Grp03 Article 1 Medication adherence in heart failure patients.pdf
Document Details
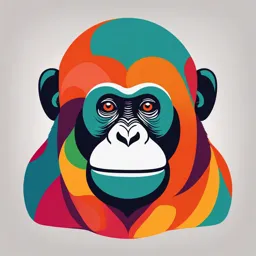
Uploaded by TrustingConcreteArt
2023
Tags
Full Transcript
Cheng et al. Journal of Pharmaceutical Journal of Pharmaceutical Policy and Practice (2023) 16:81 https://doi.org/10.1186/s40545-023-00582-9...
Cheng et al. Journal of Pharmaceutical Journal of Pharmaceutical Policy and Practice (2023) 16:81 https://doi.org/10.1186/s40545-023-00582-9 Policy and Practice REVIEW Open Access The use of technology to improve medication adherence in heart failure patients: a systematic review of randomised controlled trials Chloe Cheng1, Gemma Donovan2, Naseer Al‑Jawad3 and Zahraa Jalal1*    Abstract Background Heart failure is an ever-growing contributor to morbidity and mortality in the ageing population. Medication adherence rates among the HF population vary widely in the literature, with a reported range of 10–98%. Technologies have been developed to improve adherence to therapies and other clinical outcomes. Aims This systematic review aims to investigate the effect of different technologies on medication adherence in patients with heart failure. It also aims to determine their impact on other clinical outcomes and examine the poten‑ tial of these technologies in clinical practice. Methods This systematic review was conducted using the following databases: PubMed Central UK, Embase, MED‑ LINE, CINAHL Plus, PsycINFO and Cochrane Library until October 2022. Studies were included if they were randomised controlled trials that used technology to improve medication adherence as an outcome in heart failure patients. The Cochrane Collaboration’s Risk of Bias tool was used to assess individual studies. This review was registered with PROS‑ PERO (ID: CRD42022371865). Results A total of nine studies met the inclusion criteria. Two studies showed statistically significant improvement in medication adherence following their respective interventions. Eight studies had at least one statistically significant result in the other clinical outcomes it measured, including self-care, quality of life and hospitalisations. All studies that evaluated self-care management showed statistically significant improvement. Improvements in other outcomes, such as quality of life and hospitalisations, were inconsistent. Conclusion It is observable that there is limited evidence for using technology to improve medication adherence in heart failure patients. Further studies with larger study populations and validated self-reporting methods for medica‑ tion adherence are required. Keypoints The evidence in this review shows the potential for technology use in practice to improve health outcomes for patients with heart failure. Still, there is uncertainty in the evidence on its impact on medication adherence. *Correspondence: Zahraa Jalal [email protected] Full list of author information is available at the end of the article © The Author(s) 2023. Open Access This article is licensed under a Creative Commons Attribution 4.0 International License, which permits use, sharing, adaptation, distribution and reproduction in any medium or format, as long as you give appropriate credit to the original author(s) and the source, provide a link to the Creative Commons licence, and indicate if changes were made. The images or other third party material in this article are included in the article’s Creative Commons licence, unless indicated otherwise in a credit line to the material. If material is not included in the article’s Creative Commons licence and your intended use is not permitted by statutory regulation or exceeds the permitted use, you will need to obtain permission directly from the copyright holder. To view a copy of this licence, visit http://​creat​iveco​mmons.​org/​licen​ses/​by/4.​0/. The Creative Commons Public Domain Dedication waiver (http://​creat​iveco​ mmons.​org/​publi​cdoma​in/​zero/1.​0/) applies to the data made available in this article, unless otherwise stated in a credit line to the data. Cheng et al. Journal of Pharmaceutical Policy and Practice (2023) 16:81 Page 2 of 14 Technologies presented in the studies are generally acceptable for participants, which is informative for future implementations into practice. There was a lack of high-quality studies investigating technology’s use on medication adherence in heart failure. More extensive studies with improvements to study design are needed to ultimately conclude the effects on medication adherence. Keywords Medication adherence, Heart Failure, Technology, Self-care management Introduction application (app) technology; however, they only evalu- Heart failure (HF) is a debilitating chronic condition ated CVD as a whole. Even in systematic reviews with high morbidity and mortality affecting over 900,000 that studied technology use in HF, MA was not the pri- people in the UK, with a higher prevalence above age mary objective. There is also limited evidence assess- 65 [1–3]. Similarly, the population of HF patients per ing MA specifically in HF. Hence, this systematic year continues to increase. HF patients are roughly review aims to assess the impact of technology on MA in equally split between those with reduced Ejection Frac- HF patients. tion (HFrEF) and those with preserved or mildly reduced Ejection Fraction (HFpEF or HFmrEF). All three Aim types usually require pharmacological therapy to man- age symptoms, co-morbidities, and heart function. 1. To investigate the effect of different technologies on These include diuretics, Angiotensin-Converting Enzyme medication adherence in patients with heart failure. (ACE) inhibitors, beta-blockers, and Angiotensin Recep- 2. To determine the impact of different technologies on tor Blockers (ARBs). Medication Adherence (MA) is other clinical outcomes for heart failure patients and defined as "the extent to which a patient’s action matches examine the potential of these technologies in clini- the agreed recommendations". MA rates among cal practice. the HF population vary widely in the literature, with a reported range of 10–98% [6, 7]. Low adherence rates are associated with worse cardiac event-free survival, Methods all-cause mortality, cardiovascular hospitalisations, and This systematic review was conducted according to a more significant symptom burden [8, 9]. Studies have PRISMA guidelines and was registered with PROSPERO shown a greater rate of non-adherence in the elderly pop- (registration ID: CRD42022371865). ulation, potentially due to co-morbidities and polyphar- macy. On the other hand, high MA is linked to fewer Search strategy HF symptoms, lower rates of hospitalisation, and fewer An electronic search of the following databases was deaths. conducted for research articles: PubMed Central UK, MA is affected by multiple factors, including patient Embase (Ovid), MEDLINE (Ovid), CINAHL Plus beliefs, socioeconomic influences, and the type of pre- (EBSCO), PsycINFO (Ovid) and Cochrane Library, from scribed therapies. Educational interventions to 2000 until October 2022. The keywords’ heart failure’, address these issues have been shown to be effective. ’medication adherence’, and ’technology’ were used in this However, this does not guarantee that medications will review. Filters for Randomised Controlled Trials (RCTs) be taken, especially if regimens require multiple doses and the English language were applied. The MeSH term’s each day or a patient has various medications to take. function was utilised to generate other keywords for Interventions to tackle the lack of medication adherence each database (see example search strategy in Additional have been the focus of technological advancements, for file 1: Table S1. Reference lists of included studies were example, mobile applications, electronic pill boxes, auto- reviewed to identify additional relevant RCTs. mated telephone calls, and messaging. Previous trials using these technologies have shown significant increases Study inclusion criteria in refilling medications, specifically in patients with car- Studies that were RCTs and included technology as an diovascular diseases (CVD) such as hypercholesterolae- intervention to improve adherence to HF medication mia, hypertension, and coronary heart disease. were included. Studies were required to have a usual Systematic reviews have previously evaluated specific care control group. Studies with patients aged 18 or over technological interventions such as mobile health and were included, and participants could be of any class of Cheng et al. Journal of Pharmaceutical Policy and Practice (2023) 16:81 Page 3 of 14 the New York Heart Association (NYHA) classification due to reasons listed in the PRISMA diagram in Fig. 1. of HF. Studies needed to include patients taking at least Subsequently, nine studies were included [15–23]. Six one medication related to HF and have a precise MA out- of the nine studies were RCTs [18–23], two studies were come measure. Studies involving patients under the age pilot RCTs [15, 16], and one study was a randomised of 18 and non-RCTs: observational studies, systematic controlled feasibility trial. A meta-analysis was con- reviews, meta-analyses, grey literature, abstract available sidered; however, because of the heterogeneity of the out- only, unpublished studies, and quasi-randomised trials, come measure tools (specifically the self-reporting tools were excluded, along with those that were not in the Eng- used to measure medication adherence), and variations lish language. in other factors such as sample size and intervention length, this made it difficult to bring together an appro- Study selection and data extraction priate statistical analysis. All the identified studies were imported and intensively analysed to remove duplicate records manually. The first Study characteristics and design author (CC) screened all titles and abstracts using the Included studies were published between 2004 and 2022. inclusion criteria. This was checked by another author Seven studies were conducted in the United States [15– (ZJ). All researchers screened the full texts of the remain- 17, 19, 20, 22, 23]. One study was conducted in the Neth- ing articles independently to identify eligible studies (CC, erlands and another in Argentina [18, 21]. All studies GD, and ZJ). Any studies that displayed uncertainty were involved one or more intervention arms against a control discussed among all the authors to reach a consensus. All arm [15–23]. All study controls were usual care, defined relevant studies underwent data extraction for country of as participants undergoing follow-up without the inter- origin, number of study arms, intervention description, vention [15–23]. However, three studies gave their usual length of intervention, outcome measures, sample size, care participants the intervention technology dice to and results for comparison. either measure medication adherence as a study outcome or programmed it to be silent [16, 17, 19] All nine studies Outcomes assessed used a similar study design [15–23]. The sample size var- The primary outcome assessed was MA in HF. Secondary ied between 29 and 382 participants, with 1032 partici- outcomes were quality of life, self-care/self-management pants and intervention duration ranging from 28 days to behaviours, HF knowledge, number of hospital admis- 12 months [15–23]. Table 1 provides a summary of study sions, mortality, health status, self-efficacy, depression, characteristics. clinical follow-up attendance, patient satisfaction, physi- ological measures (e.g., NT-proBNP and HbA1C), and Technology characteristics physical activity. A range of technologies was used in the included studies: Remote medication monitoring systems, telemonitor- Quality assessment ing devices, electronic pill boxes, apps/text-messaging, Quality assessment was conducted using the Cochrane electronic pill organiser reminders, and web-based tech- Collaboration’s Risk of Bias Tool to assess each study’s nologies [15–23]. Six studies used their technology inter- risk of bias according to the following seven domains: ventions as a form of patient education to increase MA random sequence generation, allocation concealment, [16, 18–21, 23]. The other three studies used medication blinding of participants and personnel, blinding of out- reminders via the technologies to improve MA [15, 17, come assessment, incomplete outcome data, selective 22]. reporting bias, and other bias. Each study’s domains Three studies used single interventions (their respec- were allocated to low-risk, unclear-risk, or high-risk of tive technology devices). Still, participants were bias. The risk of bias summary graphs were gener- contacted by telephone if they were identified as non- ated using RevMan software version 5.4. adherent to their medication [15, 16] or classed as high- risk based on their adherence data or answers about Results symptoms, HF knowledge, and medication-taking behav- Search results iours. One study used medication event monitoring The initial search of databases identified 1157 records. system (MEMS) feedback in addition to a counselling No additional studies were identified from the reference intervention known as the ’Theory of Planned Behaviour lists of included studies. Once duplicates were removed, (TPB)’. Another study used a home telemonitor- and RCT filters were applied, 166 studies were screened ing system entailing an app with multiple functionalities using titles and abstracts. After exclusion, 19 studies were such as physiological measure monitoring and educa- screened using full-text, and ten studies were excluded tion. One study used various interventions such as Cheng et al. Journal of Pharmaceutical Policy and Practice (2023) 16:81 Page 4 of 14 Fig. 1 Preferred Reporting Items for Systematic Reviews and Meta-Analyses (PRISMA) flow chart of the study selection process face-to-face self-management sessions, telephone rein- electronic tool to provide educational materials on medi- forcement sessions, and a self-management toolkit (with cations. devices such as a weight scale and an electronic pill organiser reminder). Web-based technology in one Assessment of medication adherence study created a Web-interface for participants with three MA was measured in various ways across the studies. components: patient’s medical record, education guide, Six studies solely used self-reporting tools or question- and messaging system with practice nurses. Gold- naires [15, 18, 20–23]. Two studies used the modified stein et al. used a 2 × 2 study design, where a telehealth Morisky scale or questions derived from it [21, 23]. intervention versus a control group took place alongside Other self-reporting measures included an adherence a mobile health intervention against a control group. questionnaire from Voils et al. , the ’Heart Failure The mobile health intervention in Felker et al. involved Compliance Scale’ , a self-reporting question from two aspects: personalised text messages to summarise the Medical Outcomes Study (MOS) and a self- physical activity performance and goal setting and an reporting question of the day’s medication was missed Table 1 Study characteristics for the included studies Study Study design Sample size Intervention Control group Intervention arms Length of Primary outcomes Secondary intervention outcomes Hale et al. , USA Randomized con‑ Intervention = 13 MedSentry remote Usual care: using 2 arms (control ver‑ 90 days Medication adher‑ Not applicable trolled pilot study Control = 16 medication moni‑ their usual medica‑ sus intervention) ence (n = 29) toring system- med‑ tion reminder Health status ication contained in method Quality of life individual bins with Emergency participants alerted department (ED) when it is time to visits and hospitali‑ take it zations Gallagher et al. , Randomized con‑ Intervention = 20 Telemonitoring Usual care with pas‑ 2 arms (control ver‑ 30 days Adherence to loop 30-day all cause USA trolled pilot study Control = 20 (in combination sive monitoring sus intervention) diuretics readmission (n = 40) with the GlowCap Attendance at system- patients follow-up told they may be Acceptability of Cheng et al. Journal of Pharmaceutical Policy and Practice contacted for moni‑ telemonitoring toring in interven‑ tion group Goldstein et al. , Randomized Smartphone Telehealth interven‑ Usual care with 2 × 2 arms (two 28 days Medication adher‑ Not applicable USA controlled feasibility (n = 30) tion (electronic pill intervention device control versus ence trial Intervention = 15 box) and m-health that did not provide intervention) Mastery of inter‑ (2023) 16:81 Control = 15 intervention (app reminders—"silent 1.Pillbox silent vention (partici‑ Container (n = 30) on smartphone) condition" 2.Pillbox reminding pants ability to use Intervention = 15 3.Smartphone silent their given device Control = 15 4.Smartphone and its functions) (n = 60) reminding Device ratings Boyne et al. , Randomised con‑ Intervention = 197 Telemonitoring Usual care with easy 2 arms (control ver‑ 12 months Disease-specific Not applicable Netherlands trolled trial Control = 185 device (Health‑ access to a HF nurse sus intervention) knowledge (n = 382) Buddy)- connected and 4 outpatient Disease-specific to a landline. Par‑ clinic visits during self-care ticipants answered follow-up Disease-specific daily questions self-efficacy about symptoms, Disease-specific knowledge, and adherence to behaviour by therapy touching one of the device keys Wu et al. , USA Randomised con‑ TPB + MEMS Theory of Planned Usual care 3 arms 9 months Cardiac event-free Not applicable trolled trial group = 27 Behaviour (TPB) 1.TPB and MEMS survival TPB = 27 AND Medication (PLUS) Quality of life Control = 28 Event Monitoring 2.TPB (LITE) Medication adher‑ (n = 82) System (MEMS) 3. Usual care ence feedback (Control) Page 5 of 14 Table 1 (continued) Study Study design Sample size Intervention Control group Intervention arms Length of Primary outcomes Secondary intervention outcomes Felker et al. , Randomised Con‑ Intervention = 92 Mobile Health Usual care 2 arms (intervention 3 months Change in mean Change in medica‑ USA trolled Trial Control = 95 (mHealth) interven‑ versus control) (6 months follow- daily step count tion adherence (n = 187) tion- educational up as well) from baseline Quality of life skill-based teaching through 3 months Physiological meas‑ for medication ure of disease status management (NT-proNBP, HbA1c) Metabolic profiling Yanicelli et al. , Randomised Con‑ Intervention = 20 Home telemonitor‑ Usual care 2 arms (intervention 90 days Changes in self- Rehospitalisation Argentina trolled Trial Control = 20 ing system (HTS)- versus control) care (n = 40) application (app) Changes in treat‑ that collects physi‑ ment adherence Cheng et al. Journal of Pharmaceutical Policy and Practice ological measures and symptoms, with an educational and messaging function Young et al. , Randomised Con‑ Intervention = 54 Electronic pill Usual care with 2 arms (intervention 12-week SM train‑ SM adherence: Physical activity USA trolled Trial organizer reminder standard dis‑ versus control) ing Frequencies of (2023) 16:81 Control = 51 (n = 105) charge teaching 3 + 6-month follow- daily weighing and scheduled up Following low- follow-up doctor’s sodium diet appointment Taking prescribed medication Exercising Attending follow- up appointments Clinical biomarkers All-cause readmis‑ sions ED visits Ross et al. , USA Randomised con‑ Intervention SPPARO (System Usual care 2 arms (control ver‑ 12 months Self-efficacy Patient satisfaction trolled trial group = 54 Providing Access to sus intervention) Adherence (medica‑ Control group = 53 Records Online): tion and general) (n = 107) Web-based electronic medical record Web-based educa‑ tional guide Web-based mes‑ saging system Page 6 of 14 Cheng et al. Journal of Pharmaceutical Policy and Practice (2023) 16:81 Page 7 of 14 in the last seven days. Two studies exclusively used the mobile health groups used a self-reported meas- a dose count from the technological intervention device ure. The study that used a question from MOS as their measure outcome [16, 19]. One study used two also used a dose count from the intervention device, outcome measures depending on the intervention- the but only for the intervention group. The outcome telehealth groups used a dose count percentage, whilst measures for each study are shown in Table 2. Table 2 The effectiveness of technology on medication adherence Study and Country Method of measuring medication adherence Effect on medication adherence P-value Hale et al. , USA One question from Medical Outcomes Study (MOS) At 90 days follow-up, Intervention group medica‑ 0.610 MedSentry data- counted as ’missed if not taken tion adherence was 69% (n = 9) versus 73% (n = 13) within 1 h measured using a MOS question Medication adherence in the intervention group did not improve from baseline of 98.7% versus 94.2% at 90 days follow-up using data from the MedSentry device Gallagher et al. , USA Percentage of days where number of correct doses Adherence to diuretics (> 88% doses taken) was 0.640 were taken 29.4% (n = 5 participants) in the intervention group at (Mann–Whitney U test used to compare adherence 30 days follow-up, compared to 36.8% (n = 7) in the percentages between groups) control group Goldstein et al. , USA Pillbox opening recording At 28 days follow-up, intention-to-treat analyses 0.480 Smartphone- electronic self-report (patients recorded showed patients in both intervention groups adhered their medication-taking on a log available on the to their medications 79% of the time, a mean of 84% medication adherence app – options for each event for pillbox and 73% for smartphone intervention. This were taking a medication or skipping it) is compared to a mean of 78% adherence in the pas‑ sive medication device groups (from 76% in pillbox and 79% in smartphone) Per protocol analysis found that the intervention groups had a mean adherence rate of 85% versus a mean of 80% in the control groups Boyne et al. , Netherlands Heart Failure Compliance Scale Perceived importance of medication increased after 0.107 (Higher scores indicate better adherence) 6 (93.5% intervention versus 88% control, p = 0.012) and 12 months (93.5% intervention versus 89.8% control, p = 0.037) Estimated medication adherence based on X was greater in the intervention at 12 months (100% intervention versus 98.7% control) but was not found to be statistically significant) Wu et al. , USA MEMS (> 88% recorded opening as sched‑ PLUS patients had significantly better medication 0.021 uled = adherent; adherence versus control group at 2 months follow- < 88% = non = adherent) up (82% intervention versus 59% control, p = 0.05) and at 9 months follow-up (74% intervention versus 36% control, p = 0.012) Felker et al. , USA Adherence questionnaire developed by Voils et al Medication adherence did not change from baseline 0.470 to 3 months (Least Squares (LS)-mean change: –0.08 in mHealth vs –0.15 in usual care; LS-mean differ‑ ence = 0.07; 95% CI: –0.12, 0.26) Yanicelli et al. , Argentina Morisky Modified Scale (MMS) questionnaire Mean MMS overall scores in 3 months follow-up were 0.800 (Higher scores indicated better adherence) 4.73 (intervention) and 4.73 (control) Young et al. , USA Self-reported number of days where medication had Intervention group also reported significantly fewer 0.030 been missed in the previous 7 days days missing any doses of prescribed medication Mean number of days for any missed medication in the previous 7 days was 0.39 in the intervention group versus 0.81 (control) at 3 months and 0.26 (intervention) versus 0.80 (control) at 6 months. Esti‑ mated marginal mean was 0.30 (intervention) versus 0.80 (control). 95% CI: -0.51 (-0.97, -0.05) Ross et al. , USA Questions derived from Morisky Scale At 6 months, medication adherence scores were 3.5 0.150 (Intervention) versus 3.4 (control), with a CI of + 0.1 (-0.2, 0.4). At 12 months, scores were 3.6 (intervention) versus 3.4 (control) with a CI of + 0.2 (-0.1, 0.6) Cheng et al. Journal of Pharmaceutical Policy and Practice (2023) 16:81 Page 8 of 14 Effect of medication adherence Self‑care management Eight of the nine studies showed improvement in MA Four studies showed significant improvement in self- following the intervention [15–19, 21–23] (Table 2). The care/self-management or general adherence to self-care remaining study is unclear as they did not report the [18, 21–23] (Table 5). direction of their scoring system for medication adher- ence. However, only two studies showed a statisti- Patient satisfaction with the intervention cally significant improvement in MA. The study by Wu Two studies evaluated device rating/patient satisfac- et al. used MEMS feedback as an educational tool for the tion as an outcome towards the intervention- one study patient. It showed 74% of the intervention group adher- observed that patients statistically significantly preferred ent to medication versus 36% in the control group. m-health over telehealth. The other resulted in 88% The study by Young et al. used an electronic pill organ- of participants rating the device as somewhat or very easy iser reminder as a medication reminder intervention and to use. Ross et al. measured patient satisfaction with found improvement with a marginal mean of 0.8 days if doctor patient-communication, with improvements seen any medication was missed in the last seven days for the in some domains, but which were not significant. control group versus 0.3 days in the intervention group. Other outcomes One study assessed depression and did not show Effect on clinical and non‑clinical outcomes improvement. Follow-up attendance did not improve A table of other clinical outcomes examined in included in another study. Boyne et al. showed statistically studies can be found in Additional file 1: Table S2. These significant improvement in disease-specific knowledge at included: quality of life, self-care/self-management the end of follow-up. Three studies assessed self-effi- behaviours, HF knowledge, number of hospital admis- cacy, two of which did not improve [18, 23], while one did sions, mortality, health status, self-efficacy, depression,. Two trials measured physical activity- one showed clinical follow-up attendance, patient satisfaction, physi- significant improvement in mean daily step count , ological measures, and physical activity. whilst the other did not in activity minutes, calories burnt, or daily activity counts. Quality of life Lastly, two studies evaluated physiological parame- Table 3 shows only one of the three studies that assessed ters such as HbA1c and NT-proBNP with no significant the quality of life showed significant improvement in differences shown [20, 22]. One of those studies also quality of life. Another study showed significance, evaluated metabolic profiling with significant changes but the quality of life was worse in the intervention group observed for the intervention in 13 metabolites. than in control. A-value was not available in the pre- vious research. Risk of bias of included trials The risk of bias summary graphs were generated using Hospitalisation RevMan 5.4 software (Figs. 2, 3). Six studies reported Hospitalisations showed variations in the results, with appropriate random sequence generation [16–18, 21–23], two of five studies showing a significant difference in the with the other three showing unclear risk [15, 19, 20]. In number of hospital admissions [15, 19] (Table 4). contrast, six studies showed unclear allocation conceal- ment [15, 18–21, 23], two with low risk [16, 22], and one Health‑status reporting a high risk of bias. Blinding of participants One study evaluated health status and did not show sig- and personnel showed a high risk of bias for all studies as nificance. it wouldn’t have been possible to blind participants, due Table 3 Quality of life outcome measures and results Study Measurement of quality of life Results P-value(s) Hale et al. , USA Minnesota Living with Heart Mean score for quality of life at 90 days follow-up for interven‑ 0.002 Failure Questionnaire tion group (PLUS) was 62.2 for intervention group versus 28.2 in (Higher score indicates worser quality of life) control group Wu et al. , USA Minnesota Living with Heart Failure Questionnaire Mean score for quality of life at 90 days follow-up for interven‑ 0.464 (Higher score indicates worser quality of life) tion group (PLUS) was 32.9 for intervention group versus 40.1 in control group Felker et al. , USA Kansas City Cardiomyopathy Questionnaire Significant difference in quality of life (difference 1.1 with 95% 0.009 CI [1.4, 9.6]). Higher in intervention Cheng et al. Journal of Pharmaceutical Policy and Practice (2023) 16:81 Page 9 of 14 Table 4 Hospitalisation outcome measures and results Study Clinical outcomes Results P-value(s) Hale et al. , USA Hospitalisations Number of participants who had one or more 0.340 (HF), 0.340 ED visits HF-related hospitalisations were 4 in inter‑ (non-HF), 0.040 vention group versus 1 in control group and (all-cause) non-HF related hospitalisations were 1 in inter‑ 0.600 (HF), 0.990 vention group versus 4 in control group after (non-HF), 0.680 90 days. All-cause hospital admissions were (all-cause) significant, with 1 in intervention group versus 4 in control group Number of participants who had one or more HF-related ED visits were 1 in intervention group versus 3 in control group and non-HF related ED visits were 3 in intervention group versus 4 in control group after 90 days. All-cause ED visits were not significant, with 3 in the inter‑ vention group versus 6 in the control group Gallagher et al. , USA 30-day all cause readmission Number of readmissions were 6 in the interven‑ 0.720 tion group and 4 in the control group after 30 days follow-up Wu et al. , USA Cardiac event-free survival Event-free survival was significantly longer for 0.010 (Including ED visit, hospitalization, death) the patients in both intervention groups than the control Yanicelli et al. , Argentina Rehospitalisation (from electronic medical Number of rehospitalisation were 0 for inter‑ 0.500 record) vention group and 2 in control group after 3 months follow-up Young et al. , USA All-cause readmissions Number of participants readmitted to hospital 0.088 ED visits was 10 for intervention group versus 3 in the control group Number of participants admitted for ED visits at 90 days were 9 for intervention group versus 11 in control group Bolded p-values represent the significance values used as part of the synthesis. For fairness of comparison, all-cause hospitalisation was used in Hale et al, to encompass all hospitalisations regardless of their relation to heart failure to the type of intervention involved. However, blinding Discussion personnel could have been possible, with only one of the This systematic review aimed to determine technology’s studies mentioning that the study team members were impact on MA in HF patients. This review involved nine blinded to group assignments. Six studies reported RCTs with a total of 1032 participants and used some an unclear risk of bias for blinding of outcome assess- form of technology to support MA and evaluate this as ment [15, 17, 18, 20, 21, 23], with the other three showing an outcome [15–23]. Two RCTs showed statistically sig- low risk [16, 19, 22]. Assessment of incomplete outcome nificant improvement in MA, both of which used mixed data showed six studies with a low risk [15–18, 21, 22], interventions [19, 22]. Six RCTs showed improvement, two with unclear risk [19, 20], and one study being a high but results were not statistically significant [15–18, 21, risk for attrition bias. Selective reporting assessment 23], and one RCT showed unclear improvement. displayed seven studies with a low risk of bias [15, 16, 18, Depending on the study, the technologies provided differ- 20–23] and two studies for unclear risk [17, 19]. Lastly, ent purposes- patient education or medication reminders seven studies exhibited a low risk of other bias [15–17, (with no known comparisons on which is more effective 19–21, 23], specifically on whether the tools they used as in MA). To our knowledge, no other reviews assess tech- outcome measurements for MA were reported as being nology for MA, specifically in HF. validated, known to be validated, or showed a quantita- It is worth noting that the two RCTs that showed sig- tive measure of adherence. The other two studies did not nificant improvement did not use technology in iso- show this, therefore, were classed as high risk for bias [18, lation [19, 22]. They were used in conjunction with 22]. non-technology components, for example, synchronous communication with healthcare professionals (HCPs), remote logging of symptoms, and behavioural change therapy (TPB) [19, 22]. Multi-disciplinary involvement Cheng et al. Journal of Pharmaceutical Policy and Practice (2023) 16:81 Page 10 of 14 Table 5 Self-care/general adherence outcome measures and results Study Clinical outcome and measurement Results P-value(s) Boyne et al. , Netherlands European Heart Failure Self-Care Behaviour Scale Mean self-care score at 12 months follow-up was < 0.001 (EHFScB) 17.4 for intervention group versus 20.8 in control (uncorrected) (Lower scores indicate better results) group < 0.001 at T = 12 (cor- rected) Yanicelli et al. , Argentina European Heart Failure Self-Care Behaviour Scale Mean self-care score at 3 months follow-up was 0.004 (EHFScB) 80.03 for intervention group versus 69.43 in (Higher scores indicate better results) control group Young et al. , USA Other self-management adherence Mean number of days for weighing self per week < 0.005 was 4.8 in the intervention group versus 1.9 (con‑ < 0.005 trol) at 3 months and 4.6 (intervention) versus 1.5 < 0.005 (control) at 6 months. Estimated marginal mean was 4.7 (intervention) versus 1.7 (control). 95% CI: 2.98 (2.10, 3.86) Mean number of days for following low-sodium diet per week was 5.6 in the intervention group versus 3.1 (control) at 3 months and 5.1 (interven‑ tion) versus 2.3 (control) at 6 months. Estimated marginal mean was 5.3 (intervention) versus 2.7 (control). 95% CI: 2.62 (1.74, 3.50) Mean number of days for exercising per week was 5.4 in the intervention group versus 3.4 (control) at 3 months and 4.5 (intervention) versus 3.1 (control) at 6 months. Estimated marginal mean was 4.9 (intervention) versus 3.3 (control). 95% CI: 1.66 [0.79, 2.53] Ross et al. , USA General Adherence Scale from the Medical Out‑ Mean score for general adherence was 85 in the 0.020 comes Study (MOS) intervention group versus 78 in control group. (Higher scores indicate better adherence) 95% CI + 6.4 (1.8, 10.9) Bolded P-values represent the significance values used as part of the synthesis. For fairness of comparison, corrected values (where applicable to the study) were used as opposed to uncorrected values was common in most studies [16, 18, 19, 21–23]. Previ- cost-effective approach to predict clinical outcomes, ous evidence shows that combined approaches are more they have the potential to produce the ’Hawthorne effect’ likely to improve MA in HF patients, specifically in older whereby certain behaviours evaluated in studies differ adults. This supports the notion that technology if between participants due to their awareness of being used in practice, should be part of a multi-disciplinary assessed [26, 27]. This leads to overestimations of proper approach to the care of an HF patient. adherence [26, 27]. One study in our review exemplified Where similar improvements have been seen in past this, which attributed overestimations to ’socially accept- reviews for specific technologies in CVD, most have able answers’ , and two others note self-reporting attributed a lack of significant improvement to small use in various outcomes as a limitation [15, 17]. MEMS/ sample sizes [12, 25]. Our findings also showed two pilot digital monitoring- used in three studies [16, 17, 19], is studies [15, 16], one feasibility study , and four stud- considered a more accurate way of measuring MA. ies that had a relatively small sample size [19, 21–23]. However, it does not guarantee the correct dose is taken. These studies noted they were not sufficiently powered Hence, results should be interpreted with caution. to test the intervention’s actual effect. In addition, lim- The study population of some studies may have con- ited research assesses MA technology use in HF. This was tributed to potential ceiling effects observed in this observed in mobile health-specific reviews by Coorey review. For instance, one study reported a baseline adher- et al. and Al-Arkee et al., both only included one RCT ence of 98% , leaving little room for the interven- relating specifically to HF and commented on small sam- tion to produce a significant effect. Five of the studies ple sizes as a limitation [12, 25]. reported using the majority of already adherent/moti- MA was assessed mainly using self-reporting tools or vated patients [16–19, 22], where it was unclear if this questionnaires in seven studies [15, 17, 18, 20–23]. Some was intentional (except Goldstein et al. which attributed tools, however, were not validated or widely known this to their recruitment methods). Therefore, this largely [18, 20, 22]. Whilst self-reporting methods provide a excluded the non-adherent population- the ideal targets Cheng et al. Journal of Pharmaceutical Policy and Practice (2023) 16:81 Page 11 of 14 of these interventions. Participant recruitment methods including advertisements and mailing, may have auto- matically pooled these patient cohorts. Previous literature suggests that a willingness to participate in tri- als through such methods voluntarily can be indicative of the motivation and education the patient already has. Therefore, the selection of these patients is more likely to result in ceiling effects. The opposite has been suggested for non-adherent patients, where significant differences are more likely to be seen. Despite all trials assessing a range of other clini- cal outcomes, there was much variation in the results, especially for the quality of life and hospital admissions. These findings are consistent with a review from Allida et al., which evaluated the use of mobile health in HF patients- limited evidence was found for the effective- ness of technology interventions on quality of life and hospital admission. Variations for these two out- comes (shown in Tables 3 and 4) could be explained by the heterogeneity of the measurement tools used. Our self-care/management and general adherence results differed from Allida et al., where all trials that assessed it showed statistical significance, mainly using validated or well-known tools [18, 21–23]. This demonstrates these technologies’ potential to impact remote non-pharmaco- logical care in HF positively. Another review on the use of smartphones in healthcare applications raised the impor- tance of technology in providing education, self-man- agement, and remote monitoring. This links to the multi-intervention aspects of most studies in our review that may have contributed to improvements in self-care/ management and general adherence. Three out of four Fig. 2 Risk of bias summary graph and the outcome of each domain trials involving self-care used educational-based tech- for included studies nologies [18, 21, 23], and two of those studies monitored Fig. 3 Risk of bias graph of included studies with percentages for each risk of bias domain Cheng et al. Journal of Pharmaceutical Policy and Practice (2023) 16:81 Page 12 of 14 patient symptoms via the technologies [21, 22]. There- Recommendations for policy and practice fore, these technologies must have multiple functions to Technology can potentially improve MA in HF patients; improve MA and non-pharmacological behaviours. however, our review has shown insufficient evidence for Only four RCTs assessed patient satisfaction with the this. The RCTs included in this review showed uncer- intervention technology [15–17, 21]; however, all of them tain and inconsistent results for the quality of studies showed most participants finding their technologies easy and effectiveness of the technologies. On the other hand, to use or helpful for their treatment plans. These results these technologies were generally easy to use or help- are useful for informing technology utilisation in a clini- ful for patients. Therefore, recommendations for clini- cal setting, specifically in a disease population with an cal practice cannot be made without solid evidence from older age demographic (all studies showed participants good-quality studies. over the age of 50), and where previous research has sug- gested links between HF patients and susceptibility to Future research cognitive impairment. It is worth noting all studies Our findings from this review indicate the need for did not explicitly state the degree of cognitive impair- further research. Future RCTs should be sufficiently ment in their patient characteristics but stated the need powered, primarily targeting non-adherent patients, for their technologies to be appropriate for them. and use blinded outcome assessors. Using validated Our findings accord with a cardiac tele-rehabilitation self-reporting tools, in addition to electronic monitor- review which showed the technologies’ high usability, ing, should be used together to increase the accuracy utility, and acceptability, especially in the COVID-19 cli- of results. Future studies should continue to assess mate. The review also noted potential preferences usability and patient satisfaction with technologies for types of technologies , but they still appeared and explore the most effective mechanisms for sup- usable and satisfactory to patients. A study in our review porting MA, such as whether providing education or observed the preference for mobile health over telehealth medication reminders are more effective. Using simi- due to the easy integration of the mobile app into their lar validated self-report tools will allow future system- daily routines. This indicates that patients may prefer atic reviews to include meta-analyses to generate more technologies that are easy to use and are already familiar. robust conclusions on intervention effectiveness. A previous review on mobile health notes that portability and multi-feature access (such as viewing patient records and monitoring) is helpful for patients and their health- Conclusion care providers. Additionally, tailored approaches Evidence for the effectiveness of technology in medi- based on the individual’s needs and behaviours should be cation adherence is currently weak. Whilst it has considered , particularly motivation- a known con- indicated positive improvements for some outcomes, tributor to adherence , which was not addressed in particularly self-care, further evidence is needed for most studies. its impact on MA. More powered trials that include larger sample sizes and mostly non-adherent cohorts Strengths and limitations are required to build on existing studies and inform the This systematic review was conducted in accordance with future incorporation of technology into routine clinical PRISMA guidelines to reduce researcher bias. However, practice. it was often difficult to ascertain if any improvements in MA and other outcomes were solely due to the technol- Abbreviations ogy or the other non-technology intervention compo- HF Heart failure nents. Other studies that may have been relevant were HFrEF Heart failure with reduced ejection fraction excluded, such as grey literature, non-RCTs, and studies HFpEF or HFmrEF Heart failure with preserved or mildly reduced Ejec‑ tion Fraction not in English. However, we used RCTs, which are well- ARBs Angiotensin Receptor Blockers known to place at the highest level of the hierarchy of MA Medication adherence evidence. Some studies tested the intervention on app Mobile health and application NYHA New York Heart Association a certain number of medications; for e.g., Wu et al. pro- RCTs Randomised Control Trials vided MEMs feedback for only one of the medications MEMS Medication event monitoring system. HF patients take multiple medications; therefore, HCPs Healthcare professionals total adherence may have differed. Cheng et al. Journal of Pharmaceutical Policy and Practice (2023) 16:81 Page 13 of 14 Supplementary Information 7. Rasmussen AA, Wiggers H, Jensen M, Berg SK, Rasmussen TB, Borregaard B, et al. Patient-reported outcomes and medication adherence in patients The online version contains supplementary material available at https://​doi.​ with heart failure. Eur Heart J Cardiovasc Pharmacother. 2021;7:287–95. org/​10.​1186/​s40545-​023-​00582-9. 8. Ruppar TM, Cooper PS, Mehr DR, Delgado JM, Dunbar-Jacob JM. Medica‑ tion adherence interventions improve heart failure mortality and read‑ Additional file 1: Table S1. Example search strategy- EMBASE. Table S2. mission rates: systematic review and meta-analysis of controlled trials. J Other clinical outcomes and their effects. Am Heart Assoc. 2016;5: e002606. 9. Wu J-R, Moser DK. Medication adherence mediates the relationship between heart failure symptoms and cardiac event-free survival in Acknowledgements patients with heart failure. J Cardiovasc Nurs. 2018;33:40–6. Not applicable. 10. Smaje A, Weston-Clark M, Raj R, Orlu M, Davis D, Rawle M. Factors associ‑ ated with medication adherence in older patients: a systematic review. Author contributions Aging Medicine. 2018;1:254–66. Study conception and design CC, GD, ZJ, NA; database searches CC, GD, ZJ; 11. Simon ST, Kini V, Levy AE, Ho PM. Medication adherence in cardiovascular study selection and data extraction CC, GD, ZJ.; analysis, CC, GD, ZJ.; manu‑ medicine. BMJ. 2021;374:n1493. script drafting CC, GD, ZJ, NA; manuscript revision and editing CC, GD, ZJ,NA. 12. Al-Arkee S, Mason J, Lane DA, Fabritz L, Chua W, Haque MS, et al. Mobile All authors have read and agreed to the published version of the manuscript. apps to improve medication adherence in cardiovascular disease: sys‑ tematic review and meta-analysis. J Med Internet Res. 2021;23: e24190. Funding 13. Allida S, Du H, Xu X, Prichard R, Chang S, Hickman LD, et al. mHealth edu‑ Not applicable. cation interventions in heart failure. Cochrane Database Syst Rev. 2020. https://​doi.​org/​10.​1002/​14651​858.​CD011​845.​pub2. Availability of data and materials 14. Cochrane. 8.5 The Cochrane Collaboration’s tool for assessing risk of bias. Further supplementary material is available on request. 2022. https://​handb​ook-5-​1.​cochr​ane.​org/​chapt​er_8/​8_5_​the_​cochr​ane_​ colla​borat​ions_​tool_​for_​asses​sing_​risk_​of_​bias.​htm. Accessed 21 Dec 2022. Declarations 15. Hale TM, Jethwani K, Kandola MS, Saldana F, Kvedar JC. A remote medica‑ tion monitoring system for chronic heart failure patients to reduce read‑ Ethics approval and consent to participate missions: a two-arm randomized pilot study. J Med Internet Res. 2016;18: Not applicable. e91. 16. Gallagher BD, Moise N, Haerizadeh M, Ye S, Medina V, Kronish IM. Telem‑ Consent for publication onitoring adherence to medications in heart failure patients (TEAM-HF): a Not applicable. pilot randomized clinical trial. J Card Fail. 2017;23:345–9. 17. Goldstein CM, Gathright EC, Dolansky MA, Gunstad J, Sterns A, Redle JD, Competing interests et al. Randomized controlled feasibility trial of two telemedicine medica‑ The authors have no competing interests to declare. tion reminder systems for older adults with heart failure. J Telemed Telecare. 2014;20:293–9. Author details 1 18. Boyne JJ, Vrijhoef HJ, Spreeuwenberg M, De Weerd G, Kragten J, Gorgels School of Pharmacy, Institute of Clinical Sciences, University of Birmingham, AP. Effects of tailored telemonitoring on heart failure patients’ knowledge, Edgbaston, Birmingham B15 2TT, UK. 2 Generated Health Ltd, Mercury House, self-care, self-efficacy and adherence: a randomized controlled trial. Eur J 117 Waterloo Road, London SE1 8UL, England. 3 School of Computing, The Cardiovasc Nurs. 2014;13:243–52. University of Buckingham, Hunter Street, Buckingham MK18 1EG, UK. 19. Wu J-R, Corley DJ, Lennie TA, Moser DK. Effect of a medication-taking behavior feedback theory-based intervention on outcomes in patients Received: 27 April 2023 Accepted: 7 June 2023 with heart failure. J Card Fail. 2012;18:1–9. 20. Felker GM, Sharma A, Mentz RJ, She L, Green CL, Granger BB, et al. A randomized controlled trial of mobile health intervention in patients with heart failure and diabetes. J Card Fail. 2022;28:1575–83. 21. Yanicelli LM, Goy CB, del González VC, Palacios GN, MartÃnez EC, Herrera References MC. Non-invasive home telemonitoring system for heart failure patients: 1. British heart foundation. Fact and figures, information for journalist-BHF. a randomized clinical trial. J Telemed Telecare. 2021;27:553–61. 2022. https://​www.​bhf.​org.​uk/​what-​we-​do/​news-​from-​the-​bhf/​conta​ct-​ 22. Young L, Hertzog M, Barnason S. Effects of a home-based activation inter‑ the-​press-​office/​facts-​and-​figur​es. Accessed 18 Dec 2022. vention on self-management adherence and readmission in rural heart 2. National institute for health and care excellence. Prevalence, background failure patients: the PATCH randomized controlled trial. BMC Cardiovasc information, Heart failure–chronic, CKS, NICE. 2022. https://​cks.​nice.​org.​ Disord. 2016;16:176. uk/​topics/​heart-​failu​re-​chron​ic/​backg​round-​infor​mation/​preva​lence/. 23. Ross SE, Moore LA, Earnest MA, Wittevrongel L, Lin C-T. Providing a web- Accessed 18 Dec 2022. based online medical record with electronic communication capabilities 3. National institute for health and care excellence. Prognosis, background to patients with congestive heart failure: randomized trial. J Med Internet information, heart failure–chronic, CKS, NICE. 2022. https://​cks.​nice.​org.​ Res. 2004;6: e12. uk/​topics/​heart-​failu​re-​chron​ic/​backg​round-​infor​mation/​progn​osis/. 24. Andrews AM, Russell CL, Cheng A-L. Medication adherence interventions Accessed 18 Dec 2022. for older adults with heart failure: a systematic review. J Gerontol Nurs. 4. National institute for health and care excellence. Management, Heart 2017;43:37–45. failure–chronic, CKS, NICE. 2022. https://​cks.​nice.​org.​uk/​topics/​heart-​failu​ 25. Coorey GM, Neubeck L, Mulley J, Redfern J. Effectiveness, acceptability re-​chron​ic/​manag​ement/. Accessed 18 Dec 2022. and usefulness of mobile applications for cardiovascular disease self- 5. National Institute for Health and Care Excellence. Introduction, medicines management: systematic review with meta-synthesis of quantitative and adherence: medicines adherence: involving patients in decisions about qualitative data. Eur J Prev Cardiol. 2018;25:505–21. prescribed medicines and supporting adherence. 2009. https://​www.​ 26. Stirratt MJ, Dunbar-Jacob J, Crane HM, Simoni JM, Czajkowski S, Hilliard nice.​org.​uk/​guida​nce/​cg76/​chapt​er/​intro​ducti​on. Accessed 18 Dec 2022. ME, et al. Self-report measures of medication adherence behavior: recom‑ 6. Shah D, Simms K, Barksdale D, Wu J-R. Improving medication adherence mendations on optimal use. Transl Behav Med. 2015;5:470–82. of patients with chronic heart failure: challenges and solutions. Res Rep 27. McCambridge J, Witton J, Elbourne DR. Systematic review of the Haw‑ Clin Cardiol. 2015;6:87. thorne effect: new concepts are needed to study research participation effects. J Clin Epidemiol. 2014;67:267–77. Cheng et al. Journal of Pharmaceutical Policy and Practice (2023) 16:81 Page 14 of 14 28. Wu J-R, Moser DK, Chung ML, Lennie TA. Objectively measured, but not self-reported, medication adherence independently predicts event-free survival in patients with heart failure. J Card Fail. 2008;14:203–10. 29. van Onzenoort HAW, Menger FE, Neef C, Verberk WJ, Kroon AA, de Leeuw PW, et al. Participation in a clinical trial enhances adherence and persis‑ tence to treatment. Hypertension. 2011;58:573–8. 30. Allemann SS, Nieuwlaat R, Navarro T, Haynes B, Hersberger KE, Arnet I. Congruence between patient characteristics and interventions may partly explain medication adherence intervention effectiveness: an analysis of 190 randomized controlled trials from a Cochrane systematic review. J Clin Epidemiol. 2017;91:70–9. 31. Mosa ASM, Yoo I, Sheets L. A systematic review of healthcare applications for smartphones. BMC Med Inform Decis Mak. 2012;12:67. 32. Cannon JA, Moffitt P, Perez-Moreno AC, Walters MR, Broomfield NM, McMurray JJV, et al. Cognitive impairment and heart failure: systematic review and meta-analysis. J Card Fail. 2017;23:464–75. 33. Ramachandran HJ, Jiang Y, Teo JYC, Yeo TJ, Wang W. Technology accept‑ ance of home-based cardiac telerehabilitation programs in patients with coronary heart disease: systematic scoping review. J Med Internet Res. 2022;24: e34657. 34. Hamine S, Gerth-Guyette E, Faulx D, Green BB, Ginsburg AS. Impact of mHealth chronic disease management on treatment adherence and patient outcomes: a systematic review. J Med Internet Res. 2015;17: e52. 35. George J, Elliott RA, Stewart DC. A systematic review of interventions to improve medication taking in elderly patients prescribed multiple medications. Drugs Aging. 2008;25:307–24. 36. Aubeeluck E, Al-Arkee S, Finlay K, Jalal Z. The impact of pharmacy care and motivational interviewing on improving medication adherence in patients with cardiovascular diseases: a systematic review of randomised controlled trials. Int J Clin Pract. 2021;75: e14457. 37. Burns PB, Rohrich RJ, Chung KC. The levels of evidence and their role in evidence-based medicine. Plast Reconstr Surg. 2011;128:305–10. Publisher’s Note Springer Nature remains neutral with regard to jurisdictional claims in pub‑ lished maps and institutional affiliations. Ready to submit your research ? Choose BMC and benefit from: fast, convenient online submission thorough peer review by experienced researchers in your field rapid publication on acceptance support for research data, including large and complex data types gold Open Access which fosters wider collaboration and increased citations maximum visibility for your research: over 100M website views per year At BMC, research is always in progress. Learn more biomedcentral.com/submissions