Lecture Notes - Estimating Risk PDF
Document Details
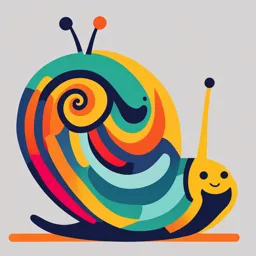
Uploaded by AmpleJudgment
Bishop Gorman High School
Tags
Summary
These lecture notes cover the calculation of measures used in public health and epidemiology, focusing on determining risk. The concepts of relative risk, odds ratios, and absolute risk are explained in detail. Examples and case studies are included.
Full Transcript
An Estimating Risk: We will mainly be learning about how to calculate measures that are important in public health and epidemiological research, particularly for those measures of associations related to case control studies and cohort studies. These measures include relative risk and odds ratios. W...
An Estimating Risk: We will mainly be learning about how to calculate measures that are important in public health and epidemiological research, particularly for those measures of associations related to case control studies and cohort studies. These measures include relative risk and odds ratios. When we think of Abby and the aims of epidemiology, they mainly pertain to disease prevention. This can be achieved by determining the risk of disease, identifying risk factors, and developing strategies for disease prevention. To meet these objectives, we need to rely on several measures, which we will learn about today. For instance, when we want to determine the risk of disease by person, place, and time, we turn to absolute risk. This includes measures such as incidence and prevalence, which you are all familiar with. Another objective of epidemiology is to identify risk factors for disease. This is accomplished through the design of studies such as cohort studies and case control studies. But how do we actually pinpoint which particular exposure is associated with our disease of interest? This is where measures of association come in. For cohort studies, we use relative risk, while for case control studies, we use odds ratios. Lastly, when considering potential strategies to combat disease, we want to have the highest yield in terms of disease reduction. To do this, we need to know how much of the disease would be reduced if we eliminated a certain risk factor. This is where the measure of attributable risk comes in. Let's Girst discuss absolute risk. Absolute risk is the likelihood of an individual in a population developing a certain disease. It essentially measures the incidence of disease within a given population. This provides an indication of the magnitude of risk in a group of people who have a certain exposure, and the risk of developing the disease over a period of time. If we look back at incidence, we recall that it measures the new cases in a population over time. This indicates the force of the disease or conversion of health status. It can be expressed as cumulative incidence or incidence density, which takes into account accumulated person time. However, it does not provide any information about the association between the exposure and the disease because no comparison is made. Let's look at some examples of absolute risk. In the Girst example, it is stated that congenital rubella appears in 80 to 100% of infants if maternal infection of rubella occurs during the Girst trimester of pregnancy. This provides us with information about the absolute risk, but it does not give us information about the measure of association between maternal rubella infection during the Girst trimester and congenital rubella in the infant because there is no comparison group. We need to consider infants whose mothers had infections in the second or third trimester as well. In the second example, it is stated that vertical transmission of parvovirus B19 is approximately 35%. While this is important information, it only provides us with the absolute risk of parvovirus B19 from mother to child during gestational development. It does not have a comparison group, which is necessary to determine associations. The same is said about the last example. Approximately 90% of infants perinatally or infected during the Girst year of life develop chronic hepatitis B virus infection. Again, the comparison is only implicit. There is no explicit comparison, which is needed to determine whether an association exists. Thus, these examples are considered only as absolute risk. To truly examine whether an association exists between exposure and a disease or health outcome, we need to have comparison groups - groups without the disease and groups without the exposure. This way, we can see if there is an excess risk of disease in the exposed group. If the association is strong, then there is more evidence to support that the relationship is causal. A court team always considers the inGluence of confounding factors, as we discussed in the previous lectures. Several studies are utilized to help investigators examine research questions that seek to learn more about the relationship between exposure and outcome, particularly case-control studies and cohort studies. Each study design has a measure of association that is linked to it. For example, the cohort study is able to yield a relative risk. It can also yield an odds ratio because of the way it is designed. However, for the most part, a relative risk is seen as a stronger measure of association. So when people ask what the measure of association is in a cohort study, the answer is a relative risk. Case-control studies, on the other hand, cannot calculate relative risk. They are only able to determine odds ratios. So when you think of a case-control study, you should immediately think of the odds ratio. Before we go into the calculation and interpretation of these measures, let's revisit the general steps in every research. We Girst start with an idea a research question we would like to address and Gind out more about. We hone our question and determine our population of interest. We think about exposure assessment and the deGinition of our outcome of interest. We sample our population and determine exposure and disease status based on our conceptualized measures of them. We then estimate whether an association exists between our exposure and disease using appropriate statistical models and evaluate whether the Gindings support a potential causal association. These two steps are where we are now in the semester, and today we will discuss estimating risk. To do this, we rely on the handy dandy contingency table, otherwise known as the two-by-two table. This is an important tool that is utilized to help determine the association between exposure and disease by cross-classifying exposure status and disease status. The columns of a two-by-two table represent disease status - yes or no - and the rows represent exposure status - yes or no. Some people switch it up depending on what they are examining. My suggestion is to only stick with one format to avoid confusion with the formulas when starting the calculations. As mentioned earlier, the columns are for disease status. The Girst column consists of people who have the disease, and the second column is for people without the disease. The rows represent exposure status. The Girst row is for everyone who is exposed, and the second row is for everyone who is not exposed. So what you end up with are four categories of people. In block A, you have people who have the disease and are also exposed. In block B, you have people who are exposed but do not have the disease. In block C, you have people who have the disease but were not exposed. And in block D, you have people who are not diseased and were not exposed. You can have your totals on the sides of the table. On the far right, you have the total number exposed as a sum of A + B. Underneath, you have the total unexposed with C + D. On the bottom left, you have the total number of diseased with A + C, and the total number of not diseased with B + D. This table is crucial as you move forward in calculating measures of association for case-control and cohort studies. When you are asked to construct a two-by-two table, refer to the side and set up the two-by-two table. This way, you can Gill in the table with the information that is given in the prompt. Relative risk is the probability of an event happening in exposed people compared to the probability of the event happening in non-exposed people. To understand this concept, let's consider the design of a cohort study. In a cohort study, we start with exposure status and follow people for a speciGied period of time to determine whether disease develops. Therefore, it is logical that the relative risk, which is the measure of association for cohort studies, is deGined as the probability of disease development in exposed individuals compared to the probability of disease development in unexposed individuals. Essentially, it is a ratio of two incidence rates. It is important to note that we cannot calculate relative risk in a case-control study because these studies do not have incidence data. This is because case-control studies start with disease and then go to exposure. The relative risk has the conditional risk of disease among the exposed in the numerator and the conditional risk of disease among the unexposed in the denominator. This can be seen in the formula on the bottom right of the slide. In the numerator, we have A / (A + B), which represents the number of exposed people who develop the disease over the total number of people exposed. In the denominator, we have C / (C + D), which represents the number of unexposed people who develop the disease over the total number of people who were unexposed. While the calculation itself is simple, it is important to understand the meaning of the resulting number. If the relative risk is equal to one, it means that the risk of disease in the exposed group is equal to the risk of disease in the unexposed group. This suggests that there is no evidence of an association between the exposure and the disease. However, if the relative risk is greater than one, it indicates a positive association, as the risk among the exposed is higher than the risk among the unexposed. On the other hand, a relative risk less than one suggests an inverse association, meaning that the risk among the exposed is lower than that of the unexposed. This can be seen in the example of physical activity and obesity development, where the relative risk would likely be less than one. Let's look at an example to further understand how to calculate relative risk. In this scenario, we are examining the association between cigarette smoking (deGined as greater than or equal to 1 pack per day) and heart attacks (yes or no) over a Give-year follow-up period. To calculate the relative risk, we need to compare the incidence of heart attacks in the exposed group to the incidence in the unexposed group. In this case, the exposed group consists of 1074 smokers, and the unexposed group consists of 1076 non-smokers. To calculate the incidence among smokers, we divide the number of smokers who had heart attacks (8.2%) by the total number of smokers (1074). This gives us an incidence of 8.2% among the exposed group. Similarly, to calculate the incidence among non-smokers, we divide the number of non-smokers who had heart attacks (2.4%) by the total number of non-smokers (1076). This gives us an incidence of 2.4% among the unexposed group. Using these values, we can calculate the relative risk using the formula on the bottom of the slide. We plug in the incidences we calculated earlier (8.2% for the numerator and 2.4% for the denominator) and calculate a relative risk of 3.4. This means that the risk of heart attacks is 3.4 times higher in smokers compared to non-smokers. In summary, relative risk is a measure of association that compares the risk of disease in exposed individuals to the risk in unexposed individuals. It is calculated by dividing the incidence in the exposed group by the incidence in the unexposed group. A relative risk of one indicates no association, while a relative risk greater than one suggests a positive association and a relative risk less than one suggests an inverse association. Or, how do we go about interpreting these numbers? Let's start with the Give-year incidence we calculated for smokers and non-smokers. As you recall, the incidence for smokers was 8.2%. This means that the probability of heart attacks is 8.2% among people who smoke at least one pack a day. The probability of a heart attack among people who were unexposed or did not smoke is 2.4%. The relative risk we obtained was 3.4. This means that there is a 3.4 greater probability of heart attacks for people who smoke at least one pack of cigarettes per day compared to people who did not smoke at all. This indicates a positive association and excess risk among the exposed of our disease of interest. For the second example, we are examining the relationship between a history of sexual abuse and suicide attempts in adolescents. We have our numbers for each group listed in the table. We again calculate the incidence rate among the exposed and the unexposed. We get 60.8% as the incidence of suicide attempts for people who had a history of sexual abuse, compared to 24.7% among people who did not have a history of sexual abuse. We can use the incidence among our exposed and unexposed groups to calculate our relative risk. We plug the incidence values into the formula, with 16.8% going into the numerator because this represents the incidence of our outcome, suicide attempts, among people who were exposed, meaning those who had a history of sexual abuse. Then we take 24.7% and plug it into our denominator because this represents the incidence among adolescents with no history of sexual abuse. We calculate this to get a relative risk of 2.46. Let's quickly review what we found. We found that the probability of a suicide attempt among adolescents with a history of sexual abuse was 60.8%. Among our exposed group, we found that the probability of suicide attempts was only 24.7%. This relative risk of 2.46 indicates a positive association and excess of suicide attempts among adolescents who were sexually abused compared to those who were not. Based on this value, we can say that there is a 2.5-fold greater probability of a suicide attempt for adolescents with a history of sexual abuse compared to those with no history of sexual abuse. For the third example, we would like to compare the incidence of lung cancer between two cities, SpringGield and Shelbyville. Both cities have a small population of 100 residents. In SpringGield, there is polluted air, thus this city is categorized as our exposed group. In SpringGield, there are 20 cases of lung cancer. In our second city, Shelbyville, there is no polluted air, thus it is considered our unexposed group. In Shelbyville, there are 10 cases of lung cancer. We construct our two-by-two table using the information we were given. This time, for our exposure status rows, we have SpringGield for the exposed and Shelbyville for the unexposed. We calculate the incidence rate for each city. SpringGield has an incidence rate of 20% because 20 out of 100 residents developed lung cancer. Shelbyville's number is halved because only 10 out of 100 residents were diagnosed with lung cancer. In this scenario, because our exposure status is basically being a resident in either SpringGield or Shelbyville, we can calculate the relative risk for each city. Let's start with SpringGield. What is the relative risk for lung cancer among SpringGield residents? We would have the incidence of SpringGield in the numerator and the incidence of lung cancer among Shelbyville residents in the denominator. So we plug in 20%/10%, which results in a relative risk of two. Now, let's look at the relative risk from the other city's perspective, with Shelbyville being the exposed group. We have all the numbers we need: 20% incidence in SpringGield and 10% incidence in Shelbyville. In the relative risk formula, we have the incidence of lung cancer among Shelbyville residents in the numerator and the incidence of lung cancer in SpringGield in the denominator. We are going to consider SpringGield as our unexposed group, with a probability of lung cancer among residents being 20%. In comparison, Shelbyville residents have a 10% probability of lung cancer, resulting in a relative risk of 0.5. This means that there is a twofold greater probability of lung cancer among SpringGield residents compared to Shelbyville residents. However, when looking at it from Shelbyville's perspective, the relative risk is 0.5, indicating a 50% reduction in risk of lung cancer for Shelbyville residents compared to SpringGield residents. To interpret this as a reduction in risk, we can calculate the percentage by subtracting the relative risk from 1. For example, a relative risk of 0.7 would result in a 30% reduction in risk for Shelbyville residents. It is important to remember to subtract the relative risk from 1 when interpreting as a reduction in risk. Alternatively, we can state that residents of Shelbyville have 0.5 times the risk of lung cancer compared to residents of SpringGield. Both interpretations are correct. Now, let's calculate the relative risk for the research question provided. The study aimed to investigate the association between coffee consumption and anxiety in a population-based sample of adults. Among 10,000 coffee drinkers, 500 developed anxiety, while among 20,000 non-coffee drinkers, 200 developed anxiety. This results in a relative risk of 5, indicating a positive association between coffee consumption and anxiety. However, it is important to note that this result may not be statistically signiGicant as our sample population may not accurately represent the target population. We will consider SpringGield as our control group, with a 20% probability of lung cancer among its residents. In comparison, Shelbyville residents have a 10% probability of lung cancer, resulting in a relative risk of 0.5. This means that SpringGield residents have twice the probability of developing lung cancer compared to Shelbyville residents. However, from Shelbyville's perspective, the relative risk is 0.5, indicating a 50% lower risk of lung cancer for its residents compared to those in SpringGield. To interpret this as a decrease in risk, we can calculate the percentage by subtracting the relative risk from 1. For example, a relative risk of 0.7 would result in a 30% decrease in risk for Shelbyville residents. It is important to remember to subtract the relative risk from 1 when interpreting a decrease in risk. Alternatively, we can state that residents of Shelbyville have half the risk of developing lung cancer compared to residents of SpringGield. Both interpretations are correct. Now, let's calculate the relative risk for the research question provided. The study aimed to investigate the association between coffee consumption and anxiety in a population-based sample of adults. Among 10,000 coffee drinkers, 500 developed anxiety, while among 20,000 non-coffee drinkers, 200 developed anxiety. This results in a relative risk of 5, indicating a positive association between coffee consumption and anxiety. However, it is important to note that this result may not be statistically signiGicant as our sample population may not accurately represent the target population.