Ghost in the (Hollywood) Machine: 2020 PDF
Document Details
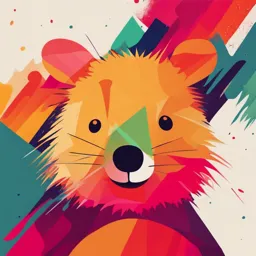
Uploaded by ChivalrousButtercup
Universität Würzburg, Technische Universität Chemnitz
2020
Pei-Sze Chow
Tags
Summary
This article explores the nascent use of artificial intelligence in the film industry, specifically during the greenlighting stage of film projects. It examines the ethical, cultural, and industrial implications of AI's increasing role in influencing decisions about film production. The study analyzes case studies, interviews, and media discourse to understand how AI might affect creativity, labor, and audience reception.
Full Transcript
Repositorium für die Medienwissenschaft Pei-Sze Chow Ghost in the (Hollywood) machine: Emergent applications of artificial intelligence in the film industry 2020-07-06 https://doi.org/10.25969/mediarep/14307 Veröffentlichungsversion / published version Zeitschriftenartikel /...
Repositorium für die Medienwissenschaft Pei-Sze Chow Ghost in the (Hollywood) machine: Emergent applications of artificial intelligence in the film industry 2020-07-06 https://doi.org/10.25969/mediarep/14307 Veröffentlichungsversion / published version Zeitschriftenartikel / journal article Empfohlene Zitierung / Suggested Citation: Chow, Pei-Sze: Ghost in the (Hollywood) machine: Emergent applications of artificial intelligence in the film industry. In: NECSUS_European Journal of Media Studies. #intelligence, Jg. 9 (2020-07-06), Nr. 1, S. 193– 214. DOI: https://doi.org/10.25969/mediarep/14307. Erstmalig hier erschienen / Initial publication here: https://necsus-ejms.org/ghost-in-the-hollywood-machine-emergent-applications-of-artificial-intelligence-in-the-film- industry/ Nutzungsbedingungen: Terms of use: Dieser Text wird unter einer Creative Commons - This document is made available under a creative commons - Namensnennung - Nicht kommerziell - Keine Bearbeitungen 4.0/ Attribution - Non Commercial - No Derivatives 4.0/ License. For Lizenz zur Verfügung gestellt. Nähere Auskünfte zu dieser Lizenz more information see: finden Sie hier: https://creativecommons.org/licenses/by-nc-nd/4.0/ https://creativecommons.org/licenses/by-nc-nd/4.0/ EUROPEAN JOURNAL OF MEDIA STUDIES www.necsus-ejms.org Ghost in the (Hollywood) machine: Emergent applications of artificial intelligence in the film industry Pei-Sze Chow NECSUS 9 (1), Spring 2020: 193–214 URL: https://necsus-ejms.org/ghost-in-the-hollywood-machine- emergent-applications-of-artificial-intelligence-in-the-film-indus- try/ Abstract This article examines the nascence of artificial intelligence (AI) appli- cations in the film industry at the greenlighting stage, where decisions are made as to the feasibility and earning potential of film projects. Through a qualitative analysis of company case studies, interviews, and media discourse, I interrogate and tease out the ethical, cultural, and industrial implications emerging from the use of AI in influencing decisions about film production, particularly the ways the use of AI might influence notions of creativity, labour, and reception. The arti- cle sets out possible research agendas for the future to critically engage with this emerging phenomenon. Keywords: box office performance, film production, film studies, in- telligence, machine learning, predictive algorithms, script analysis Introduction While the applications of intelligent technologies in sectors such as retail, transportation, medicine, and surveillance are now demonstrating their po- tential to radically shape and influence social and economic life, the growing role of artificial intelligence (AI) in the creative industries is perhaps less prominent. While in the fields of music, architecture, fashion design, and vis- ual art, intelligent algorithms have made some impact as a ‘co-creative agent in activities that have been human prerogative until now’, cinema – and NECSUS – EUROPEAN JOURNAL OF MEDIA STUDIES particularly the mainstream Hollywood variety – remains, at least on the sur- face, resistant to the notion of machine creativity and the allure of algorithms as artistic co-creators. Film, as a particularly complex multi-stage and multi- modal gesamtkunstwerk, has only so far seen attempts to incorporate AI tech- nologies into parts of the cinematic production process in both small and significant ways, whether through image and graphics creation, object recog- nition during photography, animation, special effects generation, and edit- ing, amongst others. However, it is in the film industry’s business and com- mercial aspects and at the early production stages that AI is now making strides. It was around the period 2018-2019 that a slate of articles started ap- pearing in the trade press, reporting on how Hollywood companies are grad- ually adopting AI tools developed by new technology start-ups to analyse fi- nancial, script, and/or audience data and ultimately influence studios’ com- missioning decisions. At the time of writing this article in early 2020, Warner Bros. had just announced their new deal with the technology company Cine- lytic and this revelation gained traction in major news outlets, prompting much speculation and also criticism about what the impact this machine-hu- man partnership will have on artistic creativity, diversity, and novelty for filmmaking. Broadly put, AI is ‘the study of how to build or programme computers to enable them to do what minds can do’. Machine learning, as a specific sub- field of AI, are computational techniques that can be understood as either scientific models or operational algorithms that learn. That is, within an arti- ficially intelligent system or agent, machine learning enables the agent to perceive and act in an environment: to generalise, gather input, use prior knowledge, form new concepts, amongst other behaviours. That the use of machine learning has moved into completely different disciplinary and institutional domains from its specialised engineering beginnings – what Adrian Mackenzie calls the ‘unruly generalisation’ of machine learning across different settings – has engendered a certain curiosity and anxiety about the way humans and algorithms impact each other. Within the film and television industry, the astronomical rise of the popularity of streaming video on demand (SVOD) platforms, the (propriety) artificially intelligent personalisation algorithms that these platforms use to curate and recom- mend titles to individual viewers, and the growing convergence between the technology and entertainment industries has forced major studios to refocus 194 VOL 9 (1), 2020 GHOST IN THE (HOLLYWOOD) MACHINE their attention on their production and distribution methods, and in partic- ular the speed and fluidity with which new, novel, and high quality projects are pushed to audiences. This article examines the nascent emergence of AI applications in the film industry, specifically at the pre-production and greenlighting stage, where decisions are made as to the feasibility and earning potential of film projects. Based on case studies of two of the more high-profile companies providing predictive analytics to the film business, interviews conducted with their chief technology officers (CTO), and an analysis of the media discourse sur- rounding recent developments in AI in the film industry, I interrogate the critical discussions emerging from the use of intelligent algorithms in the film business. Concerns explored include the notion that the data used to train such algorithms reflect certain biases (racial, gender, or ideological) that, if left unaddressed, may shape mainstream film production and notions of diversity and novelty in problematic ways. Further, what impact will the use of AI-enabled tools in the film industry have on its human labour force, and how will this change job scopes? This article thus seeks to outline current ef- forts to navigate these questions and tease out further nuances in the cultural and industrial implications of AI’s growing influence in film production pro- cesses, particularly the ways AI might influence notions of creativity, labour, and reception. While this phenomenon is still in its infancy and the first use cases have not been made available for scrutiny, there is nonetheless an ur- gency in articulating such concerns and possible implications to create more awareness about the potential trajectories for the use of AI in film culture and in media production more broadly. Situated between the fields of media studies and critical algorithm studies, this article is less concerned with the technical details of the algorithms or the systems that underlie them, but ra- ther with ‘the meanings and implications that algorithmic systems may have’ and the socio-cultural phenomena driven by algorithmic systems. This article will contribute to current scholarly debate on AI and the audio- visual arts, wherein existing humanistic and social science research on the relationship between AI and cinema is particularly scant. I begin with a brief overview of the motivations for the film business to employ AI technologies and interrogate the ways machine intelligence is seeking to replace human intelligence. I then detail the AI tools offered by companies already working with partners in the film industry and review the popular (mis)perceptions and critical problems raised around their use in the CHOW 195 NECSUS – EUROPEAN JOURNAL OF MEDIA STUDIES creative milieu. Here lies the question: what impacts will arise from such ‘hy- brid human-machine behaviour’? Finally, I offer three paths forward for further research and critical interdisciplinary engagement with this emerg- ing convergence between artificial intelligence and the film machine. Machine learning and solutionism in the film industry In the context of ‘technological solutionism’ – a popular ideology among those in the technology industry that many, if not all, of the world’s problems can be solved by implementing technological solutions – many of the companies and their AI-powered platforms described in this article were formed to solve what is perceived to be a particular problem faced by pro- ducers and studios in the film business when it comes to the high financial risks involved in making decisions about which screenplays to invest in. Greenlighting films based on human ‘gut feeling’ and perceived as probable box office successes according to subjective assessments of what audiences prefer at a certain point in time do not always turn out to be profitable gam- bles. Michael Pokorny and John Sedgwick describe the reason behind the sin- gle ‘brutal constant’ in the film industry, which is that only a small proportion of films made ever make profits: In pure investment terms, the low incidence of hit films would not be a problem, if there were a basis or methodology for predicting with any level of accuracy which of the large numbers of films released annually will turn out to be hits. The problem is that such a methodology would not appear to exist – in essence, it is impossible to predict, on a consistent and regular basis, which of the hundreds of annual film re- leases will turn out to be profitable, the process being to all intents and purposes an apparently random one. It now appears that, just seven years after the publication of Pokorny and Sedgwick’s essay, this conundrum has been solved by an adjacent sector: the tech industry. By combining various sophisticated AI and machine learning techniques, big data, and principles from risk analysis within the context of the filmed entertainment business, several start-ups have sought to address some of executive Hollywood’s biggest questions when faced with new film projects: will it be a box office hit and, more importantly, should we green- light it? The new technology companies that are providing their AI-powered so- lutions to a largely conservative film industry are addressing different facets 196 VOL 9 (1), 2020 GHOST IN THE (HOLLYWOOD) MACHINE of the same problem – where some focus on providing analytics based on the content of screenplays, others focus on providing project management solutions that include predictions and insights based on casting and talent choices, budget considerations, and national and global trends. What con- nects these different approaches is an attempt to relieve the human actor (i.e. producers, studio executives) of the mundane, low-level tasks that usually take up a lot of time (e.g. research, gathering data, making decisions) and can fall prey to human subjectivity or one’s limited experience and incomplete knowledge. These tasks can now be easily executed in a matter of minutes using apparently objective metrics by an artificially intelligent system. This demonstrates a keen business-analytical sensibility to tackling film media as a form of commodity that circulates and operates in a particular constella- tion: there is a product, specific resources and materials needed to produce and market this product, consumers, domestic and international markets, and certain notions of value within this constellation. Time is one of these resources that hold a lot of value in the film industry, and thus the main value proposition of these companies is that their products will save producers and executives valuable time that can be better spent on more complex tasks. AI as a decision support tool at the greenlighting stage It is important to note that while the following two company profiles are the two most high-profile commercial entities to have launched AI tools aimed at predicting the success of a film, they are by no means the first to have developed predictive algorithms dedicated to this goal, with researchers cre- ating machine learning-powered models as early as 2006. This section outlines the two companies’ AI solutions for the film industry, their goals, and articulates the way they position themselves vis-à-vis the filmmaking process. ScriptBook ScriptBook was established in 2015 and currently has six employees in Ant- werp, Belgium. Founded by its CEO Nadira Azermai, who holds degrees in engineering, law, and applied economics, the company seeks to replace the processes of subjective decision-making undertaken by executives at film CHOW 197 NECSUS – EUROPEAN JOURNAL OF MEDIA STUDIES companies and distributors with their web-based solution of a ‘decision sup- port AI’ that combines the techniques of data mining, machine learning, nat- ural language processing, and feature engineering. ScriptBook uses these techniques to process input (film screenplays) and generate a unique output (analytics about the features of the screenplay, its commercial viability across different facets, and a final recommendation to greenlight or reject), reduc- ing what might have taken days or weeks of research and deliberation as well as a fair amount of uncertainty and risk-taking down to a matter of minutes. What ScriptBook’s validation tool seeks to complement is decades of ex- pertise honed by these gatekeepers and tastemakers of Hollywood. What a producer would have gained in experience over years of reading and making subjective value judgements about screenplays is now standardised and au- tomated by ScriptBook’s algorithms powered by big data and over 6,500 scripts (and counting) of varying box office success levels. Yet the company is keen to highlight that their algorithm is emphatically not a replacement for a human decision-maker. Rather, their tool exists to provide a wealth of in- formation that would otherwise require resources far and beyond what is at the disposal of human agents, and ultimately to assist the user in their final deliberations about a screenplay. Upon logging in and uploading a screenplay, ScriptBook’s interface dis- plays what the company calls the ‘Script DNA’, featuring a dashboard that shows predictions for the screenplay’s genre(s), MPAA (Motion Picture Asso- ciation of America) rating, and a list of similar movies. Further statistics and analytics are presented graphically to show a scene analysis, calculations of each character’s likeability, emotions by scene (including an overall sum- mary), as well as measurements of gender equality in the screenplay based on the parameters of the Bechdel Test and statistics on the percentage of characters, speaking lines, interactions, and screen presence for the different genders. A second section features further analytics about the potential audi- ence, predicting the gender and age breakdown of the target audience and predictions of audience satisfaction ratings on IMDb, Rotten Tomatoes, and the film’s Metacritic score. An interactive graph plots where the screenplay might be positioned in relation to other existing films in the market, allowing the user to view different graphs according to the selected relationships be- tween audience reach, production budget, and/or total US box office earn- ings. Finally, and perhaps the section that speaks most directly to studio ex- ecutives, ScriptBook provides financial forecasts relating to the predicted production budget (based on certain parameters like cast, writers, number of 198 VOL 9 (1), 2020 GHOST IN THE (HOLLYWOOD) MACHINE scenes, locations), US and international box office returns, the return on in- vestment (ROI), and even a world map showing in which territories the screenplay is likely to find a receptive audience. Azermai claims that ScriptBook can produce financial forecasts with an 86 per cent success rate and process up to 1,000 scripts each day. To feed their algorithms with training data, the company started by first targeting companies in the North American film market who were more willing to share screenplays and data about their films with a yet-unknown technology. According to the company’s CTO, Michiel Ruelens, European film and me- dia companies are now more receptive to sharing their data and screenplays, which has meant more diversity in ScriptBook’s training data that will help to improve its accuracy. The cinemas of Nigeria, India, and Chinese-language regions are the next challenge for the company, where the prolific produc- tion volume of Nollywood, Bollywood, and Huallywood present a greater opportunity to further diversify and enrich ScriptBook’s offering and make it a more globally oriented tech solution for film companies internation- ally. Aside from and building on their decision support AI product, the com- pany is simultaneously delving more directly into the creative realm by pro- ducing a generative AI called DeepStory, what they hope will be a sophisti- cated script generator tool that can be used by screenwriters in the film in- dustry. Here, AI moves from being a tool that supports decision-making to being a co-writer in the writer’s room. ScriptBook writes that while Deep- Story’s generative engine (created by transferring the data and parameters from their script analysis AI to a language model) is still at the develop- mental stages, it is already capable of more sophisticated output than existing text generators which tend to produce narratives that are incoherent and lack the ability to parse for meaning and relevance to an overall narrative. Cinelytic On 8 January 2020 the Hollywood studio Warner Bros. announced that it had signed a deal with a Los Angeles-based AI company called Cinelytic to adopt their AI-driven project management workflow system. This is the larg- est and most high-profile entertainment company to have publicly partnered with Cinelytic, a four-year-old company whose platform – only launched in 2019 – provides a variety of predictive analytics tools to aid decision-making CHOW 199 NECSUS – EUROPEAN JOURNAL OF MEDIA STUDIES at the greenlighting stage. While the behemoth studio will not be incorporat- ing Cinelytic wholesale to automate decisions about which films to make, studio executives will instead use the AI-driven platform to reduce the amount of time spent on repetitive tasks such as providing ‘dollar-figure pa- rameters for packaging, marketing and distribution decisions, including re- lease dates’. Cinelytic’s platform mimics the project workflow that producers carry out, integrating several tools for each step along the way within a dashboard interface and, where relevant, uses AI to help with accuracy, speed, and the scale of analysis. The company bills its platform as ‘[t]he only end-to-end, self-service data, analytics and predictive intelligence platform in the enter- tainment industry’. Its features include film and talent analytics, where users can toggle between cast options to see how well the film would do, dis- tribution and release analytics to inform strategies, financial modelling to as- sist with budgeting, and real-time predictive forecasting intelligence for rev- enue prediction across different territories. While ScriptBook’s platform is based on the user submitting a script and their AI generating the various pre- dictions based on the story’s inherent features, Cinelytic’s platform requires users to key in specific data into individual fields describing the main features of a film project, including the choice of talent (i.e. which specific directors, actors, writers, or producers), budget, run time, themes, release strategy, whether it is an original film or an adaptation or sequel, and so on. The tool for revenue prediction alone, for instance, contains up to nineteen input fields. The predictive model then runs the user’s input against information from databases such as Nielsen, Variety Insight, and the major social media platforms to produce a detailed analysis of predicted revenues in different contexts. As the company emphasises, the Cinelytic platform is meant to be used at the greenlighting stage, the stage where producers already have a firmer idea of the kinds of projects they potentially want to initiate, after having whittled all the pitches received down to a handful of screenplays that they now want to consider seriously for financing. This is the stage where, typi- cally, weeks of research and deliberation is spent on analysing the potential revenues that can be derived from each project and negotiating the right cast- ing choices for the film, amongst other aspects. For most of these tasks, there is much grunt work being done, usually on pages and pages of spreadsheets by human analysts, whether it is to calculate potential financial outcomes for a film release across a certain number of theatres at a particular time of the 200 VOL 9 (1), 2020 GHOST IN THE (HOLLYWOOD) MACHINE year, or to produce a dollar-figure analysis of the bankability of hiring one director over another. Further, the accuracy of any analysis also depends on the databases being used, many of which are very costly – a hurdle for inde- pendent film companies on smaller budgets more than the big studios. It is thus clearly not the case that Hollywood is averse to using technological and scientific methods to produce intelligence that would support decision-mak- ing in the business of film. Hollywood has indeed, over its long history, also developed its own financial models and ways of calculating and managing risk for film projects, but much of that still relies on having the right re- sources (time, money, and manpower) and may be prone to human error in various ways. In my interview with the company’s co-founder and CTO Dev Sen, a for- mer NASA scientist who had developed risk assessment and risk manage- ment systems for rapid space shuttle launches, Sen emphasises that Cine- lytic’s platform does not seek to replace decision-making when it comes to creative matters, since those decisions would already have been made by the user before plugging information into the platform. As he describes, the user (an investor, producer, studio executive, or independent company) would al- ready have curated a shortlist about the casting options and other movie at- tributes based on the screenplay. The platform simply does the number- crunching and modelling based on the user’s input to provide detailed infor- mation that the user would use to support her decision-making process. Essentially, what Cinelytic offers its clients is a project management tool not unlike what Sen developed at NASA, proposing a system that is methodical, robust, and capable of accomplishing with a higher degree of accuracy within a significantly shorter period of time what teams of human analysts would take much longer to do. The race to create the most efficient and accurate AI tool to assist deci- sion-makers in the film business is evidently on, and as is clear from the pro- files above, targeting the financial and business aspect of the film and media entertainment industry seems a natural fit for AI technologies that are par- ticularly adept at processing large amounts of data and potentially offer speed, scale, and a certain level of accuracy and objectivity to the decision- making process. Other companies that have entered the fray include an Is- raeli start-up called Vault that predicts the types of audiences for a film based on online reception to trailers; also Pilot Movies, which uses machine learn- ing to make box office predictions based on audience analytics. The film stu- CHOW 201 NECSUS – EUROPEAN JOURNAL OF MEDIA STUDIES dio 20th Century Fox has their own system that uses machine vision to ex- amine trailer footage, label objects and events within these, then cross-refer- ence it with data generated for other trailers. Working with Google’s Ad- vanced Solutions Lab, data scientists at the film studio created Merlin Video, ‘a computer vision tool that learns dense representations of movie trailers to help predict a specific trailer’s future moviegoing audience’. Hollywood’s problem with AI What is significant about the partnership between Cinelytic and Warner Bros. is that this is the first public announcement (and admission) that a major Hol- lywood entertainment company will now rely on AI for what was previously thought of as a decidedly human-only and, to a certain extent, creative pro- cess. Prior to this, studios had been reluctant to make public the extent of their involvement with AI, as recounted by ScriptBook’s CEO Azermai, whose previous deals with production companies had to include non-disclo- sure agreements (NDA). This reluctance can be attributed to an adher- ence to the notion of film as a creative field that relies on human ingenuity, craftsmanship, and expertise. In particular, producers, talent scouts, agents, and others in the film industry whose jobs are based on their ability to create, identify, and assess creative and monetary value – or, as Pierre Bourdieu writes, to ‘consecrate’ – may find their authority and influence being chipped away by AI agents built to do the same tasks. Within the corpus of media coverage on the use of AI in Hollywood stud- ied for this article, there is a noticeable range of opinions about the use of AI applications in film that ranges from the negative to the cautiously op- timistic. On the optimistic end, publications such as the technology-focused news site The Verge and the academic journal Science are pragmatic about the utility of using AI tools to aid decision-making in the business of film, arguing that such algorithms provide a more accurate, albeit imperfect, prediction about the performance of a film that would realistically only be utilised as an aid by its human users. That is, human producers or investors would ultimately hold the final decision. On the other end of the spectrum, some articles suggest the eventual replacement of human workers from the film production process with AI-enabled automation. An article from The New York Times reporting on the use of AI in the creation of visual effects argues that AI will indeed replace specialists by using computer vision and 202 VOL 9 (1), 2020 GHOST IN THE (HOLLYWOOD) MACHINE neural networks to automate the tedious task of creating special effects frame by frame. Yet, while putting practitioners out of a job, the technology at this stage still contains flaws and in some cases requires humans to make ad- justments to the final output. A more doomsday-laden remark opens Tatiana Siegel’s report on Cinelytic’s deal with Warner Bros. in The Hollywood Re- porter: ‘Resistance is futile.’ While the rest of Siegel’s article presents the case for how Hollywood executives would use AI as a time-saving tool for decision-making, it still emphasises the anxieties inherent in the common narrative that human creativity and intelligent machines simply do not mix. This demonstrates a journalistic tendency – matching a general trend in AI coverage in popular media – of presenting a pessimistic scenario where hu- man jobs are replaced by algorithms in a black box, and where cinematic cre- ativity and diversity will be reduced in favour of homogeneity and standard- isation across the film industry. For the most part, these articles do rely on generalisations and tend to play to the public’s lack of knowledge of the actual limitations of specific intelligent algorithms in particular contexts, and this ignorance is especially potent in our contemporary mediascape where algo- rithms are widely perceived to have become ‘a key site of power’. Specific to the case studies described above, one obvious critique – and one that is certainly a valid concern – is that the introduction of AI into the decision-making process at the greenlighting stage will spell the end of crea- tive, novel, and ‘risky’ projects being produced, and this would be detrimental to the diversity of the film ecosystem at various levels. Screenplays assessed to be financial failures, even if they contained creative or novel aspects, would be dismissed in favour of screenplays that follow the templates of suc- cessful films, which, given Hollywood’s taste for popular and ‘safe’ narratives and representations, would be detrimental to current efforts to dismantle structural inequalities in film. Following this line of thought, actors, di- rectors, or writers who have been proven successes in the past would be hired again and again, displacing new and emerging talent who have yet to estab- lish strong track records. Practitioners of minority ethnicities, or stories and character relations that deviate from a predominantly white, conservative, heteronormative patriarchal worldview would be assigned a low probability of success based on historical trends. That is, if the datasets being used to train the algorithms are already biased in specific ways, using them would only perpetuate the same biases in the industry, cancelling out the progress being made in the current fight for greater diversity, equality, and fair representa- tion in the industry. CHOW 203 NECSUS – EUROPEAN JOURNAL OF MEDIA STUDIES This line of argument results in a concern that film culture will become homogenised. Given the influential role of screen media in shaping worldviews, the mediation and repetition of certain images and representa- tions has the capacity to ‘destroy the relationship between sign and referent leading to a condition of hyper-reality in which images take on a distinct ex- istence and meaning’ for the viewing subject and certain values and ideas become normalised. Given also Hollywood’s relative dominance of the global entertainment landscape, a more extensive use of AI in the film indus- try to screen and select which stories to tell would have far-reaching ethical and cultural implications for the ways people understand themselves and each other. As Sy Taffel notes, various forms of (largely racial) discrimination have long been pervasive within media representations and technologies, and that ‘whiteness, alongside a male gaze and bourgeois ideology have long been problematically universalised and invisibly normalised’. If care is not taken to address and eliminate potential sources of bias inherent in the film-cultural data from which algorithms learn, the potential trade-off for the efficiency that machine intelligence offers is that ‘they tend to normalise the situations in which they are entangled’. The film ecosystem (and indeed most other cultural business sectors) comprises two interconnected but nevertheless separate domains. One is the creative, artistic, and qualitative side that is concerned with the production of ideas, stories, and talent; the other is the business end that is concerned with the quantitative aspects of financing, marketing, and distribution of those ideas, stories, and talent. It is in this latter domain that a wealth of data is being produced, and if there is one thing that AI is good at it is analysing and processing data. This ability to analyse and process data at speed and at scale is furthermore a concern for those in the film business whose jobs now ap- pear to be threatened. These are the employees within studios or production companies who conduct market research, industry analyses, audience sur- veys, financial forecasts – the very tasks that can now be executed within minutes by the AI platforms. In this case, their fears are certainly valid, and it is up to company executives to decide how reliant they will be on the AI tools over their human colleagues. 204 VOL 9 (1), 2020 GHOST IN THE (HOLLYWOOD) MACHINE Addressing the pushback There are evidently several tensions inherent in this coupling of AI with the film business, with many critics from both within and outside of the film in- dustry highlighting potential ethical pitfalls that the use of AI may bring to cinematic production. Companies like ScriptBook and Cinelytic are invaria- bly cognizant of such critiques and spend significant amounts of time ad- dressing them through press releases and at frequent speaking engagements. Their responses typically focus on insisting on the adaptability of the AI models, their higher degree of objectivity, the notion that creativity can be mathematically modelled, and that the eventual impact of their predictive models is only minimal since the actual creative decisions are either made before or after the involvement of AI. Across these various defensive strate- gies is the strong belief that the technology will be able to deal with and po- tentially even solve problems of bias and lack of diversity. While at an academic and industry conference in November 2019 ad- dressing the intersections between AI and creativity I witnessed various articulations of the same anxieties detailed above directed at the representa- tives of AI companies who were working on applying AI to creative and busi- ness problems in the creative industries. ScriptBook’s CTO Reulens was part of a panel titled ‘Just a Member of the Band’ that explored how AI extends human creative abilities. In his talk ‘Democratising Filmed Entertainment Through AI’, Ruelens sought to push back against the pushback, explaining that the deep learning processes in their algorithm takes into account a broad swathe of attributes and factors that can mitigate against reproducing formu- laic results, while also arguing that the system’s loss function helps to contin- ually reconfigure the model’s predictive accuracy by taking into account em- pirical experience. As for the question of bias, Ruelens acknowledged that the data collected is already biased and that the algorithm is only as good as its dataset, and so the company’s task is to put in their ‘best effort’ to collect as many scripts as possible to build a varied and diverse dataset. Elsewhere, in an essay aimed at addressing critics’ scepticism about the ‘perceived irreconcilability between computerized algorithms and the hu- man creative process’, Ruelens suggests that the question of whether an AI agent is capable of judging the value of human creativity is what drives critics’ concerns. To this, he emphasises the ability of ScriptBook’s machine learning-driven platform – and indeed of AI’s general ability – to detect pat- terns from large datasets of tens of thousands of screenplays and to correlate CHOW 205 NECSUS – EUROPEAN JOURNAL OF MEDIA STUDIES ‘the content of all of those scripts to financial and critical success in an objec- tive manner’. The essay goes on to argue that stories that stand the test of time inherently share similar structural elements and that thus ‘creativity tends to follow structure’, where AI is capable of learning and analysing com- mon structural elements at a very large scale. Despite not knowing what ‘orig- inality’ is, the agent is still able to produce predictions that ‘make sense on a human, intuitive level’. Hence, even while algorithmic agents are unable to experience emotional responses when analysing a script, what they do well is to spot patterns that elicit those emotions in humans and ultimately incorpo- rate these patterns into their predictions. Cinelytic’s CTO Sen is also keen to emphasise that he and his team are conscious of the problems of diversity and of bias creeping into their system, and this might be more salient in their talent analytics tool than the others. In a future iteration of the platform, the company plans, amongst other up- grades, to incorporate a variant of a recommender system into its talent an- alytics tool, using natural language processing and machine vision to poten- tially analyse actors’ screen performances and subsequently recommend a curated selection of casting options for a particular character profile. This, Sen believes, could be a method and tool that helps to make visible unestab- lished but high-potential acting talent who may only have one credit to their name or have only been in films that few have seen, for instance. The companies see themselves as providing a service that is beneficial to the film community, following the dominant imaginary of AI providing ef- ficiency and maximisation, and have in various ways vocalised their awareness of the potential ethical problems as an attempt to build trust. One strategy is to emphasise disclaimers, that their platforms simply serve as an informational aid and that the human is still ultimately in charge of the final decisions, while also reminding critics of the fact that AI models as they stand today are still a long way off from achieving true intelligence that matches or even surpasses the human ability to create. Thus, it is evident that both Ru- elens and Sen are careful to position their respective platforms as ‘decision support tools’ and are not aiming to supplant human executives. Another strategy is to argue that the onus lies on users or audiences to reject formulaic material and to highlight where the algorithm is decidedly off the mark; and since the systems are continuously learning from feedback and thus able to ‘self-correct’, it would certainly take into account flops that it had suggested as potential successes and learn from the feedback loop. This way, changes and the diversity in audience preferences and cultural trends are, to an extent, 206 VOL 9 (1), 2020 GHOST IN THE (HOLLYWOOD) MACHINE fed back into the system, and this would theoretically improve the algo- rithm’s accuracy. It is further interesting to note that none of the key people responsible for developing the algorithms in both companies – and indeed many of their other competitors – come from a background in film or the entertainment business. This is a necessary requisite to create an objective distance from the film industry, as having prior experience may not be useful for the objectiv- ity of their product. Ruelens emphasises that this distance is an important element in ensuring that not only will there be no conflict of interest between them and their clients, but also that their programming is not influenced by insider knowledge and industry-inflected biases. Sen’s career history as a NASA scientist and the fact that he is using knowledge and technological ex- pertise gained from a high-risk environment and applying it to a creative field has also lent Cinelytic a particular appearance of objectivity and relia- bility that the company is keen to emphasise. In different ways, both compa- nies articulate the message that their roles are simply to develop tools to help solve problems, a perspective that falls very much in line with the discourse of tech solutionism, where creativity is framed as a ‘problem’ that needs to be ‘solved’ or improved by technology. Three paths forward for critical research In reality, the time when intelligent algorithms can produce original and cre- ative cinematic screenplays indistinguishable from those written by humans is still a long way off, despite some advances being made in this particular area. For now, more attention needs to be paid to the precise ways that AI is being used in the film industry, what it produces and changes, who it influ- ences, and what longer-term impacts it may have on film culture. The issue of trust also underlies much of the tensions and relationships between AI and the film industry, and care must be taken to identify and articulate how these AI companies, their clients, critics, and audiences understand and influence each other. As Virginia Dignum argues, AI systems, in whatever context they are operating, ‘must be introduced in ways that build trust and understanding, and respect human and civil rights’. To assert that AI will soon take over both the business and creative aspects of the film industry and put people out of jobs is to some extent an overre- CHOW 207 NECSUS – EUROPEAN JOURNAL OF MEDIA STUDIES action, albeit a justifiable concern. Simon Chandler, writing in Forbes, high- lights some probable futures of how AI film prediction platforms might be used in Hollywood. Citing South Korean researchers who created a bot that excelled at predicting critical and box office failures using deep learning based on data harnessed from plot summaries and critical rankings from ag- gregation sites like Rotten Tomatoes, Chandler surmised that it might be likely that more Hollywood studios would use AI for this specific use – that is, to rely on such predictive analytics to weed out screenplays deemed as flops before they even end up on executives’ desks. This is precisely what companies like ScriptBook and Cinelytic have developed, albeit in slightly different forms – a risk assessment tool that automates number-crunching and research using big data to produce business intelligence for its users. As studios have only just begun to adopt these intelligent predictive technolo- gies into their business workflows, it remains to be seen how film culture is impacted in the long run. Hence, more qualitative research needs to be done as the use of AI in film – at the various stages of production – progresses. By way of a conclusion, this section describes some possible approaches and ways forward in this area. Examining the long-term impact of AI use in the commissioning process What is the impact on film-cultural production of using AI tools? Details and analyses about real use cases are currently limited, as companies are loathe to reveal too much or are under NDAs with the film companies they have deals with. Press releases from either film studios or AI companies are nec- essarily skewered to showcase successful cases or to present evidence of the positive aspects of how machine learning supports creativity and sound busi- ness decision-making. Furthermore, little has been said or revealed about how outliers like independent, low-budget, or art film productions might fare in the Hollywood system in a future driven (at least partially) by AI. More longitudinal research on case studies is therefore needed to verify the actual significance and impact that hybrid human-AI decision-making has on cre- ative industries and on film over a period of time. 208 VOL 9 (1), 2020 GHOST IN THE (HOLLYWOOD) MACHINE Ethnographic studies of both AI and film practitioners What are the social and ethical impacts of the use of AI in the film industry? Another strand of research would relate to the gamut of practitioners in- volved in this specific milieu, ranging from the AI engineers and chiefs of the technology companies providing services to the film practitioners whose work will be or is already impacted by the adoption of AI technologies (such as editors, studio executives, producers, cast, and other crew). More under- standing and empirical evidence in this area could support and drive future work on how to manage bias in the process or how practitioners work to- gether to manage the complex balance between the desire for diversity and novelty in the film business and the efficiency and accuracy of the predictive analytics based on historical data that might contain its own problems. For instance, more ethnographic work could be done on understanding how those who work on programming the algorithm or coding data see them- selves as part of the film creative process (or not), and how this influences their work and their interactions. In this area, examining the ways the differ- ent parties build trust, construct codes of conduct, speak about value, articu- late their identities, and negotiate relationships of power within this constel- lation of interactions may surface important insights into the effects gener- ated by the use of intelligent algorithms. Technicalities of the algorithms How do algorithms behave? Research focusing on the impact and behaviour of the algorithms ‘in the wild’ relates to the field of ‘machine behaviour’, an emerging interdisciplinary field that incorporates anthropological and eth- nographic methods from the humanities and social sciences into AI research, focusing particularly on the interactions between humans and machines and the socio-political impacts of the behaviour of algorithms used in society. This approach further speaks to a broader critical milieu that dissolves the boundaries between human and machine, preferring to conceptualise both as material actants where (creative) agency arises from the processes and re- lations between them, and that recognises that algorithmic machines are in fact, as Donna Haraway declares, ‘lively’. Following Haraway’s legendary cyborg, critics such as Hito Steyerl are at- tentive to the ways humans ‘feed affect, thought, and sociality into algorithms’ while algorithms ‘feed back into what used to be called subjectivity’. Re- search into the growing influence of AI and machine learning in the film and CHOW 209 NECSUS – EUROPEAN JOURNAL OF MEDIA STUDIES media industries can be interrogated within this interdisciplinary field of the- oretical and methodological work to produce critical insights into these emergent articulations of human-machine interactions and their impact on historical and current perceptions of creativity. A significant part of this ap- proach would thus involve a higher degree of granularity in analysing and explaining how, for example, ScriptBook’s algorithms process a script, how the deep learning model is reconfigured in response to feedback, or how much of its behaviour can be attributed to its human engineers. How do en- gineers label and classify the training data (e.g. attributes in screenplays, def- initions of a box office success) and how does this influence the efficacy of the AI model? Further, how does moderation take place, and do external hu- man stakeholders or economic forces have an effect on the behaviour exhib- ited by the algorithms? To what extent are the AI models used in film pow- ered by what I call culturally adaptable algorithms – algorithms that adapt, interact, and respond to wider shifts and phenomena in culture – and what implications would this have? While this particular strand of research appears to rely on the film and media studies researcher having a greater depth of understanding of compu- ting and the technicalities of the algorithms, this is not what I nor the authors of the Nature review article advocate. Instead, I posit that this is an oppor- tunity for film and media studies to engage meaningfully with a relatively new and impactful technology that is now inserting itself not only into our field of inquiry and creative practice, but more generally into nearly all parts of public and private life. In the creative and artistic milieu within which film sits, it is now more urgent than ever to understand, make transparent, and interrogate the behaviours of intelligent algorithms, their creators, and users. Author Pei-Sze Chow is a Marie Skłodowska-Curie Fellow at the Department of Me- dia Studies in Aarhus University. Her research sits at the intersection of screen aesthetics and media geography, focusing on questions of space, iden- tity, and representation in peripheral screen cultures. She is the author of Transnational Screen Culture in Scandinavia and co-editor of A History of Danish Cinema, both forthcoming in 2021. 210 VOL 9 (1), 2020 GHOST IN THE (HOLLYWOOD) MACHINE References Boden, M (ed.). Artificial intelligence. Handbook of Perception and Cognition, second edition. San Diego: Academic Press, 1996. _____. ‘Creativity and artificial intelligence’, Artificial Intelligence, No. 103, 1998: 347-356. Bourdieu, P. The field of cultural production: Essays on art and literature, edited by R. Johnson. New York: Columbia University Press, 1993. Brundage, M., Avin, S., Clark, J., Toner, H., Eckersley, P., Garfinkel, B., Dafoe, A., et al. ‘The Malicious Use of Artificial Intelligence: Forecasting, Prevention, and Mitigation’, ArXiv:1802.07228 [Cs], 20 February 2018: http://arxiv.org/abs/1802.07228. Bucher, T. If…then: Algorithmic power and politics. New York: Oxford University Press, 2018. Campo-Rembado, M. and Oakley, S. ‘How 20th Century Fox Uses ML to Predict a Movie Audience’, Google Cloud Blog, 29 October 2018: https://cloud.google.com/blog/products/ai-machine-learn- ing/how-20th-century-fox-uses-ml-to-predict-a-movie-audience/ (accessed on 17 January 2020). Chandler, S. ‘Hollywood Is Using Artificial Intelligence To Pick Its Next Blockbuster’, Forbes, 10 January 2020: https://www.forbes.com/sites/simonchandler/2020/01/10/hollywood-is-using-artificial- intelligence-to-pick-its-next-blockbuster/ (accessed on 14 January 2020). Daniele, A. and Song, Y. ‘AI + Art = Human’, Proceedings of the 2019 AAAI/ACM Conference on AI, Ethics, and Society – AIES ’19, 2019: 155-161. Dignum, V. ‘Ethics in Artificial Intelligence: Introduction to the Special Issue’, Ethics and Information Technology, Vol. 20, No. 1, March 2018: 1-3. Du, J., Xu, H., and Huang, X. ‘Box Office Prediction Based on Microblog’, Expert Systems with Applica- tions, Vol. 41, No. 4, March 2014: 1680-1689. Edgerton, G. ‘SILICON VALLEY + HOLLYWOOD = CONVERGENCE 2.0’, Critical Studies in Television Online (blog), 31 January 2020: https://cstonline.net/silicon-valley-hollywood-convergence-2-0- by-gary-r-edgerton/ (accessed on 31 January 2020). Frederick, E. ‘Artificial Intelligence Predicts Which Movies Will Succeed – and Fail – Simply from Plot Summaries’, Science, 2 August 2019: https://www.sciencemag.org/news/2019/08/artificial-intelli- gence-predicts-which-movies-will-succeed-and-fail-simply-plot (accessed on 29 January 2020). Gillespie, T. ‘Algorithm’ in Digital keywords: A vocabulary of information society and culture, edited by B. Peters. Princeton Studies in Culture and Technology. Princeton: Princeton University Press, 2016: 18-30. Haraway, D. ‘A Cyborg Manifesto: Science, Technology, and Socialist Feminism in the Late Twentieth Century’ in Simians, cyborgs, and women: The reinvention of nature. London: Free Association Books, 1991: 149-181. Holford, W. ‘The future of human creative knowledge work within the digital economy’, Futures, No. 105, 2019: 143-154. Kim, Y., Cheong, Y., and Lee, J. ‘Prediction of a Movie’s Success From Plot Summaries Using Deep Learning Models’, Proceedings of the Second Workshop on Storytelling, 2019: 127-135. Latour, B. Reassembling the social: An introduction to actor-network-theory. Oxford: Oxford University Press, 2005. Lee, K., Park, J., Kim, I., and Choi, Y. ‘Predicting movie success with machine learning techniques: ways to improve accuracy’, Information Systems Frontiers, Vol. 20, 2018: 577-588. Mackenzie, A. ‘The Production of Prediction: What Does Machine Learning Want?’, European Journal of Cultural Studies, Vol. 18, no. 4-5, August 2015: 429-445. _____. Machine learners: Archaeology of a data practice. Cambridge: The MIT Press, 2017. Metz, C. ‘Lights, Camera, Artificial Action: Start-Up Is Taking A.I. to the Movies’, The New York Times, 26 March 2018. Morozov, E. To save everything, click here: The folly of technological solutionism. New York: PublicAffairs, 2013. CHOW 211 NECSUS – EUROPEAN JOURNAL OF MEDIA STUDIES Napoli, P. ‘On Automation in Media Industries: Integrating Algorithmic Media Production into Media Industries Scholarship’, Media Industries Journal, Vol. 1, No. 1, 2014: 33-38: https://doi.org/10.3998/mij.15031809.0001.107 Natarajan, S. and Bauer, J. ‘AI: Hollywood’s Rising Star’, Broadcasting & Cable, 23 July 2019: https://www.broadcastingcable.com/blog/ai-hollywoods-rising-star (accessed on 28 January 2020). Philpott, S. and Mutimer, D. ‘Inscribing the American Body Politic: Martin Sheen and Two American Decades’, Geopolitics, Vol. 10, No. 2, July 2005: 335-355. Pokorny, M., and Sedgwick, J. ‘The Financial and Economic Risks of Film Production’ in Film and risk, edited by M. Hjort. Detroit: Wayne State University Press, 2012: 181-196. Rahwan, I., Cebrian, F., Obradovich, N., Bongard, J., Bonnefon, J., Breazeal, C., Crandall, J., et al. ‘Ma- chine Behaviour’, Nature, Vol. 568, No. 7753, April 2019: 477-486. Ruelens, M. Interview. Interview by Pei-Sze Chow, 20 November 2019. Rose, S. ‘“It’s a War between Technology and a Donkey” – How AI Is Shaking up Hollywood’, The Guardian, 16 January 2020: https://www.theguardian.com/film/2020/jan/16/its-a-war-between- technology-and-a-donkey-how-ai-is-shaking-up-hollywood (accessed on 17 January 2020). Russell, S. ‘Machine Learning’ in Artificial intelligence, edited by M. Boden. Handbook of Perception and Cognition, second edition. San Diego: Academic Press, 1996: 89-133. ScriptBook. ‘Artificial Intelligence (A.I.) and the Myth of It Killing Creativity’, ScriptBook Blog, 3 May 2018: https://blog.scriptbook.io/artificial-intelligence-a-i-and-the-myth-of-it-killing-creativity- 9909957cec14 (accessed on 13 January 2020). Seth, R. ‘What New Technology Means For The Future Of Film’, British Vogue, 13 June 2019: https://www.vogue.co.uk/article/what-vr-ai-and-interactivity-mean-for-the-future-of-film (ac- cessed on 28 January 2020). Sen, D. Interview with Dev Sen, Co-Founder and CTO of Cinelytic. Interview by Pei-Sze Chow, 29 January 2020. Shao, P. Hua Laiwu Dianying Gailun [Introduction to Huallywood Cinema]. Hangzhou: Zhejiang Uni- versity Press, 2014. Sharda, R. and Delen, D. ‘Predicting Box-Office Success of Motion Pictures with Neural Networks’, Expert Systems with Applications, Vol. 30, No. 2, February 2006: 243-254. Siegel, T. ‘Warner Bros. Signs Deal for AI-Driven Film Management System (Exclusive)’, The Hollywood Reporter, 8 January 2020: https://www.hollywoodreporter.com/news/warner-bros-signs-deal-ai- driven-film-management-system-1268036 (accessed on 13 January 2020). Smith, S., Choueiti, M., Pieper, K., Yao, K., Case, A., and Choi, A. Inequality in 1,200 popular films: Exam- ining portrayals of gender, race/ethnicity, LGBTQ & disability from 2007 to 2018. USC Annenberg In- clusion Initiative, September 2019. Steyerl, H. ‘Proxy Politics: Signal and Noise’, e-flux journal, No. 60, December 2014: https://www.e- flux.com/journal/60/61045/proxy-politics-signal-and-noise/. Taffel, S. ‘Automating Creativity – Artificial Intelligence and Distributed Cognition’, spheres: Journal for Digital Cultures, No. 5 Spectres of AI, November 2019: 1-9. Vincent, J. ‘Hollywood is quietly using AI to help decide which movies to make’, The Verge, 28 May 2019: https://www.theverge.com/2019/5/28/18637135/hollywood-ai-film-decision-script-analy- sis-data-machine-learning (accessed on 28 June 2019). Zhang, L., Luo, J., and Yang, S. ‘Forecasting Box Office Revenue of Movies with BP Neural Network’, Expert Systems with Applications, Vol. 36, No. 3, April 2009: 6580-6587. 212 VOL 9 (1), 2020 GHOST IN THE (HOLLYWOOD) MACHINE Notes Daniele & Song 2019, p. 155. Natarajan & Bauer 2019. Boden 1996, p. xv. Russell 1996, pp. 89-90. Mackenzie 2017, p. 6. Edgerton 2020. Napoli 2014, p. 34. Bucher 2018, p. 29 Gillespie 2016, p. 27. Rahwan et al. 2019, p. 482. Morozov 2013. Pokorny & Sedgwick 2012, p. 181. See for instance Sharda & Delen 2006, Zhang et al. 2009, and Du et al. 2014. A demo version is available at https://demo.scriptbook.io/. Seth 2019. Shao 2014. Ruelens 2019. A statistical model that predicts and writes text by implementing deep learning techniques. Siegel 2020. Cinelytic 2020. Sen 2020. Campo-Rembado & Oakley 2018. Seth 2019. Bourdieu 1993, p. 76. Twelve press articles across broadsheets and industry publications gathered from the period 2018-2020 were studied. Vincent 2019. Frederick 2019. Metz 2018. Siegel 2020. Bucher 2018, p. 32. Smith et al. 2019. Philpott & Mutimer 2005, p. 355. Taffel 2019, p. 7. CHOW 213 NECSUS – EUROPEAN JOURNAL OF MEDIA STUDIES Mackenzie 2015, p. 442. This was the Beyond Conference 2019 in Edinburgh, UK, organised by the UK Research and In- novation agency, which had a focus on the applications of artificial intelligence in the creative industries. ScriptBook 2018. Ibid., my emphasis. Ibid. Holford 2019, p. 144. Ruelens 2019. Dignum 2018, p. 1. Kim et al. 2019. Chandler 2020. Rahwan et al. 2019. Latour 2005. Haraway 1991, p. 152. Steyerl 2014. 214 VOL 9 (1), 2020