Cognitive Science: An Introduction to the Study of Mind (PDF)
Document Details
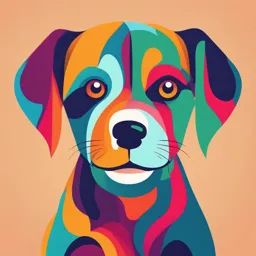
Uploaded by ImpeccableEpiphany1282
null
Jay Friedenberg, Gordon Silverman, Michael J. Spivey
Tags
Summary
This textbook, "Cognitive Science: An Introduction to the Study of Mind," explores the interdisciplinary field of cognitive science. The book covers various approaches to understanding the mind, including philosophical, psychological, neurological, and computational perspectives, presenting a comprehensive overview for students and researchers.
Full Transcript
JAY FRIEDENBERG / GORDON SILVERMAN / MICHAEL JAMES SPIVE Y...
JAY FRIEDENBERG / GORDON SILVERMAN / MICHAEL JAMES SPIVE Y FRIEDENBERG / SILVERMAN / SPIVE Y Teaching isn’t easy. | Learning never ends. We are here for you. COGNITIVE SCIENCE Learn more about SAGE teaching and learning solutions for your course at sagepub.com/collegepublishing. AN INTRODUCTION TO About SAGE THE STUDY OF MIND Founded in 1965, SAGE is a leading independent academic and professional publisher of innovative, high-quality content. Known for our commitment to quality and innovation, SAGE has helped inform and educate a global community of scholars, practitioners, researchers, and students across a broad range of subject areas. Cover image: iStock.com/Just_Super Cognitive Science Fourth Edition Sara Miller McCune founded SAGE Publishing in 1965 to support the dissemination of usable knowledge and educate a global community. SAGE publishes more than 1000 journals and over 600 new books each year, spanning a wide range of subject areas. Our growing selection of library products includes archives, data, case studies and video. SAGE remains majority owned by our founder and after her lifetime will become owned by a charitable trust that secures the company’s continued independence. Los Angeles | London | New Delhi | Singapore | Washington DC | Melbourne Cognitive Science An Introduction to the Study of Mind Fourth Edition Jay Friedenberg Manhattan College Gordon Silverman Manhattan College Michael J. Spivey University of California-Merced FOR INFORMATION: Copyright © 2022 by SAGE Publications, Inc. All rights reserved. Except as permitted by U.S. copyright SAGE Publications, Inc. law, no part of this work may be reproduced or distributed 2455 Teller Road in any form or by any means, or stored in a database or Thousand Oaks, California 91320 retrieval system, without permission in writing from the E-mail: [email protected] publisher. All third-party trademarks referenced or depicted herein SAGE Publications Ltd. are included solely for the purpose of illustration and are 1 Oliver’s Yard the property of their respective owners. Reference to these 55 City Road trademarks in no way indicates any relationship with, or London EC1Y 1SP endorsement by, the trademark owner. United Kingdom Printed in the United States of America SAGE Publications India Pvt. Ltd. ISBN 9781544380155 B 1/I 1 Mohan Cooperative Industrial Area Mathura Road, New Delhi 110 044 India SAGE Publications Asia-Pacific Pte. Ltd. 18 Cross Street #10-10/11/12 China Square Central This book is printed on acid-free paper. Singapore 048423 Sponsoring Editor: Jessica Miller Project Associate: Ivey Mellem Production Editor: Astha Jaiswal Copy Editor: Gillian Dickens Typesetter: C&M Digitals (P) Ltd. Cover Designer: Candice Harman Marketing Manager: Victoria Velasquez 21 22 23 24 25 10 9 8 7 6 5 4 3 2 1 BRIEF TABLE OF CONTENTS Preface xix About the Authors xxvii CHAPTER 1 Introduction: Exploring Mental Space 1 CHAPTER 2 The Philosophical Approach: Enduring Questions 25 CHAPTER 3 The Psychological Approach: A Profusion of Theories 57 CHAPTER 4 The Cognitive Approach I: Vision, Pattern Recognition, and Attention 83 CHAPTER 5 The Cognitive Approach II: Memory, Imagery, Concepts, and Problem Solving 111 CHAPTER 6 The Neuroscience Approach: Mind as Brain 147 CHAPTER 7 The Network Approach: Mind as a Web 189 CHAPTER 8 The Evolutionary Approach: Change Over Time 229 CHAPTER 9 The Linguistic Approach: Language and Cognitive Science 263 CHAPTER 10 The Emotional Approach: Mind as Emotion 297 CHAPTER 11 The Social Approach: Mind as Society 319 CHAPTER 12 The Artificial Intelligence Approach I: The Computer as a Cognitive Agent 355 CHAPTER 13 The Artificial Intelligence Approach II: Embedded Intelligence and Robotics 401 CHAPTER 14 The Embodied Ecological Approach: A Dynamic Future for Cognitive Science? 437 Glossary 475 References 495 Index 529 DETAILED TABLE OF CONTENTS Preface xix About the Authors xxvii CHAPTER 1 Introduction: Exploring Mental Space 1 A Brave New World 1 What Is Cognitive Science? 2 Representation 3 Types of Representation 5 Computation 7 The Tri-Level Hypothesis 8 Differing Views of Representation and Computation 10 The Interdisciplinary Perspective 12 The Philosophical Approach 14 ´ INTERDISCIPLINARY CROSSROADS: Science and Philosophy 14 The Psychological Approach 15 The Cognitive Approach 16 The Neuroscience Approach 17 The Network Approach 18 The Evolutionary Approach 18 The Linguistic Approach 19 The Emotion Approach 19 The Social Approach 20 The Artificial Intelligence Approach 20 The Robotics Approach 21 The Embodied Ecological Approach 21 Integrating Approaches 22 Summing Up: A Review of Chapter 1 22 CHAPTER 2 The Philosophical Approach: Enduring Questions 25 What Is Philosophy? 25 Chapter Overview 25 The Mind–Body Problem: What Is Mind? 26 Monism 28 Evaluating the Monist Perspective 29 Dualism 30 Substance Dualism 31 Property Dualism 32 Evaluating the Dualist Perspective 32 Functionalism: Are Minds Limited to Brains? 34 Evaluating the Functionalist Perspective 36 The Knowledge Acquisition Problem: How Do We Acquire Knowledge? 37 Evaluating the Knowledge Acquisition Debate 39 The Mystery of Consciousness: What Is Consciousness and How Does It Operate? 41 The What-It’s-Like Argument 42 Mind as an Emergent Property 44 Evaluating the Emergent View of Mind 46 Consciousness: One or Many? 46 Consciousness and Neuroscience 49 ´ INTERDISCIPLINARY CROSSROADS: Philosophy, Neuroscience, and Binocular Rivalry 51 Consciousness and Artificial Intelligence 53 Overall Evaluation of the Philosophical Approach 55 Summing Up: A Review of Chapter 2 56 CHAPTER 3 The Psychological Approach: A Profusion of Theories 57 What Is Psychology? 57 Psychology and the Scientific Method 58 Intelligence Tests 61 Mental Atoms, Mental Molecules, and a Periodic Table of the Mind: The Voluntarist Movement 62 Evaluating the Voluntarist Approach 64 Structuralism: What the Mind Is 65 Evaluating the Structuralist Approach 66 Functionalism: What the Mind Does 66 Evaluating the Functionalist Approach 68 The Whole Is Greater Than the Sum of Its Parts: Mental Physics and the Gestalt Movement 69 ´ INTERDISCIPLINARY CROSSROADS: Gestalt Phenomenology, Experimental Psychology, and Perceptual Grouping 71 Evaluating the Gestalt Approach 74 Mini Minds: Mechanism and Psychoanalytic Psychology 75 Evaluating the Psychoanalytic Approach 77 Mind as a Black Box: The Behaviorist Approach 78 Evaluating the Behaviorist Approach 80 Overall Evaluation of the Psychological Approach 81 Summing Up: A Review of Chapter 3 81 CHAPTER 4 The Cognitive Approach I: Vision, Pattern Recognition, and Attention 83 Some History First: The Rise of Cognitive Psychology 83 The Cognitive Approach: Mind as an Information Processor 84 Modularity of Mind 85 Evaluating the Modular Approach 85 Theories of Vision and Pattern Recognition: How Do We Recognize Objects? 86 Template Matching Theory 87 Evaluating Template Matching Theory 87 Feature Detection Theory 88 Evaluating Feature Detection Theory 90 Recognition by Components Theory 91 Evaluating Recognition by Components Theory 93 ´ INTERDISCIPLINARY CROSSROADS: Computational Vision and Pattern Recognition 94 Evaluating Marr’s Computational Approach to Vision 96 Feature Integration Theory 96 Evaluating Feature Integration Theory 100 Theories of Attention: How Do We Pay Attention? 100 Broadbent’s Filter Model 101 Evaluating the Filter Model 103 Treisman’s Attenuation Model 103 The Deutsch–Norman Memory Selection Model 103 The Multimode Model of Attention 104 Kahneman’s Capacity Model of Attention 105 Evaluating the Capacity Model of Attention 107 Evaluating the Model-Building Approach 107 Summing Up: A Review of Chapter 4 108 CHAPTER 5 The Cognitive Approach II: Memory, Imagery, Concepts, and Problem Solving 111 Types of Memory: How Do We Remember? 111 Sensory Memory 112 Working Memory 114 Scanning Items in Working Memory 117 Long-Term Memory 118 Memory Models 121 The Modal Model 121 Evaluating the Modal Model 122 The Working Memory Model 123 Evaluating the Working Memory Model 125 Visual Imagery: How Do We Imagine? 125 The Kosslyn and Schwartz Theory of Visual Imagery 126 Image Structures 126 Image Processes 127 Evaluating the Kosslyn and Schwartz Theory 129 Concepts: How Do We Represent Our Knowledge of Concepts? 132 Problem Solving: How Do We Solve Problems? 136 The General Problem Solver Model 139 Evaluating the General Problem Solver Model 140 ´ INTERDISCIPLINARY CROSSROADS: Artificial Intelligence, Problem Solving, and the SOAR Model 141 Evaluating the SOAR Model 143 Overall Evaluation of the Cognitive Approach 144 Summing Up: A Review of Chapter 5 144 CHAPTER 6 The Neuroscience Approach: Mind as Brain 147 The Neuroscience Perspective 147 Methodology in Neuroscience 148 Techniques for the Study of Brain Damage 148 Evaluating Techniques for the Study of Brain Damage 148 Brain Recording Methods 149 Positron Emission Tomography 150 Functional Magnetic Resonance Imaging 150 Magnetoencephalography 151 Knife-Edge Scanning Microscope 152 Brain Stimulation Techniques 152 Electrode Stimulation 152 Transcranial Magnetic Stimulation 152 Optogenetics 153 The Small Picture: Neuron Anatomy and Physiology 153 The Big Picture: Brain Anatomy 156 Directions in the Nervous System 157 The Cortex 157 The Split Brain 159 The Neuroscience of Visual Object Recognition 160 Visual Agnosias 161 Apperceptive Agnosia 162 Associative Agnosia 163 Face Perception 165 ´ INTERDISCIPLINARY CROSSROADS: Perceptual Binding and Neural Synchrony 167 The Neuroscience of Attention 168 Neural Models of Attention 170 A Component Process Model 170 Distributed Network Models 172 Disorders of Attention 173 Hemispatial Neglect 173 Attention-Deficit Hyperactivity Disorder 174 The Neuroscience of Memory 175 Learning and Memory 175 The Hippocampal System 176 Neural Substrates of Working Memory 178 Evaluating the Neuroscience of Working Memory 180 Neural Substrates of Long-Term Memories 180 The Neuroscience of Executive Function and Problem Solving 181 Theories of Executive Function 183 Overall Evaluation of the Neuroscience Approach 186 Summing Up: A Review of Chapter 6 186 CHAPTER 7 The Network Approach: Mind as a Web 189 The Network Perspective 189 Artificial Neural Networks 190 Characteristics of Artificial Neural Networks 193 Early Conceptions of Neural Networks 194 Backpropagation 195 NETtalk: An Example of a Backpropagation Artificial Neural Network 197 The Elman Net: An Example of a Simple Recurrent Network 199 Evaluating the Connectionist Approach 200 Advantages 200 Problems and Disadvantages 201 Semantic Networks: Meaning in the Web 203 Characteristics of Semantic Networks 203 A Hierarchical Semantic Network 205 Evaluating the Hierarchical Semantic Network Model 207 Evaluating Semantic Networks 208 Network Science 211 Centrality 212 Hierarchical Networks and the Brain 212 Small-World Networks: It’s a Small World After All 213 Ordered and Random Connections 215 Egalitarians and Aristocrats 217 Neuroscience and Networks 217 Small-World Networks and Synchrony 219 Percolation 220 Percolation and Psychology 220 The Future of Network Science 222 Overall Evaluation of the Network Approach 222 ´ INTERDISCIPLINARY CROSSROADS: Emotions and Networks 223 Summing Up: A Review of Chapter 7 226 CHAPTER 8 The Evolutionary Approach: Change Over Time 229 The Evolutionary View 229 A Little Background: Natural Selection and Genetics 230 Comparative Cognition 232 Cognitive Adaptation in Animals 233 ´ INTERDISCIPLINARY CROSSROADS: Evolutionary Processes and Artificial Life 235 Comparative Neuroscience 237 Evaluating the Comparative Approach 240 Evolutionary Psychology 241 Evolved Psychological Mechanisms 242 Evolution and Cognitive Processes 244 Categorization 244 Memory 245 Logical Reasoning 247 Judgment Under Uncertainty 249 Language 252 Behavioral Economics: How We Think About Profit and Loss 253 Sex Differences in Cognition 255 Evaluating Evolutionary Psychology 258 Overall Evaluation of the Evolutionary Approach 261 Summing Up: A Review of Chapter 8 261 CHAPTER 9 The Linguistic Approach: Language and Cognitive Science 263 The Linguistic Approach: The Importance of Language 263 The Nature of Language 264 Language Processing 265 Phonology 265 Morphology 268 Word Recognition 269 Syntax 270 Semantics 274 Pragmatics 276 Language Acquisition 277 Domain-General and Domain-Specific Mechanisms in Language Acquisition 279 Evaluating Language Acquisition 281 Language Deprivation 282 Evaluating Language Deprivation 284 ´ INTERDISCIPLINARY CROSSROADS: Language, Philosophy, and the Linguistic Relativity Hypothesis 285 Evaluating the Linguistic Relativity Hypothesis 286 Language Use in Nonhuman Animals 286 Evaluating Language Use in Nonhuman Animals 288 Neuroscience and Linguistics: The Wernicke–Geschwind Model 289 Evaluating the Wernicke–Geschwind Model 292 Artificial Intelligence and Linguistics: Natural Language Processing 293 Computer Language Programs and IBM’s Watson 293 Evaluation of Natural Language Processing 294 Overall Evaluation of the Linguistic Approach 294 Summing Up: A Review of Chapter 9 295 CHAPTER 10 The Emotional Approach: Mind as Emotion 297 Emotion and Cognitive Science 297 What Is Emotion? 297 Theories of Emotion 298 Basic Emotions 299 Emotions, Evolution, and Psychological Disorders 300 Disgust 302 Fear 302 Anger 302 Sadness 303 Happiness 303 Emotions and Neuroscience 304 The Chemical and Electrical Basis of Emotional Computation 305 Hot and Cold: Emotion–Cognition Interactions 306 Emotion and Perception/Attention 307 Emotion and Memory 308 Emotion, Mood, and Memory 309 Emotion and Decision Making 310 Emotions and Reasoning by Analogy 311 Emotions and Artificial Intelligence: Affective Computing 312 ´ INTERDISCIPLINARY CROSSROADS: Emotion, Robotics, and the Kismet Project 314 Overall Evaluation of the Emotional Approach 317 Summing Up: A Review of Chapter 10 317 CHAPTER 11 The Social Approach: Mind as Society 319 Social Cognition 319 Social Cognitive Neuroscience 321 Topics in Social Cognitive Neuroscience 322 Evolution 322 Attention 323 Mirror Neurons 325 Social Cognition as the Brain’s Default State 327 Is Social Cognitive Neuroscience Special? 328 Advantages of the Social Cognitive Neuroscience Approach 329 Theory of Mind 329 ToM and Neuroscience 330 Autism 332 Autism and ToM 333 Other Social Cognitive Disorders 334 Attitudes 334 Cognitive Dissonance 336 Attitudes and Cognitive Processes 337 Perception 337 Attention 338 Interpretation 338 Learning 338 Memory 338 Attitudes and Neuroscience 339 Impressions 340 The Dual-Process Model of Impression Formation 340 Attribution 341 Attribution Biases 342 Attribution and Cognitive Processes 342 Attribution and Neuroscience 343 ´ INTERDISCIPLINARY CROSSROADS: Game Theory and the Prisoner’s Dilemma 345 Stereotypes 347 Stereotypes and Cognitive Processes 347 Ingroups and Outgroups 348 Automatic Stereotyping 348 Stereotyping and Neuroscience 349 Prejudice 350 The Stereotype Content Model of Prejudice 350 Overall Evaluation of the Social Approach 351 Summing Up: A Review of Chapter 11 352 CHAPTER 12 The Artificial Intelligence Approach I: The Computer as a Cognitive Entity 355 Cognitive Science and Artificial Intelligence 355 Defining AI 358 Practical AI 360 Introduction 361 AI Implementation (“Hardware”) 363 Information and Intelligent Agents 366 Logic, Classical and Fuzzy 367 Reasoning Modalities 367 The Legacies of Turing and Zadeh 368 Turing’s State Variable Approach 368 Fuzzy Problem Solving 371 Intelligent Agents That Think, Learn, and Make Decisions 372 Fundamental Concepts of the IA 373 Basic Models 375 Machine Learning and the Data Sciences 377 The Trial-and-Error Learning Method 377 A Simple Algorithmic Method (Regression Line Algorithm) 378 Data Clustering 378 Reconsideration of Data Sources 380 Deep Learning (DL) 382 DL Software 383 Learning Experiences 384 Artificial General Intelligence 386 AGI Problems 388 Reverse Engineering the Brain 389 Methodologies 390 The “Organic Brain” and Wetware 392 Assessment of AI and AGI 395 Summing Up: A Review of Chapter 12 400 CHAPTER 13 The Artificial Intelligence Approach II: Embedded Intelligence and Robotics 401 Mechanical Beginnings 403 Embodied Cognitive Science 403 The Design of Intelligent Robots as “Biologically Inspired” 403 The Importance of Biology 404 Robotic Embodied Intelligence 405 Defining and Describing a Robot 405 The Intelligent Agent Paradigm 407 Properties of an Autonomous Entity 408 Environments of Intelligent Agents 409 A Simple yet “Sophisticated” Robot 410 Evolutionary Embodiments: The Merger of Human Cognitive Behavior, Biology, and Intelligent Agents 411 Challenges to DL and Its Algorithms 412 Emerging Tools 413 Evolutionary Learning Algorithms and Intelligent Agents 414 Evolutionary Computation 415 The Evolutionary Mutation Process 416 Robotic EA Examples 418 Robotic Embodiments 419 Robotic Realizations 421 Cooperating Intelligent Agents and Swarming 427 Swarming Robotics 427 Particle Robots: An Emerging Technology 428 Embedded Intelligence as an Emotion Machine 430 Machine–Human Interactions 431 Brain Waves 432 The Plasticity of the Human Brain 433 Machines Can Teach Humans 433 Overall Evaluation of Embedded Intelligence 434 Summing Up: A Review of Chapter 13 436 CHAPTER 14 The Embodied Ecological Approach: A Dynamic Future for Cognitive Science? 437 Embodied and Extended Cognition 437 Perceptual Symbol Systems and Motor Affordances 438 Perceptual Simulations 442 Evaluating Embodied Cognition 445 Dynamical Systems Theory 446 Nonlinearity 446 Predictability 447 State Space and Trajectories 448 Attractors 450 Dynamical Representation 451 ´ INTERDISCIPLINARY CROSSROADS: Multiple Approaches to Levels of Explanation in Cognitive Science 452 Dynamical Versus Classical Cognitive Science 454 The Continuity of Mind 454 Modularity Versus Distribularity 455 Component-Dominant Versus Interaction-Dominant Dynamics 455 Internalism Versus Externalism 456 Amodal Versus Modal Representations 457 Feed-Forward Versus Recurrent Pathways 457 Evaluating the Dynamical Perspective 458 Ecological and Extended Cognition 459 Ecological Perception 459 Sensorimotor Interaction 462 Extended Cognition 464 Evaluating Ecological and Extended Cognition 466 Integrating Cognitive Science 467 Integration Across Disciplines 467 Integration Across Levels of Description 468 Integration Across Methodologies 469 Integration Across Cultural Differences 469 The Benefits of Cognitive Science 471 The Future 472 Summing Up: A Review of Chapter 14 472 Glossary 475 References 495 Index 529 PREFACE O ne of the most challenging mysteries remaining to science is the human mind. The brain, which serves as the core engine of the mind, is the most complex object in the universe. It is made up of billions of cells sending signals back and forth to each other over trillions of connections. How can we make sense of all this? Recent years have seen great strides in our understanding, and this has been due in part to developments in technology. In this book, we provide an up-to-date introduction to the study of the mind, examining it from an interdisciplinary perspective. We attempt to understand the mind from the perspective of different fields. Among these are philosophy, psychology, neuroscience, networks, evolution, emotional and social cognition, linguistics, artificial intelligence, robotics, and the new framework of embodied cognition. Beyond this, we make attempts to bridge some of these fields, showing what research at the intersection of these disciplines is like. Each chapter in this text is devoted to a particular disciplinary approach and examines the methodologies, theories, and empirical findings unique to each. Come with us as we explore the next great frontier—our inner world. WHAT’S NEW IN THIS EDITION For this fourth edition, new content has been added throughout. In Chapter 1 (Intro- duction), the treatment of formal logic and production systems has been more richly elaborated with concrete examples. Also, a summary of the new Embodied Ecological Approach in Chapter 14 has been included. In Chapter 2 (The Philosophical Approach), a more in-depth exploration of syllogistic reasoning has been added, along with a more detailed discussion of reductionism and how it contrasts with emergence. Also, the discussion of Searle’s Chinese room thought experiment has been expanded. In Chapter 3 (The Psychological Approach), a discussion of intelligence tests has been added. In Chapter 4 (The Cognitive Approach I), the description of Anne Treisman’s feature integration theory of visual attention was expanded, along with some discus- sion of Desimone and Duncan’s biased competition account. In Chapter 5 (The Cogni- tive Approach II), a section on conceptual representation has been added. In Chapter 6 (The Neuroscience Approach), the descriptions of various brain-recording methods have been expanded, and discussions of the somatosensory homunculus and of sparse distributed coding were added. In Chapter 7 (The Network Approach), treatments of Elman’s simple recurrent network and McClelland and Rogers’s connectionist model of category knowledge have been added, along with an expanded discussion of pattern completion. In Chapter 8 (The Evolutionary Approach), a discussion of foraging skills in animals has been included, and the treatment of gender differences in spatial abilities has xix been expanded. Chapter 9 (The Linguistic Approach) has been substantially rearranged. The role of linguistics (and Chomsky in particular) in the formation of cognitive science is emphasized early on. The treatment of phonology, morphology, syntax, semantics, and language acquisition has been expanded significantly. Also, sections on spoken word recognition and cognitive linguistics have been added. In Chapter 10 (The Emotional Approach), Lisa Feldman Barrett’s proposal that emotions are not discrete but can par- tially overlap one another has been added, as well as a discussion of how color perception can influence affect. In Chapter 11 (The Social Approach), the discussions of the mir- ror neuron system, autism, the prisoner’s dilemma, and stereotype formation have been slightly expanded. Chapter 12 (The Artificial Intelligence Approach I) has been substan- tially rearranged in its treatment of Alan Turing and Lotfi Zadeh, with a new focus on intelligent agents, Bayesian probability, deep learning, and brain–computer interfaces. In Chapter 13 (The Artificial Intelligence Approach II), the discussion of reactive and deliberative architectures has been expanded and sections have been added on robotic embodied intelligence, evolutionary algorithms, and swarming robotics. Chapter 14 (The Embodied Ecological Approach) was converted from a “future-looking conclu- sion” chapter into a “state-of-the-art dynamical, embodied, ecological” chapter. The dis- cussions of dynamical systems theory and ecological perception have been significantly expanded, and a section on embodied cognition has been added. xx Cognitive SCienCe A Matrix for Exploring Cognitive Science Across Disciplines Chapter Chapter Primary Topic/ Secondary Topic/ No. Name/Title Summary Issues Issues Methodologies Major Figures Evaluation 1 introduction An introduction interdisciplinary Concepts no methodologies thagard Cognitive science is to cognitive study Propositions discussed Harnish unique in that it binds science and Representation Production rules Pylyshyn together different summary overview and computation Declarative Marr perspectives and of different interdisciplinary and procedural methodologies in the perspectives perspective knowledge study of mind Categories Analogies of mental representation 2 the the search for the mind–body Monism Deductive Aristotle Provides a broad Philosophical wisdom and problem Dualism and inductive Plato perspective; asks Approach knowledge; frames Functionalism nature–nurture reasoning Berkeley fundamental broad questions Knowledge debate Democritus questions; not an about mind acquisition Reductionism Descartes empirical approach Consciousness emergence Ryle Clark Hume Locke Chalmers nagel Jackson Searle Churchland Dennett 3 the the scientific the scientific theory and Scientific method Wundt Multiple theoretical Psychological study of mind and method hypothesis introspection titchener positions; first Approach behavior voluntarism independent Phenomenology James systematic and Structuralism and dependent Wertheimer scientific study of Functionalism variables Koffka mental phenomena; gestalt experimental Kohler problems with psychology and control Freud introspection and Psychoanalytic groups Watson phenomenology psychology Stream of Pavlov Behaviorism consciousness Skinner Levels of consciousness xxi (Continued) (Continued) xxii Chapter Chapter Primary Topic/ Secondary Topic/ No. Name/Title Summary Issues Issues Methodologies Major Figures Evaluation 4 the Cognitive the information- information- template experimentation neisser Fruitful synergistic Approach i processing view processing matching Modeling Fodor use of experimentation of mind; use of perspective Feature Selfridge and model building a computer as Modularity detection norman a metaphor for Pattern Computational Marr mind; use of recognition vision treisman process models Attention Feature Broadbent and assumption of integration Deutch modularity theory Posner Models of Snyder attention Kahneman Biederman 5 the Cognitive the information- Memory Memory types: experimentation Sperling Common set of Approach ii processing view Models of memory sensory, Modeling Baddeley assumptions of mind; use of visual imagery working, and Same as Atkinson underlying information a computer as Problem solving long term Cognitive Shiffrin processing and a metaphor for the modal, and Approach i Anderson modularity; concepts mind; use of working memory chapter Kosslyn of representation and process models models Block computation need to and assumption of the Kosslyn– newell be reconciled with modularity Schwartz theory Simon connectionism (Same as Cognitive of visual imagery Sternberg Approach i chapter) Heuristics Means-ends analysis the gPS and SoAR models 6 the the study of neuroscience the split brain Case studies Sperry the marriage neuroscience nervous system methodology Dorsal and Lesion studies Sacks of cognitive and Approach anatomy and neuron anatomy ventral pathways Cell-recording Humphreys neuroscience physiology that and physiology Agnosias techniques Posner perspectives underlies and gives Brain anatomy Plasticity eeg, eRP, CAt, Lashley in cognitive rise to cognitive neuroscience Hippocampal Pet, and fMRi Hebb neuroscience is a good function of visual object function Meg andtMS Shallice integrative approach; recognition, Action schemas engel specification of attention, and scripts Singer biological structures memory, executive Metacognition and processes of function, and Binding and cognitive abilities problem solving neural synchrony Chapter Chapter Primary Topic/ Secondary Topic/ No. Name/Title Summary Issues Issues Methodologies Major Figures Evaluation 7 the network view of mind as Serial and parallel Perceptrons Software McCulloch Significant advantages Approach an interconnected processing Back simulations of Pitts to using networks set of nodes or Artificial neural propagation artificial neural Hopfield for understanding web; processing networks Stability and networks Kohonen learning and consists of the Semantic plasticity Comparison of grossberg knowledge spread of activation networks Catastrophic results with theory Collins representation; through the web network science interference and empirical data Quillian challenges in building Spreading Rumelhart networks that rival the activation McClelland brain Retrieval cues Watts Priming Strogatz Propositional Buchanan networks Small-world networks 8 the Mind as the natural selection general-purpose experimentation Darwin Powerful theoretical evolutionary adapted product of evolved versus domain- Cross-species Buss framework, but not Approach selection forces psychological specific view of comparison Cosmides all mental processes mechanisms mind tooby may be adaptive; Comparative Wason selection edelman good integration with cognition task neuroscience; domain- evolution Heuristics and specific processing and cognitive fallacies view clashes with processes exaptation, general-purpose Behavioral molecular drive, processor view economics and spandrels Artificial life (Continued) xxiii (Continued) xxiv Chapter Chapter Primary Topic/ Secondary Topic/ No. Name/Title Summary Issues Issues Methodologies Major Figures Evaluation 9 the the the nature of Phonology and Case studies gardner Multiple perspectives Linguistic multidisciplinary language morphology Developmental Premack and techniques Approach study of language Primate language Syntax and studies Savage- brought to bear on use semantics experimentation Rumbaugh the complex cognitive Language Animal language Sapir topic of language; acquisition studies Whorf advances in computer- Language Critical period Chomsky based language deprivation Second- Broca comprehension Linguistic language Wernicke relativity acquisition tanenhaus hypothesis Phrase Clark grammar structure, Lakoff the Wernicke– transformational Marslen- geschwind model and universal Wilson natural language grammar Saffran processing Aphasias Speech recognition Pragmatic analysis 10 the emotions and emergence evolutionary experimentation Damasio need for an emotional moods influence synthesis accounts of the iowa Fox understanding of Approach all major cognitive approach psychological gamblingtask Minsky information flow and processes Flashbulb and disorders Solomon neural circuits that and should be autobiographical Analytical underlie emotions incorporated into memory rumination and how these impact cognitive theories Mood-congruent hypothesis thought; more work and models of mind and mood- the feel- needed on how dependent good, do-good cognitions affect memory phenomenon emotions Role of synapses CogAff in emotional architecture processing Social robots Affective computing Chapter Chapter Primary Topic/ Secondary Topic/ No. Name/Title Summary Issues Issues Methodologies Major Figures Evaluation 11 the Social thinking about Mirror neurons Anterior and Brain imaging Fiske Automatic versus Approach people is different theory of mind posterior the ultimatum ochsner and effortful processing from thinking Attitudes attention game Lieberman is a common theme; about objects; Cognitive systems Social dilemmas Rizzolatti the historical focus social cognitive dissonance Autism Siegal and on the individual is neuroscience is a impressions Prisoner’s varley too narrow, cognitive good example of the Attributions dilemma Frith science must embrace interdisciplinary Stereotyping Schacter the social environment approach Prejudice 12 the Artificial Defining the Historical Universal Cognitive models turing new computational intelligence concept of artificial perspective computation turingtest Minsky technologies may Approach i intelligence; influence ofturing Chatbots Kurzweil lead to the densities machine Predictive evolutionary Craik required to achieve representation of architectures computing Hawkins the requirements cognitive function Artificial general Russell needed to implement intelligence norvig an intelligence that Agent-based McCarthy is beyond the human architectures: goertzel level; a fundamental Multiagent dilemma persists: systems “Brains must have programs yet at the same time must not be programmed.” (Continued) xxv (Continued) xxvi Chapter Chapter Primary Topic/ Secondary Topic/ No. Name/Title Summary Issues Issues Methodologies Major Figures Evaluation 13 the Artificial the intelligent importance of Hierarchical, Cognitive turing in some activities, intelligence agent (iA) biology reactive, modeling Minsky machines already Approach ii paradigm; applying embodiment hybrid robotic Simulation Brooks outperform humans; a the principles to and situational architectures Russell great many problems the design of iAs; aspects of iA emotion in iAs norvig need to be addressed cognitive models (structure) Breazeal in the future: of iAs; robotic expert systems Arkin perception, finely embodiments; Building a brain honed reasoning, biological and Robotic and manipulative social influences on architectures capabilities of adult robots humans; the more we try to replicate human intelligence, the more we may learn to appreciate and understand humans 14 the An evaluation of Cognitive Predictability nonlinear gibson Benefits of cognitive embodied the embodiment science needs to Randomness modeling Dreyfus science are many and ecological approach to do a better job Constructivism Use of state Brooks widespread throughout Approach cognitive science explaining the space, Barsalou engineering, medicine, and the ecological role of the body trajectories, and Pulvermüller education, and other perception and of physical attractors to Borghi fields; lack of a single framework environments describe cognitive turvey unified theory in cognition, as phenomena Spivey well as individual and cultural differences the dynamical systems approach ABOUT THE AUTHORS Jay Friedenberg is Professor of the Psychology Department at Manhattan College, where he directs the Cognitive Science Program. He is interested in both vision and the philosophy of mind. He teaches courses in physiological psychology, cognition and learning, sensation and perception, and artificial intelligence and robotics. He has pub- lished several articles on visual estimation of center of mass. His current research proj- ects focus on the aesthetics of geometrical shapes. He has published books on artificial intelligence, dynamical systems theory, and psychology. He is a member of the Interna- tional Association of Empirical Aesthetics, the Eastern Psychological Association, the Vision Science Society, the Psychonomic Society, and Phi Beta Kappa. He obtained his PhD in cognitive psychology in 1995 at the University of Virginia. Gordon Silverman is Professor Emeritus of Electrical and Computer Engineering at Manhattan College. His professional career spans more than 55 years of corporate, teaching, consulting, and research experience, during which he has developed a range of scientific instruments, particularly for use in physiological psychology research envi- ronments. He is the holder of eight patents, some related to behavior modification. The author of more than 20 journal articles and books, he has also served on the faculties of The Rockefeller University and Fairleigh Dickinson University. His current research interests include telemedicine, rehabilitation medicine, artificial intelligence, and bio- medical instrumentation and modeling. He holds engineering degrees from Columbia University and received a PhD in system science from New York University Polytechnic School of Engineering in 1972. Michael J. Spivey is Professor of Cognitive Science at the University of California, Merced. He earned his BA in Psychology at the University of California, Santa Cruz, and his PhD in Brain and Cognitive Sciences at the University of Rochester. After 12 years as a psychology professor at Cornell University, Spivey moved to UC Merced to help build their Department of Cognitive and Information Sciences. He has published over 100 journal articles and book chapters on the embodiment of cognition and inter- actions between language, vision, memory, syntax, semantics, and motor movement. His research uses eye tracking, computer-mouse tracking, and dynamical systems theory to explore how brain, body, and environment work together to make a mind what it is. In 2010, Spivey received the William Procter Prize for Scientific Achievement from the Sigma Xi Scientific Research Honor Society. xxvii CHAPTER ONE INTRODUCTION Exploring Mental Space A BRAVE NEW WORLD Learning Objectives We are in the midst of a scientific revolution. For centuries, science has made great strides in our understanding of the external observ- After reading this chapter, able world. Physics revealed the motion of the planets, chemistry you will be able to: discovered the fundamental elements of matter, and biology has told us how to understand and treat disease. But during much of this 1. List at least five time, there were still many unanswered questions about something disciplines that participate in perhaps even more important to us—the human mind. the field of cognitive science. What makes mind so difficult to study is that, unlike the phe- 2. Describe what a mental nomena described above, it is not something we can easily observe, representation is. measure, or manipulate. In addition, the mind is the most complex 3. Describe what mental entity in the known universe. To give you a sense of this complexity, computation is. consider the following. The human brain is estimated to contain 10 billion to 100 billion individual nerve cells or neurons. Each of 4. Define what these neurons can have as many as 10,000 connections to other neu- interdisciplinary means. rons. This vast web of neural tissue is the core engine of the mind and helps generate a wide range of amazing and difficult-to-under- stand mental phenomena, such as perception, memory, language, emotion, and social interaction. The past several decades have seen the introduction of new technologies and methodologies for studying this intriguing organ, and its relationship to the body, and to the environment. We have learned more about the mind in the past half-century than in all the time that came before that. This period of rapid discovery has coin- cided with an increase in the number of different disciplines—many of them entirely new—that study mind. Since then, a coordinated effort among the practitioners of these disciplines has come to pass. This diversely interdisciplinary approach has since become known as cognitive science. Unlike the sciences that came before, which were focused solely on the world of physical events in physical space, this new endeavor now turns its full attention to discovering the fascinating mental events that take place in mental space. 1 WHAT IS COGNITIVE SCIENCE? Cognitive science can be roughly summed up as the scientific interdisciplinary study of the mind. Its primary methodology is the scientific method—although, as we will see, many other methodologies also contribute. A hallmark of cognitive science is its interdisciplinary approach. It results from the efforts of researchers working in a wide array of fields. These include philosophy, psychology, linguistics, artificial intelligence (AI), robotics, and neuroscience, among others. Each field brings with it a unique set of tools and perspectives. One major goal of this book is to show that when it comes to studying something as complex as the mind, no single perspective is adequate. Instead, intercommunication and cooperation among the practitioners of these disciplines will tell us much more. The term cognitive science refers not so much to the sum of all these disciplines but to their intersection or converging work on specific problems. In this sense, cognitive science is not a unified field of study like each of the disciplines themselves but, rather, a collaborative effort among researchers working in the various fields. The glue that holds cognitive science together is the topic of mind and, for the most part, the use of scientific methods. In the concluding chapter, we talk more about the issue of how unified cogni- tive science really is. To understand what cognitive science is all about, we need to know what its theo- retical perspective on the mind is. This perspective began with the idea of computation, which may alternatively be called information processing. Cognitive scientists started out viewing the mind as an information processor. Information processors must both represent information and transform information. That is, a mind, according to this perspective, must incorporate some form of mental representation and processes that act on and manipulate that information. We will discuss these two ideas in greater detail later in this chapter. Cognitive science is often described as having been heavily influenced by the devel- opment of the digital computer. Computers are, of course, information processors. Think for a minute about a personal computer. It performs a variety of information- processing tasks. Information gets into the computer via input devices, such as a keyboard or modem. That information can then be stored on the computer—for example, on its hard drive—in the form of binary representations (coded as 0s and 1s). The information can then be processed and manipulated using software, such as a text editor. The results of this processing may next serve as output, either to a monitor or to a printer. In like fashion, we may think of people performing similar tasks. Information is “input” into our minds through perception—what we see or hear. It is stored in our memories and processed in the form of thought. Our thoughts can then serve as the basis of “outputs,” such as language or physical behavior. Of course, this analogy between the human mind and computers is highly abstract and imperfect. The actual physical way in which data are stored on a computer bears little resemblance to human memory formation. But both systems are characterized by some form of computation (e.g., binary computation in the case of computers and analog computation in the case of brains). In fact, it is not going too far to say that most 2 Cognitive SCienCe cognitive scientists view the mind as a machine or mechanism whose workings they are trying to understand. REPRESENTATION As mentioned before, representation has been seen as fundamental to cognitive science. But what is a representation? Briefly stated, a representation is something that stands for something else. Before listing the characteristics of a representation, it is helpful to describe briefly four categories of representation. (1) A concept stands for a single entity or group of entities. Single words are good examples of concepts. The word apple refers to the concept that represents that particular type of fruit. (2) Propositions are statements about the world and can be illustrated with sentences. The sentence “Mary has black hair” is a proposition that is itself made up of a few concepts. (3) Rules are yet another form of representation that can specify the relationships between propositions. For example, the rule “If it is raining, I will bring my umbrella” makes the second proposition contingent on the first. (4) An analogy helps us make comparisons between two similar situations. We will discuss all four of these representations in greater detail in the “Interdisciplinary Crossroads” section at the end of this chapter. There are four crucial aspects of any representation (Hartshorne, Weiss, & Burks, 1931–1958). First, a “representation bearer” such as a human or a computer must realize a representation. Second, a representation must have content—meaning it stands for one or more objects. The thing or things in the external world that a representation stands for are called referents. Third, a representation must also be “grounded.” That is, there must be some way in which the representation and its referent come to be related. Fourth, a representation must be interpretable by some interpreter, either the representation bearer or somebody else. These and other characteristics of representations are discussed next. The fact that a representation stands for something else means it is symbolic. We are all familiar with symbols. We know, for instance, that the dollar symbol ($) is used to stand for money. The symbol itself is not the money but, instead, is a surrogate that refers to its referent, which is actual money. In the case of mental representation, we say that there is some symbolic entity “in the mind” that stands for real money. Figure 1.1 shows a visual representation of money. Mental representations can stand for many different types of things and are by no means limited to simple conceptual ideas such as “money.” Research suggests that there are more complex mental representations that can stand for rules—for example, knowing how to drive a car and analogies, which may enable us to solve certain problems or notice similarities (Thagard, 2000). Human mental representations, especially linguistic ones, are said to be semantic, which is to say that they have meaning. Exactly what constitutes meaning and how a repre- sentation can come to be meaningful are topics of debate. According to one view, a repre- sentation’s meaning is derived from the relationship between the representation and what it is about. The term that describes this relation is intentionality. Intentionality means “directed on an object.” Mental states and events are intentional. They refer to some actual thing or things in the world. If you think about your brother, then the thought of your brother is directed toward him—not toward your sister, a cloud, or some other object. Chapter one introduCtion 3 Figure 1.1 Different aspects of the symbolic representation of money. The Mind Representation (Symbolic) $ Intentionality Referent (Nonsymbolic) The World Source: PhotoObjects.net/Thinkstock. An important characteristic of intentionality has to do with the relationship between inputs and outputs to the world. An intentional representation must be triggered by its referent or things related to it. Consequently, activation of a representation (i.e., thinking about it) should cause behaviors or actions that are somehow related to the referent. For example, if your friend Sally told you about a cruise she took around the Caribbean last December, an image of a cruise ship would probably pop into mind. This might then cause you to ask her if the food onboard was good. Sally’s mention of the cruise was the stimulus input that activated the internal representation of the ship in your mind. Once it was activated, it caused the behavior of asking about the food. This relation between inputs and outputs is known as an appropriate causal relation. Symbols can be assembled into what are called physical symbol systems, or more simply as formal logical systems. In a formal logical system, symbols are combined into expressions. These expressions can then be manipulated using processes. The result of a process can be a new expression. For example, in formal logic, symbols can be words like animals or mammals, and expressions could be statements like “animals that nurse their young are mammals.” The processes would be the rules of deduction that allow us to derive true concluding expressions from known expressions. In this instance, we could start off with the two known expressions “animals that nurse their young are mammals,” and “whales nurse their young,” and generate the new concluding expression: “whales are mammals.” (There are, in fact, a few nonmammals that nurse their young, but that is 4 Cognitive SCienCe for an advanced biology textbook.) More on this below, where we discuss propositions and syllogisms. According to the physical symbol system hypothesis (PSSH), a formal logical system can allow for intelligence (Newell & Simon, 1976). Since we as humans appear to have representational and computational capacity, being able to use things that stand for things, we seem to be intelligent. Beyond this, we could also infer that machines are intelligent, since they too have this capacity, although of course, this is debated. Several critiques have been leveled against the PSSH (Nilsson, 2007). For example, it is argued that the symbols computers use have no meaning or semantic quality. To be meaningful, symbols have to be connected to the environment in some way. People and perhaps other animals seem to have meaning because we have bodies and can perceive things and act on them. This “grounds” the symbols and imbues them with semantic qual- ity. Computing machines that are not embodied with sensors (e.g., cameras, microphones) and effectors (e.g., limbs) cannot acquire meaning. This issue is known as the symbol grounding problem and is in effect a reexpression of the concept of intentionality. A counterargument to this is that computer systems do have the capability to designate. An expression can designate an object if it can affect the object itself or behave in ways that depend on the object. One could argue that a robot capable of per- ceiving an object like a coffee mug and able to pick it up could develop semantics toward it in the same way that a person might. Ergo, the robot could be intelligent. Also, there are examples of AI programs like expert systems that have no sensor or effector capability yet are able to produce intelligent and useful results. Some expert systems, like MYCIN, are able to more accurately diagnose certain medical disorders than are members of the Stanford University medical school (Cawsey, 1998). They can do this despite not being able to see or act in the world. Types of Representation The history of research in cognition suggests that there are numerous forms of mental representation. Paul Thagard (2000), in Mind: Introduction to Cognitive Science, proposes four: concepts, propositions, rules, and analogies. Although some of these have already been alluded to and are described elsewhere in the book, they are so central to many ideas in cognitive science that it is, therefore, useful to sketch out some of their major characteristics again here. A concept is perhaps the most basic form of mental representation. A concept is an idea that represents things we have grouped together. The concept “chair” does not refer to a specific chair, such as the one you are sitting in now, but it is more general than that. It refers to all possible chairs no matter what their colors, sizes, and shapes. Concepts need not refer to concrete items. They can stand for abstract ideas—for example, “justice” or “love.” Concepts can be related to one another in complex ways. They can be related in a hierarchical fashion, where a concept at one level of organization stands for all members of the class just below it. “Golden retrievers” belongs to the category of “dogs,” which in turn belongs to the category of “animals.” We discuss a hierarchical model of concept representation in the network approach chapter. The question of whether concepts are innate or learned is discussed in the philosophical approach chapter. Chapter one introduCtion 5 A proposition is a statement or assertion typically posed in the form of a simple sentence. An essential feature of a proposition is that it can be proved true or false. For instance, the statement “The moon is made out of cheese” is grammatically correct and may represent a belief that some people hold, but it is a false statement. We can apply the rules of formal logic to propositions to determine the validity of those propositions. One logical inference is called a syllogism. A syllogism consists of a series of propositions. The first two (or more) are premises, and the last is a conclusion. Take the following syllogism: Premise 1: All men like football. Premise 2: Charlie is a man. Conclusion: Charlie likes football. Obviously, the conclusion can be wrong if either of the two premises is not fully and completely true. If 99% of men like football, then it might not be true that Charlie likes football, even if he is a man. And if Charlie is not a man, then Charlie may or may not like football, even assuming all men like it. Logical conclusions are only as reliable as the premises on which they are based. In the artificial intelligence approach chapter, we will discuss how probabilities (like 99%) can be used in probabilistic reasoning (and even fuzzy logic) to work with premises that are not fully and completely true. You may have noticed that propositions are representations that incorporate con- cepts. The proposition “All men like football” incorporates the concepts “men” and “football.” Propositions are more sophisticated representations than concepts because they express relationships—sometimes very complex ones—between concepts. The rules of logic are best thought of as computational processes that can be applied to prop- ositions to determine their validity. However, logical relations between propositions may themselves be considered a separate type of representation. The evolutionary approach chapter provides an interesting account of why logical reasoning, which is difficult for many people, can be made easier under certain circumstances. Formal logic is at the core of a type of computing system that produces effects in the real world: production systems. Inside a production system, a production rule is a con- ditional statement of the following form: “If x, then y,” where x and y are propositions. In formal logic, the “if” part of the rule is called the antecedent, and the “then” part is called the consequent. In a production system, the “if” part of the rule is called the condition, and the “then” part is called the action. If the proposition that is contained in the condi- tion (x) is verified as true, then the action that is specified by the second proposition (y) should be carried out, according to the rule. The following rules help us drive our cars: If the light is red, then step on the brakes. If the light is green, then step on the accelerator. In fact, it is production rules like these that are used in the computational algorithms that run self-driving cars. Notice that, in the first rule, the two propositions are “the light is 6 Cognitive SCienCe red” and “step on the brakes.” We can also form more complex rules by linking proposi- tions with “and” and “or” statements: If the light is red or the light is yellow, then step on the brakes. If the light is green and nobody is in the crosswalk, then step on the accelerator. The or that links the two propositions in the first part of the rule specifies that when either proposition is true, the action should be carried out. By contrast, when an and links these two propositions, then the rule specifies that both must be true before the action can occur. Rules bring up the question of what knowledge really is. We usually think of knowl- edge as factual. Indeed, a proposition such as “Candy is sweet,” if validated, does pro- vide factual information. The proposition is then an example of declarative knowledge. Declarative knowledge is used to represent facts. It tells us what is and is demonstrated by verbal communication. Procedural knowledge, by comparison, refers to skills. It tells us how to do something and is demonstrated by action. If we say that World War II was fought during the period 1939 to 1945, we have demonstrated a fact learned in history class. If we ski down a snowy mountain slope in the winter, we have demon- strated that we possess a specific skill. It is, therefore, very important that information- processing systems have some way of representing actions if they are to help an organism or machine perform those actions. Rules are just one way of representing procedural knowledge. In the cognitive approach chapters, we discuss two cognitive, rule-based sys- tems: the atomic components of thought and SOAR (state, operator, and result) models. In the ecological embodied approach chapter, we discuss more sensorimotor ways of representing procedural knowledge (and even declarative knowledge). Another specific type of mental representation is the analogy—although, as is pointed out below, the analogy can also be classified as a form of reasoning. Thinking analogically involves applying one’s familiarity with an old situation to a new situation. Suppose you had never ridden on a train before but had taken buses numerous times. You could use your understanding of bus riding to help you figure out how to take a ride on a train. Applying knowledge that you already possess and that is relevant to both scenarios would enable you to accomplish this. Based on prior experience, you would already know that you have to first determine the schedule, perhaps decide between express and local service, purchase a ticket, wait in line, board, stow your luggage, find a seat, and so on. Analogies are a useful form of representation because they allow us to generalize our learning. Not every situation in life is entirely new. We can apply what we already have learned to similar situations without having to figure out everything all over again. Several models of analogical reasoning have been proposed (Forbus, Gentner, & Law, 1995; Holyoak & Thagard, 1995). COMPUTATION As mentioned earlier, representations are only the first key component of the traditional cognitive science view of mental processes. Representations by themselves are of little use Chapter one introduCtion 7 unless something can be done with them. Having the concept of money doesn’t do much for us unless we know how to calculate a tip or can give back the correct amount of change to someone. In this cognitive science view, the mind performs computations on represen- tations. It is, therefore, important to understand how these mental mechanisms operate. What sorts of mental operations does the mind perform? If we wanted to get details about it, the list would be endless. Take the example of mathematical ability. If there were a separate mental operation for each step in a mathematical process, we could say the mind adds, subtracts, divides, and so on. Likewise, with language, we could say that there are separate mental operations for making a noun plural, putting a verb into past tense, and so on. It is better, then, to think of mental operations as falling into broad categories. These categories can be defined by the type of operation that is performed or by the type of information acted on. An incomplete list of these operations would include sensation, perception, attention, memory, language, mathematical reasoning, logical rea- soning, decision making, and problem solving. Many of these categories may incorporate virtually identical or similar subprocesses—for example, scanning, matching, sorting, and retrieving. Figure 1.2 shows the kinds of mental processes that may be involved in solving a simple addition problem. The Tri-Level Hypothesis Any given information process can be described at several different levels. According to the tri-level hypothesis, biological or artificial information-processing events can be evaluated on at least three different levels (Marr, 1982). The highest or most abstract level of analysis is the computational level. At this level, one is concerned with two tasks. The first is a clear specification of what the problem is. Taking the problem as it may originally have been posed, in a vague manner perhaps, and breaking it down into its main constitu- ents or parts can bring about this clarity. It means describing the problem in a precise way such that the problem can be investigated using formal methods. It is like asking, What exactly is this problem? What does this problem entail? The second task one encounters at the computational level concerns the purpose or reason for the process. The second task consists of asking, Why is this process here in the first place? Inherent in this analysis is adaptiveness—the idea that biological mental processes are learned or have evolved to enable the organism to solve a problem it faces. This is the primary explanatory perspec- tive used in the evolutionary approach. We describe a number of cognitive processes and the putative reasons for their evolution in the evolution chapter. Stepping down one level of abstraction, we can next inquire about the way in which an information process is carried out. To do this, we need an algorithm, a formal pro- cedure or system that acts on informational representations. It is important to note that algorithms can be carried out regardless of a representation’s meaning; algorithms act on the form, not the meaning, of the symbols they transform. One way to think of algorithms is that they