OECD Initial Policy Considerations for Generative Artificial Intelligence 2023 PDF
Document Details
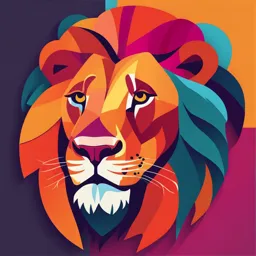
Uploaded by DelightedPolonium
Stanford University
2023
Philippe Lorenz, Karine Perset (OECD), Jamie Berryhill (OECD)
Tags
Summary
This OECD publication outlines initial policy considerations for generative artificial intelligence (AI). It explores the potential benefits and risks, including societal and economic implications. It addresses topics such as labor market impacts, copyright uncertainties, and potential for misinformation.
Full Transcript
INITIAL POLICY CONSIDERATIONS FOR GENERATIVE ARTIFICIAL INTELLIGENCE OECD ARTIFICIAL INTELLIGENCE PAPERS September 2023 No. 1 2 | INITIAL POLICY CONSIDERATIONS FOR GENERATIVE ARTIFICIAL INTELLIGENCE OECD Working Papers should not be reported as representing the official views of the OECD or of its...
INITIAL POLICY CONSIDERATIONS FOR GENERATIVE ARTIFICIAL INTELLIGENCE OECD ARTIFICIAL INTELLIGENCE PAPERS September 2023 No. 1 2 | INITIAL POLICY CONSIDERATIONS FOR GENERATIVE ARTIFICIAL INTELLIGENCE OECD Working Papers should not be reported as representing the official views of the OECD or of its member countries. The opinions expressed and arguments employed are those of the authors. Working Papers describe preliminary results or research in progress by the author(s) and are published to stimulate discussion on a broad range of issues on which the OECD works. Comments on Working Papers are welcomed, and may be sent to Directorate for Science, Technology and Innovation, OECD, 2 rue André Pascal, 75775 Paris Cedex 16, France. Note to Delegations: This document is also available on O.N.E under the reference code: DSTI/CDEP/AIGO/RD(2023)5/FINAL This document, as well as any data and any map included herein, are without prejudice to the status of or sovereignty over any territory, to the delimitation of international frontiers and boundaries and to the name of any territory, city or area. © OECD 2023 The use of this work, whether digital or print, is governed by the Terms and Conditions to be found at http://www.oecd.org/termsandconditions. OECD ARTIFICIAL INTELLIGENCE PAPERS INITIAL POLICY CONSIDERATIONS FOR GENERATIVE ARTIFICIAL INTELLIGENCE |3 Initial policy considerations for generative artificial intelligence Philippe Lorenz, Karine Perset (OECD), Jamie Berryhill (OECD) Generative artificial intelligence (AI) creates new content in response to prompts, offering transformative potential across multiple sectors such as education, entertainment, healthcare and scientific research. However, these technologies also pose critical societal and policy challenges that policy makers must confront: potential shifts in labour markets, copyright uncertainties, and risk associated with the perpetuation of societal biases and the potential for misuse in the creation of disinformation and manipulated content. Consequences could extend to the spreading of mis- and disinformation, perpetuation of discrimination, distortion of public discourse and markets, and the incitement of violence. Governments recognise the transformative impact of generative AI and are actively working to address these challenges. This paper aims to inform these policy considerations and support decision makers in addressing them. Keywords: Generative artificial intelligence, AI, digital economy, science and technology OECD ARTIFICIAL INTELLIGENCE PAPERS 4 | INITIAL POLICY CONSIDERATIONS FOR GENERATIVE ARTIFICIAL INTELLIGENCE Table of contents Foreword 5 Executive summary 6 1 Introduction to generative AI 8 Generative AI is centre stage in public, academic and political discourse 8 2 Select policy issues raised by generative AI 12 3 Potential futures for generative AI 24 4 Conclusion 29 References 30 Notes 38 Generative AI is being adopted rapidly in key industry sectors Generative AI considerably amplifies mis- and disinformation’s scale and scope Bias and discrimination Intellectual Property Rights (IPR) issues, including copyright Generative AI could impact labour markets on a different scale and scope Development trajectories of large-language and image-generating models Generative AI markets are projected to continue growing rapidly in key areas. Potential future concerns and risks Risk mitigation measures 12 13 17 19 20 24 26 26 28 FIGURES Figure 1.1. Sims-like environment for AI agents Figure 2.1. ChatGPT explains RLHF as part of an overview by HuggingFace Figure 2.2. Cases of “jailbreaks” Figure 2.3. GPT performance on academic and professional exams Figure 3.1. Comparison of Midjourney images from v1 to v5 11 18 18 21 25 OECD ARTIFICIAL INTELLIGENCE PAPERS INITIAL POLICY CONSIDERATIONS FOR GENERATIVE ARTIFICIAL INTELLIGENCE |5 Foreword Generative artificial intelligence (AI) came onto the scene in 2018 with releases of deepfakes closely followed by generative pre-trained transformers (GPTs) and other large language models (LLMs). It gained worldwide attention in 2022 with text-to-image generators and ChatGPT. Generative AI has the potential to revolutionise industries and society. Sectors such as education, entertainment, healthcare, and scientific research already use it to create individualised and scalable content, automate tasks, generate hypotheses, and improve productivity. However, policymakers need to consider significant societal and policy implications, such as the technology’s potential impact on labour markets and debates around whether training generative AI systems on copyrighted material could constitute infringement. Potential risks include generative AI perpetuating biases and being misused through disinformation, deepfakes, and other manipulated content with severe consequences. The resulting widespread social, political, and economic repercussions could include disinformation on key scientific issues, perpetuating stereotypes and discrimination, distorting public discourse, creating and spreading conspiracy theories and other disinformation, influencing elections, distorting markets, and even inciting violence. There are more questions than answers about how this technology will shape our environments and interactions, and policy is struggling to keep up with developments. That said, governments recognise the transformative nature of generative AI and are acting to keep pace with change. For example, in May 2023, the Group of Seven (G7) countries committed to advance international discussions of its governance in pursuit of inclusive and trustworthy AI, which included the establishment of the Hiroshima AI Process by governments in collaboration with the OECD. The OECD, including through its OECD.AI Policy Observatory (https://oecd.ai), is committed to helping governments keep up with the rapid change in generative AI. This paper was drafted by Philippe Lorenz, an external AI consultant. Strategic direction and additional analysis and content were provided by Karine Perset, Head of the OECD.AI Policy Observatory, and Jamie Berryhill, AI Policy Analyst, OECD. Sebastian Hallensleben (CEN-CENELEC JTC 21 and VDE) advised on the strategic approach and scope. The paper benefited from the review and input of the OECD Working Party on Artificial Intelligence Governance (AIGO), including delegates from Business at OECD (BIAC), the European Commission, and Japan. The team gratefully acknowledges the input from OECD colleagues Jerry Sheehan, Audrey Plonk, Hanna-Mari Kilpelainen, and Riccardo Rapparini of the Directorate for Science, Technology and Innovation (STI); and Angelica Salvi Del Pero and Stijn Broecke of the Directorate for Employment, Labour and Social Affairs (ELS). The team also thanks Misha Pinkhasov for editing the paper and Andreia Furtado for editorial and publishing support. OECD ARTIFICIAL INTELLIGENCE PAPERS 6 | INITIAL POLICY CONSIDERATIONS FOR GENERATIVE ARTIFICIAL INTELLIGENCE Executive summary Generative AI systems create novel content and can bring value as autonomous agents Generative artificial intelligence (AI) systems create new content—including text, image, audio, and video—based on their training data and in response to prompts. The recent growth and media coverage of generative AI systems, notably in the areas of text and image generation, has spotlighted AI’s capabilities, leading to significant public, academic, and political discussion. In addition to generating synthetic content, generative AI systems are increasingly used as autonomous agents with new functionality enabling them to operate on real-time information and assist users in new ways, such as by making bookings autonomously. Investment banks, consulting firms, and researchers project that generative AI will create significant economic value, with some estimating as much as USD 4.4 trillion per year. Generative AI could revolutionise industries and society but carries major risks Generative AI is already used to create individualised content at scale, automate tasks, and improve productivity. Generative AI is yielding benefits in key sectors such as software development, creative industries and arts (e.g., artistic expression through music or image generation), education (e.g., personalised exam preparation), healthcare (e.g., information on tailored preventative care), and internet search. However, alongside the benefits, there are significant policy implications and risks to consider, including in the areas of mis- and disinformation, bias, intellectual property rights, and labour markets. Major mis- and disinformation risks from synthetic content call for novel policy solutions Humans are less and less capable of differentiating AI from human-generated content, amplifying risks of mis- and disinformation. This can cause material harm at individual and societal levels, particularly on science-related issues, such as vaccine effectiveness and climate change, and in polarised political contexts. Mitigation measures include increasing model size, developing models that provide evidence and reference source material, watermarking, “red-teaming,” whereby teams adopt an attacker mindset to probe the model for flaws and vulnerabilities, and developing AI systems that help detect synthetic content. However, these measures have limitations and are widely expected to be insufficient, calling for innovative approaches that can address the scale of the issue. Generative AI, like other types of AI, can echo and perpetuate biases contained in training data Generative AI can echo, automate, and perpetuate social prejudices, stereotypes and discrimination by replicating biases contained in training data. This can exacerbate the marginalisation or exclusion of specific groups. Mitigation approaches include enhanced inclusivity in and curation of training data, explainability research, auditing, model fine-tuning through human feedback, and “red teaming”. OECD ARTIFICIAL INTELLIGENCE PAPERS INITIAL POLICY CONSIDERATIONS FOR GENERATIVE ARTIFICIAL INTELLIGENCE |7 Legal systems are grappling with generative AI’s implications for intellectual property rights In particular, generative AI models are trained on massive amounts of data that includes copyrighted data, mostly without the authorisation of rights-owners. Another ongoing debate is whether artificially generated outputs can themselves be copyrighted or patented and if so, to whom. Progress in generative AI may increase job task exposure in high-skilled occupations Generative AI’s availability to the public has heightened focus on its potential impact on labour markets. Measures of language model performance on standardised tests, such as the bar exam for qualifying attorneys in the United States, surprised many with its strong results relative to human test-takers, suggesting possible increased job task exposure in high-skilled occupations, though lower-skilled occupations have for now been the most exposed to automation. The OECD Employment Outlook notes that AI can benefit jobs by creating demand for new tasks and complementary skills, resulting in new jobs for which human labour has a comparative advantage. Recent research shows that generative AI can improve the performance of less skilled workers. Security, surveillance, over-reliance, academic dishonesty and concentration are also risks In addition to present-day considerations of generative AI, a longer-term view helps envision the technology’s future trajectories. Generative AI and the synthetic content it produces with varying quality and accuracy can exacerbate challenges. This content proliferates in digital spaces where it is used to train generative AI models, resulting in and a vicious negative cycle in the quality of online information. It also raises concerns about automated and personalised cyber-attacks, surveillance and censorship, overreliance on generative systems despite their flaws, academic dishonesty, and concentrations of power and resources. Agency, power-seeking, non-aligned sub-goals and other potential emergent behaviours require attention Over the longer term, emergent behaviours, of which the existence is debated in the AI community, suggest additional risks. These behaviours include increased agency, power-seeking, and developing unknown sub-goals determined by machines to achieve core objectives programmed by a human but that might not be aligned with human values and intent. Some deem that if these risks are not addressed, they could lead to systemic harms and the collective disempowerment of humans. The growing impact and capability of generative AI systems has led to reflection and debates among researchers and members of the OECD.AI Expert Group on AI Futures about whether these types of models could eventually lead to artificial general intelligence (AGI), the stage at which autonomous machines could have human-level capabilities in a wide variety of use cases. Due to its potential broad societal impacts, AGI’s potential benefits and risks deserve attention, as do the potentially imminent impacts of narrow generative AI systems that may be just as significant as AGI. The longer-term benefits and risks of generative AI could demand solutions on a larger, more systemic scale than the risk mitigation approaches already underway. These measures and others are the topic of ongoing OECD work, including work of the OECD.AI Expert Group on AI Futures. OECD ARTIFICIAL INTELLIGENCE PAPERS 8 | INITIAL POLICY CONSIDERATIONS FOR GENERATIVE ARTIFICIAL INTELLIGENCE 1 Introduction to generative AI Generative AI systems create content based on training data and in response to user prompts. Their recent growth and media coverage have spotlighted AI’s capabilities, leading to significant public, academic and political discussion. In addition to creating synthetic content, generative AI systems are increasingly used as autonomous agents, allowing models to move beyond the cut-off dates in their training data to give them new potential. Generative AI has the potential to revolutionise industries and society and is already used to create individualised and scalable content, automate tasks, and improve productivity. Such systems offer significant upside but also risks for policymakers to address. Generative AI is centre stage in public, academic and political discourse Recent growth generative AI systems has drawn attention AI’s capabilities Generative artificial intelligence (AI) systems create new content in response to prompts based on their training data. The recent growth and coverage of generative AI systems has spotlighted AI’s capabilities. They include, for example, ChatGPT and BARD for text; Midjourney and Stable Diffusion for images; WaveNet and DeepVoice for audio; Make-A-Video and Synthesia for video; and multi-model systems that combine several types of media. Companies are now creating positions for “prompt engineers”, venture capitalists are positioning themselves as generative-AI investors, and governments are considering regulatory tools. Language models – one mode of generative AI – were discussed in-depth in the recent OECD report AI Language Models: Technological, socio-economic and policy considerations (OECD, 2023[1]). Other modes, such as image, audio, and video generation, are also evolving rapidly with the technology and broader policy landscape. 1 OECD ARTIFICIAL INTELLIGENCE PAPERS INITIAL POLICY CONSIDERATIONS FOR GENERATIVE ARTIFICIAL INTELLIGENCE |9 Governments quickly recognised that generative AI is transformative and are taking action While generative AI has begun to revolutionise industry and society in numerous positive ways, the technology can also be misused through disinformation, deepfakes, and other manipulated content with severe negative consequences. Despite ideas about how this technology will shape our environments and interactions, policy is struggling to keep up with technological developments, and numerous questions require answers. At the same time, governments have been quick to recognise the transformative nature of generative AI and are taking action to keep pace with change. For example, in May 2023, the Group of Seven (G7) countries committed to advance international discussions of AI governance in pursuit of inclusive and trustworthy AI and established the Hiroshima AI Process in collaboration with the OECD under the Japanese G7 Presidency to help improve governance of generative AI. 2 Generative AI is rooted in established AI concepts Although generative AI systems appear novel, their model design is based on deep neural networks (which loosely imitate information processing of neurons in the human brain) that developed incrementally through international academic and applied research since the 1950s (Goodfellow, Bengio and Courville, 2016[2]). The visible results of generative AI models are due to recent developments in the discipline of machine learning (ML). ML leverages deep neural networks to emulate human intelligence by being exposed to data (training) and finding patterns that are then used to process previously unseen data. This allows the model to generalise based on probabilistic inference, i.e. informed guesses, rather than causal understanding. Unlike humans, who learn from only a few examples, deep neural networks need hundreds of thousands, millions, or even billions, meaning that machine learning requires vast quantities of data. Few companies can create large generative AI systems and models So far, few technology companies in the world have the technological skills and capital to create major generative AI systems and models (Chawla et al., 2023[3]), such as foundation models that “are capable of a range of general tasks…[and] can be built ‘on top of’ to develop different applications for many purposes” (Ada Lovelace Institute, 2023[4]). A few multinational enterprises have been investing in AI for some time to enable their business models, be it search, advertising, or social networks. These entities seem positioned to capture a large part of the initial value created by generative AI, with systems marketed internationally embedded in software as-a-service on cloud platforms or, more recently, placed directly on devices. Open-source actors, researchers, start-ups, and SMEs are also very active However, these companies are a part of an ecosystem that includes researchers, small and medium-sized enterprises (SMEs), and other actors that contribute to and derive value from generative AI. Open-source communities are also active in the ecosystem. AI traditionally relies on a mix of proprietary and free and open-source software (FOSS) models, libraries, datasets, and other resources for commercial or noncommercial purposes under a variety of licenses. 3 Although a number of AI companies operate proprietary generative AI systems and commercialise access to them, several companies are developing open systems. The emergence of several open-source generative AI models (Dickson, 2023[5]), such as Stable Diffusion and Meta’s Llama 2, 4 contributes to the rapid innovation and development of these technologies and could mitigate ‘winner-take-all dynamics’ that lead a few firms to seize a large part of the market. Yet open sourcing entails other risks when bad actors can leverage open-source generative AI models, which will be explored in forthcoming OECD work. OECD ARTIFICIAL INTELLIGENCE PAPERS 10 | INITIAL POLICY CONSIDERATIONS FOR GENERATIVE ARTIFICIAL INTELLIGENCE Generative AI creates novel content with real-world implications A core trait of human intelligence is the cognitive capacity humans have to create content (Chawla et al., 2023[3]). Generative AI models use “prompts” (specific requests) to produce synthetic text, images, audio, and video that, already today, can be nearly impossible to distinguish from human creation (Nightingale and Farid., 2022[6]); (Abbott and Rothman, 2022[7]). The quality of large language models (LLMs) that enable text-generation has improved rapidly since the publication of the Transformers architecture by Google researchers in 2017 and reached a turning point with the release of ChatGPT in November 2022. 5 As the first conversational agent accessible through a convenient and intuitive user interface, the release surprised governments, organisations, and individuals around the world. ChatGPT is estimated to have around 100 million active monthly users, making it the fastest-growing consumer software application in history (Hu, 2023[8]). The excitement in late 2022 around text generation was repeated with regard to image generation in March 2023 because of a picture of Pope Francis wearing a white puffer jacket. Many believed that the picture was authentic, but it had been created by a synthetic image-generation software based on a user’s text prompt. It was shared widely on social and traditional media networks before finally being exposed as fake. 6 Synthetic images took off after their inception in 2014 based on the work of (Goodfellow et al., 2014[9]), which paved the way for current model capabilities. Given the rapid pace of development, synthetic images are already often indistinguishable from real ones to the human eye. Autonomous generative AI agents promise significant benefits but carry tremendous risks Furthermore, generative AI systems are increasingly used as autonomous agents, adding a new dimension to the technology’s potential and allowing models to move beyond the limitation of cut-off dates in their training data. OpenAI announced plugins in early 2023 that connect ChatGPT to third-party applications to expand its offer and find new sources of data. Before that, ChatGPT users were limited to the platform’s knowledge base from late 2021, the cut-off point for the initial training data. Receiving third-party information allows the model to use real-time data to provide more accurate and timely results and services. Plugins enable ChatGPT to operate on the most recent data available, including real-time information, such as stock prices or news articles, and to assist users in new ways (e.g., through autonomous ordering and booking). Similarly, Bing Chat is connected to the Internet and aware of current events (Conway, 2023[10]). Agent activities are not limited to machines acting on human instructions and prompts. Researchers at Stanford University and Google Research created a virtual environment in which 25 generative AI agents interacted with each other over the course of two days and exhibited human-like behaviour such as reasoning about their research careers or planning about attending social events (Figure 1.1) (Park et al., 2023[11]). While these unexpected actions were termed “emergent abilities” by some researchers (Wei et al., 2022[12]), others found these actions illusory based on the metrics programmers chose to evaluate the models (Schaeffer, Miranda and Koyejo, 2023[13]). While the debate is ongoing, the autonomous behaviour and hints of agency that very large generative AI models could be capable of enlarges the scope of their possible application, as well as the scope of considerations and unknowns for how the technology might develop. OECD ARTIFICIAL INTELLIGENCE PAPERS INITIAL POLICY CONSIDERATIONS FOR GENERATIVE ARTIFICIAL INTELLIGENCE | 11 Figure 1.1. Sims-like environment for AI agents Source: (Park et al., 2023[11]). These types of systems offer significant upside but also carry risks, such as from their ability to create and spread mis- and disinformation, use their increased agency to carry out undesired actions, or even misrepresent themselves by impersonating humans (Hurler, 2023[14]). Policymakers everywhere are taking notice, with G7 leaders setting up the Hiroshima AI Process in May 2023. 7 The European Parliament has been advocating for considering generative AI systems as general-purpose AI, which would classify them as high-risk applications and entail mandatory conformity assessments and other requirements. 8 OECD ARTIFICIAL INTELLIGENCE PAPERS 12 | INITIAL POLICY CONSIDERATIONS FOR GENERATIVE ARTIFICIAL INTELLIGENCE 2 Select policy issues raised by generative AI Generative AI is predicted to create significant economic value and social well-being and has begun to do so in key sectors. Yet generative AI can also echo, automate, and perpetuate mis- and disinformation, bias, and discrimination, and training on copyrighted data could infringe on intellectual property. The OECD finds the net impact of AI on employment to be ambiguous so far, mainly affecting job quality – generally positively – with little evidence of significant negative effects on their quantity. However, outcomes such as language models’ strong performance on standardised tests, suggest that job-task exposure to generative AI could increase and that high-skilled occupations are most exposed to recent advances. Generative AI is being adopted rapidly in key industry sectors Generative AI is predicted to create significant economic value and social well-being. Companies have begun adopting the technology to create new business opportunities, and start-ups are competing for venture capital. Popular use cases and applications to date include pre-processing data, image compression and classification, medical imaging, personalisation, and intuitive user experience (UX) interfaces (Polaris, 2023[15]). Several generative AI applications have begun to yield benefits in areas including: • Code development – Copilot, a coding assistant developed jointly by OpenAI and GitHub, autocompletes and generates code based on developers' prompts (Dohmke, 2022[16]). Other models to generate code include CodeGen (Nijkamp et al., 2023[17]). Code refactoring (improving OECD ARTIFICIAL INTELLIGENCE PAPERS INITIAL POLICY CONSIDERATIONS FOR GENERATIVE ARTIFICIAL INTELLIGENCE | 13 pre-existing code without altering its functionality) is another area where generative AI is assisting developers (Ingle, 2023[18]). • Creative industries and arts – In music, AI melody generators have been available for a while, helping artists craft new music from scratch or based on previous bars, improve composition (Yang, Chou and Yang, 2017[19]), and process singing (Gómez et al., 2018[20]). In image generation, applications such as Stable Diffusion and Dall-E 2 provide new opportunities to generate artforms for the advertising, media, movie, and other industries. • Education – Education is among the sectors expecting change in the near-term, as school children and students experiment with generative AI applications to learn (like OpenAI’s GPT Khanmigo 9) and prepare for exams (Baidoo-Anu and Ansah, 2023[21]). Such applications can create educational material, write letters of recommendation, and design course syllabuses, improving the efficiency of teachers (Pettinato Oltz, 2023[22]). • Healthcare – Generative AI models play important roles as interfaces for patients and healthcare providers (Bommasani et al., 2021[23]). Patients are already benefiting from information on preventive care (Demner-Fushman, Mrabet and Abacha, 2020[24]) and explanations of medical conditions and treatments. The use of Vik, a chatbot that responds to the fears and concerns of patients diagnosed with breast cancer, is shown to result in better medication adherence rates (Chaix et al., 2019[25]). Another promising application is the discovery and development of new drugs using generative AI chemistry models. Companies such as Insilico Medicine are conducting FDA-approved clinical trials of cancer treatments designed using large biological, chemical, and textual generative and predictive engines (Insilico Medicine, 2023[26]). 10 • Search – Search-engines are underpinning their search capabilities with conversational generative AI models such as Microsoft Bing with OpenAI’s GPT-4. 11 One of the most discussed topics in AI and search is whether search engines that provide links to users will be disrupted by conversational agents that provide better search experiences (Sriram and Mehta, 2023[27]). Generative AI considerably amplifies mis- and disinformation’s scale and scope Humans were found, already in 2022, to be almost incapable of differentiating AI from human generated news in 50% of cases (Kreps, Mccain and Brundage., 2022[28]), meaning that generative AI can amplify risks both of misinformation (the unintended spread of false information) and of deliberate disinformation by malicious actors. 12 Leading-edge generative AI models have multimodal capabilities that can exacerbate these risks, for example by combining text with image or video or even voices. Unintentional misinformation or intentional deception can cause material harm at an individual level (e.g., influencing decision-making about vaccines) (Weidinger et al., 2022[29]) and, on a larger scale, erode societal trust in the information ecosystem (Ognyanova et al., 2020[30]) and the fact-based exchange of information that underpins science, evidence-based decision-making, and democracy (OECD, 2022[31]). Research findings related to human interpretation of AI-generated content underscore the potential risk of AI-driven mis- and disinformation, and emphasise the importance of disclosing the use of AI systems (Box 2.1). OECD ARTIFICIAL INTELLIGENCE PAPERS 14 | INITIAL POLICY CONSIDERATIONS FOR GENERATIVE ARTIFICIAL INTELLIGENCE Box 2.1. Selected research findings on human interpretations of synthetic content Humans trust content less when AI authorship is disclosed Research to date finds that humans perceive AI-generated news to be less accurate than human writing and that they trust news less when AI authorship is disclosed. This finding might apply to other domains where generative AI is used to produce text, such as social media posts or communications by companies and governments. Humans find synthetic faces more trustworthy than real faces Although AI-generated faces are nearly indistinguishable from real ones, humans perceive synthetic faces to be more trustworthy than real faces. This has been explained by the fact that synthetic images resemble “average” faces, which are perceived to be more trustworthy. Sources (Longoni et al., 2022[32]); (Nightingale and Farid., 2022[6]); (Sofer et al., 2015[33]). The functionality of text-to-text generative AI models, or language models, easily leads them to produce misinformation. They are trained to predict words or statements based on a probability assessment. However, the accuracy of the predicted following word depends on the context rather than probability (Weidinger et al., 2022[34]). Truth depends on context, but LLMs based on probabilistic inference have no ability to reason and thus might never achieve completely accurate outputs (LeCun, 2022[35]). This also bears on LLMs’ potential as a tool to detect information for the purpose of countering it (Weidinger et al., 2022[34]). “Hallucinations” and over-reliance also require addressing Another worrying feature of LLMs is their propensity to “hallucinate” (i.e., to generate incorrect yet convincing outputs), particularly when an answer is not available in the training data (OECD, 2023[1]). This can allow them to create convincing misinformation, hate speech, or reproduce biases. Risks also include excessive trust and overreliance on the model, resulting in a dependency that can interfere with developing skills, and even lead to losing skills (OpenAI, 2023[36]). 13 This issue will worsen with increasing model capabilities, areas of application, and user trust as average users will be unable to fact-check the models’ responses (Passi and Vorvoreanu, 2022[37]). Synthetic content can be particularly powerful in politics, science, and law enforcement Risks associated with text-to-image generative AI models make clear how rapid technological progress is. Numerous “photographs” on Twitter and other online platforms depicted well-known political figures and heads of state taking surprising actions yet were very credible, demonstrating the power of synthetic imagery, particularly in polarised political contexts. Another issue has been the manipulation of scientific images to produce mis- and disinformation, threatening trust within research communities as well as science’s reputation with the general public. Use of synthetic images by climate change deniers (Galaz et al., 2023[38]) and the spread of COVID-19 disinformation (Coldewey and Lardinois, 2023[39]) serve as cases in point. Targeted disinformation campaigns leveraging different modes of generative AI can mislead and manipulate public opinion (Weidinger et al., 2022[29]) (OECD, 2022[31]). LLMs could help conduct targetedinfluence operations, i.e., “covert or deceptive efforts to influence the opinions of a target audience” (Goldstein et al., 2023[40]). They can significantly reduce propaganda costs and increase its scale (Buchanan et al., 2021[41]). The dynamics of influence operations could also be altered in unpredictable OECD ARTIFICIAL INTELLIGENCE PAPERS INITIAL POLICY CONSIDERATIONS FOR GENERATIVE ARTIFICIAL INTELLIGENCE | 15 ways, such through more convincing messaging by using LLMs capable of better cultural and linguistic immersion in target audiences (Goldstein et al., 2023[40]). OpenAI reported that its own red teaming efforts found GPT-4 to rival human propagandists, “especially if teamed with a human editor”, and can develop plausible-sounding plans to reach propaganda goals (OpenAI, 2023[36]). Despite users’ wariness of AI authorship (Box 2.1), research in 2019 found AI-generated fake news to be more credible to human raters than human-written disinformation (Zellers et al., 2019[42]). In the early weeks of the Russian invasion of Ukraine in March 2022, a deepfake video depicting Ukrainian President Volodymyr Zelensky admitting defeat and demanding Ukrainian soldiers surrender surfaced on social media and was uploaded to a Ukrainian news website by hackers (Allynn, 2022[43]). Although crude in video and audio quality, this attempt to deceive the public was seen as a harbinger of future public deception enabled by more powerful models (Boháček and Farid, 2022[44]). Such deepfakes are increasingly realistic and convincing as advancements are made in both audio and video generation techniques and models. Mitigating mis- and disinformation requires leveraging and improving known solutions The risks of generating mis- and disinformation at scale with generative AI systems demand novel solutions. Companies and other organisations have faced issues related to incorrect or false information for a long time and put systems in place to address them. However, traditional fact-checking and other existing solutions are generally not scalable in the face of AI-based automation of disinformation. User education alone becomes insufficient when AI generates more and more convincing disinformation. In addition, it shifts responsibility from systems, companies, and governments to individuals. While researchers are exploring potential paths forward, there are still more questions than answers about potential remedies to AI-generated and spread mis- and disinformation. Weidinger et al. (2022) point to several methods for reducing misinformation at scale. Scaling-up (i.e., increasing) model size is often advocated to improve model accuracy but deemed insufficient (Bender and Koller, 2020[45]) (Lin, Hilton and Evans, 2022[46]). Research is also ongoing to prompt models to substantiate their statements, such as by referencing sources from the Internet (Nakano et al., 2021[47]) or forcing them to provide evidence to support their claims (Menick et al., 2022[48]), and augmenting retrieval model architecture by having models retrieve information from larger databases to make predictions (Borgeaud et al., 2021[49]). To mitigate image-generating AI misinformation risks, some suggest adding watermarks that enable identification of synthetic imagery, restricting code so that it cannot easily be introduced into applications, and developing guidelines that include ethical guidelines for the production and distribution of AI-generated images (Nightingale and Farid., 2022[6]). Some research also suggests that watermarking model output could be possible for text generation as well (Kirchenbauer et al., 2023[50]) (Abdelnabi and Fritz, 2021[51]). The Coalition for Content Provenance and Authenticity (C2PA) seems to be coordinating promising collaboration among relevant actors to mitigate misinformation risks (Box 2.2). OECD ARTIFICIAL INTELLIGENCE PAPERS 16 | INITIAL POLICY CONSIDERATIONS FOR GENERATIVE ARTIFICIAL INTELLIGENCE Box 2.2. Coalition for Content Provenance and Authenticity (C2PA) C2PA is a consortium which develops open standards that certify the source and provenance of online content. Its steering committee consists of major IT and media companies. Since 2021, C2PA has delivered several versions of its technical standards for content provenance and authenticity. These standards serve as a model for storing and accessing cryptographically verifiable information whose trustworthiness can be assessed based on a defined trust model. The aim is to enable the global optin adoption of digital provenance techniques by creating an ecosystem of digital provenance-enabled applications for individuals and organisations while meeting appropriate security and privacy requirements and human rights considerations. Source: https://c2pa.org. The misinformation mitigation elements discussed above can also help mitigate disinformation, though additional actions, such as use-limits and monitoring, may be needed to address intentional AI generation and spreading of false information. Research labs use red-teaming to test a model and try to deny user requests that could lead their model to generate disinformation (OpenAI, 2023[36]) (Ganguli et al., 2022[52]). This can be done with human testing and/or with other generative models (OECD, 2023[1]). A growing body of technical literature documents varying deepfake detection techniques, an addition to the watermarking efforts touched on above. Fake-news and deepfake detection use different methods that range from plotting LLMs against LLMs (Zellers et al., 2019[42]) to augmenting human deepfake video raters with stateof-the-art deepfake detection systems – found to be more accurate than having either humans or the detection systems rate content alone (Groh et al., 2022[53]). Novel solutions to address mis- and disinformation from generative AI are also imperative Current approaches have limitations. The OECD.AI Network of Experts has, in particular, discussed that: • While it may be possible to develop mechanisms that detect subtle traces of origin in AI-generated images, this is not always true of AI-generated text. In particular, short texts such as social media posts or product reviews do not contain enough data to reliably distinguish human and machinegenerated content. Human editing of AI-generated text can further obscure its origins (Sadasivan et al., 2023[54]), though this might not be possible at scale. • As with other technologies, bad actors will seek to circumvent mitigation measures. These statesponsored or commercial actors will not declare their bots or disinformation as AI-generated or follow guidelines or codes of conduct. Obligations to do so will not stop them, just as the illegality of cyberattacks does not prevent cyberattacks. This is exacerbated by the global nature of the internet that enables such actors to take refuge in “safe” jurisdictions. • Although most large generative AI models are controlled by large companies, open-source models are increasingly available, some of which can be queried by any user from any computer (OECD, 2023[1]). This effectively bypasses the potential guardrails and restrictions on use. However, some OECD.AI experts note that using open-source models still requires significant expertise and capacity and that bypassing guardrails might not be trivial when the models are fine-tuned with built-in mitigations. Overall, research finds that detection algorithms for video, audio/voice, and images are unreliable. A major reason for this is that attackers can generate new deepfakes that detection models are not yet familiar with (Le et al., 2023[55]). In the case of text, detection algorithms can be evaded by adding another model that OECD ARTIFICIAL INTELLIGENCE PAPERS INITIAL POLICY CONSIDERATIONS FOR GENERATIVE ARTIFICIAL INTELLIGENCE | 17 rephrases the generative AI output text, which is known as a “paraphrasing” attack (Sadasivan et al., 2023[56]). To complicate matters, the watermarking schemes of distinct language models can themselves be learned and applied to detect text as watermarked in “spoofing attacks”, such that companies or developers behind the targeted LLM could be falsely accused of generating plagiarised text, spam, or fake news (Sadasivan et al., 2023[56]). Since defensive and offensive techniques are in constant competition, new research is trying to increase systems’ robustness against such attacks (Krishna et al., 2023[57]). Bias and discrimination Generative AI models can replicate biases present in their training data Generative AI can echo, automate, and perpetuate social prejudices, stereotypes, and discrimination by absorbing biases contained in resources used as training data. In the case of language models, these are language resources or language models themselves, including pre-trained models (OECD, 2023[1]). These outputs could further marginalise or exclude specific groups (Bender et al., 2021[58]). Examples include models that display negative sentiment towards social groups, link occupations to gender (Weidinger et al., 2022[34]), or express bias regarding specific religions (Abid, Farooqi and Zou, 2021[59]). Some models also try to evade responding when asked potentially biased questions such as whether women should be allowed to vote, using so-called “hedging” behaviour – which may increase users’ trust in the model because it seems to display caution (OpenAI, 2023[36]). Bias is not limited to text-based systems and extends to other types of models such as image models. For example, synthetic image outputs were found to over-represent white skin colour and masculinity in three different image-generating models (Luccioni et al., 2023[60]), with another model ranking synthetic images of females in more domestic and household environments than their male counterparts (Lucy and Bamman, 2021[61]). Other research points to biased relationships between race, gender, and economic status in image-generating systems’ outputs (Fraser, Kiritchenko and Nejadgholi, 2023[62]). 14 While biasreinforcement is an ongoing issue with machine-learning, the ease-of-use and rapid adoption of recent generative AI systems risks increasing the dissemination of discriminatory outputs. Documentation, data curation, and auditing are basic mechanisms to mitigate bias Measures to mitigate bias include taking stock of training data for represented and missing groups and narratives (Dodge et al., 2021[63]) (Gebru et al., 2018[64]), curation or semi-automatic curation of datasets to reach fairer results (Denton et al., 2020[65]) (Hutchinson et al., 2021[66]), explainability and interpretability research (Weidinger et al., 2022[34]), and applying auditing processes (Zakrzewski, 2023[67]). Red teaming and reinforcement learning by human feedback are key for commercial models Research labs that market their models often use more forceful approaches. These include “red teaming”, in which teams adopt an attacker mindset to probe the model for flaws and vulnerabilities. 15 They either rely on human experts or use language models (Perez et al., 2022[68]). Other approaches include combinations of dataset cleaning – such as classifiers to filter out erotic content – and “Reinforcement Learning by Human Feedback” (RLHF) algorithms (Markov et al., 2022[69]). RLHF, as illustrated in Figure 2.1, is a multi-step, fine-tuning approach to shape a model’s behaviour, such as getting it to respond less to disallowed content or refuse to give instructions on how to harm oneself (OpenAI, 2023[36]). While important, these strategies cannot guarantee a model’s safety. Numerous cases of ”jailbreaks” intentionally exploiting models to get them to respond inappropriately have been documented (Figure 2.2). 16 In addition, some research points out that RLHF might have limitations in terms of scalability, cost and quality of human OECD ARTIFICIAL INTELLIGENCE PAPERS 18 | INITIAL POLICY CONSIDERATIONS FOR GENERATIVE ARTIFICIAL INTELLIGENCE feedback (Christiano, 2023[70]) (Lambert et al., 2022[71]), or the potential to introduce new biases from the humans providing feedback (Shah, 2023[72]). Figure 2.1. ChatGPT explains RLHF as part of an overview by HuggingFace Source: https://huggingface.co/blog/rlhf. Figure 2.2. Cases of “jailbreaks” Source (OpenAI, 2023[36]). OECD ARTIFICIAL INTELLIGENCE PAPERS INITIAL POLICY CONSIDERATIONS FOR GENERATIVE ARTIFICIAL INTELLIGENCE | 19 Intellectual Property Rights (IPR) issues, including copyright Generative AI raises intellectual property rights issues, particularly concerning unlicensed content in training data, potential copyright, patent and trademark infringement of AI creations, and ownership of AIgenerated works. Generative AI models are trained on data that includes unauthorised copyrighted material Generative AI models are being trained on massive amounts of data that includes copyrighted data, mostly without authorisation of the rights-owners. In 2019, the World Intellectual Property Organisation (WIPO) convened sessions about the implications of AI for IP. The WIPO Secretariat published a paper in May 2020 on IP policy and AI that highlighted eight key issues, including questions such as whether the use of copyrighted data without authorisation constitutes an infringement of copyright and, if so, whether there should be an exception that allows for the training of machine learning models (WIPO, 2020[73]). Legal cases on the applicability of fair use principles versus copyright infringement are ongoing Whether commercial entities can legally train ML models on copyrighted material is contested in Europe and the US. In the US, the outcome could be determined by the applicability of the fair use principle, which limits the exclusive rights of copyright owners (House of Representatives, 1976[74]). Fair use requires courts to weigh four statutory factors and, if ruled applicable, could result in non-infringement, allowing commercial entities to use copyrighted material in their training sets (Lorenz, 2022[75]) (Zirpoli, 2023[76]). 17 Several lawsuits were filed in the US against companies that allegedly trained their models on copyrighted data without authorisation to make and later store copies of the resulting images (Zirpoli, 2023[76]). 18 These decisions will set legal precedents and impact the generative AI industry from start-ups to multinational tech companies. They will also affect policy in areas beyond IP, such as research and development (R&D) and industrial policy, the geopolitics of technology, foreign affairs, and national security (see section on AI futures). Recent research could also demonstrate instances in which the fair-use doctrine might not apply – cases in which a foundation model generates outputs that are very similar to copyrighted data (Henderson et al., 2023[77]). This work suggests that model development might need to ensure that outputs remain sufficiently different from copyrighted material to remain covered by fair use. Can novel outputs generated by AI be copyrighted or patented and if so, by whom? Generative AI creates new images, text, and audio that are novel, raising questions about whether generated outputs can be copyrighted or patented. Because legal systems around the world differ in their treatment of IP rights such as patents and copyrights, the treatment of AI-generated works varies between countries (Murray, 2023[78]). 19 To date, most jurisdictions agree that works generated autonomously by AI are not copyrightable (Craig, 2021[79]). US copyright law requires human authorship to register a copyright claim and copyrights cannot be awarded to generative AI systems. European legal systems have come to a similar interpretation of the matter, although the decisive requirement is originality, which according to the European Court of Justice (ECJ) is fulfilled if the work reflects “the author’s own intellectual creation” (Infopaq International (C-5/08), 2009[80]); (Deltorn and Macrez, 2018[81]). If generative AI systems cannot be awarded copyrights, the work could be assigned to somebody else, such as the system programmer. Jurisdictions in UK Commonwealth tradition allude to computergenerated works and attribute authorship to the person laying the groundwork for the machine’s creation (Deltorn and Macrez, 2018[81]); (Craig, 2021[79]); (Murray, 2023[78]) 20 The rapid spread of generative AI OECD ARTIFICIAL INTELLIGENCE PAPERS 20 | INITIAL POLICY CONSIDERATIONS FOR GENERATIVE ARTIFICIAL INTELLIGENCE models, the amounts of venture capital they attract, and the growing numbers of applications claiming copyrights for AI-generated works recently led the US Copyright Office to issue a policy statement on its approach to registration, confirming that it will not register works produced by a machine (U.S. Copyright Office, 2023[82]) and launching a website for updates on this topic. 21 Generative AI could impact labour markets on a different scale and scope Labour markets could face a significant shakeup with both positive and negative effects While to date, AI has mainly impacted the quality of jobs rather than their quantity (Box 2.3), there are signals that labour markets could soon face a significant shakeup with both positive and negative effects. Technological progress, falling costs, and increasing availability of workers with AI skills indicate that OECD economies could be on the brink of an AI revolution (OECD, 2023[83]). Advances in generative AI have heightened focus on the potential impact of AI on labour markets. In addition to language models, modes such as image, audio, and video generation are receiving increased attention. Multimodal capabilities combining text and image generation, such as those of GPT-4 released by OpenAI in March 2023, could further broaden the range of actions which AI systems perform, and thus their potential labour market impacts. Box 2.3. AI in the 2023 edition of the OECD Employment Outlook The 2023 edition of the OECD Employment Outlook, the OECD’s flagship publication on labour market developments in OECD countries, includes analysis of the impact of AI on the labour market and of policy measures to benefit from AI in the workplace while addressing its risks. The Outlook finds that the net impact of AI in general on employment to be ambiguous. While AI displaces some human labour (displacement effect), the greater productivity it brings (productivity effect) could increase labour demand. AI can also create new tasks, resulting in the creation of new jobs for which human labour has a comparative advantage (reinstatement effect), particularly for workers with skills complementary to AI. To date, AI has mainly impacted the quality of jobs – generally, in positive ways. For example, worker well-being and satisfaction increased through the reduction of tedious or dangerous tasks. However, some risks, such as increased work intensity and stress, are materialising. There are also risks to privacy and fairness. Workers in finance and manufacturing whose employers uses AI worry about their privacy and these risks tend to be greater for socio-demographic groups already disadvantaged in the labour market. While the Employment Outlook found little evidence of significant negative effects from AI on the quantity of jobs, this research mostly predates the latest public release of generative AI applications. Negative employment effects of AI might take time to materialise: AI adoption is still relatively low and/or firms might prefer to rely on voluntary workforce adjustments i.e., attrition. Source: (OECD, 2023[83]). Language models perform increasingly well on standard aptitude tests Measures of AI exposure evaluate the overlap between tasks performed in a job and those AI could theoretically do. Those examined in the Employment Outlook show that AI had advanced in performing non-routine cognitive tasks such as information-ordering, memorisation, and perceptual speed even before OECD ARTIFICIAL INTELLIGENCE PAPERS INITIAL POLICY CONSIDERATIONS FOR GENERATIVE ARTIFICIAL INTELLIGENCE | 21 recent advances in generative AI applications (OECD, 2023[83]). AI tools can already answer 80% of the literacy questions and two-thirds of the numeracy questions in the OECD Survey of Adult Skills of the Programme for International Assessment of Adult Competencies (PIAAC). Experts believe AI will be able to solve the entire PIAAC literacy and numeracy tests by 2026. The strong performance of GPT-3.5 and GPT-4 on the Bar Exam (used by US jurisdictions to qualify lawyers) and other standard tests surprised many (Figure 2.3). 22 Figure 2.3. GPT performance on academic and professional exams Source: https://openai.com/research/gpt-4. Generative AI could impact higher-skilled jobs The occupational range and extent of AI exposure might rapidly become larger as generative AI use is increasingly incorporated (OECD, 2023[83]) in jobs such as legal research, technical support and fixing computer bugs, or customer service. High-skilled occupations have been most exposed to recent advances in AI, including business professionals; managers; science and engineering professionals; and legal, social and cultural professionals (OECD, 2023[83]). Nevertheless, low-skilled workers are still the most exposed to the risk of automation, at least for the time being. While the literature on labour-market effects caused by generative AI is recent and not necessarily peer reviewed yet, research by OpenAI suggests that generative AI systems could further expose higher-income OECD ARTIFICIAL INTELLIGENCE PAPERS 22 | INITIAL POLICY CONSIDERATIONS FOR GENERATIVE ARTIFICIAL INTELLIGENCE jobs to automation (Eloundou et al., 2023[84]), with the impact on task-exposure potentially over twice as large as that of other powerful deep-learning algorithms. Similarly, researchers examining the capabilities of large language models (LLMs) found greater exposure for industries that recruit employees with higher education (Felten, Raj and Seamans, 2023[85]). They found that the sectors most affected are legal services, securities, commodities, and investment. Professions based on writing and coding would be more exposed to the risk of displacement from LLMs than those that rely on science or critical thinking (Eloundou et al., 2023[84]). Language models may benefit lower skilled workers comparatively more Research by the Massachusetts Institute of Technology found that ChatGPT significantly reduces the time people spend conducting tasks while improving output quality (Noy et al., 2023[86]). It also showed the tool to have a greater impact on the productivity of workers with lower aptitude, such as junior employees, allowing them to catch up with their more senior colleagues and reducing inequality in the workplace. ChatGPT was not found to improve workers’ skill levels but to reduce the effort needed. Similarly, other research has found that AI tools could increase low-skilled workers’ productivity by an estimated average of 14 percentage points, as opposed to high-skilled workers’ productivity, which would generally remain unaffected (Brynjolfsson, Li and Raymond, 2023[87]). The findings in these sources are generally based on limited experiments and thus should not be overly generalised. Similarly, coding assistants like GitHub’s Copilot decrease the time spent by software developers on a specific test task by over 50%. Copilot provides snippets and allows for autocompleting code. In an experiment, this reduced developers’ time spent implementing an HTTP server in JavaScript by 55.8% over a control group without access to the coding assistant (Peng et al., 2023[88]). The increased efficiency can increase job satisfaction (Noy et al., 2023[86]) or, in the case of Copilot, potentially lower entry barriers for roles in software development (Peng et al., 2023[88]). A significant proportion of occupations could be impacted The OECD finds that occupations at the highest risk of automation from AI account for about 27% of employment and that a significant share of workers (three in five) worry about losing their jobs entirely to AI in the next ten years – particularly those who already work with AI (OECD, 2023[83]). The advent of the latest generative AI technologies is sure to heighten automation concerns across a wide range of job categories. Research on language-based generative AI finds that 32.8 percent of jobs in the International Standard Classification of Occupations (ISCO) could be impacted on a full scale, 36.5 percent could be partially impacted, and only 30.7 percent would not be affected by generative AI models (Zarifhonarvar, 2023[89]). This puts pressure organisations to adapt to generative AI and support their workforces, and on policymakers to steer labour market developments and transitions. Policy is needed to reap labour market benefits and address risks and unknowns As emphasised in the OECD Employment Outlook (OECD, 2023[83]), the benefits and risks of AI in the workplace, coupled with its rapid pace of development and deployment, underscore the need for decisive policy action to reap the benefits it offers and address the risks for workers’ rights and well-being. There is a need to enable both employers and workers to reap the benefits of AI while adapting to it, notably through training and social dialogue. The adoption of AI on tasks and jobs will change skill needs. In the OECD AI Surveys of Employers and Workers, many companies using AI say they provide training for AI, but that lack of skills remains a major barrier to adoption (OECD, 2023[83]). Companies also report that AI has increased the importance of human skills even more than that of specialised AI skills. Countries have taken some action to prepare their OECD ARTIFICIAL INTELLIGENCE PAPERS INITIAL POLICY CONSIDERATIONS FOR GENERATIVE ARTIFICIAL INTELLIGENCE | 23 workforce for AI-induced job changes, especially through skilling efforts, but initiatives remain limited in scale (OECD, 2023[83]). Less is known about training efforts focused on generative AI, though its needs could overlap with AI more broadly. OECD work also shows that labour market outcomes are better when the adoption of technologies is discussed with workers (OECD, 2023[83]). Organisational change strategies are needed, including building awareness of what is needed to bridge emerging skills gaps (transversal skills), improve current skills (re-skilling), and develop new ones (upskilling), while encouraging openness towards AI technologies and working to prevent anxiety around misperceptions (Morandini et al., 2023[90]). At the same time, there is an urgent need for policy action to address the risks that AI can pose when used in the workplace – in terms of privacy, safety, fairness and labo