Mental Models p2 PDF
Document Details
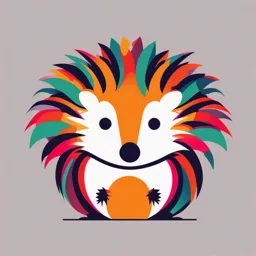
Uploaded by scrollinondubs
Stanford School of Medicine
Tags
Summary
This document discusses mental models and second-order thinking, highlighting the importance of considering the effects of one's actions beyond immediate consequences. It provides examples from history, exploring instances where failing to consider long-term implications led to unforeseen problems.
Full Transcript
Technology is fine, but the scientists and engineers only partially think through their problems. They solve certain aspects, but not the total, and as a consequence it is slapping us back in the face very hard. Barbara McClintock1 The People Who Appear in this Chapter Hardin,...
Technology is fine, but the scientists and engineers only partially think through their problems. They solve certain aspects, but not the total, and as a consequence it is slapping us back in the face very hard. Barbara McClintock1 The People Who Appear in this Chapter Hardin, Garrett. 1915-2003 - American ecologist and philosopher. The common thread in his work was an interest in bioethics. Cleopatra VII Philopator. 69-30 BCE - She was the last Ptolemy to rule Egypt and has been talked about ever since. The subject of numerous biographies, histories, plays, and movies, she is one of history’s true legends. Wollstonecraft, Mary. 1759-1797 - English writer and philosopher. She wrote a huge variety of works, from novels and histories to philosophy and children’s books. Her daughter Mary Shelley wrote Frankenstein. Second-Order Thinking Almost everyone can anticipate the immediate results of their actions. This type of first-order thinking is easy and safe but it’s also a way to ensure you get the same results that everyone else gets. Second-order thinking is thinking farther ahead and thinking holistically. It requires us to not only consider our actions and their immediate consequences, but the subsequent effects of those actions as well. Failing to consider the second- and third-order effects can unleash disaster. It is often easier to find examples of when second-order thinking didn’t happen—when people did not consider the effects of the effects. When they tried to do something good, or even just benign, and instead brought calamity, we can safely assume the negative outcomes weren’t factored into the original thinking. Very often, the second level of effects is not considered until it’s too late. This concept is often referred to as the “Law of Unintended Consequences” for this very reason. We see examples of this throughout history. During their colonial rule of India, the British government began to worry about the number of venomous cobras in Delhi. To reduce the numbers, they instituted a reward for every dead snake brought to officials. In response, Indian citizens dutifully complied and began breeding the snakes to slaughter and bring to officials. The snake problem was worse than when it started because the British officials didn’t think at the second level. Second-order effects occur even with something simple like adding traction on tires: it seems like such a great idea because the more you have the less likely you are to slide, the faster you can stop, and thus the safer you are. However, the second-order effects are that your engine has to work harder to propel the car, you get worse gas mileage (releasing more detrimental carbon dioxide into the atmosphere), and you leave more rubber particles on the road. This is why any comprehensive thought process considers the effects of the effects as seriously as possible. You are going to have to deal with them anyway. The genie never gets back in the bottle. You can never delete consequences to arrive at the original starting conditions. «Stupidity is the same as evil if you judge by the results.» Margaret Atwood 2 In an example of second-order thinking deficiency, we have been feeding antibiotics to livestock for decades to make the meat safer and cheaper. Only in recent years have we begun to realize that in doing so we have helped create bacteria that we cannot defend against. In 1963, the UC Santa Barbara ecologist and economist Garrett Hardin proposed his First Law of Ecology: “You can never merely do one thing.”3 We operate in a world of multiple, overlapping connections, like a web, with many significant, yet obscure and unpredictable, relationships. He developed second-order thinking into a tool, showing that if you don’t consider “the effects of the effects,” you can’t really claim to be doing any thinking at all. When it comes to the overuse of antibiotics in meat, the first-order consequence is that the animals gain more weight per pound of food consumed, and thus there is profit for the farmer. Animals are sold by weight, so the less food you have to use to bulk them up, the more money you will make when you go to sell them. The second-order effects, however, have many serious, negative consequences. The bacteria that survive this continued antibiotic exposure are antibiotic resistant. That means that the agricultural industry, when using these antibiotics as bulking agents, is allowing mass numbers of drug-resistant bacteria to become part of our food chain. High degrees of connections make second-order thinking all the more critical, because denser webs of relationships make it easier for actions to have far-reaching consequences. You may be focused in one direction, not recognizing that the consequences are rippling out all around you. Things are not produced and consumed in a vacuum. «When we try to pick out anything by itself, we find it hitched to everything else in the Universe.» John Muir4 Second-order thinking is not a way to predict the future. You are only able to think of the likely consequences based on the information available to you. However, this is not an excuse to power ahead and wait for post-facto scientific analysis. Could these consequences of putting antibiotics in the feed of all animals have been anticipated? Likely, yes, by anyone with even a limited understanding of biology. We know that organisms evolve. They adapt based on environmental pressures, and those with shorter life cycles can do it quite quickly because they have more opportunities. Antibiotics, by definition, kill bacteria. Bacteria, just like all other living things, want to survive. The pressures put on them by continued exposure to antibiotics increase their pace of evolution. Over the course of many generations, eventually mutations will occur that allow certain bacteria to resist the effects of the antibiotics. These are the ones that will reproduce more rapidly, creating the situation we are now in. — Sidebar: Second-Order Problem Second-order thinking teaches us two important concepts that underlie the use of this model. If we’re interested in understanding how the world really works, we must include second and subsequent effects. We must be as observant and honest as we can about the web of connections we are operating in. How often is short-term gain worth protracted long-term pain? Second-Order Problem Warren Buffett used a very apt metaphor once to describe how the second-order problem is best described by a crowd at a parade: Once a few people decide to stand on their tip-toes, everyone has to stand on their tip-toes. No one can see any better, but they’re all worse off. _ Buffett, Warren. “Letter to Shareholders, 1985.” BerkshireHathaway.com. Retrieved from: http:// www.berkshirehathaway.com/letters/1985.html Let’s look at two areas where second-order thinking can be used to great benefit: 1. Prioritizing long-term interests over immediate gains 2. Constructing effective arguments Second-order thinking and realizing long-term interests: This is a useful model for seeing past immediate gains to identify long-term effects we want. This is often a conflict for us, as when we choose to forgo the immediate pleasure of candy to improve our long-term health. The first-order effect is this amazing feeling triggered by pure sugar. But what are the second-order effects of regular candy consumption? Is this what I want my body or life to look like in ten years? Second- order thinking involves asking ourselves if what we are doing now is going to get us the results we want. Finding historical examples of second-order thinking can be tricky because we don’t want to evaluate based solely on the outcome: “It all turned out well, so he must have thought through the consequences of his actions.” Even if you can glimpse the long-term gain from your short-term pain, there is no guarantee you are going to get there. In 48 BC, Cleopatra of Egypt was in a terrible position.5 Technically co-regent with her brother, in a family famous for murdering siblings, she was encamped in a swampy desert, ousted from the palace, stuck with no solid plan for how to get back. She was Queen, but had made a series of unpopular decisions which left her with little support, and which gave her brother ample justification for trying to have her assassinated. What to do? At the same time the great Roman general Caesar arrived in Egypt, chasing down his enemy Pompey and making sure the Egyptians knew who really was in charge on the Mediterranean. Egypt was an incredibly fertile, wealthy country, and as such was of great importance to the Romans. The way they inserted themselves in Egypt, however, made them extremely unpopular there. In order to survive, Cleopatra had to make some decisions. Should she try to work things out with her brother? Should she try to marshal some support from another country? Or should she try to align herself with Caesar? In Cleopatra: A Life, Stacy Schiff explains that even in 48 BC at the age of 21, Cleopatra would have had a superb political education, based on both historical knowledge and firsthand exposure to the tumultuous events of life on the Mediterranean. She would have observed actions taken by her father, Auletes, as well as various family members, that resulted in exile, bribery, and murder from either the family, the Romans, or the populace. She would have known that there were no easy answers. As Schiff explains, “What Auletes passed down to his daughter was a precarious balancing act. To please one constituency was to displease another. Failure to comply with Rome would lead to intervention. Failure to stand up to Rome would lead to riots.” In this situation it was thus imperative that Cleopatra consider the second-order effects of her actions. Short-term gain might easily lead to execution (as indeed it already had for many of her relatives). If she wanted to be around for a while, she needed to balance her immediate goals of survival and the throne, with the future need for support to stay on it. In 48 BC Cleopatra chose to align herself with Caesar. It seems likely she would have known the first-order effects of this decision: Namely that it would anger her brother, who would increase his plotting to have her killed, and that it would anger the Egyptian people, who didn’t want a Roman involved in their affairs. She probably anticipated that there would be short-term pain, and there was. Cleopatra effectively started a civil war, with a siege on the palace that left her and Caesar trapped there for months. In addition, she had to be constantly vigilant against the assassination schemes of her brother. So why did she do it? Developing Trust for Future Success Trust and trustworthiness are the results of multiple interactions. This is why second-order thinking is so useful and valuable. Going for the immediate payoff in our interactions with people, unless they are a win-win, almost always guarantees that interaction will be a one-off. Maximizing benefits is something that happens over time. Thus, considering the effects of the effects of our actions on others, or on our reputations, is critical to getting people to trust us, and to enjoy the benefits of cooperation that come with that. In reality we will never know for sure. We can only make an educated guess. But given that Cleopatra ruled Egypt quite successfully for many years after these events, her decision was based on seeing the effects of the effects. If she could somehow make it through the short-term pain, then her leadership had much greater chances of being successful with the support of Caesar and Rome than without it. As Schiff notes, “The Alexandrian War gave Cleopatra everything she wanted. It cost her little.” In winning the civil war Caesar got rid of all major opposition to Cleopatra and firmly aligned himself with her reign. Being aware of second-order consequences and using them to guide your decision-making may mean the short term is less spectacular, but the payoffs for the long term can be enormous. By delaying gratification now, you will save time in the future. You won’t have to clean up the mess you made on account of not thinking through the effects of your short-term desires. — Sidebar: Developing Trust for Future Success Constructing an effective argument: Second-order thinking can help you avert problems and anticipate challenges that you can then address in advance. For example, most of us have to construct arguments every day. Convincing your boss to take a chance on a new form of outreach, convincing your spouse to try a new parenting technique. Life is filled with the need to be persuasive. Arguments are more effective when we demonstrate that we have considered the second-order effects and put effort into verifying that these are desirable as well. In late 18th-century England, women had very few rights. Philosopher Mary Wollstonecraft was frustrated that this lack of rights limited a woman’s ability to be independent and make choices on how to live her life. Instead of arguing, however, for why women should get rights, she recognized that she had to demonstrate the value that these rights would confer. She explained the benefits to society that would be realized as a result of those rights. She argued for the education of women because this would in turn make them better wives and mothers, more able to both support themselves and raise smart, conscientious children. Her thoughts, from A Vindication of the Rights of Woman, are a demonstration of second-order thinking: Asserting the rights which women in common with men ought to contend for, I have not attempted to extenuate their faults; but to prove them to be the natural consequence of their education and station in society. If so, it is reasonable to suppose that they will change their character, and correct their vices and follies, when they are allowed to be free in a physical, moral, and civil sense. 6 Empowering women was a first-order effect of recognizing that women had rights. But by discussing the logical consequences this would have on society, the second-order effects, she started a conversation that eventually resulted in what we now call feminism. Not only would women get freedoms they deserved, they would become better women, and better members of society. A word of caution Second-order thinking, as valuable as it is, must be tempered in one important way: You can’t let it lead to the paralysis of the Slippery Slope Effect, the idea that if we start with action A, everything after is a slippery slope down to hell, with a chain of consequences B, C, D, E, and F. Garrett Hardin smartly addresses this in Filters Against Folly: Those who take the wedge (Slippery Slope) argument with the utmost seriousness act as though they think human beings are completely devoid of practical judgment. Countless examples from everyday life show the pessimists are wrong…If we took the wedge argument seriously, we would pass a law forbidding all vehicles to travel at any speed greater than zero. That would be an easy way out of the moral problem. But we pass no such law.7 In practical life, everything has limits. Even if we consider second and subsequent effects, we can only go so far. During waves of Prohibition fever in the United States and elsewhere, conservative abstainers have frequently made the case that even taking the first drink would be the first step towards a life of sin. They’re right: It’s true that drinking a beer might lead you to become an alcoholic. But not most of the time. Thus we need to avoid the slippery slope and the analysis paralysis it can lead to. Second-order thinking needs to evaluate the most likely effects and their most likely consequences, checking our understanding of what the typical results of our actions will be. If we worried about all possible effects of effects of our actions, we would likely never do anything, and we’d be wrong. How you’ll balance the need for higher-order thinking with practical, limiting judgment must be taken on a case-by-case basis. Conclusion We don’t make decisions in a vacuum and we can’t get something for nothing. When making choices, considering consequences can help us avoid future problems. We must ask ourselves the critical question: And then what? Consequences come in many varieties, some more tangible than others. Thinking in terms of the system in which you are operating will allow you to see that your consequences have consequences. Thinking through a problem as far as you can with the information you have allows us to consider time, scale, thresholds, and more. And weighing different paths is what thinking is all about. A little time spent thinking ahead can save us massive amounts of time later. The theory of probability is the only mathematical tool available to help map the unknown and the uncontrollable. It is fortunate that this tool, while tricky, is extraordinarily powerful and convenient. Benoit Mandelbrot1 The People Who Appear in this Chapter Bayes, Thomas. 1702-1761 - English statistician and minister. The theorem which bears his name is a critical part of probability mathematics. He never published it himself, and so we have to thank his friend Richard Price for not losing this work to history. Atkins, Vera. 1908-2000 - Romanian-British intelligence officer. She received high honors from both the British and French governments for her work for the allies during World War II. Probabilistic Thinking Probabilistic thinking is essentially trying to estimate, using some tools of math and logic, the likelihood of any specific outcome coming to pass. It is one of the best tools we have to improve the accuracy of our decisions. In a world where each moment is determined by an infinitely complex set of factors, probabilistic thinking helps us identify the most likely outcomes. When we know these our decisions can be more precise and effective. Are you going to get hit by lightning or not? Why we need the concept of probabilities at all is worth thinking about. Things either are or are not, right? We either will get hit by lightning today or we won’t. The problem is, we just don’t know until we live out the day. Which doesn’t help us at all when we make our decisions in the morning. The future is far from determined and we can better navigate it by understanding the likelihood of events that could impact us. Our lack of perfect information about the world gives rise to all of probability theory, and its usefulness. We know now that the future is inherently unpredictable because not all variables can be known and even the smallest error imaginable in our data very quickly throws off our predictions. The best we can do is estimate the future by generating realistic, useful probabilities. So how do we do that? Probability is everywhere, down to the very bones of the world. The probabilistic machinery in our minds—the cut-to- the-quick heuristics made so famous by the psychologists Daniel Kahneman and Amos Tversky—was evolved by the human species in a time before computers, factories, traffic, middle managers, and the stock market. It served us in a time when human life was about survival, and still serves us well in that capacity.2 But what about today—a time when, for most of us, survival is not so much the issue? We want to thrive. We want to compete, and win. Mostly, we want to make good decisions in complex social systems that were not part of the world in which our brains evolved their (quite rational) heuristics. For this, we need to consciously add in a needed layer of probability awareness. What is it and how can I use it to my advantage? There are three important aspects of probability that we need to explain so you can integrate them into your thinking to get into the ballpark and improve your chances of catching the ball: 1. Bayesian thinking 2. Fat-tailed curves 3. Asymmetries Thomas Bayes and Bayesian thinking: Bayes was an English minister in the first half of the 18th century, whose most famous work, “An Essay Toward Solving a Problem in the Doctrine of Chances”, was brought to the attention of the Royal Society by his friend Richard Price in 1763—two years after his death. The essay concerned how we should adjust probabilities when we encounter new data, and provided the seeds for the great mathematician Pierre Simon Laplace to develop what we now call Bayes’s Theorem. The core of Bayesian thinking (or Bayesian updating, as it can be called) is this: given that we have limited but useful information about the world, and are constantly encountering new information, we should probably take into account what we already know when we learn something new. As much of it as possible. Bayesian thinking allows us to use all relevant prior information in making decisions. Statisticians might call it a base rate, taking in outside information about past situations like the one you’re in. Consider the headline “Violent Stabbings on the Rise.” Without Bayesian thinking, you might become genuinely afraid because your chances of being a victim of assault or murder is higher than it was a few months ago. But a Bayesian approach will have you putting this information into the context of what you already know about violent crime. You know that violent crime has been declining to its lowest rates in decades. Your city is safer now than it has been since this measurement started. Let’s say your chance of being a victim of a stabbing last year was one in 10,000, or 0.01%. The article states, with accuracy, that violent crime has doubled. It is now two in 10,000, or 0.02%. Is that worth being terribly worried about? The prior information here is key. When we factor it in, we realize that our safety has not really been compromised. Conversely, if we look at the diabetes statistics in the United States, our application of prior knowledge would lead us to a different conclusion. Here, a Bayesian analysis indicates you should be concerned. In 1958, 0.93% of the population was diagnosed with diabetes. In 2015 it was 7.4%. When you look at the intervening years, the climb in diabetes diagnosis is steady, not a spike. So the prior relevant data, or priors, indicate a trend that is worrisome. It is important to remember that priors themselves are probability estimates. For each bit of prior knowledge, you are not putting it in a binary structure, saying it is true or not. You’re assigning it a probability of being true. Therefore, you can’t let your priors get in the way of processing new knowledge. In Bayesian terms, this is called the likelihood ratio or the Bayes factor. Any new information you encounter that challenges a prior simply means that the probability of that prior being true may be reduced. Eventually some priors are replaced completely. This is an ongoing cycle of challenging and validating what you believe you know. When making uncertain decisions, it’s nearly always a mistake not to ask: What are the relevant priors? What might I already know that I can use to better understand the reality of the situation? — Sidebar: Conditional Probability Conditional Probability Conditional probability is similar to Bayesian thinking in practice, but comes at it from a different angle. When you use historical events to predict the future, you have to be mindful of the conditions that surrounded that event. Events can be independent, like tossing a coin, or dependent. In the latter case, it means the outcomes of an event are conditional on what preceded them. Let’s say the last three times I’ve hung out with you and we’ve gone for ice cream, I’ve picked vanilla. Do you conclude that vanilla is my favorite, and thus I will always choose it? You want to check first if my choosing vanilla is independent or dependent. Am I the first to choose from 100 flavors? Or am I further down the line, when chocolate is no longer available? My ice cream choice is independent if all the flavors are available each time someone in my group makes a choice. It is dependent if the preceding choices of my friends reduce what is available to me. In this case, the probability of my choosing vanilla is conditional on what is left after my friends make their choices. Thus, using conditional probability means being very careful to observe the conditions preceding an event you’d like to understand. Now we need to look at fat-tailed curves: Many of us are familiar with the bell curve, that nice, symmetrical wave that captures the relative frequency of so many things from height to exam scores. The bell curve is great because it’s easy to understand and easy to use. Its technical name is “normal distribution.” If we know we are in a bell curve situation, we can quickly identify our parameters and plan for the most likely outcomes. Fat-tailed curves are different. Take a look. At first glance they seem similar enough. Common outcomes cluster together, creating a wave. The difference is in the tails. In a bell curve the extremes are predictable. There can only be so much deviation from the mean. In a fat-tailed curve there is no real cap on extreme events. The more extreme events that are possible, the longer the tails of the curve get. Any one extreme event is still unlikely, but the sheer number of options means that we can’t rely on the most common outcomes as representing the average. The more extreme events that are possible, the higher the probability that one of them will occur. Crazy things are definitely going to happen, and we have no way of identifying when. — Sidebar: Orders of Magnitude Think of it this way. In a bell curve type of situation, like displaying the distribution of height or weight in a human population, there are outliers on the spectrum of possibility, but the outliers have a fairly well-defined scope. You’ll never meet a man who is ten times the size of an average man. But in a curve with fat tails, like wealth, the central tendency does not work the same way. You may regularly meet people who are ten, 100, or 10,000 times wealthier than the average person. That is a very different type of world. Let’s re-approach the example of the risks of violence we discussed in relation to Bayesian thinking. Suppose you hear that you had a greater risk of slipping on the stairs and cracking your head open than being killed by a terrorist. The statistics, the priors, seem to back it up: 1,000 people slipped on the stairs and died last year in your country and only 500 died of terrorism. Should you be more worried about stairs or terror events? _ Always be extra mindful of the tails: They might mean everything. Some use examples like these to prove that terror risk is low—since the recent past shows very few deaths, why worry?3 The problem is in the fat tails: The risk of terror violence is more like wealth, while stair-slipping deaths are more like height and weight. In the next ten years, how many events are possible? How fat is the tail? The important thing is not to sit down and imagine every possible scenario in the tail (by definition, it is impossible) but to deal with fat-tailed domains in the correct way: by positioning ourselves to survive or even benefit from the wildly unpredictable future, by being the only ones thinking correctly and planning for a world we don’t fully understand. — Sidebar: Anti-fragility Asymmetries: Finally, you need to think about something we might call “metaprobability”—the probability that your probability estimates themselves are any good. This massively misunderstood concept has to do with asymmetries. If you look at nicely polished stock pitches made by professional investors, nearly every time an idea is presented, the investor looks their audience in the eye and states they think they’re going to achieve a rate of return of 20% to 40% per annum, if not higher. Yet exceedingly few of them ever attain that mark, and it’s not because they don’t have any winners. It’s because they get so many so wrong. They consistently overestimate their confidence in their probabilistic estimates. (For reference, the general stock market has returned no more than 7% to 8% per annum in the United States over a long period, before fees.) Orders of Magnitude Nassim Taleb puts his finger in the right place when he points out our naive use of probabilities. In The Black Swan, he argues that any small error in measuring the risk of an extreme event can mean we’re not just slightly off, but way off—off by orders of magnitude, in fact. In other words, not just 10% wrong but ten times wrong, or 100 times wrong, or 1,000 times wrong. Something we thought could only happen every 1,000 years might be likely to happen in any given year! This is using false prior information and results in us underestimating the probability of the future distribution being different. _ Taleb, Nassim. The Black Swan: The Impact of the Highly Improbable, 2nd edition. New York: Random House, 2010. Anti-fragility How do we benefit from the uncertainty of a world we don’t understand, one dominated by “fat tails”? The answer to this was provided by Nassim Taleb in a book curiously titled Antifragile. Here is the core of the idea. We can think about three categories of objects: Ones that are harmed by volatility and unpredictability, ones that are neutral to volatility and unpredictability, and finally, ones that benefit from it. The latter category is antifragile—like a package that wants to be mishandled. Up to a point, certain things benefit from volatility, and that’s how we want to be. Why? Because the world is fundamentally unpredictable and volatile, and large events— panics, crashes, wars, bubbles, and so on—tend to have a disproportionate impact on outcomes. There are two ways to handle such a world: try to predict, or try to prepare. Prediction is tempting. For all of human history, seers and soothsayers have turned a comfortable trade. The problem is that nearly all studies of “expert” predictions in such complex real-world realms as the stock market, geopolitics, and global finance have proven again and again that, for the rare and impactful events in our world, predicting is impossible! It’s more efficient to prepare. What are some ways we can prepare—arm ourselves with antifragility—so we can benefit from the volatility of the world? The first one is what Wall Street traders would call “upside optionality”, that is, seeking out situations that we expect have good odds of offering us opportunities. Take the example of attending a cocktail party where a lot of people you might like to know are in attendance. While nothing is guaranteed to happen —you may not meet those people, and if you do, it may not go well— you give yourself the benefit of serendipity and randomness. The worst thing that can happen is...nothing. One thing you know for sure is that you’ll never meet them sitting at home. By going to the party, you improve your odds of encountering opportunity. The second thing we can do is to learn how to fail properly. Failing properly has two major components. First, never take a risk that will do you in completely. (Never get taken out of the game completely.) Second, develop the personal resilience to learn from your failures and start again. With these two rules, you can only fail temporarily. No one likes to fail. It hurts. But failure carries with it one huge antifragile gift: learning. Those who are not afraid to fail (properly) have a huge advantage over the rest. What they learn makes them less vulnerable to the volatility of the world. They benefit from it, in true antifragile fashion. Let’s say you’d like to start a successful business, but you have no business experience. Do you attend business school or start a business that might fail? Business school has its benefits, but business itself—the rough, jagged real-world experience of it—teaches through rapid feedback loops of success and failure. In other words, trial and error carries the precious commodity of information. The Antifragile mindset is a unique one. Whenever possible, try to create scenarios where randomness and uncertainty are your friends, not your enemies. _ Taleb, Nassim. Antifragile. New York: Random House, 2012. Another common asymmetry is people’s ability to estimate the effect of traffic on travel time. How often do you leave “on time” and arrive 20% early? Almost never? How often do you leave “on time” and arrive 20% late? All the time? Exactly. Your estimation errors are asymmetric, skewing in a single direction. This is often the case with probabilistic decision- making. Far more probability estimates are wrong on the “over- optimistic” side than the “under-optimistic” side. You’ll rarely read about an investor who aimed for 25% annual return rates who subsequently earned 40% over a long period of time. You can throw a dart at the Wall Street Journal and hit the names of lots of investors who aim for 25% per annum with each investment and end up closer to 10%. The spy world Successful spies are very good at probabilistic thinking. High- stakes survival situations tend to make us evaluate our environment with as little bias as possible. When Vera Atkins was second in command of the French unit of the Special Operations Executive (SOE), a British intelligence organization reporting directly to Winston Churchill during World War II4, she had to make hundreds of decisions by figuring out the probable accuracy of inherently unreliable information. Atkins was responsible for the recruitment and deployment of British agents into occupied France. She had to decide who could do the job, and where the best sources of intelligence were. These were literal life-and-death decisions, and all were based in probabilistic thinking. First, how do you choose a spy? Not everyone can go undercover in high stress situations and make the contacts necessary to gather intelligence. The result of failure in France in WWII was not getting fired; it was death. What factors of personality and experience show that a person is right for the job? Even today, with advancements in psychology, interrogation, and polygraphs, it’s still a judgment call. For Vera Atkins in the 1940s, it was very much a process of assigning weight to the various factors and coming up with a probabilistic assessment of who had a decent chance of success. Who spoke French? Who had the confidence? Who was too tied to family? Who had the problem-solving capabilities? From recruitment to deployment, her development of each spy was a series of continually updated, educated estimates. Getting an intelligence officer ready to go is only half the battle. Where do you send them? If your information was so great that you knew exactly where to go, you probably wouldn’t need an intelligence mission. Choosing a target is another exercise in probabilistic thinking. You need to evaluate the reliability of the information you have and the networks you have set up. Intelligence is not evidence. There is no chain of command or guarantee of authenticity. The stuff coming out of German-occupied France was at the level of grainy photographs, handwritten notes that passed through many hands on the way back to HQ, and unverifiable wireless messages sent quickly, sometimes sporadically, and with the operator under incredible stress. When deciding what to use, Atkins had to consider the relevancy, quality, and timeliness of the information she had. She also had to make decisions based not only on what had happened, but what possibly could. Trying to prepare for every eventuality means that spies would never leave home, but they must somehow prepare for a good deal of the unexpected. After all, their jobs are often executed in highly volatile, dynamic environments. The women and men Atkins sent over to France worked in three primary occupations: organizers were responsible for recruiting locals, developing the network, and identifying sabotage targets; couriers moved information all around the country, connecting people and networks to coordinate activities; and wireless operators had to set up heavy communications equipment, disguise it, get information out of the country, and be ready to move at a moment’s notice. All of these jobs were dangerous. The full scope of the threats was never completely identifiable. There were so many things that could go wrong, so many possibilities for discovery or betrayal, that it was impossible to plan for them all. The average life expectancy in France for one of Atkins’s wireless operators was six weeks. Finally, the numbers suggest an asymmetry in the estimation of the probability of success of each individual agent. Of the 400 agents that Atkins sent over to France, 100 were captured and killed. This is not meant to pass judgment on her skills or smarts. Probabilistic thinking can only get you in the ballpark. It doesn’t guarantee 100% success. There is no doubt that Atkins relied heavily on probabilistic thinking to guide her decisions in the challenging quest to disrupt German operations in France during World War II. It is hard to evaluate the success of an espionage career, because it is a job that comes with a lot of loss. Atkins was extremely successful in that her network conducted valuable sabotage to support the Allied cause during the war, but the loss of life was significant. Conclusion Successfully thinking in shades of probability means roughly identifying what matters, coming up with a sense of the odds, doing a check on our assumptions, and then making a decision. We can act with a higher level of certainty in complex, unpredictable situations. We can never know the future with exact precision. Probabilistic thinking is an extremely useful tool to evaluate how the world will most likely look so that we can effectively strategize. Insurance Companies The most probability-acute businesses in the world are insurance companies—because they must be. When we think of insurance, we might think of life insurance (the probability of a policyholder dying at a certain age), or auto insurance (the probability of being in a car accident), or maybe home insurance (the probability of a tree falling on the house). With the statistics available to us, the probabilities of these things are easy to price and predict across a large enough population. But insurance is deeply wide-ranging, and insurers will insure almost any event, for a price. Insurance policies have been taken out on Victoria’s Secret models’ legs, on baseball players’ arms, on the Pepsi Challenge and the NCAA tournament, and even on a famous country singer’s breasts! How is this possible? Only with a close attention to probability. What the great insurance companies in the world know how to do is pay attention to the important factors, even if they’re not totally predictable, and price accordingly. What is the probability of a Victoria’s Secret model injuring her legs badly enough to end her career? One in 10,000? One in 100,000? Getting it right would mean evaluating her lifestyle, her habits, her health, her family history—and coming up with a price and a set of conditions that are good enough to provide a profit on average. It’s not unlike handicapping a race at the horse tracks. You can always say yes to insuring, but the trick is to come up with the right price. And for that we need probability. Supporting Idea: Causation vs. Correlation Confusion between these two terms often leads to a lot of inaccurate assumptions about the way the world works. We notice two things happening at the same time (correlation) and mistakenly conclude that one causes the other (causation). We then often act upon that erroneous conclusion, making decisions that can have immense influence across our lives. The problem is, without a good understanding of what is meant by these terms, these decisions fail to capitalize on real dynamics in the world and instead are successful only by luck. No Correlation The correlation coefficient between two measures, which varies between -1 and 1, is a measure of the relative weight of the factors they share. For example, two phenomena with few factors shared, such as bottled water consumption versus suicide rate, should have a correlation coefficient of close to 0. That is to say, if we looked at all countries in the world and plotted suicide rates of a specific year against per capita consumption of bottled water, the plot would show no pattern at all. Perfect Correlation On the contrary, there are measures which are solely dependent on the same factor. A good example of this is temperature. The only factor governing temperature—velocity of molecules—is shared by all scales. Thus each degree in Celsius will have exactly one corresponding value in Fahrenheit. Therefore temperature in Celsius and Fahrenheit will have a correlation coefficient of 1 and the plot will be a straight line. Weak to Moderate Correlation There are few phenomena in human sciences that have a correlation coefficient of 1. There are, however, plenty where the association is weak to moderate and there is some explanatory power between the two phenomena. Consider the correlation between height and weight, which would land somewhere between 0 and 1. While virtually every three-year-old will be lighter and shorter than every grown man, not all grown men or three-year-olds of the same height will weigh the same. This variation and the corresponding lower degree of correlation implies that, while height is generally speaking a good predictor, there clearly are factors other than height at play. In addition, correlation can sometimes work in reverse. Let’s say you read a study that compares alcohol consumption rates in parents and their corresponding children’s academic success. The study shows a relationship between high alcohol consumption and low academic success. Is this a causation or a correlation? It might be tempting to conclude a causation, such as the more parents drink, the worse their kids do in school. However, this study has only demonstrated a relationship, not proved that one causes the other. The factors correlate— meaning that alcohol consumption in parents has an inverse relationship with academic success in children. It is entirely possible that having parents who consume a lot of alcohol leads to worse academic outcomes for their children. It is also possible, however, that the reverse is true, or even that having kids who do poorly in school causes parents to drink more. Trying to invert the relationship can help you sort through claims to determine if you are dealing with true causation or just correlation. Causation Whenever correlation is imperfect, extremes will soften over time. The best will always appear to get worse and the worst will appear to get better, regardless of any additional action. This is called regression to the mean, and it means we have to be extra careful when diagnosing causation. This is something that the general media and sometimes even trained scientists fail to recognize. Consider the example Daniel Kahneman gives in Thinking Fast and Slow:5 Depressed children treated with an energy drink improve significantly over a three-month period. I made up this newspaper headline, but the fact it reports is true: if you treated a group of depressed children for some time with an energy drink, they would show a clinically significant improvement. It is also the case that depressed children who spend some time standing on their head or hug a cat for twenty minutes a day will also show improvement. Whenever coming across such headlines it is very tempting to jump to the conclusion that energy drinks, standing on the head, or hugging cats are all perfectly viable cures for depression. These cases, however, once again embody the regression to the mean: Depressed children are an extreme group, they are more depressed than most other children—and extreme groups regress to the mean over time. The correlation between depression scores on successive occasions of testing is less than perfect, so there will be regression to the mean: depressed children will get somewhat better over time even if they hug no cats and drink no Red Bull. We often mistakenly attribute a specific policy or treatment as the cause of an effect, when the change in the extreme groups would have happened anyway. This presents a fundamental problem: how can we know if the effects are real or simply due to variability? Luckily there is a way to tell between a real improvement and something that would have happened anyway. That is the introduction of the so-called control group, which is expected to improve by regression alone. The aim of the research is to determine whether the treated group improves more than regression can explain. In real life situations with the performance of specific individuals or teams, where the only real benchmark is the past performance and no control group can be introduced, the effects of regression can be difficult if not impossible to disentangle. We can compare against industry average, peers in the cohort group or historical rates of improvement, but none of these are perfect measures. The test of a first-rate intelligence is the ability to hold two opposing ideas in mind at the same time and still retain the ability to function. One should, for example, be able to see that things are hopeless yet be determined to make them otherwise. F. Scott Fitzgerald1 The People Who Appear in this Chapter Jacobi, Carl. 1804-1851 - German mathematician who made fundamental contributions to elliptic functions, dynamics, and number theory. Bernays, Edward. 1891-1995 - Austrian-American. Known as “the Father of Public Relations”. Although his influence cannot be doubted, his legacy is one of brilliant but sometimes unethical strategies that consumers and citizens are still navigating today. Bogle, John. 1929 - American investor, business magnate, and philanthropist. He is the founder and retired chief executive of The Vanguard Group. Lewin, Kurt. 1890-1947 - German-American psychologist. Often recognized as the founder of social psychology, he was one of the first to study group dynamics and organizational development. Nightingale, Florence. 1820-1910 - English social reformer, statistician, and the founder of modern nursing. By turning nursing into a profession and collecting detailed statistics on hospital conditions, she improved the lives of people all over the world. Inversion Inversion is a powerful tool to improve your thinking because it helps you identify and remove obstacles to success. The root of inversion is “invert,” which means to upend or turn upside down. As a thinking tool it means approaching a situation from the opposite end of the natural starting point. Most of us tend to think one way about a problem: forward. Inversion allows us to flip the problem around and think backward. Sometimes it’s good to start at the beginning, but it can be more useful to start at the end. Think of it this way: Avoiding stupidity is easier than seeking brilliance. Combining the ability to think forward and backward allows you to see reality from multiple angles. There are two approaches to applying inversion in your life. 1. Start by assuming that what you’re trying to prove is either true or false, then show what else would have to be true. 2. Instead of aiming directly for your goal, think deeply about what you want to avoid and then see what options are left over. Set your assumptions: The 19th century German mathematician Carl Jacobi became famous for a number of reasons—including solving some ungodly difficult problems—but is perhaps best remembered for his advice to “invert, always invert.” Jacobi solved a range of problems by starting with the endpoint. When faced with proving an axiom in a difficult math problem, he might instead assume a property of the axiom was correct and then try to determine the consequences of this assumption. From that point, he could work out surprising, and at times counterintuitive, insights. — Sidebar: The Most Successful Detective of All Time Jacobi was not the first mathematician to use inversion. In fact, inversion is a staple of mathematical, philosophical, and scientific inquiry. We can look around today and appreciate that we can’t see atoms and quarks, but we know they exist because we can make predictions about their behavior and test those predictions. Or we can go back 2,300 years and look at the work of the Greek Hippasus, a follower of Pythagoras.2 (Yes, the one with the Theorem.) His attempts to derive the square root of 2, and his original direct approach to solving the problem (essentially, dividing larger and larger whole numbers into each other) were both fruitless and time consuming. He hit an impasse, realizing that he’d never be able to definitely solve the problem by thinking forward. In his increasing frustration, Hippasus decided to take the reverse route, thinking about what the square root of 2 might imply, and working backwards from there. If he couldn’t find it the way he had expected to, he’d start by proving what the number couldn’t be. His quest forever changed what we understood about mathematics, and led to the discovery of the first irrational number. Mathematics is not the only area where using inversion can produce surprising and non-intuitive results. In the 1920s the American Tobacco Company wanted to sell more of their Lucky Strike cigarettes to women. Men were smoking, but women weren’t. There were pervasive taboos against women smoking—it was seen as a man’s activity. Women therefore presented an untapped market that had the potential of providing huge revenue. The head of the company thought that they needed to convince women that smoking would make them thinner, riding on the slimness trend that had already begun, so he hired Edward Bernays, who came up with a truly revolutionary marketing campaign.3,4 In the style of the inversion approach described above, Bernays did not ask, “How do I sell more cigarettes to women?” Instead, he wondered, if women bought and smoked cigarettes, what else would have to be true? What would have to change in the world to make smoking desirable to women and socially acceptable? Then—a step farther—once he knew what needed to change, how would he achieve that? The Most Successful Detective of All Time The first great detective to capture the public imagination was Sherlock Holmes. He solved cases in ways that were unfathomable to others, yet seemed obvious in retrospect. He gave the appearance of being a magician, but really he was an excellent observer. He was also a master of inversion. In his third case, “A Scandal in Bohemia,”5 Holmes is hired by a king to recover a compromising photograph in which the king appears with an American opera singer, Irene Adler. The king is fearful that Adler will use the picture of the two of them to prevent his upcoming marriage or to blackmail him in the future. He does not want to live under this threat, and so hires Sherlock Holmes to retrieve the picture from Adler. Presented with this task, what does Holmes do? What would you do? Does he study Adler for months to determine where, based on her personality, she is likely to hide the picture? Does he break into her house and perform a systematic exploration of every nook and cranny? No. Instead, he inverts the problem. If it is true that Adler has this compromising picture of the king and is planning to blackmail him, what would also be true? Likely that she would greatly value the photo as it will bring her money, and that it would be hidden in an accessible location so she could retrieve it in a hurry. We tend to keep our most prized possessions where we can easily grab them in case of emergency. So Holmes contrives a scenario in which he is able to be in her house while Watson creates an illusion of a fire on the street outside. Believing the threat, Adler takes the photo out of its hiding place before escaping. In one instant Holmes both confirms the existence of the photo and now knows its whereabouts. By starting with the logical outcome of his assumptions and seeking to validate those, he advances his case with significantly more efficiency and accuracy than if he had searched first for proof of the assumptions themselves. To tackle the idea of smoking as a slimming aid, he mounted a large anti-sweets campaign. After dinner, it was about cigarettes, not dessert. Cigarettes were slimming, while desserts would ruin one’s figure. But Bernays’s real stroke of genius lay not just in coming out with adverts to convince women to stay slim by smoking cigarettes; “instead, he sought nothing less than to reshape American society and culture.”6 He solicited journalists and photographers to promote the virtues of being slim. He sought testimonials from doctors about the health value of smoking after a meal. He combined this approach with …altering the very environment, striving to create a world in which the cigarette was ubiquitous. He mounted a campaign to persuade hotels and restaurants to add cigarettes to dessert-list menus, and he provided such magazines as House and Garden with feature articles that included menus designed to preserve readers ‘from the dangers of overeating’…. The idea was not only to influence opinion but to remold life itself. Bernays approached designers, architects, and cabinetmakers in an effort to persuade them to design kitchen cabinets that included special compartments for cigarettes, and he spoke to the manufacturers of kitchen containers to add cigarette tins to their traditional lines of labeled containers for coffee, tea, sugar, and flour.7 The result was a complete shift in the consumption habits of American women. It wasn’t just about selling the cigarette, it was reorganizing society to make cigarettes an inescapable part of the American woman’s daily experience. Bernays’s efforts to make smoking in public socially acceptable had equally startling results. He linked cigarette smoking with women’s emancipation. To smoke was to be free. Cigarettes were marketed as “torches of freedom.” He orchestrated public events, including an infamous parade on Easter Sunday in 1929 which featured women smoking as they walked in the parade. He left no detail unattended, so public perception of smoking was changed almost overnight. He both normalized it and made it desirable in one swoop. Although the campaign utilized more principles than just inversion, it was the original decision to invert the approach that provided the framework from which the campaign was created and executed. Bernays didn’t focus on how to sell more cigarettes to women within the existing social structure. Sales would have undoubtedly been a lot more limited. Instead he thought about what the world would look like if women smoked often and anywhere, and then set about trying to make that world a reality. Once he did that, selling cigarettes to women was comparatively easy. This inversion approach became a staple of Bernays’s work. He used the descriptor “appeals of indirection”, and each time when “hired to sell a product or service, he instead sold whole new ways of behaving, which appeared obscure but over time reaped huge rewards for his clients and redefined the very texture of American life.”8 What are you trying to avoid? Instead of thinking through the achievement of a positive outcome, we could ask ourselves how we might achieve a terrible outcome, and let that guide our decision-making. Index funds are a great example of stock market inversion promoted and brought to bear by Vanguard’s John Bogle.9 Instead of asking how to beat the market, as so many before him, Bogle recognized the difficulty of the task. Everyone is trying to beat the market. No one is doing it with any consistency, and in the process real people are losing actual money. So he inverted the approach. The question then became, how can we help investors minimize losses to fees and poor money manager selection? The results were one of the greatest ideas—index funds—and one of the greatest powerhouse firms in the history of finance. The index fund operates on the idea that accruing wealth has a lot to do with minimizing loss. Think about your personal finances. Often we focus on positive goals, such as “I want to be rich,” and use this to guide our approach. We make investing and career choices based on our desire to accumulate wealth. We chase after magical solutions, like attempting to outsmart the stock market. These inevitably get us nowhere, and we have usually taken some terrible risks in the process which actually leave us worse off. Instead, we can try inverting the goal. It becomes, not getting rich, but avoiding being poor. Instead of trying to divine the decisions that will bring wealth, we first try to eliminate those behaviors that are guaranteed to erode it. There are some pretty obvious ones. Spending more than we make, paying high interest rates on debt so that we can’t tackle paying back the principal, and not starting to save as early as we can to take advantage of the power of compounding, are all concrete financial behaviors that cost us money. We can more readily secure wealth by using inversion to make sure we are not doing the worst things that prevent the accumulation of wealth. — Sidebar: Inversion Leads to Innovation One of the theoretical foundations for this type of thinking comes from psychologist Kurt Lewin.10 In the 1930s he came up with the idea of force field analysis, which essentially recognizes that in any situation where change is desired, successful management of that change requires applied inversion. Here is a brief explanation of his process: 1. Identify the problem 2. Define your objective 3. Identify the forces that support change towards your objective 4. Identify the forces that impede change towards the objective 5. Strategize a solution! This may involve both augmenting or adding to the forces in step 3, and reducing or eliminating the forces in step 4. Even if we are quite logical, most of us stop after step 3. Once we figure out our objective, we focus on the things we need to put in place to make it happen, the new training or education, the messaging and marketing. But Lewin theorized that it can be just as powerful to remove obstacles to change. The inversion happens between steps 3 and 4. Whatever angle you choose to approach your problem from, you need to then follow with consideration of the opposite angle. Think about not only what you could do to solve a problem, but what you could do to make it worse—and then avoid doing that, or eliminate the conditions that perpetuate it. «He wins his battles by making no mistakes.» Sun Tzu11 This inversion approach was used by Florence Nightingale to help significantly reduce the mortality rate of British soldiers in military hospitals in the late 19th century. She is often remembered as the founder of modern nursing, but she was also an excellent statistician and was the first woman elected to the Royal Statistical Society in 1858. During the first winter of the Crimean War, 1854–55, the British Army endured a death rate of 23%. The next winter that rate had dropped to 2.5%.12 The main reason for the change was a much better understanding of what was actually killing the soldiers, an understanding that rested on the detailed statistics that Florence Nightingale started to collect. She demonstrated that the leading cause of death by far was poor sanitation. In her famous polar-area chart, a completely new way of presenting data at the time, she captured a visual representation of the statistics that made them easy to understand. Improve the sanitary conditions in the hospitals, she explained, and many soldiers’ lives will be saved. Inversion Leads to Innovation Using inversion to identify your end goal and work backward from there can lead to innovation. If you had to make a guess on who invented closed circuit television (CCTV) in the United States, whom would you choose? A large institution like the Department of Defense? A telecom company? Some techie in a police department? You probably wouldn’t name the late Marie Van Brittan Brown, who, along with her husband Albert Brown, filed the first patent for a closed circuit monitoring system in 1966. She was a nurse, living in the Jamaica neighborhood of Queens, New York, and as such worked irregular hours. When she was home alone, she felt unsafe. In an interesting example of inversion, she decided to do something about it. In the same situation, most of us would work forward, thinking of safety-oriented additions we can make to our existing set-up, like more locks, or having a friend stay over. Van Brittan Brown, however, went a step further, asking what would need to change in order for her to feel safer. She identified that it was her inability to see and communicate with persons outside her door that made her feel the most vulnerable when home alone. Working backward, her thinking may have gone something like this: what can I do to change that situation? What would have to be in place? Van Brittan Brown followed this through, and CCTV was born. Van Brittan Brown and her husband designed a camera system that would move between four holes in the door, feeding the images to a TV monitor set up in the home. The images would allow her to get a complete view of who was at the door, and additional technology allowed for communication with the person outside without the door being opened. Further, they developed a feature that would allow her to either let the person in, or sound an alarm to notify a neighbor or watchman. To be fair, we will likely never know the thought process that led Van Brittan Brown to develop and patent this technology, but her story demonstrates that working backward from a goal can spur the innovation to reach it. Nightingale’s use of statistics helped to identify the real problem of army hospital deaths. She was able to demonstrate not only what the army could do to improve outcomes, but, just as important, what they had to avoid doing to stop making things worse. She reflected on the knowledge that could be derived from statistics and, in another instance of inversion thinking, she advocated for their use as a means of prevention. 13 It became not so much “how do we fix this problem,” but “how do we stop it from happening in the first place.” She took the knowledge and experience she gained in the Crimea and began gathering statistics not just for British Army field hospitals, but for domestic ones as well. She demonstrated that unsanitary conditions in military hospitals were a real problem causing many preventable deaths.14 Nightingale’s advocacy for statistics ultimately went much further than British military hospitals. But her use of statistics to improve sanitary conditions can be seen as an example of applied inversion. She used them to advocate for both solving problems and the invert, preventing them. «Hence to fight and conquer in all your battles is not supreme excellence; supreme excellence consists in breaking the enemy’s resistance without fighting.» Sun Tzu15 Conclusion Inversion shows us that we don’t always need to be geniuses, nor do we need to limit its application to mathematical and scientific proofs. Simply invert, always invert, when you are stuck. If you take the results of your inversion seriously, you might make a great deal of progress on solving your problems. Anybody can make the simple complicated. Creativity is making the complicated simple. Charles Mingus1 The People Who Appear in this Chapter William of Ockham. 1285-1347 - English philosopher and theologian. He is considered to be one of the major figures of medieval thought, and contributed to many branches of philosophy, like logic and ethics, as well as theology. Hume, David. 1711-1776 - Scottish philosopher, historian and economist. Best known today for his influential system of philosophical empiricism, skepticism and naturalism, resting on the idea that all human knowledge is founded solely in experience. Sagan, Carl. 1934-1996 - American astronomer, astrophysicist, and great communicator of science. He assembled the first physical messages sent into space. He also narrated and co-wrote Cosmos: A Personal Voyage, the most widely watched series in the history of American Public television. Rubin, Vera. 1928-2016 - American astronomer. She received multiple honors for her achievements in astronomy, and spent her life advocating for and mentoring women in science. Occam’s Razor Simpler explanations are more likely to be true than complicated ones. This is the essence of Occam’s Razor, a classic principle of logic and problem-solving. Instead of wasting your time trying to disprove complex scenarios, you can make decisions more confidently by basing them on the explanation that has the fewest moving parts. We all jump to overly complex explanations about something. Husband late getting home? What if he’s been in a car accident? Son grew a centimeter less than he did last year? What if there is something wrong with him? Your toe hurts? What if you have bone cancer? Although it is possible that any of these worst case scenarios could be true, without any other correlating factors, it is significantly more likely that your husband got caught up at work, you mismeasured your son, and your shoe is too tight. We often spend lots of time coming up with very complicated narratives to explain what we see around us. From the behavior of people on the street to physical phenomena, we get caught up in assuming vast icebergs of meaning beyond the tips that we observe. This is a common human tendency, and it serves us well in some situations, such as creating art. However, complexity takes work to unravel, manage, and understand. Occam’s Razor is a great tool for avoiding unnecessary complexity by helping you identify and commit to the simplest explanation possible. Named after the medieval logician William of Ockham, Occam’s Razor is a general rule by which we select among competing explanations. Ockham wrote that “a plurality is not to be posited without necessity”—essentially that we should prefer the simplest explanation with the fewest moving parts.2,3 They are easier to falsify, easier to understand, and generally more likely to be correct. Occam’s Razor is not an iron law but a tendency and a mind-frame you can choose to use: If all else is equal, that is if two competing models both have equal explanatory power, it’s more likely that the simple solution suffices. Of course, it’s unlikely that Ockham himself derived the idea. It had been in use since antiquity. Nor was Ockham the last to note the value of simplicity. The principle was stated in another useful way by the 18th-century Scottish philosopher David Hume, in his famous Enquiry Concerning Human Understanding. Writing about the truth or untruth of miracles, Hume stated that we should default to skepticism about them.4 Why? It wasn’t simply that Hume was a buzzkill. He had a specific, Occam-like reason for being cautious about miracles. By definition, a miracle is something which has happened outside of our normal understanding of the way nature works. If the miracle was not outside of our common experience, we wouldn’t consider its occurrence miraculous. If there was a simple explanation for the occurrence based on mostly common knowledge, we likely wouldn’t pay much attention to it at all. Therefore, the most simple explanation for a miracle is that the miracle-witnesser is not describing the event correctly, or the miracle represents a more common phenomenon that we currently don’t properly understand. As scientist and writer Carl Sagan explains in The Demon Haunted World, A multitude of aspects of the natural world that were considered miraculous only a few generations ago are now thoroughly understood in terms of physics and chemistry. At least some of the mysteries of today will be comprehensively solved by our descendants. The fact that we cannot now produce a detailed understanding of, say, altered states of consciousness in terms of brain chemistry no more implies the existence of a ‘spirit world’ than a sunflower following the Sun in its course across the sky was evidence of a literal miracle before we knew about phototropism and plant hormones.5 The simpler explanation for a miracle is that there are principles of nature being exploited that we do not understand. This is Hume’s and Sagan’s point. Dark what? In the mid-1970s astronomer Vera Rubin had a very interesting problem. She had a bunch of data about the behavior of galaxies piling up that wasn’t explained by contemporary theories.6,7,8 Rubin had been observing the behavior of the Andromeda Galaxy, and had noticed something very strange. As explained in an article on Astronomy.com, “the vast spiral seemed to be rotating all wrong. The stuff at the edges was moving just as fast as the stuff near the center, apparently violating Newton’s Laws of Motion (which also govern how the planets move around our Sun).” This didn’t make any sense. Gravity should exert less pull on distant objects, which should move slower. But Rubin was observing something entirely different. One possible explanation was something that had been theorized as far back as 1933, by Swiss astrophysicist Fritz Zwicky, who coined the phrase “dark matter” to describe a mass we couldn’t see, but which was influencing the behavior of the orbits in the galaxies. Dark matter became the simplest explanation for the observed phenomenon, and Vera Rubin has been credited with providing the first evidence of its existence. What is particularly interesting is that to this day no one has ever actually discovered dark matter. Why are more complicated explanations less likely to be true? Let’s work it out mathematically. Take two competing explanations, each of which seem to equally explain a given phenomenon. If one of them requires the interaction of three variables and the other the interaction of thirty variables, all of which must have occurred to arrive at the stated conclusion, which of these is more likely to be in error? If each variable has a 99% chance of being correct, the first explanation is only 3% likely to be wrong. The second, more complex explanation, is about nine times as likely to be wrong, or 26%. The simpler explanation is more robust in the face of uncertainty. Dark matter is an excellent theory with a lot of explanatory power. As Lisa Randall explains in Dark Matter and the Dinosaurs, measurements of dark matter so far fit in exactly with what we understand about the Universe. Although we can’t see it, we can make predictions based on our understanding of it, and test those predictions. She writes, “It would be even more mysterious to me if the matter we can see with our eyes is all the matter that exists.”9 Dark matter is currently the simplest explanation for certain phenomena we observe in the Universe. The great thing about science, however, is that it continually seeks to validate its assumptions. Sagan wrote that “extraordinary claims require extraordinary proof.”10 He dedicated much ink to a rational investigation of extraordinary claims. He felt most, or nearly all, were susceptible to simpler and more parsimonious explanations. UFOs, paranormal activity, telepathy, and a hundred other seemingly mystifying occurrences could be better explained with a few simple real world variables. And as Hume suggested, if they couldn’t, it was a lot more likely that we needed to update our understanding of the world than that a miracle had occurred. And so, dark matter remains, right now, the simplest explanation for the peculiar behavior of galaxies. Scientists, however, continue to try to conclusively discover dark matter and thus try to determine if our understanding of the world is correct. If dark matter eventually becomes too complicated an explanation, it could be that the data describes something we don’t yet understand about the universe. We can then apply Occam’s Razor to update to what is the simplest, and thus easiest to verify, explanation. Vera Rubin herself, after noting that scientists always felt like they were ten years away from discovering dark matter without ever closing the gap, was described in an interview as thinking, “The longer that dark matter went undetected, … the more likely she thought the solution to the mystery would be a modification to our understanding of gravity.”11 This claim, demanding a total overhaul of our established theories of gravity, would correspondingly require extraordinary proof! Simplicity can increase efficiency With limited time and resources, it is not possible to track down every theory with a plausible explanation of a complex, uncertain event. Without the filter of Occam’s Razor, we are stuck chasing down dead ends. We waste time, resources, and energy. The great thing about simplicity is that it can be so powerful. Sometimes unnecessary complexity just papers over the systemic flaws that will eventually choke us. Opting for the simple helps us make decisions based on how things really are. Here are two short examples of those who got waylaid chasing down complicated solutions when simple ones were most effective. The ten-acre Ivanhoe Reservoir in Los Angeles provides drinking water for over 600,000 people. Its nearly 60 million gallons of water are disinfected with chlorine, as is common practice.12 Ground water often contains elevated levels of a chemical called bromide. When chlorine and bromide mix, then are exposed to sunlight, they create a dangerous carcinogen called bromate. In order to avoid poisoning the water supply, the L.A. Department of Water and Power (DWP) needed a way to shade the water’s surface. Brainstorming sessions had yielded only two infeasible solutions, building either a ten-acre tarp or a huge retractable dome over the reservoir. Then a DWP biologist suggested using “bird balls,” the floating balls that airports use to keep birds from congregating near runways. They require no construction, no parts, no labor, no maintenance, and cost US$0.40 each. Three million UV-deflecting black balls were then deployed in Ivanhoe and other LA reservoirs, a simple solution to a potentially serious problem. Occam’s Razor in the Medical Field Occam’s Razor can be quite powerful in the medical field, for both doctors and patients. Let’s suppose that a patient shows up at a doctor’s office with horrible flu-like symptoms. Are they more likely to have the flu or have contracted Ebola? This is a problem best solved by a concept we explored in the chapter on Probabilistic Thinking, called Bayesian Updating. It’s a way of using general background knowledge in solving specific problems with new information. We know that generally the flu is far more common than Ebola, so when a good doctor encounters a patient with what looks like the flu, the simplest explanation is almost certainly the correct one. A diagnosis of Ebola means a call to the Center for Disease Control and a quarantine—an expensive and panic-inducing mistake if the patient just has the flu. Thus, medical students are taught to heed the saying, “When you hear hoofbeats, think horses, not zebras.” And for patients, Occam’s Razor is a good counter to hypochondria. Based on the same principles, you factor in the current state of your health to an evaluation of your current symptoms. Knowing that the simplest explanation is most likely to be true can help us avoid unnecessary panic and stress. In another life-and-death situation, in 1989 Bengal tigers killed about 60 villagers from India’s Ganges delta.13 No weapons seemed to work against them, including lacing dummies with live wires to shock the tigers away from human populations. Then a student at the Science Club of Calcutta noticed that tigers only attacked when they thought they were unseen, and recalled that the patterns decorating some species of butterflies, beetles, and caterpillars look like big eyes, ostensibly to trick predators into thinking their prey was also watching them. The result: a human face mask, worn on the back of head. Remarkably, no one wearing a mask was attacked by a tiger for the next three years; anyone killed by tigers during that time had either refused to wear the mask, or had taken it off while working. — Sidebar: Occam’s Razor in the Medical Field A few caveats One important counter to Occam’s Razor is the difficult truth that some things are simply not that simple. The regular recurrence of fraudulent human organizations like pyramid schemes and Ponzi schemes is not a miracle, but neither is it obvious. No simple explanation suffices, exactly. They are a result of a complex set of behaviors, some happening almost by accident or luck, and some carefully designed with the intent to deceive. It isn’t a bit easy to spot the development of a fraud. If it was, they’d be stamped out early. Yet, to this day, frauds frequently grow to epic proportions before they are discovered. Alternatively, consider the achievement of human flight. It, too, might seem like a miracle to our 17th century friar, but it isn’t—it’s a natural consequence of applied physics. Still, it took a long time for humans to figure out because it’s not simple at all. In fact, the invention of powered human flight is highly counterintuitive, requiring an understanding of airflow, lift, drag, and combustion, among other difficult concepts. Only a precise combination of the right factors will do. You can’t just know enough to get the aircraft off the ground, you need to keep it in the air! The Razor in Leadership When Louis Gerstner took over IBM in the early 1990s, during one of the worst periods of struggle in its history, many business pundits called for a statement of his vision. What rabbit would Gerstner pull out of his hat to save Big Blue? It seemed a logical enough demand—wouldn’t a technology company that had fallen behind need a grand vision of brilliant technological leadership to regain its place among the leaders of American innovation? As Gerstner put it, “The IBM organization, so full of brilliant, insightful people, would have loved to receive a bold recipe for success—the more sophisticated, the more complicated the recipe, the better everyone would have liked it.” Smartly, Gerstner realized that the simple approach was most likely to be the effective one. His famous reply was that “the last thing IBM needs right now is a vision.” What IBM actually needed to do was to serve its customers, compete for business in the here and now, and focus on businesses that were already profitable. It needed simple, tough-minded business execution. By the end of the 1990s, Gerstner had provided exactly that, bringing IBM back from the brink without any brilliant visions or massive technological overhauls. Simple as we wish things were, irreducible complexity, like simplicity, is a part of our reality. Therefore, we can’t use this Razor to create artificial simplicity. If something cannot be broken down any further, we must deal with it as it is. How do you know something is as simple as it can be? Think of computer code. Code can sometimes be excessively complex. In trying to simplify it, we would still have to make sure it can perform the functions we need it to. This is one way to understand simplicity. An explanation can be simplified only to the extent that it can still provide an accurate understanding. Conclusion Of course, focusing on simplicity when all others are focused on complexity is a hallmark of genius, and it’s easier said than done. But always remembering that a simpler explanation is more likely to be correct than a complicated one goes a long way towards helping us conserve our most precious resources of time and energy. — Sidebar: The Razor in Leadership I need to listen well so that I hear what is not said. Thuli Madonsela1 The People Who Appear in this Chapter Honorius. 384-423 - Western Roman Emperor for 30 years. His reign was chaotic and messy, and saw Rome being sacked for the first time in almost 800 years. Stilicho. 359-408 - High ranking general in the Roman army. Half Vandal, his regency for Honorius marked the high point of Germanic advancement in the service of Rome. Arkhipov, Vasili. 1926-1998 - Russian. Retired as a Vice-Admiral in the Soviet Navy. In 1961 he was deputy commander of K-19. The events on board inspired the Harrison Ford movie, K-19: The Widowmaker. Hanlon’s Razor Hard to trace in its origin, Hanlon’s Razor states that we should not attribute to malice that which is more easily explained by stupidity. In a complex world, using this model helps us avoid paranoia and ideology. By not generally assuming that bad results are the fault of a bad actor, we look for options instead of missing opportunities. This model reminds us that people do make mistakes. It demands that we ask if there is another reasonable explanation for the events that have occurred. The explanation most likely to be right is the one that contains the least amount of intent. Assuming the worst intent crops up all over our lives. Consider road rage, a growing problem in a world that is becoming short on patience and time. When someone cuts you off, to assume malice is to assume the other person has done a lot of risky work. In order for someone to deliberately get in your way they have to notice you, gauge the speed of your car, consider where you are headed, and swerve in at exactly the right time to cause you to slam on the brakes, yet not cause an accident. That is some effort. The simpler and thus more likely explanation is that they didn’t see you. It was a mistake. There was no intent. So why would you assume the former? Why do our minds make these kinds of connections when the logic says otherwise? The famous Linda problem, demonstrated by the psychologists Daniel Kahneman2 and Amos Tversky in a 1982 paper, is an illuminating example of how our minds work and why we need Hanlon’s Razor. It went like this: Linda is 31 years old, single, outspoken, and very bright. She majored in philosophy. As a student, she was deeply concerned with issues of discrimination and social justice, and also participated in anti-nuclear demonstrations. Which is more probable? 1. Linda is a bank teller. 2. Linda is a bank teller and is active in the feminist movement. The majority of respondents chose option 2. Why? The wording used to describe her suggests Linda is feminist. But Linda could only be a bank teller, or a feminist and a bank teller. So naturally the majority of students concluded she was both. They didn’t know anything about what she did, but because they were led to believe she had to be a feminist they couldn’t reject that option, even though the math of statistics makes it more likely that a single condition is true instead of multiple conditions. In other words, every feminist bank teller is a bank teller, but not every bank teller is a feminist. Thus, Kahneman and Tversky showed that students would, with enough vivid wording, assume it more likely that a liberal-leaning woman was both a feminist and a bank teller rather than simply a bank teller. They called it the “Fallacy of Conjunction.” With this experiment, and a host of others, Kahneman and Tversky exposed a sort of tic in our mental machinery: we’re deeply affected by vivid, available evidence, to such a degree that we’re willing to make judgments that violate simple logic. We over-conclude based on the available information. We have no trouble packaging in unrelated factors if they happen to occur in proximity to what we already believe. The Linda problem was later criticized as the psychologists setting their test subjects up for failure. If it was stated in a different way, subjects did not always make the error. But this of course was their point. If we present the evidence in a certain light, the brain malfunctions. It doesn’t weigh out the variables in a rational way. What does this have to do with Hanlon’s Razor? The connection is this: When we see something we don’t like happen and which seems wrong, we assume it’s intentional. But it’s more likely that it’s completely unintentional. Assuming someone is doing wrong and doing it purposefully is like assuming Linda is more likely to be a bank teller and a feminist. Most people doing wrong are not bad people trying to be malicious. With such vividness, and the associated emotional response, comes a sort of malfunctioning in our minds when we’re trying to diagnose the causes of a bad situation. That’s why we need Hanlon’s Razor as an important remedy. Failing to prioritize stupidity over malice causes things like paranoia. Always assuming malice puts you at the center of everyone else’s world. This is an incredibly self-centered approach to life. In reality, for every act of malice, there is almost certainly far more ignorance, stupidity, and laziness. «One is tempted to define man as a rational animal who always loses his temper when he is called upon to act in accordance with the dictates of reason.» Oscar Wilde3 The end of an empire In 408 AD, Honorius was the Emperor of the Western Roman Empire. He assumed malicious intentions on the part of his best General, Stilicho, and had him executed. According to some historians, this execution may have been a key factor in the collapse of the Empire.4,5 Why? Stilicho was an exceptional military general who won many campaigns for Rome. He was also very loyal to the Empire. He was not, however, perfect. Like all people, he made some decisions with negative outcomes. One of these was persuading the Roman Senate to accede to the demands of Alaric, leader of the Visigoths. Alaric had attacked the Empire multiple times and was no favorite in Rome. They didn’t want to give in to his threats and wanted to fight him. Stilicho counseled against this. Perhaps he had a relationship with Alaric and thought he could convince him to join forces and push back against the other invaders Rome was dealing with. Regardless of his reasoning, this action of Stilicho’s compromised his reputation. Honorius was thus persuaded of the undesirability of having Stilicho around. Instead of defending him, or giving him the benefit of the doubt on the Alaric issue, Honorius assumed malicious intent behind Stilicho’s actions—that he wanted the throne and so was making decisions to shore up his power. Honorius ordered the general’s arrest and likely supported his execution. Without Stilicho to influence the relationship with the Goths, the Empire became a military disaster. Alaric sacked Rome two years later, the first barbarian to capture the city in nearly eight centuries. Rome was thus compromised, a huge contributing factor to the collapse of the Western Roman Empire. Hanlon’s Razor, when practiced diligently as a counter to confirmation bias, empowers us, and gives us far more realistic and effective options for remedying bad situations. When we assume someone is out to get us, our very natural instinct is to take actions to defend ourselves. It’s harder to take advantage of, or even see, opportunities while in this defensive mode because our priority is saving ourselves—which tends to reduce our vision to dealing with the perceived threat instead of examining the bigger picture. The man who saved the world On October 27, 1962, Vasili Arkhipov stayed calm, didn’t assume malice, and saved the world. Seriously. This was the height of the Cuban missile crisis. Tensions were high between the United States and the Soviet Union. The world felt on the verge of nuclear war, a catastrophic outcome for all. American destroyers and Soviet subs were in a standoff in the waters off Cuba. Although they were technically in International waters, the Americans had informed the Soviets that they would be dropping blank depth charges to force the Soviet submarines to surface. The problem was, Soviet HQ had failed to pass this information along, so the subs in the area were ignorant of the planned American action.6 Arkhipov was an officer aboard Soviet sub B-59—a sub that, unbeknownst to the Americans, was carrying a nuclear weapon. When the depth charges began to detonate above them, the Soviets on board B-59 assumed the worst. Convinced that war had broken out, the captain of the sub wanted to arm and deploy the nuclear-tipped torpedo. This would have been an unprecedented disaster. It would have significantly changed the world as we know it, with both the geopolitical and nuclear fallout affecting us for decades. Luckily for us, the launch of the torpedo required all three senior officers on board to agree, and Arkhipov didn’t. Instead of assuming malice, he stayed calm and insisted on surfacing to contact Moscow. Although the explosions around the submarine could have been malicious, Arkhipov realized that to assume so would put the lives of billions in peril. Far better to suppose mistakes and ignorance, and base the decision not to launch on that. In doing so, he saved the world. They surfaced and returned to Moscow. Arkhipov wasn’t hailed as a hero until the record was declassified 40 years later, when documents revealed just how close the world had come to nuclear war. The Devil Fallacy Robert Heinlein’s character Doc Graves describes the Devil Fallacy in the 1941 sci-fi story “Logic of Empire”, as he explains the theory to another character: “I would say you’ve fallen into the commonest fallacy of all in dealing with social and economic subjects—the ‘devil’ theory. You have attributed conditions to villainy that simply result from stupidity…. You think bankers are scoundrels. They are not. Nor are company officials, nor patrons, nor the governing classes back on earth. Men are constrained by necessity and build up rationalizations to account for their acts.” Hanlon’s Razor is a great tool for overcoming this fallacy, one we all fall into at one time or another. As useful as it can be, it is, however, important not to overthink this model. Hanlon’s Razor is meant to help us perceive stupidity or error, and their inadvertent consequences. It says that of all possible motives behind an action, the ones that require the least amount of energy to execute (such as ignorance or laziness) are more likely to occur than one that requires active malice. Conclusion Ultimately, Hanlon’s Razor demonstrates that there are fewer true villains than you might suppose—what people are is human, and like you, all humans make mistakes and fall into traps of laziness, bad thinking, and bad incentives. Our lives are easier, better, and more effective when we recognize this truth and act accordingly. — Sidebar: The Devil Fallacy Acknowledgements I’m forever indebted to Charlie Munger, Peter Kaufman, Warren Buffett, and Peter Bevelin, who, to varying degrees, started me down the path of multidisciplinary thinking. I owe them a huge debt of gratitude. While I’ve sought wisdom from the world, it’s a journey that one starts and never completes. When I first started learning about multidisciplinary thinking there was no source of collected wisdom—no place where I could find the big ideas from multiple disciplines in one place. This book, and indeed, this series, is meant to bring some of the big invariant ideas into the world to help us better understand the world we live in and how it interconnects. These pages are filled with ideas from many great minds. It’s only fair to point out that any idea you found in this book comes from someone else. This book would not be here without the work and talents of Rhiannon Beaubien, Morgwn Rimel, Marcia Mihotich, and Garvin Hirt. I have many friends to thank for reading the manuscript and offering thoughtful and critical feedback for which I’m grateful. Thank you Zachary Smith, Devon Anderson, Jeff Annello, Simon Hørup Eskildsen, Laurence Endersen, and Paul Ciampa. I want to thank Néna Rawdah for helping edit this from a manuscript into a book. I also want to thank my kids, William and Mackenzie, who, without knowing it, have provided me with a renewed sense of curiosity and understanding. This book is for you. I hope you pull this book off your shelf whenever you’re stuck or need inspiration in understanding our world. It’s designed to be a reference book of big ideas but also a book that pulls you in aesthetically through the intersection of design and content. Shane