MED106_5b Sampling and Random Error 95%CI PDF
Document Details
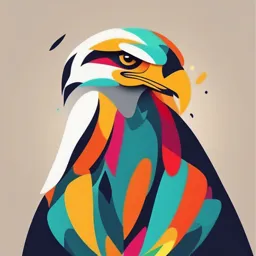
Uploaded by AppreciableDouglasFir
University of Nicosia
Avgis Hadjipapas
Tags
Summary
This document provides an overview of sampling methods, random error, and confidence intervals in medical research. It explains the concept of samples, estimates and their relationship to populations, along with the calculation of 95% confidence intervals.
Full Transcript
Sampling, random error and Confidence Intervals Avgis Hadjipapas Professor for Neuroscience and Research methods [email protected] Session LOBs LOB19: Describe the concept of the sample and how it relates to the population LOB20: Describe the concept of the estimate and its importance in medic...
Sampling, random error and Confidence Intervals Avgis Hadjipapas Professor for Neuroscience and Research methods [email protected] Session LOBs LOB19: Describe the concept of the sample and how it relates to the population LOB20: Describe the concept of the estimate and its importance in medical research. LOB21: Describe the concept of the random error (chance) LOB22: Interpret a 95% Confidence Interval What is a sample? Sample Population Sample Sample A sample is a selected subset of a source population Briefly (details in next slides), the source population is the group of all individuals in which we are interested to assess some parameter(s) Ideally, the sample should be representative of the source population The purpose of taking a sample is to study something that we cannot study in the whole population, due to the obvious practical restrictions (financial, time) Scientific research is almost always conducted in samples In very rare cases, research may be conducted in whole populations, but these populations are usually rather small Sampling http://yihui.name/en/2007/10/animations-in-survey-sampling/ Sampling Sampling is the process of selecting a number of individuals from all individuals found in a source population The sampling frame is a list (or database) containing all individuals in a population and is used for sampling The sampling units are the individuals to be potentially selected. Sampling units most of the time are individual people, but we could also have larger sampling units (i.e. families, streets, hospitals, schools, etc.) There are several different sampling methods for selecting a sample from a source population (covered in a different session..) What is the population from which the sample was taken? Source population The source population is the group of all individuals in which we are interested to assess some parameter(s) The source population can be the general population (i.e. the total population of a country or city), but can also be a specific sub-population (i.e. all smokers of a country, all patients with heart disease, all children with cancer, etc.) In descriptive research (i.e. when we want to investigate prevalence/incidence of a condition in a population), it is particularly important that the sample accurately represents the specific source population Source population In analytic research (i.e. when we investigate association between exposure and outcome), we can be more general regarding the source population, depending on the research question of interest In situations where we investigate a biological effect on some disease (i.e. effect of smoking on risk of cancer), we can be more general in identifying the source population (i.e. not necessarily restricted to specific country/region) In situations where we investigate social/cultural effects (i.e. effect of social class on risk of heart disease), we have to more careful and restrict the source population to the specific country/region from where the sample was derived Examples: Which is the source population? 1. A study investigated the prevalence of obesity in Cyprus, by recruiting a random sample of adults. Source population?? 2. A study investigated the association between smoking and oesophageal cancer among a sample of 35-65 year olds in Canada Source population?? 3. A study investigated the association between educational attainment and stroke among a sample of elderly individuals in Sweden Source population?? Introduction to statistical inference Introduction to statistical inference In order to determine the proportion of a characteristic in a population, we usually (actually almost always..) measure that in a sample Therefore what we measure is an estimate. This estimate carries an inherent error (sampling error) When the sample estimate is used to draw conclusions (inferences) about the population from which the sample was taken, this is called statistical inference Statistical inference, as the name suggests, involves the use of statistics to determine the degree of uncertainty in the estimate of interest Population parameter and sample estimate A parameter is a measurement of a quantity (or association) in a population, which we are interested about, e.g: mean age prevalence of obesity mean difference in blood pressure between men and women Odds Ratio for association between smoking and cancer An estimate is a measurement of a quantity (or association) in a sample, which aims to represent the true quantity or association in the source population (parameter) Thus, the sample estimate attempts to quantify the corresponding population parameter Population parameter and sample estimate For any given variable… Sample estimate mean = 3.75 Population parameter mean = 3.72 www.socialresearchmethods.net/ Example: Estimating the mean in a population An estimate can be the mean of a characteristic Example: We want to determine the mean BMI in Cyprus. It would be too costly and unreliable to identify ALL residents of the country and measure their weight and height Alternatively, a more feasible approach would be to take a sample from the population and determine the mean BMI in the sample This sample mean is our (sample) estimate and we will assess how close we think we are to the actual mean in the source population (population parameter) Example: Estimating the mean in a population Population (N=900,000) 28.5 Kg/m2 29.1 Kg/m2 sample (n=1000) mean BMI in Cyprus Is our estimate accurate.. ? Example: Estimating the mean in a population (sample n=1000) Population (N=900,000) 28.5 Kg/m2 28.8 Kg/m2 sample (n=1000) 28.9 Kg/m2 sample 28.1 Kg/m2 (n=1000) mean BMI in Cyprus Sampling variation and sampling error The difference (variation) between different sample estimates derived from the same source population is called sampling variation The difference in magnitude between the sample estimates and the actual population parameter caused by measuring a quantity (or association) in a sample rather than in the source population, is called sampling error Because sampling error is a result of chance, it is usually referred to as random error (or statistical error) Sample size plays a very important role in the magnitude of this random error (more on this in the next slides..) Example: Estimating the mean in a population (sample n=100) Population (N=900,000) 28.5 Kg/m2 30.2 Kg/m2 sample (n=100) sample 26.8 Kg/m2 (n=100) 29.3 Kg/m2 mean BMI in Cyprus Note: Notice what happens to both the sampling variation and the sampling (random) error when we decrease our sample size from n=1000 to n=100 ! Example: Estimating the prevalence in a population Population (N=11,000,000) 25% 38% sample (n=50) 24% sample (n=5000) Prevalence of obesity in Greece Example: Estimating an Odds Ratio in a population Population 1.80 (N=?) 3.50 sample (n=50) 5.20 sample (n=50) 3.75 sample (n=5000) OR for the association between head injury and Parkinson’s Disease Note: Same principles apply for all other measures we covered so far: Incidence Risk Ratio Rate ratio Mean difference Correlation coefficient Regression coefficient All of the above are termed estimates, when they are calculated in a sample A closer look at sampling variation Hypothetical variable with a (population) mean =5. Take repeated samples with a certain sample size n Calculate mean in the samples & plot sample estimates in a histogram: sampling distribution (sample n=3) Population parameter (true mean=5) Sampling distribution Frequency Sample means (estimates) 0 0.5 1 1.5 2 2.5 3 3.5 4 4.5 5 5.5 6 6.5 7 7.5 8 8.5 9 9.5 10 Increase sample size n (sample n=100) Population parameter (true mean=5) Sampling distribution Frequency Sample means (estimates) 0 0.5 1 1.5 2 2.5 3 3.5 4 4.5 5 5.5 6 6.5 7 7.5 8 8.5 9 9.5 10 Further increase sample size n (sample n=1000) Population parameter (true mean=5) Sampling distribution Frequency Sample means (estimates) 0 0.5 1 1.5 2 2.5 3 3.5 4 4.5 5 5.5 6 6.5 7 7.5 8 8.5 9 9.5 10 The standard error and the 95% Confidence Interval Standard Error (SE): the concept The standard error describes the uncertainty of how well the sample estimate represents the population parameter It essentially estimates the standard deviation of the sampling distribution, i.e. the average error that can occur whenever we take a sample of a certain size n A SE exists for all statistical quantities not just means The SE can be estimated from a single (!) sample For the mean: , where S is sample standard deviation and n is sample size The standard error can be used to calculate the degree of uncertainty around an estimate: the 95% Confidence Interval Confidence intervals indicate a range (interval) within which we are confident (with some degree of uncertainty) that the true population parameter lies the 95% Confidence Interval (95% CI) for a sample estimate is calculated as: Lower confidence interval sample estimate – 1.96*standard error Upper confidence interval sample estimate + 1.96*standard error Interpretation (IMPORTANT!): We are 95% confident that the population parameter is contained within the interval sample estimate +/- 1.96 SE The 95% Confidence Interval: a measure of uncertainty (sample n=20, SE=0.89) Frequency Lower confidence interval sample mean – 1.96*standard error Upper confidence interval sample mean + 1.96*standard error Lower confidence interval 5.2 – 1.96*0.89 Upper confidence interval 5.2 + 1.96*0.89 Lower confidence interval 3.45 Upper confidence interval 6.95 0 1 1.5 2 2.5 3 3.5.4 4.5 5 6 6.5 7 7.5 8 8.5 9 9.5 10 The 95% Confidence Interval: a measure of uncertainty (sample n=1000, SE=0.13) Frequency Lower confidence interval sample mean – 1.96*standard error Upper confidence interval sample mean + 1.96*standard error Lower confidence interval 5.2 – 1.96*0.13 Upper confidence interval 5.2 + 1.96*0.13 Lower confidence interval 4.95 Upper confidence interval 5.45 0 1 1.5 2 2.5 3 3.5.4 4.5 5 6 6.5 7 7.5 8 8.5 9 9.5 10 For the previous example: For a sample of n=1000 We are 95% certain that the true population mean lies between 4.95 and 5.45 => precise (low uncertainty regarding true population mean) For a sample of n=20 We are 95% certain that the true population mean lies between 3.45 and 6.95 => not very precise (high uncertainty regarding true population mean) Examples (Confidence Intervals) 1. Association between smoking (smokers vs. non-smokers) and blood pressure Result: mean difference 12.3 (95% CI: 10.8; 13.8) Interpretation of CIs: We are 95% certain that the true population mean difference lies between 10.8 and 13.8 2. Association between age and blood pressure Result: regression coefficient 3.6 (95% CI: 0.5; 6.7) Interpretation of CIs: We are 95% certain that the true population regression coefficient lies between 0.5 and 6.7 3. Association between obesity (obese vs non-obese) and hypertension (yes/no) Result: Odds Ratio 2.10 (95% CI: 0.80; 3.40) Interpretation of CIs: We are 95% certain that the true population Odds Ratio lies between 0.80 and 3.40 Session LOBs LOB19: Describe the concept of the sample and how it relates to the population LOB20: Describe the concept of the estimate and its importance in medical research. LOB21: Describe the concept of the random error (chance) LOB22: Interpret a 95% Confidence Interval Further reading (optional) Petrie A. & Sabin C. Medical Statistics at a Glance, 3rd Edition, Chapter 10 [ISBN : 978-1-4051-8051-1] http://www.bmj.com/about-bmj/resourcesreaders/publications/statistics-square-one/3-populations-andsamples