Introductory Lecture: The Scientific Method, Experimental Design and Descriptive Statistics PDF
Document Details
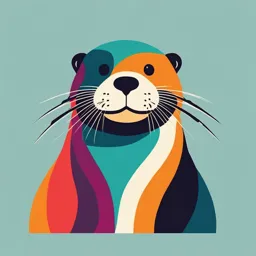
Uploaded by ProblemFreeSquirrel
Lund University
2023
BIMM01
Harry Björkbacka
Tags
Summary
Lecture notes for an introductory lecture on the scientific method, experimental design, and descriptive statistics. The lecture, part of the BIMM01 HT23 course, covers topics such as inductive and deductive reasoning, hypothesis testing. This document includes examples, discussions, and relevant references.
Full Transcript
Introductory lecture: The scientific method, experimental design and descriptive statistics BIMM01 HT23 Harry Björkbacka [email protected] ...
Introductory lecture: The scientific method, experimental design and descriptive statistics BIMM01 HT23 Harry Björkbacka [email protected] The scientific method [email protected] What is science? The intellectual and practical activity encompassing the systematic study of the structure and behaviour of the physical and natural world through observation and experiment Oxford Dictionary [email protected] What is the scientific method? The scientific method is an empirical method of acquiring knowledge that has characterized the development of science for centuries. The scientific method can be divided into steps: 1. Make an observation or ask a question 2. Do background research 3. Construct a hypothesis 4. Test the hypothesis by experiments 5. Analyse and draw conclusions 6. Report your results. [email protected] Inductive and deductive reasoning Inductive reasoning Deductive reasoning Science begins by securing Deduction is top-down observed facts, which are reasoning, as one moves collected in a theory-free from the general theory and manner draws logical conclusions about specific cases which These facts provide a firm form the basis for base from which the a hypothesis scientist reasons upward to hypotheses, laws, or theories [email protected] Holder & Marino (2017) Current Protocols in Pharmacology, 76, A.3G.1–A.3G.26. Deductive reasoning Inductive reasoning ✓ All mammals have backbones. Humans are mammals. As a paleontologist you discover a fossil of a mysterious animal has eyes to the Humans have backbones. front and lacks flat molar teeth ✓ All plants perform photosynthesis. A cactus is a plant. Predators generally have eyes to the front of their skulls and not to the side A cactus performs photosynthesis. Carnivores lack flat molar teeth All bald men are grandfathers. Harold is bald. What would your conclusion be? Is the Therefore, Harold is a grandfather. conclusion 100% certain? The conclusion is not 100% certain. While the observations Why is one conclusion faulty? strongly suggest that the animal could be a predator or carnivore, there could be exceptions or other explanations for This conclusion is faulty because the premise "All these characteristics. Inductive reasoning can suggest likely bald men are grandfathers" is not necessarily true. conclusions but cannot guarantee them with absolute Even if Harold is bald, this does not guarantee he certainty. Inductive reasoning can suggest likely conclusions is a grandfather. The conclusion is not valid but cannot guarantee them with absolute certainty. because the initial premise is incorrect. [email protected] The scientific method in practise Although the steps of the scientific Different scientific disciplines can have slightly different definitions of the scientific method are followed, the structure method is not as rigid as often described The step are not always followed in order Steps are often repeated multiple times Jumps and loops between steps is common There is a place for both inductive Induction, deduction, intuition, and guessing are all allowed when formulating a hypothesis and deductive reasoning in science Science is a creative endeavour! [email protected] Hypothetico-Deductive Method Scientists take a hypothesis or a theory and tests it indirectly by deriving from it one or more observational predictions, which are amenable to direct empirical test If the predictions are borne out by the data, then that result is taken as a confirming instance of the theory in question Why not proof? If the predictions fail to square with the data, then that fact counts as a disconfirming instance of the theory traditional statistical significance test procedures are often embedded in a hypothetico-deductive structure [email protected] Verifiability vs. Falsifiability The influential philosopher Karl Popper claimed that the scientific method does not exist. By this he meant that there is no method of discovering a scientific theory, that there is no method of verification, and that there is no method for establishing whether a hypothesis is probably true or not. No matter how many instances of white swans we may have observed, Karl Popper this does not justify the conclusion that all swans are white (1902-1994) Verifying a hypothesis by experiments only means that the hypothesis can be assumed to be true, until the hypothesis can be refuted by new experiments [email protected] Verifiability vs. Falsifiability Popper argued that science progresses by the growing set of discarded hypotheses, and those who survives are only tentatively held as true. More specifically Popper argued that science advanced with the falsification of trusted hypothesis, and the verifications of bold new conjectures There is no way to prove that the sun will rise, it is possible to formulate the theory that every day the sun will rise; if it Karl Popper does not rise on some particular day, the theory will be (1902-1994) falsified and will have to be replaced by a different one Falsifying or rejecting a hypothesis by experiments leads to real progress in science. No number of verifying experiments can prove a hypothesis to be true, only by rejecting a hypothesis can we advance to new theories [email protected] What is a research question? Good research questions should be: Focused Specific Researchable How good is the grade XI mastery of class XI material? Is there a difference in the problem-solving abilities of students who got X learning better than students who got Y learning? Is there a relationship between student achievement and the level of student anxiety? [email protected] What is a hypothesis? A hypothesis is a tentative statement saying what you expect to find in your research The hypothesis is formulated based on existing knowledge A hypothesis is a statement and not a question [email protected] Good hypotheses Good hypotheses are: Specific Testable Testable: “skipping class results in lower grades” Not testable: “It doesn't matter whether or not you skip class” Falsifiable – it should be possible to demonstrate that the hypothesis is false “Aliens exist” - inspecting every inch of the universe for absence of life is not feasible Includes independent (manipulated) and dependent (measured) variables [email protected] Types of hypotheses Hypotheses can be: Descriptive “Mastery of class X material by class XI students reaches 75%” Comparative “The problem solving ability of students who get learning X is better than students who get learning Y” Associative “There is a negative relationship between student achievement with the level of student anxiety” [email protected] The NULL hypothesis Null hypotheses are used in statistical hypothesis testing The null hypothesis can be thought of as the hypothesis that nothing is going on or being different The alternative hypothesis is that there is something going on or different Question: Are there differences in the critical thinking skills of students who study during the day are better than students who study in the morning? Null hypothesis: There is no difference in the critical thinking skills of students who study in the afternoon with students who study in the morning Alternative hypothesis: The critical thinking skills of students who study in the afternoon are different from students who study in the morning. [email protected] Testable predictions It is often useful to make predictions based on your research questions and hypothesis to guide experiments A testable prediction is a prediction of relationships that you would expect to observe if your hypothesis was true Observation: While watching chimpanzees at the zoo you notice that their activity level varies greatly over the course of the day Question: Why does chimp activity vary during the day? Hypothesis: Chimp activity pattern is affected by feeding regime Testable prediction: The fraction of time that a chimp spends moving around will be higher in the hour around feeding time than other times of day [email protected] Testable predictions Good predictions follow logically from the hypothesis Good predictions lead to obvious experiments that allow the prediction to be tested – it should be obvious what data we need to collect Note that a prediction is often included in the hypothesis Question Hypothesis Prediction [email protected] Multiple hypotheses Research questions can often lead to multiple hypotheses and predictions Common whelks (Buccinum undatum; sea snails) grouped together on a rock Observation: Whelks on rocks often occur in groups Question: Why do whelks group? [email protected] Multiple hypotheses Hypothesis 1: Whelks group for shelter from wave action Testable prediction 1: Whelks are more likely to be found in groups in areas sheltered from wave action Hypothesis 2: Whelks group for feeding Testable prediction 1: Whelks are more likely to be found in groups in areas of higher food density Note that both hypothesis could be true and research questions often requires many hypotheses and predictions to be tested [email protected] Testable predictions It is often useful to try out different ways to formulate predictions to find how to best test the hypothesis by experiments. Try out your prediction as a “if… then…” statement, “correlation” or “comparison”. Question Do students who attend more lectures get better exam results? Hypothesis Attending more lectures leads to better exam results. If… then… If a first-year student starts attending more lectures, then their exam scores will improve. Testable Correlation/effect The number of lectures attended by first-year students has predictions a positive effect on their exam scores. Comparison First-year students who attended most lectures will have better exam scores than those who attended few lectures. [email protected] The Nobel laurate and theoretical physicist Richard Feynman was/is one of the world’s most well-known scientists not only because of his many important contributions to science, but also because he had an ability to explain very complicated things simply. His taken on the scientific method is: Compare with: 1. Guess! 1. Hypothesis 2. Compute consequences 2. Testable prediction Richard Feynman 3. Compare to experiments or nature 3. Experiment (1918-1988) https://www.youtube.com/watch?v=EYPapE-3FRw [email protected] The Scientific Method summarized Observation Research Hypothesis Prediction Share results Conclusion Analysis Experiment ✓ Reject [email protected] Experimental design [email protected] Is there a ”reproducibility crisis”? 1576 researchers surveyed by Nature Don't know No, there is no 7% crisis 3% Yes, a significant Yes, a slight crisis crisis 52% 38% [email protected] Baker (2016) Nature, 533, 452-454 Potential causes? Solutions? Yes, there is a widely acknowledged "reproducibility crisis" in science, particularly in fields like psychology, medicine, and the social sciences. The crisis refers to the growing concern that many scientific studies cannot be reproduced or replicated by other researchers, which challenges the reliability of published findings. Potential Causes of the Reproducibility Crisis Selective Reporting and Publication Bias: Selective Reporting: Researchers may selectively report only positive or significant results, ignoring negative or null findings. This practice leads to a skewed understanding of the research landscape. Publication Bias: Journals often favor publishing studies with positive or novel results over those with negative or replication studies. This can result in an overrepresentation of "exciting" findings that might not be robust. P-Hacking and Data Manipulation: P-Hacking: Researchers may manipulate data analysis until they find statistically significant results (e.g., p < 0.05), even if these results are not truly meaningful. This practice inflates the likelihood of false positives. Data Manipulation: Some researchers may consciously or unconsciously adjust their data or analysis methods to achieve desired outcomes, leading to unreliable conclusions. Small Sample Sizes and Underpowered Studies: Small Sample Sizes: Many studies, especially in fields like psychology, use small sample sizes, which increases the risk of statistical noise and reduces the power to detect true effects. Underpowered Studies: Studies with low statistical power are more likely to produce false positives or false negatives, making their results less reliable. Lack of Transparency and Full Disclosure: Incomplete Reporting: Some studies fail to fully disclose methods, data, or analysis procedures, making it difficult for others to replicate the work. Lack of Data Sharing: Without access to raw data, other researchers cannot verify or build upon previous findings, hampering reproducibility. Complexity of Experimental Design: Complex Methods: Highly complex statistical methods or experimental designs may be difficult for other researchers to understand or replicate accurately. Variability in Protocols: Differences in how experiments are conducted, even if minor, can lead to different outcomes, especially in biological and social sciences. Pressure to Publish: Academic Pressure: The "publish or perish" culture in academia pressures researchers to produce significant findings quickly, which may lead to cutting corners, p-hacking, or other questionable research practices. Career Advancement: Researchers may feel pressured to produce novel or groundbreaking results to secure funding, tenure, or promotions, potentially leading to compromised research integrity. [email protected] Potential Solutions to the Reproducibility Crisis Pre-registration of Studies: Pre-registration: Researchers can pre-register their study designs, hypotheses, and analysis plans before collecting data. This reduces the likelihood of p-hacking and selective reporting, as the research plan is publicly documented in advance. Encouraging Replication Studies: Replication: Journals and funding agencies should encourage and support replication studies, which are crucial for verifying the robustness of findings. Replication should be valued as much as original research in terms of academic recognition and funding. Improving Transparency and Open Science Practices: Open Data: Researchers should share their raw data, analysis code, and full methodological details to allow others to verify their work. Open Access Publishing: Making research freely accessible can increase scrutiny and encourage more independent verification of results. Use of Larger Sample Sizes and Meta-Analyses: Larger Samples: Increasing sample sizes can improve the power of studies, reducing the risk of false positives and increasing the reliability of findings. Meta-Analysis: Conducting meta-analyses, which combine data from multiple studies, can help identify true effects and provide a more accurate picture of the evidence. Statistical Reforms: Use of Bayesian Statistics: Bayesian approaches can provide more nuanced interpretations of data, considering prior evidence and reducing over-reliance on p- values. Improved Statistical Training: Researchers should receive better training in statistics and research methods to understand the limitations and proper use of statistical tools. Cultural and Institutional Change: Changing Incentives: Academic institutions and funding bodies should reward quality and reproducibility over quantity and novelty. This could include recognizing replication studies, negative results, and rigorous methodological work. Peer Review Reform: Improving the peer review process to include checks for reproducibility, such as requiring reviewers to assess whether the methods are clear and whether the study can be independently replicated. Summary The reproducibility crisis is a significant issue in contemporary science, with multiple causes ranging from methodological flaws to cultural pressures in academia. Addressing the crisis requires concerted efforts to improve transparency, encourage replication, adopt better statistical practices, and shift academic incentives towards valuing robust, reliable research over merely novel or significant findings. The importance of experimental design Most scientists agree that poor reproducibility of scientific results is major problem Better mentorship, proper use of statistics and more robust experimental design are ways to counter the so called “reproducibility crisis” [email protected] Criteria for complying with the scientific method 1. Study important problems 2. Build on prior knowledge 3. Provide full disclosure 4. Use objective designs 5. Use valid and reliable data 6. Use valid simple methods 7. Use experimental evidence 8. Draw logical conclusions [email protected] Armstrong (2022) The scientific method – a guide to finding useful knowledge, Oxford university press, https://doi.org/10.1017/9781009092265 The importance of experimental design Scientific knowledge is built one “brick” at a time Each “brick” can lead to new questions and directions of research It is critical that each “brick” be as robust as possible to provide a solid foundation for the next line of investigation A solid foundation is built by careful experimental design Research result Research result [email protected] Issues contributing to poor reproducibility Pressure from advisors and stakeholders to find something “Publish or perish”-pressure Incomplete understanding of fast-evolving new technologies Poor analysis of the large datasets in the omics era Poor hypotheses Can be addressed Poor experimental design by improved Poor sampling experimental Low statistical power Various biases and systematic errors design Poorly controlled studies Poor statistical analysis of data P-hacking Selective reporting (“cherry picking”) of results [email protected] "Publish or Perish" Pressure: The emphasis on publication and the need for a continuous stream of publications can lead to rushed or incomplete research, compromising the quality and reproducibility of the work. Incomplete Understanding of Fast-Evolving Technologies: Rapid advancements in technology can outpace researchers' understanding, leading to misuse or misinterpretation of new tools, methods, or data. Poor Analysis of Large Datasets in the Omics Era: The complexity and volume of data generated in the omics era can pose challenges for analysis. Inadequate analytical methods may lead to misinterpretation or selective reporting. Poor Hypotheses: Formulating vague or biased hypotheses can lead to experimental designs that lack focus or relevance, contributing to weak and irreproducible results. Poor Experimental Design: Flawed experimental designs, such as insufficient controls, inappropriate sample sizes, or lack of randomization, can compromise the validity and reproducibility of findings. Various Biases and Systematic Errors: Biases, whether conscious or unconscious, can affect study design, data collection, and interpretation, leading to skewed results and poor reproducibility. Poorly Controlled Studies: Lack of control over confounding variables can introduce variability and uncertainty, making it difficult to reproduce results consistently. Poor Sampling: Inadequate or biased sampling methods can limit the generalizability of findings and contribute to variability between studies. Low Statistical Power: Insufficient sample sizes and low statistical power reduce the ability to detect true effects, increasing the likelihood of false-positive or false-negative results. Poor Statistical Analysis of Data: Inaccurate or inappropriate statistical methods can lead to incorrect conclusions and hinder the reproducibility of study findings. P-Hacking: Manipulating statistical analyses to achieve significant results (p-hacking) can lead to overestimation of effects and hinder reproducibility. Selective Reporting ("Cherry Picking") of Results: Choosing to report only statistically significant results while neglecting non-significant findings can create a biased and incomplete picture of the research, impacting reproducibility. Addressing these issues requires a combination of improved research practices, transparency, and a cultural shift in academia towards valuing rigorous and reproducible science over quantity of publications. Yes, a better understanding and use of statistics can significantly improve reproducibility and help address issues related to poor sampling, low statistical power, poor statistical analysis of data, and p-hacking. Here's how: Poor Sampling: Statistical Solution: Understanding statistical sampling methods is crucial for ensuring representative and unbiased samples. Techniques such as random sampling can help create a sample that is more likely to reflect the characteristics of the overall population. Improvement: Statistical methods, such as confidence intervals, can provide a measure of the precision of estimates based on the sample. A better understanding of these concepts can guide researchers in interpreting and reporting the reliability of their findings. Low Statistical Power: Statistical Solution: Statistical power calculations help researchers determine the required sample size to detect a meaningful effect. By understanding power analysis, researchers can ensure that their studies are adequately powered to detect real effects. Improvement: Researchers can prioritize larger sample sizes and robust study designs to increase statistical power, making their studies more likely to detect true effects. Poor Statistical Analysis of Data: Statistical Solution: A solid understanding of statistical methods is essential to perform appropriate analyses. Researchers should choose statistical tests that match the study design, avoid common pitfalls, and use correct assumptions. Improvement: Collaboration with statisticians during study design and data analysis stages can enhance the quality of statistical analyses. Additionally, transparent reporting of methods and results facilitates scrutiny and replication by others. P-hacking: Statistical Solution: Transparency and adherence to statistical principles can mitigate the risk of p-hacking. Pre-registering study protocols, specifying analysis plans in advance, and reporting all conducted analyses can help prevent selective reporting. Improvement: Researchers should focus on effect sizes and confidence intervals rather than relying solely on p-values. A deeper understanding of statistical concepts, such as the difference between exploratory and confirmatory analyses, can contribute to more reliable research. In summary, a better understanding and application of statistical methods are fundamental to improving the reproducibility of scientific research. Researchers who employ robust statistical techniques, adhere to best practices, and prioritize transparency contribute to a more reliable scientific literature. Collaborating with statisticians and continuously educating researchers about statistical concepts can further enhance the rigor and reproducibility of research findings. Experimental and observational studies Experimental studies Observational studies Variables can be controlled and No direct control of variables manipulated Can show associations, but not Manipulation of variables can show cause-and-effect-relationships cause-and-effect-relationships Generally needs more data to find significant associations In focus this week Focus for next week [email protected] They differ in their approach to data collection, the level of control over variables, and the ability to establish causation. Here are the key differences between experimental and observational studies: 1. Purpose: Experimental Studies: Purpose: To investigate cause-and-effect relationships. Manipulation: Researchers manipulate one or more independent variables to observe the effect on the dependent variable. Observational Studies: Purpose: To observe and describe phenomena without manipulating variables. Observation: Researchers observe and collect data on naturally occurring behaviors or conditions. 2. Control: Experimental Studies: Control: Researchers have control over experimental conditions, allowing them to isolate variables and establish causation. Observational Studies: Control: Researchers have limited control over variables, as they are observing events as they naturally occur. 3. Randomization: Experimental Studies:Randomization: Participants are often randomly assigned to different experimental conditions to control for potential confounding variables. Observational Studies:Randomization: Typically, there is no random assignment of participants to groups, and researchers must rely on statistical methods to control for confounding variables. 4. Intervention: Experimental Studies:Intervention: Researchers introduce an intervention or treatment to observe its effects. Observational Studies:Intervention: There is no intentional intervention by the researcher; observations are made in a natural setting. 5. Causation: Experimental Studies:Causation: Because of the controlled nature of experiments, there is a stronger basis for establishing causal relationships. Observational Studies:Causation: While observational studies can identify associations, establishing causation is more challenging due to the potential influence of confounding variables. 6. Examples: Experimental Studies: Example: A clinical trial testing the effectiveness of a new drug by randomly assigning participants to a treatment or control group. Observational Studies:Example: Studying the relationship between smoking and lung cancer by observing and collecting data on individuals in their natural environment without any intervention. 7. Ethical Considerations: Experimental Studies: Ethical Considerations: Depending on the nature of the intervention, ethical considerations may arise, especially if participants are exposed to potential risks. Observational Studies:Ethical Considerations: Generally, observational studies involve less ethical concern since researchers are observing rather than intervening directly. In summary, experimental studies involve the manipulation of variables to establish cause-and-effect relationships, while observational studies involve the observation and description of naturally occurring phenomena without direct manipulation. Both study types have their strengths and limitations, and the choice between them depends on the research question, feasibility, and ethical considerations. Elements of an experimental study Experimental units Treatments Responses Objects or subjects Procedures applied to Measurements to under study experimental units compare the treatments [email protected] Random and systematic errors All measurements contain errors! Experimental design aims to reduce errors Random errors: Systematic errors (bias): Are random fluctuations introduced by Are consistent patterns in the data methods and natural variation in that are not due to chance samples Are not handled by statistical Are handled by statistical analysis analysis Can be difficult to detect Can be removed by good experimental design [email protected] Random and systematic errors All measurements contain errors! Random errors: Systematic errors (bias): Are random fluctuations introduced by Are consistent patterns in the data methods and natural variation in that are not due to chance samples Are not handled by statistical Are handled by statistical analysis analysis Can be difficult to detect Can be removed by good experimental design Minimizing errors increases the likelihood for discovery! [email protected] Instrumental Variability: Systematic Errors: Example: A laboratory balance might have slight variations in its readings due to Calibration Issues: imperfections or fluctuations in its internal mechanisms. These variations are random errors. Example: A thermometer consistently reads temperatures higher or Environmental Variability: lower than the actual values because it is not properly calibrated. This consistent offset is a systematic error. Example: Temperature fluctuations in a lab environment can cause a reaction to proceed at Zero Error: slightly different rates during different measurements, leading to random errors. Human Errors: Example: A scale that consistently reads a value when there is nothing on it has a zero error. This systematic offset affects all measurements Example: Inconsistent reading of a measuring instrument by different individuals or by the made with that scale. same person at different times can introduce random errors. Parallax Error: Electronic Noise: Example: Reading the level of liquid in a graduated cylinder from an Example: In electronic measurements, random electrical noise can affect the precision of angle rather than at eye level can introduce a consistent error in readings, especially in sensitive instruments like oscilloscopes or sensors. volume measurements due to parallax. Sampling Variability: Instrumental Drift: Example: When collecting data from a population, random sampling errors can occur. For Example: Over time, the sensitivity or accuracy of an instrument may instance, if a small sample is not representative of the entire population, the results may change, leading to a systematic shift in measurements even when used have variability due to chance. under the same conditions. Biological Variability: Environmental Drift: Example: In biological experiments, the inherent variability among individual organisms or Example: Changes in temperature, humidity, or pressure in the cells can introduce random errors in measurements. laboratory affecting measurements consistently over time represent Measurement Fluctuations: systematic errors. Procedural Errors: Example: Fluctuations in the measurement of the same quantity multiple times due to inherent variations in the measuring process represent random errors. Example: Following an incorrect procedure in every experiment can introduce a systematic error. For instance, if a step is consistently omitted or done incorrectly, it affects all measurements. Selection Bias: Example: If only certain subjects or samples are chosen for an experiment, and these choices consistently affect the results, it introduces systematic bias into the study. It's important to note that while random errors can be reduced by increasing sample size or conducting multiple measurements, systematic errors often require identifying and correcting the underlying cause to improve the accuracy of measurements. Examples of Random errors Systematic errors [email protected] Random Errors: Definition: Random errors are unpredictable fluctuations in measurements that occur due to various sources of variability. They are inherent in any measurement process and can affect precision. Examples: Instrumental Variability: Variations in instrument readings due to imperfections or fluctuations. Environmental Variability: Fluctuations in temperature, humidity, or other environmental conditions affecting measurements. Sampling Variability: Differences between individual samples even when drawn from the same population. Reducing Random Errors: Replication: Conducting multiple measurements or experiments to average out random variations. Randomization: Randomly assigning experimental conditions or treatment groups to control for unknown sources of variability. Increased Sample Size: Larger sample sizes can help reduce the impact of random errors. Systematic Errors: Definition: Systematic errors are consistent biases or inaccuracies in measurements that occur due to flaws in the experimental setup, instruments, or procedures. They affect accuracy. Examples: Calibration Issues: Inaccuracies in instrument calibration leading to consistently high or low measurements. Zero Error: A constant offset in measurement instruments, even in the absence of the measured quantity. Selection Bias: Systematic differences between groups due to non-random selection. Reducing Systematic Errors: Calibration: Regularly calibrating instruments to ensure accuracy. Blinding: Conducting experiments without knowledge of certain conditions to prevent unintentional biases. Matching: Matching subjects or samples on relevant variables to control for potential confounders. Randomization: Randomly assigning subjects or experimental units to conditions to control for unknown factors. Standardized Protocols: Using standardized procedures to minimize variability introduced by different experimenters. Matching: Definition: Matching involves pairing subjects or experimental units based on relevant variables to control for potential confounders. Reducing Errors: Example: In a clinical trial, matching patients based on age, gender, and other relevant factors can control for variability and reduce confounding. Blinding: Definition: Blinding involves conducting experiments without certain individuals being aware of specific conditions, treatments, or measurements. Reducing Errors: Example: Double-blind experiments, where both experimenters and participants are unaware of who is receiving a treatment, can help prevent biases in data collection and interpretation. Replication: Definition: Replication involves conducting experiments multiple times to obtain consistent results. Reducing Errors: Example: Performing the same experiment under identical conditions multiple times to assess the reliability and reproducibility of results. Randomization: Definition: Randomization involves the random assignment of subjects or experimental units to different conditions or treatment groups. Reducing Errors: Example: Randomly assigning patients to treatment or control groups in a clinical trial helps control for both known and unknown sources of variability. In summary, a combination of replication, randomization, blinding, and matching can be employed to reduce both random and systematic errors, improving the reliability and validity of experimental results. Dealing with errors in scientific experiments is crucial to ensure the reliability and validity of the results. Here are some strategies commonly employed to address errors in the context of randomization, matching, replication, and blinding: Randomization: Random Sampling of Experimental Units: Purpose: To reduce selection bias and ensure that the sample is representative of the population. Implementation: Use random methods (e.g., random number generators) to select experimental units from the population. Random Allocation of Treatments: Purpose: To minimize the impact of confounding variables and ensure that treatment groups are comparable. Implementation: Randomly assign experimental units to different treatment groups. Random Order of Measurements: Purpose: To control for temporal effects and minimize order effects in experimental designs. Implementation: Randomize the order in which measurements are taken across experimental units. Matching: Within-Subject Design: Purpose: Controls for individual variability by having each experimental unit act as both a test and control. Implementation: Each subject receives both the treatment and control conditions in a randomized order. Between-Subject Design: Purpose: Controls for confounding variables by matching subjects on relevant characteristics. Implementation: Match subjects based on key variables (e.g., age, gender) to reduce variability between treatment groups. Replication: Intra-Observer Variability: Purpose: To assess and control for variations introduced by a single observer. Implementation: Have the same observer measure the same samples multiple times, and calculate the intra-observer variability. Inter-Observer Variability: Purpose: To account for variations introduced by different observers. Implementation: Have multiple observers measure the same samples independently to assess and control for inter-observer variability. Technical Replicates: Purpose: To account for method, instrument, and pipetting errors. Implementation: Conduct multiple measurements or analyses on the same sample using the same method or instrument to assess technical variability. Biological Replicates: Purpose: To account for variation in the experimental units. Implementation: Use multiple independent samples or organisms for each experimental condition to capture inherent biological variability. Blinding: Purpose: To prevent unintentional bias in the interpretation or collection of data. Implementation: Keep experimenters, observers, or participants unaware of certain experimental conditions, treatments, or outcomes to reduce the risk of bias. By incorporating these strategies, researchers can enhance the robustness of their experimental designs, minimize various sources of error, and improve the reproducibility and reliability of their findings. It's important to carefully plan and document these strategies throughout the experimental process. How to deal with errors Randomization Replication Random sampling of experimental units Intra-observer variability Random allocation of treatments variations in measurements made by a single Random order of measurements individual Inter-observer variability variation introduced by having multiple Matching individuals contributing measurements Within-subject design Technical replicates where the same experimental unit acts as method, instrument and pipetting errors both test and control Biological replicates Between-subject design variation in the experimental units subject should be matched on as many variables as possible to reduce variation Blinding To avoid unintentional influence by the experimenter [email protected] Dealing with errors in scientific experiments is crucial to ensure the reliability and validity of the results. Here are some strategies commonly employed to address errors in the context of randomization, matching, replication, and blinding: Randomization: Random Sampling of Experimental Units: Purpose: To reduce selection bias and ensure that the sample is representative of the population. Implementation: Use random methods (e.g., random number generators) to select experimental units from the population. Random Allocation of Treatments: Purpose: To minimize the impact of confounding variables and ensure that treatment groups are comparable. Implementation: Randomly assign experimental units to different treatment groups. Random Order of Measurements: Purpose: To control for temporal effects and minimize order effects in experimental designs. Implementation: Randomize the order in which measurements are taken across experimental units. Matching: Within-Subject Design: Purpose: Controls for individual variability by having each experimental unit act as both a test and control. Implementation: Each subject receives both the treatment and control conditions in a randomized order. Between-Subject Design: Purpose: Controls for confounding variables by matching subjects on relevant characteristics. Implementation: Match subjects based on key variables (e.g., age, gender) to reduce variability between treatment groups. Replication: Intra-Observer Variability: Purpose: To assess and control for variations introduced by a single observer. Implementation: Have the same observer measure the same samples multiple times, and calculate the intra-observer variability. Inter-Observer Variability: Purpose: To account for variations introduced by different observers. Implementation: Have multiple observers measure the same samples independently to assess and control for inter-observer variability. Technical Replicates: Purpose: To account for method, instrument, and pipetting errors. Implementation: Conduct multiple measurements or analyses on the same sample using the same method or instrument to assess technical variability. Biological Replicates: Purpose: To account for variation in the experimental units. Implementation: Use multiple independent samples or organisms for each experimental condition to capture inherent biological variability. Blinding: Purpose: To prevent unintentional bias in the interpretation or collection of data. Implementation: Keep experimenters, observers, or participants unaware of certain experimental conditions, treatments, or outcomes to reduce the risk of bias. By incorporating these strategies, researchers can enhance the robustness of their experimental designs, minimize various sources of error, and improve the reproducibility and reliability of their findings. It's important to carefully plan and document these strategies throughout the experimental process. How to determine appropriate sample size? The number of replicates needed to maximize the chance of being able to find statistical significance can be estimated by educated guesswork or preferably by a formal power analysis Educated guesswork Power analysis Read published studies to find Power is the chance to obtain a results of the how much variability and how desired significance level (typically 0.8 or 80%) large of an effect size is to be To calculate the sample size we need estimates expected of the effect size (d), variability (SD) and decide Decide if the number of on level of power and significance level replicates other studies have For a type I error rate of 0.05 used will suffice for your and power of 0.8: experiment 2×𝑆𝐷2 (𝑍 𝛼 +𝑍1−𝛽 ) 2 1− 2 2×𝑆𝐷2 (1.96+0.84)2 𝑛= 𝑛= 𝑑2 𝑑2 [email protected] Variables Independent variables are the manipulated or experimental variables Dependent variables are the measured variables that dependend on the manipulated variable Confounding variables are variables related to the variables under investigation in such a way that it makes it impossible to distinguish whether the effect is caused by one of the measured variables or the confounding variable e.g. the order in which the samples were measured if controls were measured first before treated samples then treated samples might differ because they sat around longer on the lab bench or the measurement equipment changes as it warms up during the course of a day Variables can be: Controlled Variables controlled within the experiment such as e.g. incubation temperatures, treatment times Uncontrolled Variables outside our control, which may still affect the outcome e.g. humidity, room temperature [email protected] Controls Before the experiment Included in the experiment Equipment precision and Some reagent quality controls are often included accuracy Specificity of antibodies Reagent quality Negative controls Specificity of antibodies Without exposure to independent (manipulated) variable Storage and expiration dates Positive controls Helps to decide if negative results are due to a technically failed assay Also helps to gage the effect size in relation to a known positive control [email protected] Warning signs in experimental design Lack of randomization Lacking repeatability and Lack of blinding reproducibility Too few experimental units Publication bias Wrong experimental units Ignoring other sources of bias Wrong questions Taking the p value too seriously Wrong statistics Accepting the wrong p value Lack of specific hypothesis, Confusing correlation with causation overzealous data mining Lack of theory Lack of controls [email protected] Descriptive statistics [email protected] The importance of statistics Most scientists agree that poor reproducibility of scientific results is major problem Better mentorship, proper use of statistics and more robust experimental design are ways to counter the so called “reproducibility crisis” https://www.youtube.com/watch?v=j7K3s_vi_1Y [email protected] Descriptive statistics All measurements (or data points calculated from raw data) reported in a table are difficult absorb and interpret After performing an experiment, the data needs Control Treatment X Treatment Y to be summarized and plotted in graphs to be 0.925 1.51631 21.597 digested and understood. 8.3 15.31618 36.656 3.825 3.466842 18.1984 9.428 4.314396 12.98 What innate qualities in measured 12.182 1.017046 42.543 data are important and needs to be 4.796 34.59 described or summarized? 6.038516 56.9825 4.797 43.454 7.438043 38.454 There are 4 important qualities of 9.383191 data that needs to be reported! 7.502 9.776 7.8 [email protected] Descriptive statistics 4 innate qualities of data 1. 2. 3. 4. Measure of central Measure of Number of Effect size: tendency: variation: measurements: Fold change Average/mean Standard Biological Percent increase Median deviation (SD) replicates Cohen’s d Inter-quartile Technical Odds ratio (OR) range (IQR) replicates Relative risk or risk Range ratio (RR) Correlation coefficient (r ) Coefficient of determination (r 2) [email protected] Types of data Type Description Example Categorical (nominal) data Non-numerical Sex, age group Ranked (ordinal) data Categories in an ordered Customer satisfaction position score, education level Discrete data Data can only take specific Number of children, number values in a given range of correct answers on a test, number of siblings Continuous (quantitative) Data can take any value in a Weight of newborn babies, data given range body temperature, blood cell count [email protected] Distributions of data Is data evenly distributed? Is the distribution symmetric? Are there outliers? Normal distribution Skewed distribution [email protected] Understanding the characteristics of data distributions, such as normal distribution and skewed distribution, involves assessing whether the data is evenly distributed, whether the distribution is symmetric, and whether there are outliers. Let's explore these concepts: Normal Distribution: Assessing Distributions: Evenly Distributed: In a normal distribution, data is evenly spread around the mean, creating a symmetrical bell-shaped curve. The Histograms: Visual representations of the data distribution can be created using majority of data points cluster around the mean, with fewer data points histograms. Normal distributions appear as a bell-shaped curve, while skewed in the tails. distributions show a longer tail on one side. Symmetric: A normal distribution is symmetric, meaning that if you were Measures of Central Tendency: to fold the distribution along the vertical line at the mean, the two halves In a normal distribution, the mean, median, and mode are approximately equal. would be nearly identical. In skewed distributions, these measures may differ, with the mean being more influenced by outliers. Outliers: Outliers in a normal distribution are relatively rare and are typically found in the tails. Data points that deviate significantly from the Quantile-Quantile (Q-Q) Plots: These plots compare the quantiles of the observed mean may be considered outliers. data with the quantiles of a theoretical normal distribution. Deviations from a straight line suggest departures from normality. Skewed Distribution: Evenly Distributed: In a skewed distribution, data is not evenly spread. In summary, understanding the distribution of data involves examining its Skewness indicates a lack of symmetry. evenness, symmetry, and the presence of outliers. Normal distributions are characterized by evenness and symmetry, while skewed distributions have a lack Symmetric: A skewed distribution is not symmetric. It has a longer tail of symmetry and may exhibit a longer tail on one side. Outliers can be present in on one side than the other. both types of distributions but are more typical in skewed distributions. Visualizations and summary statistics are useful tools for assessing these Outliers: Skewed distributions may have outliers, but they are more characteristics. likely to be found in the longer tail of the distribution. Types of Skewness: Positive Skew (Right Skew): The tail on the right side is longer. The mean is typically greater than the median. Example: Income distribution, where a few people have very high incomes. Negative Skew (Left Skew): The tail on the left side is longer. The mean is typically less than the median. Example: Test scores, where a few students score very low. Measures of central tendency - mean or median? Mean Median 25 25 Use median when the data distribution 20 20 is skewed, as the median is a better G lu k o s uuptake G lu k o s uuptake p p ta g p p ta g 15 15 representation of where most values fall in a skewed distributon Glucose Glucose 10 10 5 5 0 0 O b e h a n d la d B e h a n d la d O b e h a n d la d B e h a n d la d Untreated Treated Untreated Treated The bar represent the mean or median [email protected] Measures of central tendency - mean or median? Standard error of the mean 20 20 mean ± sem mean ± sd the median is a better Glucose uptake Glucose uptake 15 15 representation of where 10 10 most values fall in a 5 5 skewed distribution 0 0 Control Treated Control Treated 20 20 the mean±sem gives the mean median false impression that Glucose uptake Glucose uptake 15 15 10 10 there is a difference 5 5 between control and treatment 0 0 Control Treated Control Treated [email protected] Measures of variability - SD or sem? The standard error of the mean (sem) describes mean ± sd mean ± sem the variation of the mean n=7 n=26 15 15 15 if you would repeat the same experiment 10 10 10 Response Response Response 10±0.4 10±2.2 10±1.0 𝑆𝐷 5 5 10±2.6 5 𝑆𝐸𝑀 = 𝑛 0 0 0 l l l A A A o o o tr tr tr t t t en en en on on on tm tm tm C C C ea ea ea Tr Tr Tr The standard deviation describes the variation of the data points Note that the standard deviation does not change The standard error of the mean , around the mean, which is what much by increasing the however, becomes smaller when the we are most often interested in number of measurements number of measurements increases when reporting experiments [email protected] Measures of variability - SD or sem? mean ± sd mean ± sem mean ± sem 20 20 20 20 15 15 15 15 Response Response Response Response 10 10 10 10 5 5 5 5 0 0 0 0 l B o l B l l B B o tr o o t en tr tr tr t on t t en en en on on on tm C tm tm tm C C C ea ea ea ea Tr Tr Tr Tr The sd represents the variation in the Reporting sem instead of sd can experiment well lead to a false impression that there was a difference in means, and no There is a difference in means, but there is overlap of data points also a large overlap in the data points [email protected] Effect size is a statistical concept used to quantify the magnitude or strength of a relationship or difference between two groups or conditions. It provides a standardized measure that allows researchers to assess the practical significance of their findings, independent of sample size. Measures of variation provide information about the spread or dispersion of a set of data points. Here are explanations of the three listed measures of variation: Here's a brief explanation of each of the listed effect size measures: Standard Deviation (SD): The standard deviation is a measure of the amount of variation Fold Change: This is commonly used in genetics and molecular biology to represent the ratio of change in a or dispersion in a set of values. It quantifies how much individual data points differ from particular measure between two conditions or groups. For example, if the expression of a gene doubles, the fold the mean (average) of the dataset. A larger standard deviation indicates greater change would be 2. variability, while a smaller standard deviation suggests that the data points are closer to the mean. Percent Increase: This represents the proportional increase in one variable compared to another. It is calculated by taking the difference between the initial and final values, dividing by the initial value, and then multiplying by Interquartile Range (IQR): The interquartile range is a measure of statistical dispersion, 100. specifically the range within which the middle 50% of the data values lie. It is calculated as the difference between the third quartile (Q3) and the first quartile (Q1) in a dataset. Cohen's d: This is a measure of the standardized difference between two means. It's particularly useful in The IQR is less sensitive to extreme values (outliers) than the range, making it useful in comparing means across different groups and is expressed in terms of standard deviations. situations where extreme values may skew the interpretation of variability. Odds Ratio (OR): Commonly used in epidemiology and binary outcomes, the odds ratio represents the odds of an Range: The range is the simplest measure of variation and is calculated as the difference event occurring in one group compared to another. between the maximum and minimum values in a dataset. While it provides a quick assessment of the spread of data, the range can be heavily influenced by extreme values Relative Risk or Risk Ratio (RR): Similar to the odds ratio, the relative risk is used in epidemiology to compare the (outliers), and it does not consider the distribution of values within that range. risk of an event occurring in one group to the risk in another group. In summary, the standard deviation provides a more comprehensive understanding of Correlation Coefficient (r): This measures the strength and direction of a linear relationship between two variables. the distribution of values by considering their distances from the mean. The interquartile The value of 'r' ranges from -1 to 1, where -1 indicates a perfect negative linear relationship, 1 indicates a perfect range, on the other hand, focuses on the central portion of the data, making it robust positive linear relationship, and 0 indicates no linear relationship. against outliers. The range is the simplest measure, but it can be sensitive to extreme values. The choice of which measure to use often depends on the characteristics of the Coefficient of Determination (r^2): Squaring the correlation coefficient, 'r', gives the coefficient of determination. It data and the specific goals of the analysis. represents the proportion of variance in one variable that is predictable from another variable. In other words, it indicates the proportion of variability in the dependent variable that is explained by the independent variable. In general, a larger effect size indicates a more substantial or meaningful difference or relationship. Researchers often consider both statistical significance and effect size when interpreting the results of a study. While statistical significance tells you if an effect is likely real, effect size helps you understand the practical importance of that effect. The measures of central tendency, such as the average (mean) and median, provide a summary of the central or typical value in a dataset. Here's an explanation of each: Average/Mean: The average, or mean, is calculated by adding up all the values in a dataset and then dividing by the number of values. It is sensitive to extreme values or outliers, as it takes into account the magnitude of each data point. The mean is a common measure of central tendency and is used in many statistical analyses. Median: The median is the middle value in a dataset when it is ordered from least to greatest. It is not affected by extreme values or outliers, making it a robust measure of central tendency. The median is particularly useful in skewed distributions or datasets with outliers, where the mean might be influenced by these extreme values. In summary, the choice between the mean and median depends on the characteristics of the data and the goals of the analysis. The mean is appropriate when the data is approximately symmetric and not heavily influenced by outliers. On the other hand, the median is a better choice when dealing with skewed distributions or datasets with outliers, as it provides a more robust measure of the central position. Effect size The effect size is the magnitude of the difference between groups The effect size can be reported as: mean difference % difference Fold increase/decrease [email protected] Sullivan et al. (2012) J Grad Med Educ 4: 279-282 Present data in figures Categorical data Continuous data Bar graph Scatter plot Box plot 300000 250000 200000 Measured output Measured output 200000 150000 100000 100000 50000 0 0 Control Treatment X Control Treatment X In papers, however, bar graphs are often used to present continuous data - why is that? [email protected] Bar graphs are poor at presenting continuous data Bar graphs assign importance to the height of the bar rather than focusing attention on how the difference between means compares to the range of values observed excludes values above the highest error bar that are observed in the sample includes low values that never occur in the sample [email protected] Weissgerber et al. (2017) J Biol Chem 292: 20592-20598 Bar graphs are poor at presenting continuous data Many different data distributions can lead to the same bar or line graph! Summarizing the data as bar graphs with mean and SE or SD often causes readers to wrongly infer that the data are normally distributed with no outliers Wilcoxon rank sum test = Mann Whitney U test [email protected] Weissgerber et al. (2017) J Biol Chem 292: 20592-20598 While bar graphs are commonly used to represent categorical data, they are indeed also used to present continuous data in scientific papers. The choice of using bar graphs for continuous data depends on the nature of the data, the specific research question, and the preferences of the researcher. Here are some reasons why bar graphs may be used for continuous data: Clarity and Simplicity: Bar graphs are straightforward and easy to understand. They provide a clear visual representation of data, making it accessible to a wide audience, including readers who may not have a strong background in statistics. Grouped Data: Bar graphs are suitable for displaying grouped or categorized continuous data. Each bar can represent a category or group, and the height of the bar can represent a summary statistic (e.g., mean, median) for that group. Comparisons Between Groups: Bar graphs make it easy to compare values between different groups or categories. This is particularly useful when researchers want to highlight differences or trends in the data. Facilitation of Grouping and Categorization: For continuous data that has been grouped or categorized into intervals or ranges, bar graphs are an effective way to visualize these groupings. Emphasis on Central Tendency: Bar graphs often emphasize measures of central tendency (e.g., mean) within each category. This can be useful when researchers want to highlight the central values or trends in the data. Commonly Accepted and Recognizable: Bar graphs are a familiar and widely accepted form of visualization. Readers are accustomed to interpreting bar graphs, making them a convenient choice for presenting data in academic publications. Complementing Inferential Statistics: Bar graphs can complement inferential statistical analyses. While detailed statistical results might be provided in the text or tables, a bar graph can offer a visual summary that is easier to interpret. It's important to note that the appropriateness of using bar graphs for continuous data depends on the specific goals of the analysis and the message the researcher wants to convey. In cases where more detailed information is needed, other types of visualizations, such as line plots, box plots, or histograms, may be more suitable for representing the distribution and variability of continuous data. The choice of visualization should align with the research objectives and effectively communicate the findings to the target audience.