Judgment and Decision Making PDF
Document Details
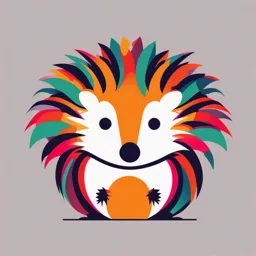
Uploaded by WellBredTurtle345
Adam Osth
Tags
Summary
This document is a set of lecture notes for a psychology course on judgment and decision-making. It covers topics including heuristics like availability and representativeness, and common cognitive biases such as loss aversion, framing effects, and base-rate neglect. The notes use examples, questions, and studies to illustrate the concepts.
Full Transcript
PSYC20007 Judgment and Decision Making Adam Osth Enlightenment (18th and 19th century) Views on Judgment and Decision Making The “Rational Man” or ”Economic Man”: Enlightenment thinkers viewed humans as making rational decisions In legal contexts: weighing up whether a...
PSYC20007 Judgment and Decision Making Adam Osth Enlightenment (18th and 19th century) Views on Judgment and Decision Making The “Rational Man” or ”Economic Man”: Enlightenment thinkers viewed humans as making rational decisions In legal contexts: weighing up whether a defendant is guilty should be compared against what a “rational man” would do in the circumstances Current Thinking We now know there are many ways in which humans make poor decisions or behave differently from a rational expected outcome Humans rely often rely on heuristics Simple shortcuts that lead to approximate solutions which can be wrong to varying degrees Simple example: If asked “What’s 10.5 * 9.8?” we can simplify this to 10 * 10 and say it’s ”around 100” Why use them? Less time and resources consumed Humans subject to biases Systematic errors in our judgments or evaluations Current Thinking An underlying theme to the lecture – we are extremely bad at assigning probability to events! As a substitute, we often use simpler cognitive operations to come up with a “best guess” as to what is happening This can be accurate in some cases and extremely misleading in others Example Question A bat and ball cost $1.10. The bat costs $1.00 more than the ball. How much does the ball cost? cents 5 Much of this work was done by Amos Tversky and Daniel Kahneman “I would say, without hesitation, if somebody were to ask me what are the most important contributions to human life from psychology, I would identify this work as maybe number one, and certainly in the top two or three. In fact, I would identify the work on reasoning as one of the most important things that we've learned about anywhere. I Amos Tversky argued at Harvard that when we were trying to identify 1937 – 1996 what should any educated person should know in the entire expanse of knowledge, I argued unsuccessfully that the work on human cognition and probabilistic reason should be up there as one of the first things any educated person should know. I am unqualified in my respect for how important this work is.” - Steven Pinker Daniel Kahneman Nobel Prize in Economics 2002 Forebears: Herbert Simon Bounded rationality: Humans reason and choose rationally, but only within the constraints imposed by their limited search and computational capacities. Satisficing: “[U]sing experience to construct an expectation of how good a solution we might reasonably achieve, and halting search as soon as a solution is reached that meets the expectation” Herbert Simon (1916- (Simon, 1990) 2001) 7 Forebears: Paul Meehl Two major findings from Meehl (1954): 1. Clinical prediction (e.g., predictions by clinicians) performs very poorly relative to statistical prediction (e.g., regression models). 2. Clinical prediction overweights case characteristics and underweights base rates. 8 Some questions! Which are more prevalent in English: words that begin with the letter “r” or words in which “r” is in the third letter position? Words with “r” in the third position are considerably more frequent! So why do we think it’s the first option? Words with “r” in the first position come to mind easily (they are easily retrieved from memory) “Rooster”, “red”, “rabbit”, “rollercoaster”, etc. Harder to think of words with “r” in the third position – “arrange”, “correspond”, “car” Some questions! Some causes of death are listed below in pairs. Which cause of death is more frequent? Homicide or appendicitis? Auto-train collision or drowning? Botulism or asthma? Asthma or tornado? Appendictis or pregnancy? Some questions! Some causes of death are listed below in pairs. Which cause of death is more frequent? Homicide or appendicitis? (91% of people think homicide) Auto-train collision or drowning? (66% of people think auto- train collision) Botulism or asthma? Asthma or tornado? Appendictis or pregnancy? Availability Heuristic (Tversky & Kahneman, 1973) Events that come to mind more easily are judged as being more probable than events that are less easily recalled Further tests in the experiments of Tversky and Kahneman Subjects studied lists of ordinary names and celebrity names. They studied both male and female names – the proportions of each were manipulated across the ordinary and celebrity categories but the underlying proportionof male and female names was 50/50 During the memory test, subjects had to judge how frequent the male or female names were Subjects gave higher frequency estimates to whichever gender had the most celebrity names E.g., if there were more celebrity male names, but less ordinary male names, subjects estimated that there were more male names Availability Heuristic (Tversky & Kahneman, 1973) The availability heuristic can lead to some very misleading consequences when we estimate probability Certain rare events that get a lot of media attention – these often come to mind when we estimate risk of danger Deaths due to vaccinations: highly publicized, yet extremely rare Deaths from assault weapons: attacks using assault weapons are quite rare – handgun violence is considerably more frequent Deaths from airplane crashes: deaths from car crashes are much more frequent Another example of availability… Tversky and Kahneman (1974) discuss a study by Chapman and Chapman that illustrates how availability can lead to an illusory correlation Illusory correlations are correlations that do not exist, but are believed to exist In this study, subjects were presented with photos of patients with various clinical diagnoses They were then asked which features of the faces indicated the clinical diagnoses Subjects claimed that patients with clinical diagnoses often had features like peculiar eyes, even if there was a negative correlation between the feature and the diagnosis in the photos Another example of availability… How is this an example of availability? Tversky and Kahneman argue that subjects are recalling a prior association between peculiar eyes and clinical disorders and using this to guide their impressions Why do we use the availability heuristic? Because we often do not know the true proportions or probabilities! Instead, we approximate these quantities by retrieving examples from memory E.g., estimating proportions of male/female names – we recall instances from the category, and if we can recall more male names we think that’s what is predominant More on the availability heuristic… As soon as we use our memories to assign judgments or probabilities, we are subject to memory limitations Tversky and Kahneman (1974) discuss how limitations of the search set influence our judgments If we are unable to retrieve examples during memory search, we cannot assign high probabilities This is why we assign higher frequencies to words that begin with “r” compared to words with “r” in the third position – we have a harder time retrieving examples of the latter Some questions, again! Tom is a university student. He has glasses, is highly introverted, and spends a lot of time on his computer. Which is Tom more likely to be – a computer science major, or a psychology major? Subjects in experiments often select “computer science major” And yet… psychology majors are considerably more frequent on university campuses! Another example When told “In a group of 100 people, there are 70 lawyers and 30 engineers. What are the chances that if we pick one person from the group at random that the person will be an engineer?” Subjects are correct in picking lawyer However, if they given in addition: “Jack is a 45 year old man. He is married and has four children. He is generally conservative, careful, and ambitious. He shows no interest in political and social issues and spends most of his free time on his many hobbies, which include home carpentry, sailing, and mathematical puzzles.” Subjects tend to assume that Jack is an engineer, despite the fact that lawyers considerably outnumber engineers The description given outweighs the job frequencies in people’s judgments! The Representativeness Heuristic (Tversky & Kahneman, 1974) We make judgments on the basis of resemblance In the previous example, instead of asking “How likely is it that Jack is an engineer?” …we instead ask “How much does Jack resemble an engineer?” A related concept… Previous question was an example of base rate neglect “Base rate”: relative frequency of something in the populations We frequently ignore base rates when making assessments and use other information to make our decision (e.g., representativeness) This can have major consequences! When clinical prediction is worse than statistical prediction… Doctors previously would ignore base rates when making assessments about diseases – symptomology might match a condition that is extremely rare E.g., if a young person shows symptoms of stomach cancer (pain in gut, bloody stools, etc.), it is much more likely to be a benign condition More on base rate neglect It’s not as if we can’t use base rates! Subjects correctly use base rates when there is no description in the problem When told there are 70/30 proportions of lawyers and engineers, subjects correctly use this information and estimate that there are more lawyers …but we ignore it even when there is a description given, even if there is no information in that description! When given a description like: “Dick is a 30 year old man. He is married with no children. A man of high ability and high motivation, he promises to be quite successful in his field. He is well liked by his colleagues.” Subjects estimate there is a 50/50 chance of him being a Okay, okay… Even when we ignore base rates, isn’t it possible that we are actually being rational? Other information in the description might be more useful than the base rates Another question regarding representativeness… ”Linda is 31 years old, single, outspoken, and very bright. She majored in philosophy. As a student, she was deeply concerned with issues of discrimination and social justice, and she also participanted in antinuclear demonstrations. Which of the following alternatives is more probable?” Linda is a bank telleter Linda is a bank teller AND is active in the feminist movement Subjects choose the second option much more frequently than the first Why is that not rational? A higher likelihood of Linda being a feminist *and* a bank teller is a violation of the conjunction rule of probability The probability of two events together (A & B) can never be higher than the probabilities of the individual events (A or B). Some probability theory basics: If A and B are completely independent of each other (no correlation), then the probability of A&B together is the probability of A multiplied by the probability of B Probabilities get smaller when you multiply them! So this will be a small quantity Conjunctions of many independent events – probabilities get close to zero Another violation of the conjunction rule Suppose Bjorn Borg reaches the Wimbledon finals in 1981. Please rank order the following outcomes from most to least likely: A. Borg will win the match (1.7) B. Borg will lose the first set (2.7) C. Borg will lose the first set but win the match (2.2) D. Borg will win the first set but lose the match (3.5) (Tversky & Kahneman, 1983) But Borg losing the match alone is truly the more likely outcome! Losing the match AND winning the first set is less likely by definition Why do we use the represenatativeness heuristic? Again, we’re bad at estimating probability or correctly weighing prior probabilities with new information! Tversky and Kahneman argue that we substitute for that with a simpler cognitive operation: resemblance! In other words, we ask: “How much does this description look like the answers I’m given?” instead of asking ”What is the probability that this person fits this occupation?” Another question about probability estimation “A certain town is served by two hospitals. In the larger hospital, about 45 babies are born each day, and in the smaller hospital, about 15 babies are born each day. As you know, about 50 percent of all babies are boys. However, the exact percentage varies from day to day. Sometimes it may be higher than 50 percent, sometimes lower. For a period of 1 year, each hospital recorded the days on which more than 60 percent of the babies born were boys. Which hospital do you think recorded such days?” The larger hospital? The smaller hospital? About the same? The answer is the smaller hospital Why the smaller hospital? The law of large numbers The larger the sample, the more likely it will represent the population The “true” probability is 50% - so larger samples will be closer to 50% than smaller ones An example with guessing coin flips: It’s not hard to have 75% accuracy with just four guesses It’s very hard to have 75% accuracy with four hundred guesses! We’re pretty bad at understanding even the very concept of probability! The Drunkard’s Walk: a history of probability theory The Ancient Greeks mastered geometry and even understood calculus, but had no concept of probability In one of their games of chance, the highest scoring outcome wasn’t the rarest! It took a long portion of human history just to formalize the coin flip Jacob Bernoulli in the 16th century formalized the Bernoulli distribution Even expert mathematicians often make some egregious errors when it comes to probability and statistics The Anchoring Heuristic When having to estimate a quantity, we always do it with an intuitive reference to something else That reference point is called the anchor Example: when asked what the population of a country we are unfamiliar with is (say, Cameroon), we may use a similar sized country as a reference point to make our judgment The Anchoring Heuristic Tversky and Kahneman (1974): even random numbers can serve as anchors! In one study, subjects were asked to estimate the proportion of African nations in the UN They were given a random number from a spinning wheel. What the subjects did not know is that the wheel only returned 10 or 65 10 group: their median estimates of African nations was 25 65 group: their median estimates of African nations was 45 Even payoffs for correct responses did not alter the effect! Why do we use these random anchors? Again, we don’t know the true frequencies or probabilities, and we need a reference point to make our decisions! Possibility that the number remains in memory due to its recency E.g., we know from many memory studies that recent information is highly available and accessible Another question Imagine that the United States is preparing for the outbreak of an unusual disease that is expected to kill 600 people. Two alternative programs to combat the disease have been proposed. Assume that the exact scientific estimates of the consequences of the programs are as follows: If program A is adopted, 200 people will be saved. If program B is adopted, there is a 1/3 probability that 600 people will be saved, and a 2/3 probability that no people will be saved. Which of the two programs would you favor? 72% of subjects chose Program A Another question Same question as last slide, but different programs: If program C is adopted, 400 people will die. If program D is adopted, there is a 1/3 probability that nobody will die, and a 2/3 probability that 600 people will die. Which of the two programs would you favor? 78% of subjects chose Program D Framing Effects Here’s the crazy thing: both sets of programs lead to the same outcomes! Program A = Program C, Program B = Program D Because there are only 600 people, “saving 200” is the same as “400 dying” Framing effects Framing in terms of gains makes subjects prefer risk aversion – going with a sure bet that will avoid losses Framing in terms of losses makes subjects prefer risk taking – preferring options that will lead to greater gains despite the risk involved Loss Aversion The previous framing effects illustrate a more general principle called loss aversion (Kahneman & Tversky, 1979) When we weigh up decision options, potential losses in our minds weigh twice as much as gains How does this relate to risk taking with negative framings? People will prefer risky options if they avoid losses E.g., deciding between programs C and D Program D is chosen because no losses will result Why is loss aversion a big deal? These are highly contrary to expected utility theory Expected utility theory was a standard in economics that argued that we are rational agents that always try to maximize the expected utility What is expected utility? It’s another term for an outcome we desire – if we are attempting to make money, profit could be considered the “utility” in the example According to classical interpretations of expected utility theory, two decisions with the same outcomes should have the same expected utility (e.g., number of people saved) Prospect Theory (Kahneman & Tversky, 1979) The findings of loss aversion and other findings were integrated into a broader theory of how we perceive gains and losses called prospect theory Prospect theory describes a psychometric function A psychometric function is a description of the psychological value or weight given to an objective experience E.g., in psychophysics, we know that the psychometric function of loudness is non-linear and negatively accelerated We require progressively more loudness to perceive an increase in volume Prospect Theory (Kahneman & Tversky, 1979) Prospect theory describes the psychometric function of value in terms of gains and losses S-shaped function: psychological value is on the y-axis, gains versus losses are on the x-axis Losses are weighted more heavily than gains On the figure, we can see that a loss of 5 cents is given a value of -40, whereas a gain of 5 cents is assigned a value of +20 The function is also non-linear As gains or losses increase, they result in progressively smaller changes in value Influence of the heuristics and biases work Influence of the Kahneman and Tversky work Massive!!! The entire field of behavioral economics Understanding the ways individuals evaluate probabilities, assess risks and rewards, and make decisions in the context of economics Prospect theory has been hugely influential Assumption that it’s no longer tenable to assume that individuals always make decisions rationally! Criticisms of the Kahneman and Tversky work Lack of formal modeling Lots of heuristics, but these are applied in post hoc fashion! Sometimes it’s unclear whether something could be described as relying on the availability or representativeness heuristic, for instance Lack of a mechanistic theory But isn’t prospect theory a theory? Prospect theory is a descriptive account of the underlying value function, but it’s unclear as to how we psychologically create those values It describes loss aversion quantitatively, but it does not answer why or how loss aversion actually occurs Criticisms of the Kahneman and Tversky work Heuristic usage often can be a good thing! People run into problems when they use the wrong heuristics, or apply them in the wrong contexts There are many cases where people can make very accurate decisions with little to no knowledge Recognition Heuristic Gigerenzer included an additional heuristic: that when we are unfamiliar with the options, we often choose the one we recognize the most Example question: Which city has more inhabitants: San Antonio or San Diego? Americans: 62% correct Germans: 100% correct Are Germans just better educated about population estimates? Probably not They just pick the city they recognized – in this case it leads them to the correct answer PredictingWimbledon Wimbledon2003 2003 Correct Predictions 72% 69% 70% 68% Amateurs 66% 66% could do as well or better than experts 60% just by betting on names they recognized! 50% ATP ATP Seedings Recognition Recognition Entry Champions Laypeople Amateurs Ranking Race Frings & Serwe (2004) 46 Fast and Frugal Heuristics Gigerenzer and Todd (1999) argue that a hallmark of human decision making is the usage of fast and frugal heuristics Fast – we can use these really quickly and with little decision time Frugal – they don’t require a great deal of computation or consideration. We simplify the information we need for the problem at hand Fast and Frugal Heuristics One such example: simple decision trees used by doctors State of the art statistical algorithms require heavy computation over as many as 19 predictors Any such formal computation with any problem requires converting each consideration to a “common currency” – usually a probability of an event occurring E.g., to predict whether a patient lives or dies, how do we simultaneously consider their weight, their family history, and their symptoms? We have to quantify them on a common scale – usually a probability of survival, so they can be combined Fast and Frugal Heuristics The decision tree on the right is far simpler – it only requires a maximum of three decisions This is easy to perform quickly! While it doesn’t perform as well as the stastical algorithm, it gets “more bang for its buck” – it performs very well considering the little time it requires to execute Does this imply that we always do better when we use heuristics? Heavens, no! Gigerenzer intended these examples as a counterpoint to the Kahneman and Tverksy work While there are many times where heuristics can lead us astray and we can make irrational decisions… …there are other contexts where heuristics can guide us to the correct decisions with little effort or knowledge of the underlying problem The important consideration is whether heuristics are appropriate for the situation at hand The danger is when we are using the wrong heuristics! Formal Theories of Decision Making Increasingly, there are a number of decision making theories that are grounded in retrieval from memory MINERVA-DM: a process model of decision making based on computational models of global similarity (Dougherty, Gettys, & Ogden, 1999) Recall from the false memory lecture: global similarity is a theory of how recognition memory judgments are made! Items are endorsed if they have high global similarity to memory traces Underlying idea is that global similarity of a cue can be converted into the probability of an outcome E.g., ”How common are deaths from plane crashes?” can be assessed by cuing memory with “plane crash” and “death” and evaluating the global similarity – how many memories are consistent with these cues Events have higher probability if there are more memories consistent with said event Formal Theories of Decision Making MINERVA DM, continued The model was able to produce the evidence for availability and representativeness heuristics and overweights rare events, all with a common mechanism This is beyond the scope of the course – it’s included just to convince you that such theories do exist! Learning Outcomes Understand each of the heuristics of Kahneman and Tversky that were discussed in this lecture Understand expected utility theory and how this was challenged by Kahneman and Tversky Know framing effects and loss aversion Understand prospect theory and the phenomena it can explain Understand more generally how rationality in probability estimation has been challenged by the Kahneman and Tversky work Understand the recognition heuristic and the concept of fast and frugal heuristics Understand the motivation behind the Minerva DM model