science Data: Data Collection-Data Management-Big Data Management-Organization/sources of Data- Importance of Data Quality- Dealing with missing or incomplete data – Data Visualization- Data Classification. Data Science project Life Cycle- Business Requirement – Data Acquisition- data Preparation- Hypothesis and Modelling- Evaluation and interpretation- Deployment- Operations-Optimization-Applications for Data Science. Data Knowledge is power, information is knowledge, and data is information in digitized form, at least as defined in IT. Hence, data is power. Data are individual facts, statistics, or items of information, often numeric. In a more technical sense, data are a set of values of qualitative or quantitative variables about one or more persons or objects Data is various kinds of information formatted in a particular way. Therefore, data collection is the process of gathering, measuring, and analyzing accurate data from a variety of relevant sources to find answers to research problems, answer questions, evaluate outcomes, and forecast trends and probabilities. Accurate data collection is necessary to make informed business decisions, ensure quality assurance, and keep research integrity. The concept of data collection isn’t a new one, as we’ll see later, but the world has changed. There is far more data available today, and it exists in forms that were unheard of a century ago. The data collection process has had to change and grow with the times, keeping pace with technology. Data collection breaks down into two methods: 1. Primary & 2. Secondary Data Collection Data collection is the process of acquiring, collecting, extracting, and storing the voluminous amount of data which may be in the structured or unstructured form like text, video, audio, XML files, records, or other image files used in later stages of data analysis. In the process of big data analysis, “Data collection” is the initial step before starting to analyze the patterns or useful information in data. The data which is to be analyzed must be collected from different valid sources. The actual data is then further divided mainly into two types known as: 1. Primary data 2. Secondary data 1. Primary data: The data which is Raw, original, and extracted directly from the official sources is known as primary data. This type of data is collected directly by performing techniques such as questionnaires, interviews, and surveys. The data collected must be according to the demand and requirements of the target audience on which analysis is performed otherwise it would be a burden in the data processing. Few methods of collecting primary data: Interview method: The data collected during this process is through interviewing the target audience by a person called interviewer and the person who answers the interview is known as the interviewee. Some basic business or product related questions are asked and noted down in the form of notes, audio, or video and this data is stored for processing. These can be both structured and unstructured like personal interviews or formal interviews through telephone, face to face, email, etc. Survey method: The survey method is the process of research where a list of relevant questions are asked and answers are noted down in the form of text, audio, or video. The survey method can be obtained in both online and offline mode like through website forms and email. Then that survey answers are stored for analyzing data. Examples are online surveys or surveys through social media polls. Observation method: The observation method is a method of data collection in which the researcher keenly observes the behaviour and practices of the target audience using some data collecting tool and stores the observed data in the form of text, audio, video, or any raw formats. In this method, the data is collected directly by posting a few questions on the participants. For example, observing a group of customers and their behaviour towards the products. The data obtained will be sent for processing. Projective Technique Projective data gathering is an indirect interview, used when potential respondents know why they're being asked questions and hesitate to answer. For instance, someone may be reluctant to answer questions about their phone service if a cell phone carrier representative poses the questions. With projective data gathering, the interviewees get an incomplete question, and they must fill in the rest, using their opinions, feelings, and attitudes. Delphi Technique. The Oracle at Delphi, according to Greek mythology, was the high priestess of Apollo’s temple, who gave advice, prophecies, and counsel. In the realm of data collection, researchers use the Delphi technique by gathering information from a panel of experts. Each expert answers questions in their field of specialty, and the replies are consolidated into a single opinion. Focus Groups. Focus groups, like interviews, are a commonly used technique. The group consists of anywhere from a half-dozen to a dozen people, led by a moderator, brought together to discuss the issue. Questionnaires. Questionnaires are a simple, straightforward data collection method. Respondents get a series of questions, either open or close-ended, related to the matter at hand. Experimental method: The experimental method is the process of collecting data through performing experiments, research, and investigation. The most frequently used experiment methods are CRD, RBD, LSD, FD. CRD- Completely Randomized design is a simple experimental design used in data analytics which is based on randomization and replication. It is mostly used for comparing the experiments. RBD- Randomized Block Design is an experimental design in which the experiment is divided into small units called blocks. Random experiments are performed on each of the blocks and results are drawn using a technique known as analysis of variance (ANOVA). RBD was originated from the agriculture sector. LSD – Latin Square Design is an experimental design that is similar to CRD and RBD blocks but contains rows and columns. It is an arrangement of NxN squares with an equal amount of rows and columns which contain letters that occurs only once in a row. Hence the differences can be easily found with fewer errors in the experiment. Sudoku puzzle is an example of a Latin square design. FD- Factorial design is an experimental design where each experiment has two factors each with possible values and on performing trail other combinational factors are derived. 2. Secondary data: Secondary data is the data which has already been collected and reused again for some valid purpose. This type of data is previously recorded from primary data and it has two types of sources named internal source and external source. i. Internal source: These types of data can easily be found within the organization such as market record, a sales record, transactions, customer data, accounting resources, etc. The cost and time consumption is less in obtaining internal sources. Financial Statements Sales Reports Retailer/Distributor/Deal Feedback Customer Personal Information (e.g., name, address, age, contact info) Business Journals Government Records (e.g., census, tax records, Social Security info) Trade/Business Magazines The internet ii. External source: The data which can’t be found at internal organizations and can be gained through external third party resources is external source data. The cost and time consumption is more because this contains a huge amount of data. Examples of external sources are Government publications, news publications, Registrar General of India, planning commission, international labour bureau, syndicate services, and other non-governmental publications. iii. Other sources: Sensors data: With the advancement of IoT devices, the sensors of these devices collect data which can be used for sensor data analytics to track the performance and usage of products. Satellites data: Satellites collect a lot of images and data in terabytes on daily basis through surveillance cameras which can be used to collect useful information. Web traffic: Due to fast and cheap internet facilities many formats of data Which is uploaded by users on different platforms can be predicted and collected with their permission for data analysis. The search engines also provide their data through keywords and queries searched mostly. Data Collection Tools 1. Word Association. The researcher gives the respondent a set of words and asks them what comes to mind when they hear each word. 2. Sentence Completion. Researchers use sentence completion to understand what kind of ideas the respondent has. This tool involves giving an incomplete sentence and seeing how the interviewee finishes it. 3. Role-Playing. Respondents are presented with an imaginary situation and asked how they would act or react if it was real. 4. In-Person Surveys. The researcher asks questions in person. 5. Online/Web Surveys. These surveys are easy to accomplish, but some users may be unwilling to answer truthfully, if at all. 6. Mobile Surveys. These surveys take advantage of the increasing proliferation of mobile technology. Mobile collection surveys rely on mobile devices like tablets or smart phones to conduct surveys via SMS or mobile apps. 7. Phone Surveys. No researcher can call thousands of people at once, so they need a third party to handle the chore. However, many people have call screening and won’t answer. 8. Observation. Sometimes, the simplest method is the best. Researchers who make direct observations collect data quickly and easily, with little intrusion or third-party bias. Naturally, it’s only effective in small-scale situations. Data Management Data management refers to the professional practice of constructing and maintaining a framework for ingesting, storing, mining, and archiving the data integral to a modern business. Data management is the spine that connects all segments of the information lifecycle. Data management works symbiotically with process management, ensuring that the actions teams take are informed by the cleanest, most current data available — which in today’s world means tracking changes and trends in real-time. Below is a deeper look at the practice, its benefits and challenges, and best practices for helping your organization get the most out of its business intelligence. 7 types of data management Data management experts generally focus on specialties within the field. These specialties can fall under one or more of the following areas: 1. Master data management: Master data management (MDM) is the process of ensuring the organization is always working with — and making business decisions based on — a single version of current, reliable information. Ingesting data from all of your data sources and presenting it as one constant, reliable source, as well as repropagating data into different systems, requires the right tools. 2. Data stewardship: A data steward does not develop information management policies but rather deploys and enforces them across the enterprise. As the name implies, a data steward stands watch over enterprise data collection and movement policies, ensuring practices are implemented and rules are enforced. 3. Data quality management: If a data steward is a kind of digital sheriff, a data quality manager might be thought of as his court clerk. Quality management is responsible for combing through collected data for underlying problems like duplicate records, inconsistent versions, and more. Data quality managers support the defined data management system. 4. Data security: One of the most important aspects of data management today is security. Though emergent practices like DevSecOps incorporate security considerations at every level of application development and data exchange, security specialists are still tasked with encryption management, preventing unauthorized access, guarding against accidental movement or deletion, and other frontline concerns. 5. Data governance: Data governance sets the law for an enterprise’s state of information. A data governance framework is like a constitution that clearly outlines policies for the intake, flow, and protection of institutional information. Data governors oversee their network of stewards, quality management professionals, security teams, and other people and data management processes in pursuit of a governance policy that serves a master data management approach. 6. Big data management: Big data is the catch-all term used to describe gathering, analyzing, and using massive amounts of digital information to improve operations. In broad terms, this area of data management specializes in intake, integrity, and storage of the tide of raw data that other management teams use to improve operations and security or inform business intelligence. 7. Data warehousing: Information is the building block of modern business. The sheer volume of information presents an obvious challenge: What do we do with all these blocks? Data warehouse management provides and oversees the physical and/or cloud-based infrastructure used to aggregate raw data and analyze it in-depth to produce business insights. The unique needs of any organization practicing data management may require a blend of some or all of these approaches. Familiarity with management areas provides data managers with the background they need to build solutions customized for their environments. Benefits of data management systems Data management processes help organizations identify and resolve internal pain points to deliver a better customer experience. First, data management provides businesses with a way of measuring the amount of data in play. A myriad of interactions occur in the background of any business — between network infrastructure, software applications, APIs, security protocols, and much more — and each presents a potential glitch (or time bomb) to operations if something goes wrong. Data management gives managers a big-picture look at business processes, which helps with both perspective and planning. Once data is under management, it can be mined for informational gold: business intelligence. This helps business users across the organization in a variety of ways, including the following: Smart advertising that targets customers according to their interests and interactions Holistic security that safeguards critical information Alignment with relevant compliance standards, saving time and money Machine learning that grows more environmentally aware over time, powering automatic and continuous improvement Reduced operating expenses by restricting use to only the necessary storage and compute power required for optimal performance Data management challenges All these benefits don’t come without climbing some hills. The ever-growing, rolling landscape of information technology is constantly changing and data managers will encounter plenty of challenges along the way. There are four key data management challenges to anticipate: The amount of data can be (at least temporarily) overwhelming. It’s hard to overstate the volume of data that must come under management in a modern business, so, when developing systems and processes, be ready to think big. Really big. Specialized third- party services and apps for integrating big data or providing it as a platform are crucial allies. Many organizations silo data. The development team may work from one data set, the sales team from another, operations from another, and so on. A modern data management system relies on access to all this information to develop modern business intelligence. Re Real-time data platform services help stream and share clean information between teams from a single, trusted source. The journey from unstructured data to structured data can be steep. Data often pours into organizations in an unstructured way. Before it can be used to generate business intelligence, data preparation has to happen: Data must be organized, de- duplicated, and otherwise cleaned. Data managers often rely on third-party partnerships to assist with these processes, using tools designed for on-premises, cloud, or hybrid environments. Managing the culture is essential to managing data. All of the processes and systems in the world won’t do you much good if people don’t know how — and perhaps just as importantly, why — to use them. By making team members aware of the benefits of data management (and the potential pitfalls of ignoring it) and fostering the skills of using data correctly, managers engage team members as essential pieces of the information process. These and other challenges stand between the old way of doing business and initiatives that harness the power of data for business intelligence. But with proper planning, practices, and partners, technologies like accelerated machine learning can turn pinch points into gateways for deeper business insights and better customer experience. Data management best practices Though specific data needs are unique to every organization’s data strategy and data systems, preparing a framework will smooth the path to easier, more effective data management solutions. Best practices like the three below are key to a successful strategy. 1. Make a plan 2. Store your data 3. Share your data 1. Make a plan Develop and write a data management plan (DMP). This document charts estimated data usage, accessibility guidelines, archiving approaches, ownership, and more. A DMP serves as both a reference and a living record and will be revised as circumstances change. Additionally, DMPs present the organization’s overarching strategy for data management to investors, auditors, and other involved parties — which is an important insight into a company’s preparedness for the rigors of the modern market. The best DMPs define granular details, including: Preferred file formats Naming conventions Access parameters for various stakeholders Backup and archiving processes Defined partners and the terms and services they provide Thorough documentation There are online services that can help create DMPs by providing step-by-step guidance to creating plans from templates. 2. Store your data Among the granular details mentioned above, a solid data storage approach is central to good data management. It begins by determining if your storage needs best suit a data warehouse or a data lake (or both), and whether the company’s data belongs on- premises or in the cloud. Then outline a consistent, and consistently enforced, agreement for naming files, folders, directories, users, and more. This is a foundational piece of data management, as these parameters will determine how to store all future data, and inconsistencies will result in errors and incomplete intelligence. 1. Security and backups. Insecure data is dangerous, so security must be considered at every layer. Some organizations come under special regulatory burdens like HIPAA, CIPA, GDPR, and others, which add additional security requirements like periodic audits. When security fails, the backup plan can be the difference between business life and death. Traditional models called for three copies of all important data: the original, the locally stored copy, and a remote copy. But emerging cloud models include decentralized data duplication, with even more backup options available at an increasingly affordable cost for storage and transfer. 2. Documentation is key. If it’s important, document it. If the entire team splits the lottery and runs off to Jamaica, thorough, readable documentation outlining security and backup procedures will give the next team a fighting chance to pick up where they left off. Without it, knowledge resides exclusively with holders who may or may not be part of a long-term data management approach. Data storage needs to be able to change as fast as the technology demands, so any approach should be flexible and have a reasonable archiving approach to keep costs manageable. 3. Share your data After all the plans are laid for storing, securing, and documenting your data, you should begin the process of sharing it with the appropriate people. Here are some critical questions to answer before other people access potentially critical information: Who owns the data? Can it be copied? Has everyone contributing to the data consented to share it with others? Who can access it and at what times? Are there copyrights, corporate secrets, proprietary intellectual property, or other off- limits information in the data set? What else does the organization’s data reveal about itself? With those and other questions answered, it’s time to find a place and means of sharing the data. Once called a repository, this role is increasingly filled by software and infrastructure as service models that are fine-tuned for big data management. Big Data Management Big data consists of huge amounts of information that cannot be stored or processed using traditional data storage mechanisms or processing techniques. It generally consists of three different variations. i. Structured data (as its name suggests) has a well-defined structure and follows a consistent order. This kind of information is designed so that it can be easily accessed and used by a person or computer. Structured data is usually stored in the well- defined rows and columns of a table (such as a spreadsheet) and databases — particularly relational database management systems, or RDBMS. ii. Semi-structured data exhibits a few of the same properties as structured data, but for the most part, this kind of information has no definite structure and cannot conform to the formal rules of data models such as an RDBMS. iii. Unstructured data possesses no consistent structure across its various forms and does not obey conventional data models’ formal structural rules. In very few instances, it may have information related to date and time. Characteristics of Big Data Management In line with classical definitions of the concept, big data is generally associated with three core characteristics: 1. Volume: This trait refers to the immense amounts of information generated every second via social media, cell phones, cars, transactions, connected sensors, images, video, and text. In petabytes, terabytes, or even zettabytes, these volumes can only be managed by big data technologies. 2. Variety: To the existing landscape of transactional and demographic data such as phone numbers and addresses, information in the form of photographs, audio streams, video, and a host of other formats now contributes to a multiplicity of data types — about 80% of which are completely unstructured. 3. Velocity: Information is streaming into data repositories at a prodigious rate, and this characteristic alludes to the speed of data accumulation. It also refers to the speed with which big data can be processed and analyzed to extract the insights and patterns it contains. These days, that speed is often real-time. Beyond “the Three Vs,” current descriptions of big data management also include two other characteristics, namely: Veracity: This is the degree of reliability and truth that big data has to offer in terms of its relevance, cleanliness, and accuracy. Value: Since the primary aim of big data gathering and analysis is to discover insights that can inform decision-making and other processes, this characteristic explores the benefit or otherwise that information and analytics can ultimately produce. Big Data Management Services When it comes to technology, organizations have many different types of big data management solutions to choose from. Vendors offer a variety of standalone or multi- featured big data management tools, and many organizations use multiple tools. Some of the most common types of big data management capabilities include the following: Data cleansing: finding and fixing errors in data sets Data integration: combining data from two or more sources Data migration: moving data from one environment to another, such as moving data from in-house data centres to the cloud Data preparation: readying data to be using in analytics or other applications Data enrichment: improving the quality of data by adding new data sets, correcting small errors or extrapolating new information from raw data Data analytics: analysing data with a variety of algorithms in order to gain insights Data quality: making sure data is accurate and reliable Master data management (MDM) :linking critical enterprise data to one master set that serves as the single source of truth for the organization Data governance: ensuring the availability, usability, integrity and accuracy of data Extract transform load (ETL): moving data from an existing repository into a database or data warehouse. Organization/Sources of Data Data organization is the practice of categorizing and classifying data to make it more usable. Similar to a file folder, where we keep important documents, you’ll need to arrange your data in the most logical and orderly fashion, so you — and anyone else who accesses it — can easily find what they’re looking for. DATA IS BEING COLLECTED The big data includes information produced by humans and devices. Device-driven data is largely clean and organized, But of far greater interest is human-driven data that exist in various formats and need more exquisite tools for proper processing and management. The big data collection is focused on the following types of data: Network data. This type of data is gathered on all kinds of networks, including social media, information and technological networks, the Internet and mobile networks, etc. Real-time data. They are produced on online streaming media, such as YouTube, Twitch, Skype, or Netflix. Transactional data. They are gathered when a user makes an online purchase (information on the product, time of purchase, payment methods, etc.) Geographic data. Location data of everything, humans, vehicles, building, natural reserves, and other objects are continuously supplied with satellites. Natural language data. These data are gathered mostly from voice searches that can be made on different devices accessing the Internet. Time series data. This type of data is related to the observation of trends and phenomena taking place at this very moment and over a period of time, for instance, global temperatures, mortality rates, pollution levels, etc. Linked data. They are based on HTTP, RDF, SPARQL, and URIs web technologies and meant to enable semantic connections between various databases so that computers could read and perform semantic queries correctly. HOW IS BIG DATA COLLECTED? There are different ways of how to collect big data from users. These are the most popular ones. 1. Asking for it the majority of firms prefer asking users directly to share their personal information. They give these data when creating website accounts or buying online. The minimum information to be collected includes a username and an email address, but some profiles require more details. 2. Cookies and Web Beacons Cookies and web beacons are two widely used methods to gather the data on users, namely, what web pages they visit and when. They provide basic statistics about how a website is used. Cookies and web beacons in no way compromise your privacy but just serve to personalize your experience with one or another web source. 3. Email tracking Email trackers are meant to give more information on the user actions in the mailbox. In particular, an email tracker allows detecting when an email was opened. Both Google and Yahoo use this method to learn their users’ behavioural patterns and provide personalized advertising. Importance of Data Quality Data quality is defined as: “The degree to which data meets a company’s expectations of accuracy, validity, completeness, and consistency” By tracking data quality, a business can pinpoint potential issues harming quality, and ensure that shared data is fit to be used for a given purpose. When collected data fails to meet the company expectations of accuracy, validity, completeness, and consistency, it can have massive negative impacts on customer service, employee productivity, and key strategies. Quality data is key to making accurate, informed decisions. And while all data has some level of “quality,” a variety of characteristics and factors determines the degree of data quality (high-quality versus low-quality). Furthermore, different data quality characteristics will likely be more important to various stakeholders across the organization. A list of popular data quality characteristics and dimensions include: 1. Completeness: Completeness is defined as a measure of the percentage of data that is missing within a dataset. 2. Timeliness: Timeliness measures how up-to-date or antiquated the data is at any given moment. 3. Validity: Validity refers to information that fails to follow specific company formats, rules, or processes. 4. Integrity: Integrity of data refers to the level at which the information is reliable and trustworthy. 5. Uniqueness: Uniqueness is a data quality characteristic most often associated with customer profiles. 6. Consistency: It ensures that the source of the information collection is capturing the correct data based on the unique objectives of the department or company. Dealing with Missing or incomplete Data The concept of missing data is implied in the name: its data that is not captured for a variable for the observation in question. Missing data reduces the statistical power of the analysis, which can distort the validity of the results. Fortunately, there are proven techniques to deal with missing data. Imputation vs. Removing Data When dealing with missing data, data scientists can use two primary methods to solve the error: imputation or the removal of data. The imputation method develops reasonable guesses for missing data. It’s most useful when the percentage of missing data is low. If the portion of missing data is too high, the results lack natural variation that could result in an effective model. The other option is to remove data. When dealing with data that is missing at random, related data can be deleted to reduce bias. Removing data may not be the best option if there are not enough observations to result in a reliable analysis. In some situations, observation of specific events or factors may be required. Before deciding which approach to employ, data scientists must understand why the data is missing. Missing at Random (MAR) Missing at Random means the data is missing relative to the observed data. It is not related to the specific missing values. The data is not missing across all observations but only within sub-samples of the data. It is not known if the data should be there; instead, it is missing given the observed data. The missing data can be predicted based on the complete observed data. Missing Completely at Random (MCAR) In the MCAR situation, the data is missing across all observations regardless of the expected value or other variables. Data scientists can compare two sets of data, one with missing observations and one without. Using a t-test, if there is no difference between the two data sets, the data is characterized as MCAR. Data may be missing due to test design, failure in the observations or failure in recording observations. This type of data is seen as MCAR because the reasons for its absence are external and not related to the value of the observation. It is typically safe to remove MCAR data because the results will be unbiased. The test may not be as powerful, but the results will be reliable. Missing Not at Random (MNAR) The MNAR category applies when the missing data has a structure to it. In other words, there appear to be reasons the data is missing. In a survey, perhaps a specific group of people – say women ages 45 to 55 – did not answer a question. Like MAR, the data cannot be determined by the observed data, because the missing information is unknown. Data scientists must model the missing data to develop an unbiased estimate. Simply removing observations with missing data could result in a model with bias. Deletion There are two primary methods for deleting data when dealing with missing data: list wise and dropping variables. List wise In this method, all data for an observation that has one or more missing values are deleted. The analysis is run only on observations that have a complete set of data. If the data set is small, it may be the most efficient method to eliminate those cases from the analysis. However, in most cases, the data are not missing completely at random (MCAR). Deleting the instances with missing observations can result in biased parameters and estimates and reduce the statistical power of the analysis. Pair wise Pair wise deletion assumes data are missing completely at random (MCAR), but all the cases with data, even those with missing data, are used in the analysis. Pairwise deletion allows data scientists to use more of the data. However, the resulting statistics may vary because they are based on different data sets. The results may be impossible to duplicate with a complete set of data. Dropping Variables If data is missing for more than 60% of the observations, it may be wise to discard it if the variable is insignificant. Imputation When data is missing, it may make sense to delete data, as mentioned above. However, that may not be the most effective option. For example, if too much information is discarded, it may not be possible to complete a reliable analysis. Or there may be insufficient data to generate a reliable prediction for observations that have missing data. Instead of deletion, data scientists have multiple solutions to impute the value of missing data. Depending why the data are missing, imputation methods can deliver reasonably reliable results. These are examples of single imputation methods for replacing missing data. Mean, Median and Mode This is one of the most common methods of imputing values when dealing with missing data. In cases where there are a small number of missing observations, data scientists can calculate the mean or median of the existing observations. However, when there are many missing variables, mean or median results can result in a loss of variation in the data. This method does not use time-series characteristics or depend on the relationship between the variables. Time-Series Specific Methods Another option is to use time-series specific methods when appropriate to impute data. There are four types of time-series data: No trend or seasonality. Trend, but no seasonality. Seasonality, but no trend. Both trend and seasonality. The time series methods of imputation assume the adjacent observations will be like the missing data. These methods work well when that assumption is valid. However, these methods won’t always produce reasonable results, particularly in the case of strong seasonality. Last Observation Carried Forward (LOCF) & Next Observation Carried Backward (NOCB) These options are used to analyze longitudinal repeated measures data, in which follow-up observations may be missing. In this method, every missing value is replaced with the last observed value. Longitudinal data track the same instance at different points along a timeline. This method is easy to understand and implement. However, this method may introduce bias when data has a visible trend. It assumes the value is unchanged by the missing data. Linear Interpolation Linear interpolation is often used to approximate a value of some function by using two known values of that function at other points. This formula can also be understood as a weighted average. The weights are inversely related to the distance from the end points to the unknown point. The closer point has more influence than the farther point. When dealing with missing data, you should use this method in a time series that exhibits a trend line, but it’s not appropriate for seasonal data. Seasonal Adjustment with Linear Interpolation When dealing with data that exhibits both trend and seasonality characteristics, use seasonal adjustment with linear interpolation. First you would perform the seasonal adjustment by computing a centered moving average or taking the average of multiple averages – say, two one-year averages – that are offset by one period relative to another. You can then complete data smoothing with linear interpolation as discussed above. Multiple Imputations Multiple imputations is considered a good approach for data sets with a large amount of missing data. Instead of substituting a single value for each missing data point, the missing values are exchanged for values that encompass the natural variability and uncertainty of the right values. Using the imputed data, the process is repeated to make multiple imputed data sets. Each set is then analyzed using the standard analytical procedures, and the multiple analysis results are combined to produce an overall result. The various imputations incorporate natural variability into the missing values, which creates a valid statistical inference. Multiple imputations can produce statistically valid results even when there is a small sample size or a large amount of missing data. K Nearest Neighbours In this method, data scientists choose a distance measure for k neighbours, and the average is used to impute an estimate. The data scientist must select the number of nearest neighbours and the distance metric. KNN can identify the most frequent value among the neighbours and the mean among the nearest neighbours. Data Visualization Data visualization is the practice of translating information into a visual context, such as a map or graph, to make data easier for the human brain to understand and pull insights from. The main goal of data visualization is to make it easier to identify patterns, trends and outliers in large data sets. The term is often used interchangeably with others, including information graphics, information visualization and statistical graphics. Data visualization is one of the steps of the data science process, which states that after data has been collected, processed and modelled, it must be visualized for conclusions to be made. Data visualization is also an element of the broader data presentation architecture (DPA) discipline, which aims to identify, locate, manipulate, format and deliver data in the most efficient way possible. Data visualization is important for almost every career. It can be used by teachers to display student test results, by computer scientists exploring advancements in artificial intelligence (AI) or by executives looking to share information with stakeholders. It also plays an important role in big data projects. As businesses accumulated massive collections of data during the early years of the big data trend, they needed a way to quickly and easily get an overview of their data. Visualization tools were a natural fit. Visualization is central to advanced analytics for similar reasons. When a data scientist is writing advanced predictive analytics or machine learning (ML) algorithms, it becomes important to visualize the outputs to monitor results and ensure that models are performing as intended. This is because visualizations of complex algorithms are generally easier to interpret than numerical outputs. Why is data visualization important? Data visualization provides a quick and effective way to communicate information in a universal manner using visual information. The practice can also help businesses identify which factors affect customer behaviour; pinpoint areas that need to be improved or need more attention; make data more memorable for stakeholders; understand when and where to place specific products; and predict sales volumes. Other benefits of data visualization include the following: the ability to absorb information quickly, improve insights and make faster decisions; an increased understanding of the next steps that must be taken to improve the organization; an improved ability to maintain the audience's interest with information they can understand; an easy distribution of information that increases the opportunity to share insights with everyone involved; eliminate the need for data scientists since data is more accessible and understandable; and An increased ability to act on findings quickly and, therefore, achieve success with greater speed and less mistakes. Data visualization and big data o The increased popularity of big data and data analysis projects has made visualization more important than ever. o Companies are increasingly using machine learning to gather massive amounts of data that can be difficult and slow to sort through, comprehend and explain. o Visualization offers a means to speed this up and present information to business owners and stakeholders in ways they can understand. o Big data visualization often goes beyond the typical techniques used in normal visualization, such as pie charts, histograms and corporate graphs. It instead uses more complex representations, such as heat maps and fever charts. o Big data visualization requires powerful computer systems to collect raw data, process it and turn it into graphical representations that humans can use to quickly draw insights. Examples of data visualization In the early days of visualization, the most common visualization technique was using a Microsoft Excel spreadsheet to transform the information into a table, bar graph or pie chart. While these visualization methods are still commonly used, more intricate techniques are now available, including the following: info graphics bubble clouds bullet graphs heat maps fever charts time series charts Some other popular techniques are as follows. Line charts. This is one of the most basic and common techniques used. Line charts display how variables can change over time. Area charts. This visualization method is a variation of a line chart; it displays multiple values in a time series -- or a sequence of data collected at consecutive, equally spaced points in time. Scatter plots. This technique displays the relationship between two variables. A scatter plot takes the form of an x- and y-axis with dots to represent data points. Tree maps. This method shows hierarchical data in a nested format. The size of the rectangles used for each category is proportional to its percentage of the whole. Treemaps are best used when multiple categories are present, and the goal is to compare different parts of a whole. Population pyramids. This technique uses a stacked bar graph to display the complex social narrative of a population. It is best used when trying to display the distribution of a population. Data Visualization Applications Common use cases for data visualization include the following: Sales and Marketing: Research from the media agency Magna predicts that half of all global advertising dollars will be spent online by 2020. As a result, marketing teams must pay close attention to their sources of web traffic and how their web properties generate revenue. Data visualization makes it easy to see traffic trends over time as a result of marketing efforts. Politics: A common use of data visualization in politics is a geographic map that displays the party each state or district voted for. Healthcare: Healthcare professionals frequently use choropleth maps to visualize important health data. A choropleth map displays divided geographical areas or regions that are assigned a certain color in relation to a numeric variable. Choropleth maps allow professionals to see how a variable, such as the mortality rate of heart disease, changes across specific territories. Scientists: Scientific visualization, sometimes referred to in shorthand as SciVis, allows scientists and researchers to gain greater insight from their experimental data than ever before. Finance: Finance professionals must track the performance of their investment decisions when choosing to buy or sell an asset. Candlestick charts are used as trading tools and help finance professionals analyze price movements over time, displaying important information, such as securities, derivatives, currencies, stocks, bonds and commodities. By analyzing how the price has changed over time, data analysts and finance professionals can detect trends. Logistics: Shipping companies can use visualization tools to determine the best global shipping routes. Data visualization tools and vendors Data visualization tools can be used in a variety of ways. The most common use today is as business intelligence (BI) reporting tool. Users can set up visualization tools to generate automatic dashboards that track company performance across key performance indicators (KPIs) and visually interpret the results. The generated images may also include interactive capabilities, enabling users to manipulate them or look more closely into the data for questioning and analysis. Indicators designed to alert users when data has been updated or when predefined conditions occur can also be integrated. Many business departments implement data visualization software to track their own initiatives. For example, a marketing team might implement the software to monitor the performance of an email campaign, tracking metrics like open rate, click-through rate and conversion rate. As data visua