Lecture 5-Biostatistics(2) PDF
Document Details
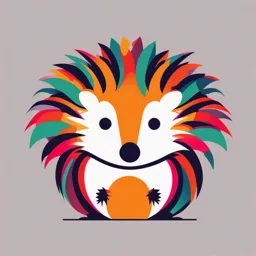
Uploaded by LucidEcoArt
Prof. Stier
Tags
Summary
This is a lecture on biostatistics, covering topics such as learning objectives, important terms, types of data, data collection, continuous vs discrete variables, scales of measurement, and more.
Full Transcript
BIOSTATISTICS Prof. Stier LEARNING OBJECTIVES Upon completion of lectures, class discussions and assigned readings, the student will be able to: 1. Explain the term “standard deviation” and relate the deviations to the mean of a bell curve. 2. Describe three measures of central tendency in...
BIOSTATISTICS Prof. Stier LEARNING OBJECTIVES Upon completion of lectures, class discussions and assigned readings, the student will be able to: 1. Explain the term “standard deviation” and relate the deviations to the mean of a bell curve. 2. Describe three measures of central tendency in common use. 3. Explain how statistics yield probability and not certainty. 4. Given a graph, accurately interpret the information, and give examples of each. 5. Calculate the mean, median, mode, variance range and standard deviation. 6. Formulate a null hypothesis and an alternative hypothesis in a given experimental simulation. 7. Select appropriate inferential statistical tests for various types of data. BIOSTATISTICS The field of Statistics is simply a standardization of the collection, organization and analysis of any quantitative data. The goal of Statistics is to predict the value of the population by gathering data from the sample. Statistical methods are useful tools for compiling large amounts of information that is understandable. IMPORTANT TERMS Population: Any entire group of items that possesses one define characteristic in common. Sample: Representative portion of the group/population Parameter: A characteristic of the group/population Statistic: Characteristic of the sample TYPES OF DATA QUANTITATIV QUALITATIVE CONTINUOU DISCRETE E DATA DATA S VARIABLE VARIABLE QUANTITATIVE DATA Represented by numbers Expressed as counts, percentages, and means Examples: Pocket depths, number of sealed teeth, number of communities with water fluoridation QUALITATIVE DATA Information that reflects the quality or nature of variables that cannot be expressed numerically Expressed as outcomes, or states, and can be counted for reporting Variables can be rank ordered Example: Patient responses to surveys DATA COLLECTION Testing a specific hypothesis or solving a particular research problem requires the collection of specific types and forms of data. To test the hypothesis, data collected needs to be quantified into numerical forms, which are classified into continuous or discrete variables. What is a variable? State, condition, concept, construct, or event whose value is free to vary CONTINUOUS VS. DISCRETE VARIABLES Continuous Variable: Capable of any degree of measurement along a linear scale Examples: Age, weight, time Discrete Variable: Counted only in terms of whole numbers Examples: sex, marital status, number of patients treated, DMFT (expressed in whole numbers because one cannot have a fraction of a tooth) CATEGORIZING DATA (ORGANIZING DATA) Scales of Measurement Nomina Ordinal Interval Ratio l Scale Scale Scale Scale NOMINAL SCALE Used to organize data collections into mutually exclusive categories Example Categorizing individuals in the profession of dentistry: Dental assistants, RDH, dental technicians, dentists The purpose of a nominal scale for measuring variable is to identify relationships which exist between characteristics No rank order/value No numeric relationship Other examples: Republicans/Democrats/Independents Yes/No/Undecided ORDINAL SCALE Used to organize data collections into mutually exclusive categories Rank is based on some criterion with no difference between ranks Example: Patient classification in clinic: L/M/H There is an algorithm to determine what it is, but there isn’t a numerically equivalent difference between L M and H Patient satisfaction survey: More positive responses could be considered higher, but it’s not expressed numerically INTERVAL SCALE Measures predetermined equivalent intervals as well as the rank order of the variable measured Has NO absolute zero, meaning that the zero point is arbitrarily established, not deemed by nature (arbitrary zero) Example: Fahrenheit scale: A one-degree difference is numerically equal all along the scale. Because there is an arbitrary zero, we can’t say that one temperature is twice as hot as another, but can assume that it is warmer RATIO SCALE Contains all the characteristics of the nominal, ordinal and interval scales, in addition to absolute zero. Example: weight: a person who weighs 200 lbs is twice as heavy as someone who weighs 100 lbs Numbers on the scale may be added, subtracted, multiplied and divided. DESCRIPTIVE VS. INFERENTIAL STATISTICS Descriptive Statistics: procedures that are used to summarize, organize and describe quantitative data. Includes: measures of central tendency, measures of dispersion, frequency distribution tables, and graphing techniques Inferential Statistics: make generalizations based on a sample of data DESCRIPTIVE STATISTICS Measures of Central Tendency (Averages) Single number that tells us where the center of data is located May be used to depict typical performance for a group The word “average” is rarely used One of three measures of central tendency are used Mean Median Mode DESCRIPTIVE STATISTICS--MEAN Mean (X) The sum total of all the observations divided by the number (N) of observations Most are familiar with it Always exists Always unique Takes into account each individual item Possible to combine means of different reports Useful in advanced statistics 1, 2, 3, 4, 5 Mean is 3 DESCRIPTIVE STATISTICS--MEDIAN Median The point on the scale of observations which is in the middle, with half occurring on one side of this point and the other half of the observations falling on the other side Put in numerical order 1, 2, 3, 4, 5 Median is 3 1, 2, 3, 4 Median is 2.5 DESCRIPTIVE STATISTICS--MODE Mode Most frequently occurring observation Can apply to categories as well as to numerical values Requires no calculation If it exists, it’s not always unique May not be representative of anything Rarely utilized 1, 2, 3, 3, 4, 5 Mode is 3 1, 2, 2, 3, 3, 4, 5 Mode is 2 and 3 MEASURE OF DISPERSION Range Variance Standard Deviation RANGE The difference between the largest and smallest numbers or scores in a distribution Although range is easy to calculate, it is unstable since it is affected by variables (extreme scores) and by itself it is not important. Example: 100-80=20 Least useful measure of dispersion, but can give a quick snapshot VARIANCE Measure of variability that indicates how far the scores are from the mean A group of observations may have the same mean yet appear very different on a graph If the observations are distant from the mean, the measures of dispersion of variance will be greater than if observations are closer to the mean Tells us how much variability there is in a data set STANDARD DEVIATION Averages how far each score deviated from the mean. The greater the variance of scores from the mean, the greater the standard deviation A mean is the point along a scale of values, while the standard deviation is a distance along the scale In a small deviation the scores are bunched together Also called “Z Score” STANDARD DEVIATION To do a standard deviation 1. Obtain the mean of the distribution 2. Subtract the mean from each score (that will be the deviation) 3. Square each deviation 4. Add all the squared deviations 5. Divide the sum by the number of cases minus 1 (that will be the variance) 6. Get the square root of the answer (that will be the standard deviation) DMFT Scores Deviation Squared Variance Standard Deviation 3 -7 -4 Deviation 5 -7 -2 16 7 -7 0 4 9 -7 2 0 11 -7 4 4 Sum=35 16 40 ÷ ö √10 Mean=7 Sum = 40 4=10 NORMAL DISTRIBUTION Bell Curve A normal distribution is bell-shaped and symmetrical to either side of the mean, with the mean and median being equal, that is 50% of the sample scores lie above the mean and 50% lie below. Distribution of the scores falls within the mathematical calculation that 68% of the scores will fall within one standard deviation of the mean (plus of minus) 99.5% of scores will fall between + 2 and –2s 99.7% of scores will fall between + 3 and –3s The importance of these probabilities are important in more complicated hypothesis testing WHAT PERCENTAGE OF STUDENTS GOT A C ON THE EXAM? LEFT-SKEWED BELL CURVE (NEGATIVE) RIGHT-SKEWED BELL CURVE (POSITIVE) WAYS TO PRESENT DATA Statistical data is frequently presented by tables, graphs and diagrams. These are basic descriptive devices which display large amounts of data. The purpose is to organize data in a clear and simple fashion. When presented with a set of raw data, the reader can usually get only a vague impression from glancing over the data. This especially is true when shown a large data set. Visual simplification is essential for a clearer understanding. WAYS TO PRESENT DATA Frequency Distribution Pictorial or graphical representations Histogram Bar Diagram Frequency Polygon Pie Diagram FREQUENCY DISTRIBUTION Common method for organizing continuous data In the first column, the variable is grouped into intervals which are equal whenever possible The second column describes the frequency of this grouping (how much data were included in that category The basic steps in constructing a frequency table: 1. Choose the classes into which the data will be grouped 2. Sort the data into these categories 3. Counting or tallying the number of items in each group. FREQUENCY DISTRIBUTION The frequency distribution in table 1 has grouped the scares in intervals of five points. Generally, the number of categories (70-74, 75-79, etc) should range from about 6-15 and whenever possible, the class intervals should be of equal length Every piece of data must fit into a category and there should be no overlap between categories, so that each item is tallied only once Scores on quiz: 72, 75, 76, 81, 84, 84, 84, 91, 98, 100 Table 1 Ranges of scores on Quiz How many scores fall into range 95-100 2 90-94 1 85-89 0 80-84 4 75-79 2 70-74 1 Total 10 PICTORIAL OR GRAPHICAL REPRESENTATIONS OF DATA Graphs reveal at a glance the location and frequency of extreme scores, the shape of the distribution and spread of the scores Graphs are constructed with an X and Y axis. X axis= abscissa and runs horizontally Y axis = runs vertically X-axis is reserved for the scale employed to measure the variable of interest (scores) The Y axis usually reflects the frequency of scores occurring along the scale of measurement (frequencies). INDEPENDENT VS. DEPENDENT VARIABLES Independent variable Condition of the experiment that is manipulated or controlled by the investigator In a non-experimental study, it is the factor(s) studied to explain or predict the dependent variable or outcome of interest Dependent variable Measure thought to change as a result of the presence, absence, or manipulation of the independent variable HISTOGRAM A bar graph which pictorializes frequency distribution to facilitate visualization of the data Generally the graph proceeds from left to right, or bottom to top The X axis represents the first column which is plotted against the frequency of the Y axis Quantitative Data on both X and Y Axis BAR GRAPH/DIAGRAM Similar to the histogram (usually vertical) but lines do not touch Categorical Data onHygienist’s X-axis Salary: $35/Hour; 40 Hours a Week $5600/month; $3920 After Taxes Bills: 1250 1000 750 Series1 500 250 0 rent loan groc utiliti cabl phon cell car insur metr cloth Spe savi s eries es e e phon ance ocar es ndin ngs e d g$ FREQUENCY POLYGON A graph which is constructed by plotting the points which correspond to the representation of a frequency distribution on both axes The points are then connected by means of straight lines Preferred when comparing two groups on one graph 1250 1000 750 Series1 500 250 0 rent loan groc utiliti cabl phon cell car insur metr cloth Spe savi s eries es e e phon ance ocar es ndin ngs e d g$ PIE DIAGRAM Another useful form of a diagram, which again usually enhances the reader's perception of the material Must have finite numbers Helpful when comparing proportions Very illustrative but may not always be accurate Allows for rapid interpretation The whole circle adds up to 100% PIE DIAGRAM The most effective presentation of data would be: Simple: Somewhere between 5-15 classes/groupings Relatively self-explanatory Doesn’t require tedious interpretation Dental Hygienist Monthly Expenses rent loans Completely labeled groceries utilities cable phone cell phone car insurance metrocard clothes Spending $ savings CORRELATION r A number which indicates the strength (or degree) and direction of the relationship between two sets of variables. The numbers which are possible as coefficients of correlation range from +1 through -1 to +1. There are three possible ways in which two variables may be correlated: Positive correlation: As one set of scores increases, the other set of scores increases correspondingly. ie/. Perio disease and heart disease Negative correlation: The variables vary in opposite directions. As one increases in value, the other decreases. (Inverse relationship) No correlation CORRELATION Source: www.thoughtco.c STRENGTH OF ASSOCIATION Value of r Strength of Association 0.00-0.25 Little if any 0.26-0.49 Weak 0.50-0.69 Moderate 0.70-0.89 High 0.90-1.00 Very high CORRELATION/CAUSALITY Correlation doesn’t always = causation Cause and effect relationships not definitive Causality A certain exposure WILL result in a particular outcome Causality depends on the strength of the association of variables, consistency, specificity, plausibility, etc. Example: Increased drowning deaths in the summer and increased consumption of ice cream in the summer. Each may increase, but one doesn’t cause the other HYPOTHESIS TESTING Types of Hypotheses: Null hypothesis Alternative hypothesis Null Hypothesis Most frequently used in research Stated in the negative “There is NO difference” Namely, the hypothesis is true Alternative Hypothesis The logical opposite of the null hypothesis Stated in the affirmative Mutually excusive and direct opposite of null, in that it stats the there IS a different Rejection of the null hypothesis is the same as acceptance of the alternative THE “P” VALUE The statistical decision to reject or accept the null hypothesis is based on probability at a set significance level (also known as the alpha level) It is expressed as a probability value or p value or the probability of findings from a study are due to chance. Most common value is p is ≤ to 0.05 TYPE 1 AND TYPE 2 ERRORS A type 1 error occurs when the null hypothesis is rejected but it is actually true and should have been accepted A type 2 error occurs when the null hypothesis is accepted, but it is actually false and should have been rejected RELIABILITY VS. VALIDITY Reliability Quality of measurement methods that suggests that the same data would have been collected each time in repeated observations of the same phenomenon Concerned with questions of stability and consistency Does the same measurement tool result in stable and consistent results when repeated over time? Validity A measure that accurately reflects the concept it is intended to measure Extent to which we are measuring what we hope to measure (and what we think we are measuring). REGRESSION ANALYSIS Used to quantify the relationship between two variables It expresses the functional relationship between the variables. Used to predict the score of one variable –dependent variable (y) based on the score of the independent variable (x). Gives the strength of the ability of two or more variables (independent) to predict another (dependent) CONFIDENCE INTERVALS Intended to generalize between the sample being studied and the population How confident are you? Confidence Interval: A statistical technique used to infer the true value of an unknown population parameter. Example: If the 95% percent CI for the mean plaque index of 1.16 in a representative sample of school children is 1.08-1.24, we can be 95 percent certain that the mean plaque index of the population was 1.08-1.24 PARAMETRIC STATISTICS Assumptions for Use of Parametric Statistics: Data are continuous Adequate sample size is used Population distribution is normal Group variances are equal T-Tests (“Tea for Two” Used to compare TWO mean scores to determine whether a statistically significant difference exists ANOVA Used when more than two means are being analyzed NON-PARAMETRIC TESTS Used when variables are discrete, sample size is small, population distributions are not normal, or group variances are not equal. Chi- Square Used to determine whether a difference exists been frequency counts of nominal (categorical or dichotomous) data by comparing observed to expected frequencies Other Nonparametric Tests- Fisher test, McNemar’s Test, Mann- Whitney QUESTION 1 In a study with the following hypothesis, “Powered toothbrushes will remove more extrinsic stain than a manual toothbrush,” what is the dependent variable? A. Powered toothbrush B. Manual toothbrush C. Extrinsic stain removal QUESTION 1 In a study with the following hypothesis, “Powered toothbrushes will remove more extrinsic stain than a manual toothbrush,” what is the dependent variable? A. Powered toothbrush B. Manual toothbrush C. Extrinsic stain removal QUESTION 2 In a correlational study regarding patterns of chocolate consumption and decay rates, the researchers found a +.2 correlation between frequency of chocolate ingestion and DMF rates. Which of the following can the authors conclude? A. Eating chocolate causes caries B. There is a weak positive relationship between the frequency of chocolate and caries C. There is a strong positive relationship between the frequency of eating chocolate and caries. D. There is no relationship between the frequency of eating chocolate and caries. QUESTION 2 In a correlational study regarding patterns of chocolate consumption and decay rates, the researchers found a +.2 correlation between frequency of chocolate ingestion and DMF rates. Which of the following can the authors conclude? A. Eating chocolate causes caries B. There is a weak positive relationship between the frequency of chocolate and caries C. There is a strong positive relationship between the frequency of eating chocolate and caries. D. There is no relationship between the frequency of eating chocolate and caries. QUESTION 3 Which of the following defines the reliability of an instrument? A reliable instrument A. Consistently measures the same way in multiple attempts B. Thoroughly measures the content area C. Accurately measures the content in relation to an existing instrument D. All of the above QUESTION 3 Which of the following defines the reliability of an instrument? A reliable instrument A. Consistently measures the same way in multiple attempts B. Thoroughly measures the content area C. Accurately measures the content in relation to an existing instrument D. All of the above QUESTION 4 Using the following data set: 1,3, 5,5,5,5,8,9,9, and 10 What is the mode for this data set? A. 1 B. 5 C. 6 D. 9 QUESTION 4 Using the following data set: 1,3, 5,5,5,5,8,9,9, and 10 What is the mode for this data set? A. 1 B. 5 C. 6 D. 9 QUESTION 5 Using the following data set: 1,3, 5,5,5,5,8,9,9, and 10 Which of the following defines the mean score? A. Sum of scores B. Sum of scores divided by the total number C. Most frequently occurring number D. Central point within the distribution QUESTION 5 Using the following data set: 1,3, 5,5,5,5,8,9,9, and 10 Which of the following defines the mean score? A. Sum of scores B. Sum of scores divided by the total number C. Most frequently occurring number D. Central point within the distribution QUESTION 6 Using the following data set: 1,3, 5,5,5,5,8,9,9, and 10 What is the mean score for this data set? A. 1 B. 5 C. 6 D. 9 QUESTION 6 Using the following data set: 1,3, 5,5,5,5,8,9,9, and 10 What is the mean score for this data set? A. 1 B. 5 C. 6 D. 9