Lecture 7 - Decision Making For eHealth PDF
Document Details
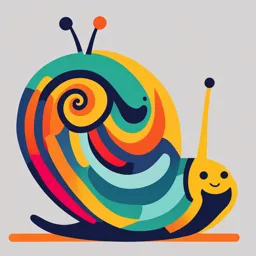
Uploaded by DefeatedRomanArt
Tags
Related
- Lecture 4 - Data Mining for eHealth c64f6c21d36348138a2a7a4e48b2771e.pdf
- Kapitel 18: eHealth und mHealth: Gesundheitskommunikation online und mobil PDF
- eHealth und mHealth: Gesundheitskommunikation online und mobil PDF
- eHealth und mHealth: Gesundheitskommunikation online und mobil PDF
- eHealth: Gesundheits-Apps und digitale Angebote PDF
- Final Term 4 eHealth PDF
Summary
This lecture discusses decision-making in eHealth, focusing on decision making under uncertainty, Bayes' theorem, and the diagnostic process. It highlights the importance of probability in medicine and how imperfect clinical data influences diagnostic testing.
Full Transcript
Lecture 7 - Decision Making for eHealth Outline Decision Making Under Uncertainty Bayes’ Theorem Diagnostic Process Decision Making Under Uncertainty Importance of probability in medical decision making: ”… good medicine does not consist in the indiscriminate application of laboratory examinations t...
Lecture 7 - Decision Making for eHealth Outline Decision Making Under Uncertainty Bayes’ Theorem Diagnostic Process Decision Making Under Uncertainty Importance of probability in medical decision making: ”… good medicine does not consist in the indiscriminate application of laboratory examinations to a patient, but rather in having so clear a comprehension of the probability and possibilities of a case to know what tests may be expected to give information of value” - Peabody, 2001 p.81 Clinical data is imperfect Degree if imperfection varies, but all clinical data - including all the results of diagnostic tests, the history given by the patient, and the findings on physical examination - are uncertain. Errors in Diagnostic Tests Lecture 7 - Decision Making for eHealth 1 Example You are the director of a large urban blood bank. All potential blood donors are tested to ensure they are not infected with human immunodeficiency virus (HIV), the causative agent of acquired immunodeficiency syndrome (AIDS). 1% of the general population is considered to be infected. You can ask whether the polymerase chain reaction (PCR), a geneamplification technique that can diagnose HIV, would be useful to identify people who have HIV. The PCR test is positive 99% of the time when the antibody is present (when the person has the disease), and is negative 99% of the time the antibody is absent (then the person does not have the disease) Bayes’ Theorem Lecture 7 - Decision Making for eHealth 2 Consider the following example. Let's say we use the PCR test on a random person from the general population. Given the statistics provided, we know that 1% of the population has HIV. The PCR test will correctly identify 99% of these individuals (true positives). However, the PCR test will also incorrectly identify 1% of the healthy population as having HIV (false positives). Using Bayes' theorem, we can calculate the probability that a person actually has HIV given that they have a positive PCR test. Let's denote the event of having HIV as H and the event of having a positive PCR test as P. We want to find P(H|P). According to Bayes' theorem: Lecture 7 - Decision Making for eHealth 3 P(H|P) = [P(P|H) * P(H)] / P(P). We know that: P(P|H) = 0.99 (the probability of a positive test given that the person has HIV), P(H) = 0.01 (the probability of a person having HIV), and P(P) is the total probability of a positive test which can be calculated as the sum of the probability of a true positive and the probability of a false positive, i.e., P(P) = P(P and H) + P(P and not H) = (0.99 * 0.01) + (0.01 * 0.99). Substituting these values into Bayes' theorem gives us the probability that a person actually has HIV given a positive PCR test. Continuing from the previous calculation, P(H|P) = [P(P|H) * P(H)] / P(P) = [0.99 * 0.01] / [(0.99 * 0.01) + (0.01 * 0.99)]. After doing the math, we get: P(H|P) = 0.5 This suggests that if a person tests positive for HIV using the PCR test, there is a 50% chance that they actually have HIV, despite the fact that the PCR test is 99% accurate. This surprising result is due to the relatively low prevalence of HIV in the population (1%). This example demonstrates the importance of considering prior probabilities (like the prevalence of a condition in a population) when interpreting diagnostic test results. Prior probability (prevalence) of an event in the population is very important Symptoms and published research results can be used to place patient into a clinical subgroup when prevalence of the disease in the subgroup is known Diagnostic Process 3 Stages First stage involved making an initial judgement about whether a patient is likely to have a disease (prior or pretest probability) Lecture 7 - Decision Making for eHealth 4 Based on epidemiology and experience Using decision support systems Prior probability is the P(A) term in Bayes’ formula Second stage involved gathering more information, often by performing a diagnostic test Aims to reduce the uncertainty Reliability of the test (i.e. sensitivity and specificity) is important Opportunity for computational methods and eHealth! Third stage is to update the initial (prior) probability estimate Find the posterior probability (Bayes’ theorem) Ideally the combination of prior probability with test results will generate a high posterior probability This is the P(A|B) term in Bayes’ formula Remarks If the prior probability is very low, a positive test result can raise the posterior probability only into the intermediate range Hence it is unlikely that a positive test result will raise the probability of disease sufficiently to make a diagnosis with increased confidence Lecture 7 - Decision Making for eHealth 5 1. A positive test result increases the probability of disease 2. Test 2 reduces uncertainty about the presence of disease (increases the probability of disease) more than test 1 If the prior probability is unreliable, Bayes’ theorem will be of little value Bayes’ theorem assumes that two tests are conditionally independent If the theorem is applied sequentially in situations in which conditional independence is violated, you will obtain inaccurate posterior probabilities Lecture 7 - Decision Making for eHealth 6