Winds of Change: Voter Blame and Storm Gudrun in the 2006 Swedish Parliamentary Election PDF
Document Details
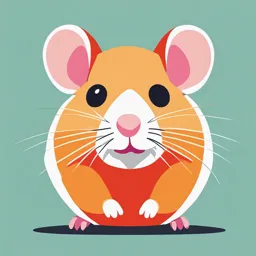
Uploaded by InstrumentalBluebell
Uppsala University
2016
Lina M. Eriksson
Tags
Summary
This article analyzes the impact of the 2005 Storm Gudrun on voter behavior in the 2006 Swedish parliamentary election. The study uses a Difference-in-Differences design to examine if the storm caused long-term shifts in party support. It is found that there was a notable shift among long-standing voters from left to right, notably impacting the incumbent Social Democratic Party, and increasing support for the main opposition party.
Full Transcript
Electoral Studies 41 (2016) 129e142 Contents lists available at ScienceDirect Electoral Studies journal homepage: www.elsevier.com/locate/electstud Winds of Change: Voter Blame and Storm Gudrun in the 2006 Swedish Parliamentary Election Lina M. Eriksson a, b, c, * a Department of Government, UPPSALA...
Electoral Studies 41 (2016) 129e142 Contents lists available at ScienceDirect Electoral Studies journal homepage: www.elsevier.com/locate/electstud Winds of Change: Voter Blame and Storm Gudrun in the 2006 Swedish Parliamentary Election Lina M. Eriksson a, b, c, * a Department of Government, UPPSALA University, Box 514, 751 20, Uppsala, Sweden €gen 16, SE-752 36, Uppsala, Sweden Center for Natural Disaster Science (CNDS), c/o Department of Earth Sciences, Uppsala University, Villava c The Europe Center (TEC), Freeman Spogli Institute for International Studies, Stanford University, Encina Hall, Stanford, CA, 94305-6165, USA b a r t i c l e i n f o a b s t r a c t Article history: Received 14 February 2015 Received in revised form 18 November 2015 Accepted 15 December 2015 Available online 19 December 2015 Can a natural disaster shift long-standing party support for the long-term? Studies of political behavior indicate that, as elections approach, voters punish or credit governments based on their responses to severe weather phenomena. It may still be considered an open question, however, if poor crisis response could trigger more durable shifts in long-standing party support. I provide empirical evidence suggesting that it could. I exploit a crucial case for the study of change in party support, Storm Gudrun (Erwin), to examine long lasting punishment effects over crisis response. The estimated effect is of a magnitude that equals the largest block-transfer of voters in Swedish history and can be seen over three parliamentary elections (2006, 2010 and 2014). © 2015 Elsevier Ltd. All rights reserved. Keywords: Retrospective voting Natural disasters Accountability Party support Regime shift Crisis response 1. Introduction Literature on vote choice has established party-identification as the most reliable predictor in national elections in the United States (Campbell et al., 1960) and other countries (Schickler and Green, 1997). With the exceptions of the Great Depression and the twoparty competition in the South of the United States following the Voting Rights Act of 1965, partisanship has also been found remarkably stable and resilient to dramatic political events, including wars, recessions and scandals (Green et al., 2002: 2-6). The enduring stability of party-identification thus suggests that changes in long-standing party support may have little to do with political evaluation. In contrast, this study relates long-term shifts in long-standing party-support to political evaluation through electoral accountability. Accountability is essential to democracy. Theoretically, it is the means by which the principal (an electoral majority) may counteract agency loss (which occurs when the elected incumbent (agent) does not represent the interest of the principal) by * Department of Government, UPPSALA University, Box 514, 751 20 Uppsala, Sweden. E-mail address: [email protected]. http://dx.doi.org/10.1016/j.electstud.2015.12.003 0261-3794/© 2015 Elsevier Ltd. All rights reserved. motivating representative political action (Strom, 2003). Agency loss assumes divergence in interests between principal and agent as well as asymmetric information (information is not completely shared between principal and agent). This may either lead to adverse selection (when the principal selects the wrong agent), or moral hazard (when the selected agent takes unobservable action against the interest of the principal). Accountability is thus the means by which the principal may try to counteract such misbehavior by motivating political action that is representative of a majority (Strom, 2003:55, 61e63, 89, 96). In general, though not being mutually exclusive options, there are three ways for accountability to come about: from the bottom up (by initiative of the principal), horizontally (by institutional design) or vertically (by retrospective voting). This paper mainly concerns the latter, which opens up for the possibility of political change by electoral sanctions (Manin et al., 1999). In this study, I examine the potentially lengthy implications of the retrospective voting mechanism among long-standing party supporters. Specifically, I investigate the possibility of sudden, substantial and long-term changes in party support expressed by the ballot as a movement from one side the political party spectrum to the other. This should not be confused with the movements of swing-voters. Rather, this study should be understood as a general examination into the possibility of profound changes in party 130 L.M. Eriksson / Electoral Studies 41 (2016) 129e142 Fig. 1. Vote Support for the S Party by Unaffected vs. Affected Municipalities. Note: Fig. 1 shows the trend in voter support for the 2002 government (S) by non-affected vs. affected municipalities from 1973 e 2014. Out of a total of 290 municipalities, the affected municipalities exhibited by the lower line are 208 with a wind speed of at least 22.5 m/s (81 kph/50 mph). The deviation in parallel trends in the affected group of municipalities between 2002 and 2006 constitutes the treatment effect on the 2006 election outcome. The counterfactual trend is the continuation in voter support for S should Gudrun not have happened, or should the crisis response have been more in line with the party-supporters’ expectations, ceteris paribus. Similarly, the shaded area between the counterfactual trend and the de facto trend, between 2006 and 2014, illustrates the long-term effects of Gudrun on party support for S over the 2010 and 2014 elections. support, with noticeable effects of at least a decade among a previously long-standing and historically stable vote-base. That is, broadly speaking, I examine under what circumstances we may expect to find the possibility of profound changes in party support. This is done by means of the retrospective voting mechanism, which I use to identify a historic shift among long-standing party supporters of the left to a long-term support for the right, triggered by the exogenous treatment of a natural disaster causing considerable destruction. To explore the possibility of profound changes in party support, I make use of a crucial case where party support shifted from the left to the right in the wake of a natural disaster. I explore retrospective voter evaluation of the Swedish incumbent Social Democratic Party's (S) poor crisis management and policy response (crisis response1) to the 2005 Storm Gudrun. The Swedish context is ideal for this kind of analysis, as S has had a historically long-standing, and remarkably stable, base of party-supporters (see Fig. 1). In so doing, this study offers a novel take on the analysis of changes in party support by connecting to an offshoot from the extant electoral accountability literature, which exploits the methodological benefits of exogenous weather phenomena in testing theoretical aspects of retrospective voting. While the initial study on retrospective voting and natural disasters found no evidence for retrospection (Abney and Hill, 1966), nearly four decades later it was followed by those who argue that voters are irrational for punishing politicians over events beyond their control (Achen and Bartles, 2004). More recent studies suggest voter myopia (Healy and Malhotra, 2009; Cole et al., 2012), as well as strategic disaster relief spending (Besley and Burgess, 2002; Reeves, 2011). While others find no evidence for such strategic spending (Chen, 2012). However, when accompanied with skilled crisis management, disaster spending has been shown to yield 1 Here crisis management may be considered the improvised management of the unexpected, or unpredictable, disaster while policy response may be considered pre-established guidelines and structures in place for handling such a crisis, as well as minimizing the risk of its occurrence and impact. Crisis response refers to both concepts. gratitude over two elections (Bechtel and Hainmuller, 2011). Similarly, Hurricane Andrew has been suggested to have favored Florida state and local political incumbents (Twigg 2012) alongside findings pointing to voter competence, even to varying levels of government responsibility (Gasper and Reeves, 2011; Sinclair et al., 2011; Healy and Malhotra, 2010; Malhotra and Kuo, 2008, 2009; Jacobsson, 2007; Arceneaux and Stein, 2006), even when racial group interests are predominant (Lay, 2009). Finally, most recent evidence suggests that democratic leadership survival is rather immune to natural disasters per se but not to the number of fatalities they cause (Flores and Smith, 2013). While this accumulated evidence for retrospective voting as a de facto accountability mechanism yield positive news, from a democratic perspective, there is yet room for additional nuanced questions. In what follows I examine if a natural disaster also can change party support by exploiting what hitherto has been an unrecognized, yet crucial, case for retrospection, the 2005 Storm Gudrun in the 2006, 2010 and 2014 Swedish parliamentary elections. Specifically, I take advantage of the historically stable votebase of S-supporters displayed in Fig. 1, which shows how stormaffected and unaffected municipalities are strikingly similar in terms of over-time trends in party support prior to Gudrun. To examine if the storm may explain the drop in S-support that follows Gudrun in the storm affected municipalities, as well as its potential durability, I use a Difference-in-Differences (DiD) design in an analysis consisting of 288 municipalities. With an economic cost of 1% in relation to GDP (which equals that of Hurricane Katrina2), Gudrun is to be considered the most costly natural disaster in Swedish history. The crisis response of the S-incumbent was perceived as poor, since it provided no money transfers to affected individuals. My most conservative estimate indicates that S experienced an average drop in vote support of about 3.8% due to the storm in the 2006 election, which carried over to the 2010 and 2014 elections, making it the most durable punishment effect thus far connected to a natural disaster event. Not only does this duration suggest that voters in affected municipalities strongly punished S for its insufficient response, but rather that they profoundly changed their party support for a long time to come. Moreover, and quite remarkably, as the retrospective effects also correlates with an increased vote support for the main opposition of the conservative Moderate Party (M) in the 2006 election, with residual effects in the 2010 and 2014 elections,3 the results also suggest a remarkable shift in ideology. In fact, I also find the same long-term trend of a shift from S to M in the 2006, 2010 and 2014 municipal elections.4 This is particularly interesting, as the gain in M-votes corresponds to a drop in the 2002 S-vote base for the 2006 parliamentary election of about 9.3%. In magnitude this equals the largest block-transfer in Swedish history of 8%,5 identified by previous research as the most important factor determining the 2006 election outcome (Oscarsson and Holmberg, 2009:7, 14e15, 21). My contribution is three-fold. First, I contribute to the literature 2 See Section 3.2 in this paper for details on the estimated effects. OLS regressions of the effect of Gudrun on the M party are available in the Supplementary material to this paper (see section IV: Additional Robustness Checks, Tables 3 and 4). 4 Swedish municipal elections take place on the same day as the parliamentary elections. OLS regressions of the long-term effects of Gudrun on the voter support for S and M in the municipal elections are found in the Supplementary material to this paper (see section: VI: Additional Corroboration of Main Findings and Robustness Checks, Tables 6, 7, 8 and 9). 5 For the substantial effects of Gudrun on the M party, see the Supplementary material (section IV: Additional Robustness Checks, Tables 3 and 4), which confirms an average gain of about 9.3% points of parliamentary votes in storm-affected areas. 3 L.M. Eriksson / Electoral Studies 41 (2016) 129e142 on retrospection and natural disasters by examining a crucial case, which treats the question of change in party support for exceptionally poor crisis response. Second, I also shed new light on the temporal dynamics of accountability. In particular, Gudrun struck 1 year and 8 months prior to the 2006 election, which provides for an analysis of the so far furthest time dimensions of electoral accountability and natural disasters within the same election cycle. Prior studies have had disasters striking 1 (Bechtel and Hainmuller, 2011) to 2.5 (Chen, 2012) to 5e8 months (Arceneaux and Stein, 2006; Lay, 2009 and Sinclair et al., 2011), or at most up to 1 year and 2 months (Jacobsson, 2007), prior to an election. And by including the 2010 and 2014 elections, I present the so far longest period of retrospective analysis in such a context, which stretches over 9 years and 8 months. Third, I contribute with novel evidence that sheds light on the outcome of the historic 2006 regime-shift in Sweden. The paper is organized as follows. First, I introduce Gudrun as a crucial case and examine the temporal dynamics of the case that can help account for the long time dimensions of voter response to Gudrun. Thereafter, I introduce the contextual setting for the electoral punishment over the storm. I then present the data, research design and methodology, followed by the empirical results and, finally, the article's conclusion. 2. Storm Gudrun: a crucial case for change in party support In relation to previously studied disasters, the time dimensions of Gudrun are unusual as she occurred in the midst of a mandate period (midst) without the mobilization of any direct compensatory government transfers (zero). While previous research has found lengthy voter gratitude over two elections in response to the crisis management of the 2002 Elbe flooding, it held the largest mobilization of disaster relief (high), including direct compensatory government transfers, in German history (Bechtel and Hainmuller, 2011). Moreover, the Elbe flooded only 1 month prior to the 2002 Federal Election, which opened a window of opportunity for the incumbent to make its crisis response an integral part of the widely broadcasted re-election campaign at the closing stages of the election cycle (end). Thus, Gudrun presents the diametrically opposed case to the Elbe disaster, which makes her a crucial case for testing the potential lengthy implications of the retrospective voting mechanism, which we would expect to identify in the form of profound changes in party support, away from S. In particular if the opposition exploited the S-party's poor response to Gudrun to its own advantage. That is, Gudrun, like Elbe, ought to be a high impact case, only with opposite outcomes for the respective incumbents. This is so, as the values of the variables of interest (zeroand-midst vs. high-and-end) are near to polar extremes. Thus, the S-party's poor crisis response to Gudrun along with the intermediate-term aspects of the crisis (zero-and-midst) may be what is needed to generate a pronounced drop in vote support by previous long-standing party supporters in the 2006 election. While also accounting for a possible historical block-transfer of a vote base of about 150,000 voters (Oscarsson and Holmberg, 2008b:314) away from S. This is likely so as the zero-and-midst dynamics allow for sufficient time for the most affected supporters to reassess their party standing. Moreover, if the mechanism at play also constitutes a long lasting change in party support, then we would expect the effect to persist over the 2010 and 2014 elections, as party-support, at least in this case, might be considered a deep-seated characteristic of the voter. Below, I provide a test of the retrospective effects of S-supporters by considering the (zero-and-midst) dynamics of Gudrun on the 2006, 2010 and 2014 parliamentary elections. By estimating these effects, I deliver empirical evidence indicating that poor crisis 131 response to natural disasters, even if occurring 1 year and 8 months prior to an election, indeed may be strongly punished by past partysupporters and have long-term effects consistent with the foregoing reasoning of change in party support. In relation to existing studies, Gudrun thus offers a crucial case for exploring the consistency of lengthy retrospection in natural disaster settings, with implications of a substantial shift in party support away from S with its historically stable vote base. 3. The government's poor crisis response and the 2006 election I now turn to the contextual setting for the electoral punishment over the poor crisis response to the storm. Before Gudrun, S had gained popularity in the 2002 parliamentary election (Fig. 1). After four years in power as a minority government the S Prime Minister, € ran Persson, was more popular than ever. He was considered Go more reliable, knowledgeable, sympathetic and understandable than his M counterpart, Bo Lundgren (Holmberg and Oscarsson, 2004:129). During the 2002 electoral campaign, S managed to strengthen its position despite large cuts in welfare spending by remaining at the center of Swedish politics, which yielded an electoral victory (Holmberg and Oscarsson, 2004: 100, 126, 129, 130, 134). Four years later in September of 2006, however, the newly formed M-led Alliance with the People's Party (FP), the Center Party (C) and the Christian Democrats (KD) won the election (Oscarsson and Holmberg, 2008a). Overall, S lost 4.9% points of its vote shares compared to 2002. The Alliance achieved 48.2% of all votes while S got 34.99%, which together with a left block, consisting of the left party (V) and the environmental party (MP), totaled 46.1%. Thus, the Alliance won with 2.1% points over the leftblock while S lost 8% of its 2002 vote-base to M (Oscarsson and Holmberg, 2008b: 314, 2009:7, 14e15, 21).6 While no study thus far has considered the government's poor crisis response to Gudrun as a variable of potential importance for the 2006 election outcome, Fig. 1 suggests it was important. Fig. 1 exhibits parallel trends in voter support for S by unaffected and storm-affected municipalities prior to 2002. From this figure, it is clear that S has had a remarkably stable vote base prior to Gudrun. The S-party has always been more popular in the north, but between 2002 and 2006 a deviation in voter support occurs in stormaffected municipalities, which continues up until the 2010 and 2014 elections. 3.1. Gudrun Late on Friday, January 7, 2005 a newly formed low-pressure system could be discerned north west of Ireland, which deepened explosively as cold streams of air intersected with the mild and humid atmosphere northwest of the British Islands (SMHI, 2013). On the night of the 8th this phenomenon had turned into Storm Gudrun, hitting the Swedish west coast with unprecedented winds of hurricane force level, reaching up to 40 m/s (144 kph/90 mph). From a meteorological perspective, storms of a similar magnitude are likely to strike only once in a century (MSB, 2013). Fig. 2 (left panel) illustrates affected municipalities in grey (the coding of non-affected vs. affected is explained in the note below the figure), which shows the path of Gudrun, entering from the 6 Opinion polls used in this study show that S was at par with the Alliance a few weeks before the election. Thus, while S seems to have lost in the final race, the party also seems to have been at disadvantage when it started (p.15). Accordingly, Gudrun may have caused S to land at par with M at the beginning of the election campaigns. 132 L.M. Eriksson / Electoral Studies 41 (2016) 129e142 Fig. 2. Unaffected vs. Affected Municipalities and Average Annual Wind Speed 1951e2008. Note: The left panel in Fig. 2 illustrates the borders of Sweden’s 290 municipalities used as units of analysis for retrospective effects in the 2006, 2010 and 2014 parliamentary elections, where those affected from Fig. 1 are tinted in grey. A municipality is coded as affected if it experienced winds between 22.5 m/s (81 kph/50 mph) and 40 m/s (144 kph/90 mph) (ranges in brackets). In total, 208 municipalities were affected with average winds of 31.2 m/s (112 kph/70 mph) whereas the average for non-affected is 14.6 m/s (52 kph/32 mph). Source: Own computation based on data from the Swedish Meteorological and Hydrological Institute (SMHI). The right panel illustrates ranges (minemax) in geostrophic wind-mean over time (1951e2008) for 11 regional triangles, derived from annual averages. The daily wind conditions for the three southern triangles are similar to those in the north, making the winds of Gudrun quite rare. The Swedish average is 9.63 m/s €rring, 2009). The original template-maps are from Statistics Sweden (SCB). (35 kph/22 mph). Source: Own computation based on data on geostrophic wind from SMHI (Wern and Ba (For interpretation of the references to colour in this figure legend, the reader is referred to the web version of this article.) southwest and exiting in the east. The right panel shows normal wind speeds, which are substantially lower than during Gudrun. In sum, seven lives were lost during the night, several hundreds were evacuated, and the central SOS-alarm system received 10 times the volume of calls it was designed to cope with. In the middle of the winter, 730,000 people (about 8% of the population) were at once left without electricity supply for up to 45 days (MSB, 2013; SCB, 2013). Fortunately, this particular winter was unusually mild, with record high temperatures of 11.8 C, or 53.24 F (SMHI, 2013). In less than 24 hrs, Gudrun felled 75 billion m3 (about 2625 billion ft3) of forest and blew out substantial parts of the landline and mobile phone systems, causing disruptions in communication for over a year to come. In the most acute phase, the impossibility to call rescue services caused many to be stranded in isolation. In addition, as many of the evacuees were housed privately, relatives in need of care and help caused loyalty conflicts regarding how much time one could take off from work. Overall, Gudrun revealed significant structural weaknesses in the Swedish emergency response system (Magnusson, 2005, MSB, 2013). Despite the largest mobilization of the Swedish military ever to have occurred in peacetime (Westling, 2006), the response was insufficient to meet public demand for help (MSB, 2013). 3.2. Economic damage In the aftermath of the storm, the economic cost of Gudrun's destruction has been estimated to 21billion SEK (MSB, 2013), or 2.8billion USD (EM-DAT, 2013), which constitutes roughly 1% of the 2004 Swedish GDP (OECD, 2013).7 This places Gudrun at par with Hurricane Katrina, while exceeding the economic cost to GDP of the 2002 Elbe flooding by 100%.8 The vast majority of this estimate represents damage to forest of 15.8billion SEK while the second largest cost of 1.8billion SEK accounts for damage to the electrical grid, followed by.8billion SEK in damage to the farming and agricultural sector (forestry excluded). The remaining 2.6billion SEK encompasses other damage (MSB, 2013). Despite this, however, S took no initiative to announce money transfers, which stands diametrically opposed to the exemplary response of the German government during the 2002 Elbe flooding, delivering compensatory payments after only two days (Bechtel and Hainmuller, 2011:855). 3.3. Citizen mobilization Upon arrival, Gudrun dramatically changed daily life for much of the southern population for a long time to come. This is especially true for numerous forest owners and those exposed to reoccurring power outages in the countryside (Guldåker, 2009). In fact, the poor 7 Estimated costs calculated using EM-DAT reported economic costs in USD and OECD listed GDP (output approach) of previous year to the disaster listed as CPC: USD, current prices, current PPPs, millions. 8 Comparative ratios are calculated using the same sources for economic costs and GDP as for Gudrun and Sweden. L.M. Eriksson / Electoral Studies 41 (2016) 129e142 crisis response to these sectors yielded wide-ranging mobilizations of angry citizens into two interest groups, Elupproret (the Electricity riot, my translation) and Forest owners, as well as extensive and long-term coverage by media of these issues. 3.4. The electricity riot In all, Gudrun blew down 5000 km (about 3125 miles) of electrical cables, sufficient to cover a two-way distance between Stockholm and Madrid, out of which one third had to be rebuilt from scratch (“500 mil,” 2005). In some cases, individuals affected by power outages had expenses of up to 600SEK/day (roughly €stlo € st,” 2005). In 67EUR or 85USD)9 for alternative fuel (“Tro response to this situation, some thousand people formed the organization, Elupproret, which successfully managed to push for €rnberg, 2005a). From changes in legislation in the energy sector (To January 1, 2006 and onwards (“Regeringen,” 2005) suppliers are obliged to guarantee disruption free technology (Karlsson, 2005). However, reimbursements only became mandatory for future storms and not for Gudrun. 3.5. Forest owners On March 31, 2005, some 5000 of the approximately 200,000 affected forest owners (Swedish Forest Agency, 2013) marched for Sergels torg in Stockholm. Armed with chain saws and stormdamaged timber parts they confronted the government. At the scene, the leader of the Alliance's Centre-party, Maud Olofsson, represented the opposition and sided with the angry forest owners € , 2005). In in arguing for the acute need in relief (Nord and Sjo contrast, the minister of agriculture, Ulrica Messing, blamed forest owners for being irresponsible and ignorant of private insurances (“Messing,” 2005). The demonstration resulted in a tax-deduction of 50SEK (roughly 5.5EUR or 7.1USD)10 per m3 (about 35 feet3) of timber, though only where it exceeded an average yearly clear fell € , 2005). But as the sudden oversupply of timber (Nord and Sjo € , 2005), caused prices to plummet with at least 30% (Nord and Sjo and as much of it was severely damaged (“Sprickor,” 2006), suboptimal prices and quality made the necessary profits for the € , 2005; promised tax-cut insufficient for many (Nord and Sjo “Sprickor,” 2006), especially as 40% of the affected forest owners 133 some forest owners viewed the Forest Agency as indirectly responsible because of their plantation policy, which favored storm-sensitive fir tree (Engman, 2005a). While the quality of Gudrun-timber expired by AugusteOctober 2005 (Engman, € rbring, 2006). 2005b), the clearance work took up to two years (So During this time, fires and bark-beetle infestations ravaged the woods (Engman, 2005a). Conflicts emerged over access with prin, 2006) and environmental regulations on vate road-owners (Frede transport hindered the use of vehicles while prices for clearance work increased by 10e30% (Engman, 2005b). In addition, payments were held back with up to 2 years as it took up to five years for the entire stock to reach the market (“Betalning,” 2005). The hazardous clearance work, moreover, sporadically caused local hospitals to overflow with injured workers (Gatu, 2005). In some cases the € jning,” 2006). In a survey, Gothenburg injuries were fatal (“Ro University Hospital found that 1/3 out of 400 forest owners became very depressed after Gudrun. Some compared the loss of their forests with a loss of a human. In a few cases, the symptoms turned €s, 2006). physical, leading to hospitalization or suicides (Ålsna Nonetheless, after some time, the government exempted the fuel €rnberg, 2005b). And in late spring of for clearance work from tax (To 2006, S began to distribute 450million SEK from the EU solidarity fund via the Forest Agency to cover parts of reforestation costs in € rbring, 2006). 2006, 2007 and 2008 (So 3.6. Gudrun in the media The effects of Storm Gudrun have carried on to the present day. From Fig. 3 it is clear that media continues to report on her consequences. In the left panel we see the total number of Storm Gudrun related articles per year by the six major nationwide newspapers: Svenska Dagbladet (SvD), Dagens Nyheter (DN), Expressen (Exp), Aftonbladet (Aft), Dagens industri (Di), € teborgsposten (GP) and the largest southern newspaper SydsGo venskan (SYD). In 2005, the articles were 397. In 2014, the annual coverage was at 14% of the 2005 coverage with 56 articles, and in 2013 it was at 27% (the same as in 2008) with 105 articles. In the right panel we can see that the distribution of this coverage has a considerable spread across newspapers, displaying a broad media interest in the storm. Moreover, on January 7, 2015, 10 years after Gudrun, national television (SVT, 2015) broadcasted 3.10 min of up- Fig. 3. A Decade of News e Gudrun in the Media from January 2005 to January 2015. Note: The left panel in Fig. 3 shows the annual news coverage referencing Storm Gudrun from January 2005 until January 2015 by the six major nationwide newspapers and the largest southern newspaper (SYD). The right panel shows the breakdown by paper. Source: Own computation based on hits pertaining to “storm Gudrun” in the abovementioned online-based newspaper’s search engines. A careful read of the newspapers have made sure to exclude articles which may have come up in relation to either just storm or the Swedish politician, Gudrun Schyman. were uninsured (Engman, 2005a and “Messing,” 2005). In addition, 9 10 Based on historical data as per January 9, 2005 from XE. Ibid. to-date interviews with affected forest owners, who just had begun to recover financially from the storm. One woman conveyed how she had lost her pension, invested into her 50 year old forest, and she could pinpoint the exact location of where the C-party leader, 134 L.M. Eriksson / Electoral Studies 41 (2016) 129e142 Maud Olofsson, had been standing when moved to tears by the sight of Gudrun's destruction when visiting in the days following the storm. 4. Data, design and methodology I now turn to the analytical framework for the electoral punishment for the poor crisis response to the storm. The units of analysis are 288 out of Sweden's 290 municipalities.11 In order to estimate electoral returns to S's crisis response, I consider a treatment indicator, called Wind-max, which is a continuous variable that measures the maximum winds during Gudrun per municipality. This variable varies between 2 m/s (7 kph/4 mph) to 40 m/s (144 kph/90 mph). The data is from the Swedish Meteorological and Hydrological Institute (SMHI). More precisely, the measure is of wind gusts, which per definition represents intense short-bursts of high-speed winds.12 This approach to measuring a storm's severity and impact is widely used as an integrated part of more advanced models common to the emergency planning and post-event recovery field used on, for example, federal, state, regional and local levels of government in the United States (US), where wind-speed is combined with surface terrain and debris models to estimate a hurricane's impact on structures while also accounting for the variations in composition of those structures. While such advanced models are still imperfect measures of de facto hurricane damages, researchers are working on improvements suitable for the purpose of estimating damage risk and loss estimates for hurricane risk mitigation, emergency preparedness, response and recovery (Subramanian et al., 2014). However, Sweden did not have such advanced systems prior to Gudrun. Likewise, data on economic damage and self-reported damage to insurance companies is only available by type of sector or damage and not by geographical units,13 and not everyone had insurance coverage. Damage to forest is only reported on a much larger scale than municipalities14 and there is lack of complete information covering all of Sweden's municipalities regarding electricity outages15 (Guldåker, 2009; Westling, 2006). Detailed estimates of Gudrun's damage for all 288 municipalities are therefore not available, which makes the wind-speed an appropriate measure of storm impact. By measuring the impact of Gudrun in this way I intend to capture the degree to which a municipality was affected. That is, as no money transfers were given, they are assumed to have been 11 While Sweden had 290 municipalities in 2006, Knivsta was formed only in 2003, by separation from Uppsala, for which reason these two municipalities have been dropped from the regression analyses. 12 The majority of the data represents maximum winds at 10 m above ground, i.e. gusts, as measured on January 8e9, 2005 per municipality. The process of identifying a measure per municipality has not been completely straight forward, as SMHI does not divide its units of analysis according to political reference points, i.e. in this case municipalities. Rather, the measuring stations are scattered across the country according to logics of natural science, with the occasional consequence of some municipalities having several stations and others none. Therefore, to derive a comparable measure for each of Sweden's 290 municipalities as accurately as possible, I have consulted two meteorologists, Gunnar Berglund at SMHI and Adam Dingwell at CNDS, in the coding of Wind-max. For an explicit outline on this please see the Supplementary material to this paper. I obtained data from Gunnar Berglund via email between November 2012 and February 2013. 13 Personal communication with Erik Elvers at Finansinspektionen, Sweden's financial supervisory authority, on June 10, 2013. 14 Personal communication with Svante Claesson at the Swedish Forest Agency on December 10, 2013. 15 As many as 100 companies of varying sizes were in the electricity supply business in storm-affected areas (Westling, 2006), working in overlapping municipalities (Guldåker, 2009), which makes the collection of data on outages per municipality very difficult, if not impossible. In any case, such a data collection effort would only be possible if accurate records covering Gudrun's disruptions were kept per municipality for all those companies, which is unknown. Still, an outage measure would only capture part of Gudrun's damage to society. gradually more needed where the damage, and thus also the effects of Gudrun, were highest.16 The supplementary material to this paper lists all affected and unaffected municipalities, and their corresponding wind-speeds, as well as descriptive statistics and explanations of all variables used in the analysis. 4.1. Research design To identify the electoral punishment-effect of Storm Gudrun on the S vote share in the 2006, 2010 and 2014 parliamentary elections, the empirical analysis relies on a Difference-in-Differences (DiD) design. That is to say, I am interested in measuring the change induced by the Gudrun estimator, Wind-max, which represents the withinmunicipalities differences between the pre-storm and post-storm period of the treatment group (affected municipalities) and control group (unaffected municipalities)17 (Allison, 1990 and Angrist and Pischke, 2008:171e172). Thus, in line with Fig. 1 this design also assumes the counterfactual scenario of a continuation in parallel trends of vote support for the S party, with its nearly identical north-south discrepancy between elections prior to the storm, unless an unexpected treatment, like Gudrun, induces a local deviation away from that trend (Angrist and Pischke, 2008:171e172). As such, the expected treatment effect is the difference between the observed trend and the continuation of a parallel trend, which from Fig. 1 seems to be about 4% points between 2002 and 2006. Likewise, the drop for S exhibited by the unaffected municipalities during the same period is assumed to have been the same for the affected municipalities less Gudrun, which ought to be about 1% points (Angrist and Pischke, 2008:171e172). The advantage of the DiD design is that it allows for simplicity in the regression model. By studying treatment and control groups from the same context, in this case from the same country, any bias caused by known and unknown variables common to the overall political landscape of Sweden, is implicitly controlled for. This also pertains to any municipal factors that are stable during the period of examination, as only a change can have caused a deviation in parallel trends (Angrist and Pischke, 2008:170). Though, in the context given here, with an imperfect randomization of treatment, an obvious weakness with the parallel assumption is that it 16 On the one hand, it may be argued that prior experiences of storms among the electorate may protect the incumbent party from severe political punishment since it allows for realistic expectations of crisis response. It is therefore interesting to note that there has only been one storm prior to Gudrun that comes close to her scale and impact. This is the storm Ada, which occurred in 1969 and affected all of southern Sweden. Following Ada, the Allhelgona storm occurred only one month later and affected the mid-east. Before Ada there was a storm in 1967, Lena, which affected the southwest. However, as it differs nearly 40 years between Gudrun and these storms it is very unlikely that they should have affected on the average voter's evaluation of the S-government's crisis response to Gudrun. Closer in time, there have been two storms affecting the south, the 1995 Snowstorm and the 1999 December storm. Combined, these two storms more or less cover the geographical area of Gudrun. While the storm in 1995 have no estimates for economic damage or any records of casualties, the 1999 December storm affected 38 of the southern municipalities used in this study, caused three casualties and generated economic damage estimated to 9.7million SEK in insurance claims (roughly 1.13million EUR or 1.13million USD according to historical data as per November 10, 2015 from XE) (MSB, 2013 and EM-DAT, 2013). Given the close proximity in time of these two storms to Gudrun, it is possible that they, to some extent, may have muted the average voter evaluation of the crisis response to Gudrun. On the other hand, it is also possible that these storms built up an accumulated dissatisfaction with previous crisis responses to storms and that the severity of Gudrun therefore came to trigger a stronger negative lash out among those voters with prior storm experience. 17 While the groups are not binary coded, to convey the intuition of the design, I speak of treatment and control as if clearly defined by such boundaries. Though, in the case considered here, such groups are not under the control of the researcher, rather it is a matter of degree of affected or unaffected, which is selected by nature. L.M. Eriksson / Electoral Studies 41 (2016) 129e142 135 Table 1 Effects of storm Gudrun on S vote shares. Dependent variable S e Vote share Election years D2002e2006 Models (1) (2) (3) (4) Wind-max SD Unemployed Disposable income Foreign Women Age 0e4 Youth Elderly Population density Population outflow Constant R2 N .20*** (.02) .17*** (.02).50*** (.17) .73*** (.17) .10 (.99) .10 (.32) .22 (.67) 1.97*** (.48).28 (.40).43* (.26) 9.80 (133.25).28 (.25) .01(1.32).42 288.05*** (.02).05** (.02).07 (.13).28* (.15) 2.25*** (.72) .34 (.27) .06 (.47) .60* (.37) .05 (.28) .31* (.20) 66.81 (93.13) .53*** (.20) 4.31(1.02).18 288 2.06(.71).31 288 D1998e2002 2.20(.59).05 288 ***p <.01 **p <.05 * p <.1 Note: OLS regression coefficients shown with robust standard errors in parentheses. Each DiD-regression includes measures of all variables per municipality. Models 1e2 measure the effects on change in vote support for S between the 2002e2006 elections. All covariates, except for Wind-max, are change measures between 2002 and 2006. For Models 3e4 the dependent variable pertains to the 1998e2002 elections. While Sweden had 290 municipalities in 2006, Knivsta was formed only in 2003, by separation from Uppsala, for which reason these two municipalities have been dropped from the analysis. overlooks the possibility of a simultaneous occurrence of some other factor, outside the treatment, in one of the groups. When localizing control variables, therefore, the identification of factors that follow a simultaneous trend of wind speed is fundamental. Yet in order for such factors to be included in the analysis they may not be causally affected by the treatment itself, as this would make them bad controls since they thus become an effect of the theorized treatment rather than a cause of the outcome of interest (Angrist and Pischke, 2008:49e51). In the following sections of this paper I have therefore been careful to only include potential confounding issues that could be argued to be of such regional concern as Gudrun into the regression analysis, while most likely being causally unaffected by the storm. variations in winds.18 In this way I avoid an over-or underestimation of Gudrun's total effect (Angrist and Pischke, 2008:49e51). Finally, to further verify the accuracy of the parallel trends assumption, I conduct a placebo test by estimating a similar DiD specification for the 1998 to 2002 pre-storm period. Ultimately, this is an important test to see how precisely the unaffected north and the affected south followed parallel trends prior to Gudrun. As illustrated by Fig. 1, this trend is almost perfect, thought not exact, as it seems that S gained a slightly stronger support in the south between 1998 and 2002. Thus, in order for the parallel trends assumption to be robust, it is important for Gudrun to have a very small effect, if at all, on the pre-storm period. 5. Empirical analysis 4.2. Model specification For now, I consider i ¼ {1,…,N} municipalities for elections in years t ¼ {1998, 2002, 2006, 2010 and 2014}. Let Xi be the continuous indicator of degree of affectedness between the current and previous period. The estimated treatment effect is then given by Y2t Y2c ðY1t Y1c Þ i.e. the posttest difference in means minus some fraction of the pretest differences in means. Primarily, I estimate the treatment effect using a DiD-model given by Yi2 Yi1 ¼ t þ dXi þ ε*i : Where Yi2 e Yi1 represents the outcome of interest, i.e. the change (D) in S's proportional representation (PR) vote share between the pretest and the posttest, and where t is the parameter that represents the intercept for the regression that measures the average change in S-support when wind speed is 0, d is the treatment effect, and ε*i ¼ εi2 e εi1, are random disturbances with the further assumption that E(ε*i jXi) ¼ E(εi2jXi) e E(εi1jXi) ¼ 0. This way of modeling the treatment effect assumes that the gap in parallel trends would have been stable over time in the absence of Gudrun (see the counterfactual trend in Fig. 1). Also, from a theoretical point of view the DiD-model is desirable since it captures the whole mandate period. Moreover, to avoid problems with bad controls, I estimate one short and long model for each regression. For the longer, I only include a restricted number of controls that presumably are causally unaffected by Wind-max, while also being likely to exhibit trends that could account for the north south divide exhibited by In this section the results from the empirical analyses are reported. First, I estimate the model described in the preceding section, which constitute the main results.19 Then, I perform a critical validity test of the main findings and a more fine-grained analysis of the main results in an extension with robustness checks. 5.1. Main results The results from Models 1 and 2 in Table 1 present the long and short DiD-regressions for the 2006 election, which show very similar results with regards to the impact of Gudrun on incumbent support. In particular, I find that the S vote share fell more, the larger the impact of Gudrun on the municipality. Model 2 shows the preferred change score equation. The correlation is significant and large, with a negative coefficient of .17 and a 95% confidence interval ranging from [.21 to .12]. To get 18 While it could be argued that Gudrun could have affected the unemployment rate, as the task of re-building following the storm was rather labor intense, this is more likely to have affected individuals already holding employment in terms of overtime, rather than the real unemployment rate. Thus, it could potentially have affected on disposable income. In any case, I have verified the effect of Gudrun on the unemployment rate and it is substantially small indicating only a.02% points increase with a statistically significant coefficient of.05, while the coefficient for Gudrun's effect on disposable income also is significant but at a value of .00. 19 In the Supplementary material to this paper I perform a further robustness test of the main results by means of a regressor variable model (see section IV: Additional Robustness Checks, Table 2). 136 L.M. Eriksson / Electoral Studies 41 (2016) 129e142 a feel for the substantive size of the effect, the expected drop in voter support for S resulting from a one standard deviation (8.7) increase in wind speed is approximately 1.5%-points.20 Gudrun's average maximum wind speed, covering all 288 municipalities, was 26.5 m/s (95 kph/59 mph) (þ a standard deviation of 8.7), which suggests that voter support for S dropped by about 4.5%points, on average21 due to the storm. This is not far from the rough estimate of a 4%-point drop seen in Fig. 1. Compared to the overall S vote share of 39.85% in 2002, which decreased to 34.99% in 2006, leaving about 87% of the 2002 vote share intact (Election Authority, 2013), the Gudrun effect constitutes about an 11.3% drop in the S vote base when using the estimated effect of 4.5% points. As this exceeds the 8% of blockmovers between S and M, identified as the most important factor for the election outcome by previous research (Oscarsson and Holmberg, 2009:7, 14e15, 21), it is not unreasonable to assume Gudrun to be a major protagonist in this drama. Thus, these results indicate that the more conservative sentiments of the 2006 election may have come in with the winds of Gudrun. And as the Alliance won the election with a narrow 2.1% points margin over a left-block alternative, Gudrun proves a very likely piece of missing evidence in the tale of the historic 2006 regime shift. How confident can we be in these results? As mentioned previously, in localizing control variables, it is a priority to identify a simultaneous occurrence, i.e. the existence of a confounding variable that correlates with wind speed and may have an effect on change in S-support between the 2002 and 2006 elections. One additional battleground issue that has been on the political forefront is that of immigration. The main anti-immigrant party, named the Sweden Democrats (SD), has had a substantial increase of more than 100% in vote shares between 2002 and 2006, before entering parliament in 2010 (Sweden Democrats, 2013). A quick look at the trends in the voter share data for SD between 2002 and 2006 from Statistics Sweden confirms that their increase is primarily in southern Sweden. In Model 2, I therefore added the change in vote support for SD to see if some of the Gudrun effect is picked up by their increase in vote support. The change is marginal. If anything, the increase in vote support for SD seems to correspond with an increase in vote support for S. Thus, since SD correlates positively with S, it cannot explain the negative correlation between Gudrun and S. Nonetheless, there may still be other factors that co-vary with wind speed within the same four-year interval. As such, variables that are time-invariant from pretest to posttest may be omitted from the analysis without introducing bias (Allison, 1990). The remaining controls therefore, are intended to capture various demographic and socioeconomic shocks, such as changes in unemployment, disposable income, share of foreigners, gender differences, share of newly born (0e4), share of youth (15e24), share of elderly (60þ), population density and population outflow.22 The effect of unemployment is significant and substantially negative, which is in line with previous research identifying jobs as one of the most important issues for block-movers (Oscarsson and Holmberg, 2009:19, 24e25, 33), as well as theories of economic voting broadly. The only other variable that significantly affects on 20 One standard deviation (8.7) coefficient (.17) ¼ 1.5. Average wind speed (26.5) coefficient (.17) ¼ 4.5. 22 Notice that other studies have used measures for economic voting broken down into sectors, such as employment share per agriculture, manufacturing, trade services, and other services (Bechtel and Hainmueller, 2011). Unfortunately, such data is unavailable here due to a change in definition of employment imposed by Statistics Sweden in 2007, as well as the standard age intervals used. This change affects all data from 2005 onwards. Thus the cut-off point falls in the middle of the time interval of interest for my analysis. Data for older definitions are available by region up to 2007, but here the sectoral breakdown is only available up until 2004. 21 drop in vote shares is newly born. Political aspects of relevance for this age group may explain the effect. For example, child-care is a relevant and debated issue that frequents on the political agenda. Models 3 and 4 in Table 1 present the placebo tests of the prestorm period 1998 to 2002. The results indicate that the S vote share is largely similar between affected and unaffected municipalities, as they follow a nearly identical trend prior to Gudrun. There is only a slightly higher support for S in the most affected municipalities. Based on Model 4 I calculate the effect of increasing wind speed (which is better captured by the variation in wind, as it deviates from the mean, rather than the mean of wind speed itself) by means of one standard deviation: 8.7*.05 ¼.44%, which is not that large an effect compared to the effect of the main results. Based on Model 2: 8.7*.17 ¼ 1.5%. Moreover, the coefficient in Model 4 is less than 1/3 of the coefficient in Model 2. And, if we compare Models 1 and 3 the difference is even larger. In sum, while the effect of the placebo test is statistically significant, it is positive rather than negative, and small in magnitude compared to the main results. The fact that the placebo is positive may be considered good, as it would have been quite problematic if the sign had been the same as for the post-storm period. That is, if the placebo had been negative, then there would have been reason to believe that something other than Gudrun, i.e. some con-founding variable, would be driving the identified treatment effect. This is so, as a negative placebo would suggest that Gudrun is picking up on some con-founding variation that was initiated prior to her own occurrence. In light of the positive sign of the placebo, therefore, we may at least disregard the possibility that some con-founding variable occurred prior to the 2002 election. The placebo-test can therefore hardly be considered as indicative of a serious violation of the parallel trends assumption, especially as it is a substantively unimportant result. Instead, I interpret the given coefficients of Models 3 and 4 as indicative of a continuation in parallel trends less Gudrun. 5.2. Threats to validity While the foregoing analysis takes into consideration the standard controls for a DiD-analysis, which are intended to capture various demographic and socioeconomic shocks that could co-vary with the continuous measure of wind-speed, there is still one critical threat to validity that we must consider. This is the clear southnorth divide caused by Gudrun's path through the south in Fig. 2 (left panel), making the north the control group. This leaves open the possibility that the main analysis is comparing the south and north as much as it is comparing affected and unaffected municipalities. For this reason it is plausible that some unobserved variable of political relevance changed between the south and north around the time of the election that had nothing to do with Gudrun. That is, the observed treatment effect might be caused by this unobserved variable. While the binary coding in Figs. 1 and 2 (left panel) is used to illustrate the notion of parallel trends between treatment and control groups, prior to Gudrun (Fig. 1) and how this has been coded by means of Gudrun's geographical movement (Fig. 2, left panel), the measurement used in the main DiD-analysis differs from this dichotomous approach by also taking into account the variation in wind-speed between the municipalities in the south, as well as between the municipalities in the north.23 Nonetheless, it may still 23 For a complete list of the variation of wind-speed between municipalities in the south please see section: II: List of Affected Municipalities and Their Experienced Wind Speeds in the Supplementary material to this paper. And, for the same variation between the municipalities in the north please see section: III: List of Unaffected Municipalities and Their Experienced Wind Speeds, also listed in the Supplementary material to this paper. L.M. Eriksson / Electoral Studies 41 (2016) 129e142 137 Table 2 -vis a South-North Divide on S Vote Shares. Critical Validity Tests of Gudrun vis-a Dependent variable S e Vote Shares Election years D2002e2006 Models (1) (2) (3) (4) SouthNorthDummy Wind-max Wind-max South SD Unemployed Disposable income Foreign Women Age 0e4 Youth Elderly Population density Population outflow Constant R2 N 3.20*** (.46) 1.98*** (.45).79 (.67) .24*** (.04) 1.54** (.67) .23*** (.04) 1.06(.42).20 288.28* (.17) .95*** (.22) 1.30 (1.16) .16 (.34) .20 (.69) 2.17*** (.54).57 (.43).55* (.28) 47.77 (153.99).34 (.28) 2.50(1.29).34 288 2.42(.82).31 288.50*** (.17) .81*** (.18) .26 (.98) .06 (.32) .32 (.66) 1.94*** (.48).32 (.39).46* (.27) 9.27 (132.42).25 (.25).52(1.35).43 288 D2002e2006 D2002e2006 (5) (6) .10*** (.04) .12*** (.04).47*** (.17) .61*** (.17) .74 (.96).21 (.35).56 (.71) 1.73*** (.53).70* (.42).14 (.29) 1.79 (101.58) -.19 (.23) 1.18(1.73).26 206 1.01(1.13).03 206 ***p <.01 **p <.05 * p <.1 Note: OLS regression coefficients shown with robust standard errors in parentheses. Each DiD-regression includes measures of all variables per municipality. All Models measure the effects on change in vote support for S between the 2002e2006 elections. Models 1e2 measure the treatment indicator as a south- north dummy corresponding to the coding of the left panel in Fig. 2 (1 for the south and 0 for the north). In addition, Models 2e3 include the Wind-max measure as a treatment indicator, which measures the variation in winds between all units of analysis. Models 5e6 only use the southern units of analysis (206 municipalities corresponding to the affected (grey) area in the left panel in Fig. 2) of the Wind-max measure. All covariates in all models, except for the treatment indicators, are change measures between 2002 and 2006. While Sweden had 290 municipalities in 2006, Knivsta was formed only in 2003, by separation from Uppsala, for which reason these two municipalities have been dropped from the analysis. This gives 288 units of analysis for Models 1e4 and 206 units of analysis for Models 5e6, which drops the northern observations from the analysis. be the case that the result in Table 1 is picking up a south-north divide generated by some other political issue of relevance for the south. To investigate this possibility further, in Table 2 I present an analysis where I use the same binary coding as in Figs. 1 and 2 (left panel) to create a variable labeled SouthNorthDummy, which is coded 1 if affected and 0 if unaffected. In Model 1 I test the SouthNorthDummy variable separately to see if it has a negative effect on the change in S-votes. Indeed, when using the binary coding of a south north divide we see a significant negative average effect on S vote shares with 3.2% points. This supports the notion that a divide between the south and north accounts for a large share of the drop in the S-incumbent party's vote support. In Model 2 I add the controls used in the main analysis for Wind-max (Model 2 in Table 1) and find that while the substantive effect decreases compared to Model 1, as it now only shows an average 1.9% points decrease in S-votes, it is still there and it is highly significant. While this confirms that there is a southnorth divide, there is no way to know from this analysis whether or not the SouthNorthDummy is actually picking up on an unobserved, and to us unknown, political change between the south and the north, or if it is a less fine tuned measure of the variation in windspeed per municipality, i.e. of Wind-max. In Model 3 I therefore test both of these measures on the change in S-votes, which shows that the Wind-max measure is robust to some other potential political -vis north divide. In fact, the SouthNorthDummy turns south vis-a out positive and insignificant. I further corroborate this finding in Model 4 by adding the remaining controls, which now estimates a positive and significant effect for the SouthNorthDummy with a substantive average increase in S-vote shares of 1.54% points in the south. What is more, the substantive size of the Gudrun coefficient has increased from .17 (Model 2 in Table 1) to .23. This implies that if there were a divide in politics between the south and north, other than the S-party's poor crisis response to Gudrun, then this unobservable and unknown issue ought to have yielded an increase in votes for S, rather than a decrease, when the storm is accounted for. Also, the substantive size of the negative effect on S-votes by Gudrun ought to be larger than previously indicated (about an average 6% points24 instead of an average 4.5% points). However, as the SouthNorthDummy is highly sensitive to the different models, i.e. as it is not robust to the Wind-max measure, and as there is neither empirical nor theoretical reason25 to believe that there were any other regional concerns than Gudrun that suddenly would have created a south-north cleavage between the 2002 and 2006 parliamentary elections, we do not have any justifications for why the SouthNorthDummy should be included in the model in the first place. Certainly, if something other than Gudrun would have yielded such a remarkable impact on the Swedish political landscape as to cause the historic disruption in parallel trends of vote-support for the incumbent party between the 2002 and 2006 parliamentary elections, displayed in Fig. 1, it should have been reported on in media, and it ought therefore not to be unknown to us. In light of these critical validity tests we may therefore only conclude that the SouthNorthDummy does not belong in the model. Nevertheless, to thoroughly dispose of the south-north confounder possibility, I run one last critical test of the validity of my main findings in Models 5 and 6 by dropping the northern units of analysis in the Wind-max measurement. This approach will allow me to examine if there is a co-variation between wind-speed and a drop in S- vote shares only in the south. Model 5 shows a significant and substantively negative average effect of 3.1% points26 of Gudrun on S-votes. This indicates that the variation in Gudrun's destructive winds between municipalities in the south had enough of an impact to generate a critical evaluation of the S-party's poor crisis response to Gudrun among party supporters. From this test it is evident that the variation in the southern impact of Gudrun, rather than a variation between a south and north divide in politics, accounts for a substantial portion of the drop in S-vote shares. In 24 Average wind speed (26.5) coefficient (.23) ¼ 6.1. Besides from a possible build up of dissatisfaction with previous government responses to storms, over time in the south, as discussed in footnote 16. 26 Average wind speed for the south (31.25) coefficient (.10) ¼ 3.1. 25 138 L.M. Eriksson / Electoral Studies 41 (2016) 129e142 Fig. 4. Unaffected North vs. Affected South by Biannual Popularity Polls for S and M. Note: Fig. 4 illustrates the north south divide in popularity of S and M between the 2002 and 2006 elections. The left panel shows the percent of respondents who sympathize with S and the right panel displays the same for M, both with 95% confidence intervals. The graphs are based on data available from the Party Preference Survey (PSU), which mostly uses provinces as units of analysis. The provincial borders are not too far off from the cut-off point in Fig. 1, so the divisions of affected and unaffected presented here are comparable to Fig. 1. Average n ¼ 9222 [min n ¼ 9090, max n ¼ 9331]. Here, north is represented by “SE06P € Norra Mellansverige (l€ an 17 þ 20e21)” and “SE07þSE08 Mellersta och Ovre Norrland (l€an 22e25)”, while the remaining provinces in the dataset represent south. Source: Own computation based on data from Statistics Sweden (SCB). Model 6, I test the robustness of this result by adding the remaining controls. As can be seen from the slight substantive increase in the negative effect of the highly significant Wind-max South coefficient, from .10 to .12, the results underlying the rebuttal of a potential south-north political division as a threat to the validity of my main findings remain robust. The result from Model 6 gives the most conservative estimate thus far of Gudrun's impact on S votes, indicating an average drop of 3.8%-points,27 which is very close to the 4% point drop seen in Fig. 1. 5.3. Extension and robustness checks In sum, the results so far suggest that the S party experienced a considerable decrease in vote shares in storm-affected municipalities in the 2006 parliamentary election. Yet, it still leaves open for unobserved confounding factors that could have caused a drop in vote support at any point between the 2002 and 2006 elections, insofar as such factors co-varies with Wind-max. One way to dispose of alternative confounders is to look at biannual popularity polls conducted in-between the two elections. If something other than Gudrun, influential enough to manipulate votes, has occurred, then, it should be identifiable from the polling data. That is, it will allow for a more explicit observation as to when the deviation in parallel trends occurred. Fig. 4 displays such trends for S and M from the “Party Preference Survey” (PSU) (Parti€kningen).28 For each 6-month period beginning sympatiunderso shortly after the 2002 election in September, the polling data measures the party popularity in November and May of every year until the 2006 election. The popularity trends for S (left graph) and M (right graph) are nearly identical between affected and unaffected municipalities before Gudrun. At the time of the storm, however, a sharp deviation in popularity is noted between the north and south for both parties. While S receives a parallel drop 27 Average wind speed for the south (31.25) coefficient (.12) ¼ 3.8. This data measures the party deemed as the best party according to the respondent and is available from Statistics Sweden, dataset identification: parti€kningen (PSU) “Med partisympati efter region och parti. (Urvalsympatiunderso €kning, se fotnoter.) 2 ggr/år 1994M05-2010M11”. sunderso 28 between Nov04 and May05, the north recovers in Nov05 whereas the south continues horizontally until shortly after the next election in September of 2006. For the M party one also notices a slight deviation in parallel trends between north and south shortly after Gudrun. In fact, M receives a boost in popularity in the stormaffected south compared to the north. A gain that more or less remains until shortly after the 2006 election. On the one hand, this indicates that the fall in S vote shares may be ascribed to the incumbent's poor crisis response toward affected citizens. On the other hand, this also seems to have opened a window of opportunity for the opposition to gain some strongholds in popularity, which lends support to a possible block-transfer from S to M. Given the foregoing analysis, it thus seems unlikely for the deviation in parallel trends of S to be accounted for by unobserved confounders, especially as they must co-vary with the variation in winds per municipality and coincide fairly well in time with Storm Gudrun. In sum, the PSU analysis provides more fine-grained evidence that the southern drop was closely timed with Gudrun. Other possible explanations would either be a forceful campaign initiated by the opposition that to a much greater extent won the hearts and minds of the southern population, or a rivaling crisis that overlapped with Gudrun. Two such incidents did coincide with the Nov04 and May05 polls, but a closer look at the evidence, examined below, shows they fail to account for the observed outcomes. On August 31, 2004, the M-led Alliance was officially formed (Alliansen, 2004) and in December the 2004 Boxing Day Tsunami struck. In fact, these are both commonly accepted explanations as to why the popularity of S dropped and M gained in the 2006 election. On the one hand, previous research argues that the Alliance's common agenda offered a clear alternative to S (Oscarsson and Holmberg, 2008b: 329, 2009:30). On the other hand, S seem to believe that their mismanagement of the 2004 Boxing Day Tsunami, which received much criticism, and seriously affected some 3000 Swedes vacationing in South East Asia (Crisis Commission, 2005), damaged the voter's trust in their ability to govern (Social Democrat's Crisis Commission, 2010:33). This latter incident is also commonly accepted as a mainstream explanation as to why S lost popularity in the 2006 election (see, for example, UI, € m, 2006 and Franchell, 2010). I will now address each 2012; Hallstro L.M. Eriksson / Electoral Studies 41 (2016) 129e142 139 Table 3 -vis the 2004 Boxing Day Tsunami on S Vote Shares and Long-term Analysis of Change in Party Support. Robustness Checks of Gudrun vis-a Dependent variable S e Vote shares Election years D2002e2006 Models (1) (2) (3) Tsunami Wind-max SD Unemployed Disposable income Foreign Women Age 0e4 Youth Elderly Population density Population outflow Constant R2 N 12.79*** (2.13) 7.83*** (1.78) .18*** (.03) 5.40*** (1.80) .15*** (.03).34* (.18) .64*** (.17).76 (1.07) .23 (.32) .27 (.66) 1.73*** (.48).23 (.39).33 (.27) 18.81 (126.33).30 (.25).42(1.32).44 288 1.77(.32).15 288 D2006e2010 2.30(.71).36 288 (4) .24*** (.02) 2.60(.52).50 288 D2010e2014 (5).10 (1.46) .20*** (.02) .44*** (.16) .48*** (.14) .83 (.85) .15 (.28).56 (.58) 1.25*** (.37) .15 (.32) .10 (.26) 86.34 (77.39).09 (.18) 3.67(1.16).57 288 (6).12*** (.02) 3.22(.47).24 288 (7) 2.67* (1.35).10*** (.02) .03 (.13).06 (.13) 1.29* (.68).25 (.24) .28 (.49) 1.03*** (.29).02 (.28).01 (.19) 48.08 (98) .07 (.17) 4.07(.98).31 288 ***p <.01 **p <.05 * p <.1 Note: OLS regression coefficients shown with robust standard errors in parentheses. Each DiD-regression includes measures of all variables per municipality. Models 1e3 measure the effects on change in vote support for S between the 2002e2006 elections. Models 4e5 measure the change for the dependent variable between the 2006e2010 elections and Models 6e7 do the same for the 2010e2014 elections. All covariates in all models, except for Wind-max and Tsunami, are change measures between 2002 and 2006. While Sweden had 290 municipalities in 2006, Knivsta was formed only in 2003, by separation from Uppsala, for which reason these two municipalities have been dropped from the analysis. of these issues in turn. As we know that the Alliance was formed before the Nov04 poll, it cannot be this event in itself that caused S to fall between Nov04 and May05. If so, it should have been discernable already between May04 and Nov04. What is more, as much work remained before the Alliance could formulate a cohesive political alternative it also seems unlikely that the announcement of an alliance in itself would have caused the southern extended drop in S popularity while boosting it for M. In fact, it was first one year later on August 31, 2005 that a common agenda on employment and the infamous income tax reduction for workers, which became the Alliance's strongest card in the 2006 election campaign, was established (Alliansen, 2005). Moreover, from Fig. 4 it is evident that M gains popularity in storm-affected municipalities prior to the launch of their agenda, which coincides with the Nov04eMay05 time-span of Gudrun. Of course, one might still say that the formation of the Alliance set in motion a process that affected the electorate between Nov04 and May05. But there is no obvious reason for those processes to have had different effects in the north and south whereas such a reason exists for Gudrun. If anything, the launch of the Alliance's agenda seems to coincide with a slight increase in popularity for S between May05-Nov05 in storm-affected municipalities. As such, there does not seem to be further theoretical reasons to control for the opposition in the 2006 election. Rather, it seems clear that the deviation in parallel trends match Gudrun. In contrast to the formation of the Alliance however, the 2004 Boxing Day Tsunami provides a more serious rival explanation due to its timing in relation to Gudrun. But here too, there is no clear reason for why the tsunami should have had divergent effects in the north and south. In fact, the Tsunami had an immediate impact on the entire political landscape of Sweden and led to a national grief that still resonates in the memory of many Swedes today. While it is true that the government was extensively criticized for it's mishandling of the crisis and media covered the affair with great attention, it is precisely because of this vast coverage one would expect a nation-wide drop in support for S and not a northern biased recovery soon after. In short, the tsunami theory also falls short in explaining the north south divide that Gudrun accounts for. To probe this, I test the tsunami theory in Table 3. Here, I use data on the percentage of the municipal population that became registered as tsunami-affected citizens at passport controls upon their return to Sweden from South East Asia (National Police Board).29 I label this variable Tsunami. The results from Models 1e3 present the long and short DiDregressions for the Tsunami. In Model 1 the effect on the change in S vote shares gives a significant correlation of 12.79. In Model 2 I add Wind-max, which turns out robust against the tsunami theory. In comparison to Table 1(Model 1), the coefficient decreases slightly from .20 to .18 while the tsunami coefficient decreases to 7.83. Model 3 adds the remaining controls from the previous DiDregression (Table 1, Model 2). These decrease the effect of Gudrun compared to Model 2 to a coefficient of .15 with a 95% confidence interval range of [.20, .10], as well as the effect of the 2004 Boxing Day Tsunami to a coefficient of 5.40 with a 95% confidence interval range of [8.94, 1.86]. This implies that the S vote share decreased with about.7% points in a typical tsunami affected municipality.30 While the 2004 Boxing Day Tsunami affected only 1.2 per mill of the population, the average drop of.7% points in vote support represents about 7 per mill of all voters.31 There seems to be a diffusion effect of negative attitudes to 7 others for every affected individual, which considering the vast media coverage does not seem too far-fetched given that mass communications and local media coverage may have interacted in synergy with individual networks, such as family, friends, colleagues, voluntary sector and associations. In short, while the tsunami did have a negative effect on the S vote support in the 2006 election, it is separate from that of Gudrun. The analysis so far has focused on the 2006 election, which leaves open the question of how lengthy the negative effects on S 29 I received the data via email from the National Police Board upon request. My personal communication in this matter was with Stina Haraldsson who sent me the data on October 10, 2013. 30 Average municipal percent of tsunami-affected individuals (.12) coefficient (5.4) ¼ .7. 31 In 2006 the reported population size for Sweden was approximately 9,107,935 (Statistics Sweden 2013). 140 L.M. Eriksson / Electoral Studies 41 (2016) 129e142 vote shares may be. In order to identify whether the drop in vote support constitutes a mere short-term punishment in the 2006 election, or a more durable trend in line with a long-term change in party support, I also test the effects of Gudrun on the 2010 and 2014 elections. In Models 4e5 the short and long DiD-regression results for the 2010 election is presented. Interestingly, the negative effect remains and continues to increase in the 2010 election, implying that the S vote share continued to fall beyond the 2006 election. In Model 4 the effect on the change in S vote shares gives a significant correlation of .24, which decreases to .20 in Model 5 when adding the controls. This gives an additional drop of 5.3% points on average in voter support for S between 2006 and 2010. In contrast, however, Model 6 shows a significant and positive correlation of.12, which decreases to.10 in Model 7 when adding the controls. This suggests that a previously felt average loss of 2.7% points in vote support for S across Gudrun affected municipalities was regained in the 2014 election. In sum, when considering the lengthy effect of the Sparty's poor handling of the crisis we have an average drop of 4.5% points in the 2006 election compared to the 2002 election. This average drop across storm affected municipalities continues to fall to an overall 9.8% points in S vote shares in the 2010 election (compared to 2002) but bounces back to an, on the whole, lengthy average loss of 7.1% points in the 2014 election (compared to 2002). The continuation in loss of vote support for S found in the 2010 and 2014 analyses is in line with the continuation in deviation of parallel trends between affected and unaffected municipalities in the 2006 to 2014 period presented in Fig. 1. It may very well be that the lengthy process of recovery from Gudrun, perhaps both in regards to prolonged disruptions in electricity supply and economic suffering in the forest sector, could have continued to trigger shifts in party support away from S after the 2006 election. This is not unlikely, as we know that the impact of Gudrun's damage in these sectors transcended beyond the 2006 election and that media continues to report on Gudrun's effects until date. In any case, and most importantly, however, the lengthy effects are strongly suggestive that many former S-supporters indeed have stayed away from the party. These results support a change in party support due to the government's poor handling of the extended crisis. In particular, as the majority of retrospective judgments of S have lasted over the 2010 and 2014 elections too, the results are more in line with a long-lasting change in voter-support by previous long-standing party-supporters than a short-term punishment. In addition, further analyses presented in the supplementary material corroborate that this long-term trend and shift in support from S also largely went to M for the long-term, in both the parliamentary and municipal elections.32 Thus, as the results suggest a fairly persistent effect, in the context here, shift in party support reasonably ought to rest on enough time for a process of long-lasting change to happen in storm-affected municipalities. 6. Conclusion The findings presented here add to our knowledge concerning electoral accountability in the crisis response to natural disasters. As Gudrun happened to strike a country with a historically long- 32 For analyses of the parliamentary votes gained by M in storm-affected areas, please see the supplementary material section IV: Additional Robustness Checks, Tables 3 and 4, and for municipal election analyses of S and M please see section VI: Additional Corroboration of Main Findings and Robustness Checks, Tables 6, 7, 8 and 9. standing and stable Social Democratic vote-base, it makes her a crucial case for the study of a possible wider change in party support, caused by the poor crisis response of S, with a de facto absence of money transfers, to Gudrun-affected individuals. The most conservative estimate of my results indicate an average drop in S vote-support of about 3.8% points in the 2006 Swedish Parliamentary Election, 1 year and 8 months after the storm. As the punishment carries over to the 2010 and 2014 elections, the results suggest a quite durable change in party support rather than just a short-term punishment. In this regard, considering that Gudrun stands at par with Hurricane Katrina with 1% of GDP in economic damage, while exceeding that of the Elbe flooding by 100%, and that money transfers were given in both these cases but not in the case of Gudrun, it seems convincing that the affected public evaluated the response as a failure. This may also have been associated with insights among S-supporters of a blatantly insufficient capacity of the central SOS-system, which overloaded in the most acute moment. Thus, Gudrun provides a clear-cut case of unusually poor crisis response, with the hitherto longest time dimension for an accountability analysis of retrospective voting, extending over 9 years and 8 months and causing very plausible sudden changes in previously longstanding party support. From a democratic perspective, these results establish the longest accountability analysis, both within an election cycle and over elections, while also corroborating previous findings in the Elbe study, indicating the potential lengthy accountability effects associated with the retrospective voting mechanism (Bechtel and Hainmuller, 2011). This opens up for the question of how long lasting can it be? Rather than speaking of short-term rewards and blame in relation to electoral accountability, we may now seriously consider the lengthy implications on politics that this mechanism may yield. The evidence from this study suggests that the effects of crisis response in a previous mandate period can continue to affect voter support in the next election cycle and cycles to come. Thus, it would be recommended to keep the possibility of such lengthy effects in mind in any given election analysis. And quite interestingly, as I find that previously long-standing S-supporters indeed have stayed away from the party over nearly 10 years, this study also opens up for questions concerning changes in party identification, as party support, at least in the context here, may be considered closely associated with party-identification. This may be of interest for other studies to explore. This study also gives a more nuanced account of the 2006 Swedish Parliamentary Election. Here, the retrospective voting theory accounts well for the large block-transfers between S and M. Perhaps it is not too unlikely to think that those S-supporters who were most affected by Gudrun, or who had family, friends, people in their social networks and neighborhoods affected, also were disappointed over the crisis response and perceived of it as insufficient and a failure of solidarity with the storm's victims. In turn, they may have been more motivated to vote for the M-led Alliance in the 2010 election as well, feeling less inclined to contribute to the higher tax-rates associated with S, which may be particularly true for those who trusted that money transfers and aid would have been available should they ever experience a crisis. This could potentially imply that left leaning social welfare parties may be more vulnerable to punishment for failing to deliver transfer support to disaster victims. In sum, the results indicate that policy makers ought to carefully consider the costbenefit analysis of policy response to natural disasters, regardless of when in the election cycle it occurs. And, it highlights the importance for political leaders to skillfully manage their way out of a crisis if they wish to keep the hearts and minds of their people in the aftermath. L.M. Eriksson / Electoral Studies 41 (2016) 129e142 Acknowledgments 141 Supplementary data related to this article can be found at http:// dx.doi.org/10.1016/j.electstud.2015.12.003. Cambridge University Press, Cambridge, pp. 1e26. €rfo €r vann alliansen. Statistics Sweden, Oscarsson, H., Holmberg, S., 2009. Da Stockholm. Oscarsson, H., Holmberg, S., 2008a. Alliansseger. Statistics Sweden, Stockholm. €ljarna och valet 2006. NorOscarsson, H., Holmberg, S., 2008b. Regeringsskifte, Va stedts Juridik, Stockholm. Reeves, A., 2011. Political disaster: unilateral powers, electoral incentives, and presidential disaster declarations. J. Polit. 73 (4), 1142e1151. Schickler, E., Green, D.P., 1997. The Stability of Party Identification in Western Democracies: Results from Eight Panel Surveys,. Comparative Political Studies 30 (4), 450e483. Sinclair, B., Hall, T.E., Alvarez, R.M., 2011. Flooding the vote: hurricane katrina and voter participation in New Orleans. Am. Polit. Res. 39 (5), 921e957. Social Democrat’s Crisis Commission, 2010. Valanalys. Report. Socialdemokraterna, Stockholm. Strom, K., 2003. Parliamentary democracy and delegation. In: Strom, K., Muller, W.C., Bergman, T. (Eds.), Delegation and Accountability in Parliamentary Democracies. Oxford University Press, Oxford, pp. 55e106. Subramanian, D., Salazar, J., Duenas-Osorio, L., Stein, R., 2014. Building and validating geographically refined Hurricane wind risk models for residential structures. Nat. Hazards Rev. 15 (3), 1e10. Twigg, D.K., 2012. The Politics of Disaster: Tracking the Impact of Hurricane Andrew. University Press of Florida, Gainesville, USA. € r€ Wern, L., B€ arring, L., 2009. Sveriges vindklimat 1901-2008: analys av fo andring i geostrofisk vind. Report. Meteorologi nr. 138/2009. Swedish Meteorological and € ping. Hydrological Institute, Norrko Westling, L., 2006. Stormen Gudrun. Vad kan vi l€ ara av naturkatastrofen 2005. Report. ET2006:02. Energymyndigheten, Bromma. References Web references Abney, G.F., Hill, L.B., 1966. Natural disasters as a political variable: the effect of a hurricane on an urban election. Am. Polit. Sci. Rev. 60 (4), 974e981. Achen, C.H., Bartels, L.M., 2004. Blind Retrospection: Electoral Responses to Drought, Flue, and Shark Attacks. Working paper. Princeton University. Allison, P.D., 1990. Change scores as dependent variables in regression analysis. Sociol. Methodol. 20, 93e114. Angrist, J.D., Pischke, J.S., 2008. Mostly Harmless Econometrics: an Empiricist's Companion. Princeton University Press, Princeton. Arceneaux, K., Stein, R., 2006. Who is held responsible when disaster strikes? the attribution of responsibility for a natural disaster in an urban election. J. Urban Aff. 28, 43e53. Bechtel, M.M., Hainmueller, J., 2011. How lasting is voter gratitude? an analysis of the short- and long-term electoral returns to beneficial policy. Am. J. Polit. Sci. 55 (4), 851e867. Besley, T., Burgess, R., 2002. The political economy of government responsiveness: theory and evidence from India. Q. J. Econ. 117 (4), 1415e1451. Campbell, A., Converse, P.E., Miller, W.E., Stokes, D.E., 1960. The American Voter. Wiley and Sons, New York. Chen, J., 2012. Voter partisanship, and the effect of distributive spending on political participation. Am. J. Polit. Sci. 00 (0), 1e18. Cole, S., Healy, A., Walker, E., 2012. Do voters demand responsive governments? evidence from Indian disaster relief. J. Dev. Econ. 97 (2), 167e181. €rslag. Report. Crisis Commission, 2005. Sverige och tsunamin e granskning och fo SOU 2005:104, Stockholm. Flores, A.Q., Smith, A., 2013. Leader survival and natural disasters. Br. J. Polit. Sci. 43 (4), 821e843. Gasper, J.T., Reeves, A., 2011. Make it rain? retrospection and the attentive electorate in the context of natural disasters. Am. J. Polit. Sci. 55 (2), 340e355. Green, D.P., Palmquist, B., Schickler, E., 2002. Partisan hearts and minds: Political parties and the social identities of voters. Yale University Press, New Haven, CT. Guldåker, N., 2009. Krishantering, hushåll och stormen Gudrun: att analysera €rmåga och sårbarheter. Doctoral dissertation. Deparhushålls krishanteringsfo ment of Human Geography, Lund University, pp. 35e40. ISBN 978-91-9765216-2. Healy, A., Malhotra, N., 2009. Myopic voters and natural disaster policy. Am. Polit. Sci. Rev. 103 (3), 387e406. Healy, A., Malhotra, N., 2010. Random events, economic losses, and retrospective voting: implications for democratic competence. Q. J. Polit. Sci. 5 (2), 193e208. Holmberg, S., Oscarsson, H., 2004. V€ aljare: Svenskt v€ aljarbeteende under 50år. Norstedts Juridik, Stockholm. Jacobson, G.C., 2007. A Divider, Not a Uniter: George W. Bush and the American People. Longman, New York. Lay, J.C., 2009. Race, retrospective voting, and disasters: the re-election of C. Ray Nagin after Hurricane Katrina. Urban Aff. Rev. 44 (5), 645e662. Malhotra, N., Kuo, A.G., 2008. Attributing blame: the public's response to Hurricane Katrina. J. Polit. 70 (1), 120e135. Malhotra, N., Kuo, A.G., 2009. Emotions as moderators of information cue use: citizen attitudes towards Hurricane Katrina. Am. Polit. Res. 37 (2), 301e326. Manin, B., Przeworski, A., Stokes, S.C., 1999. Introduction. In: Przeworski, A., Stokes, S.C., Bernard, M. (Eds.), Democracy, Accountability and Representation. Aft, 2015. Aftonbladet's online archive. Retreived on January 5, 2015 from. http:// www.aftonbladet.se. € r Sverige. Retrieved on April 20, 2013 from. Alliansen, 2004, August 31. Allians fo http://www.alliansen.se. € r arbete. Retrieved on April 20, 2013 from. Alliansen, 2005, August 31. Program fo http://www.alliansen.se. €r stormf€ € dro €jer, 2005, September 12. Dagens Nyheter. Betalningen fo allt tra Retrieved on April 20, 2014 from. http://www.dn.se. Di, 2015. Dagens Industri's online archive. Retreived on January 5, 2015 from. http:// www.di.se. DN., 2015. Dagen Nyheter's online archive. Retreived on January 5, 2015 from. http://www.dn.se. Election Authority, 2013. Previous Elections: 2006 Riksdag Election. Retrieved on April 20, 2014 from. http://www.val.se. EM-DAT, 2013. Country Profile. Retrieved on April 20, 2014 from. http://www. emdat.be. Engman, T., 2005a, February 4. Analys: “Nu måste branchen s€ atta sig ner och prata”. Dagens Nyheter. Retrieved on April 20, 2014 from. http://www.dn.se. €gare. Dagens Nyheter. Engman, T., 2005b, March 2. Åkarei konflikt med skogsa Retrieved on April 20, 2014 from. http://www.dn.se. Exp, 2015. Expressen's online archive. Retreived on January 5, 2015 from. http:// www.expressen.se. Franchell, E., 2010, May 26. Myten om medelklassen. Aftonbladet. Retrieved on April 20, 2014 from. http://www.aftonbladet.se. n, J., 2006, April 1. Gudrun ger svår tja €llossning. Dagens Nyheter. Retrieved on Frede April 20, 2014 from. http://www.dn.se. Gatu, A., 2005, June 29. Skogsbr€ ander hotar efter storm. Dagens Nyheter. Retrieved on April 20, 2014 from. http://www.dn.se. €teborgsposten’s online archive. Retreived on January 5, 2015 from. GP., 2015. Go http://www.gp.se. €m, N., 2006, September 19. D€ €r fo €rlorade S regeringsmakten. SydsvensHallstro arfo kan. Retrieved on April 20, 2014 from. http://www.sydsvenskan.se. €kra leveranser. Dagens Karlsson, L., 2005, January 24. Lag ska tvinga elbolag att sa Nyheter. Retrieved on April 20, 2014 from. http://www.dn.se. €jade myndigheternas brister. SydsMagnusson, E., 2005, April 29. Stormen avslo venskan. Retrieved on April 20, 2014 from. http://www.sydsvenskan.se. Messing kritisk mot skogs€ agare, 2005, July 6. Dagens Nyheter. Retrieved on April 20, 2014 from. http://www.dn.se. MSB., 2013. Swedish Natural Hazards Information Systems. Retrieved on April 20, 2013 from. http://www.ndb.msb.se. €, B., 2005, May 9. Uppro €rda skogsa €gare chartrade protesttåg till Nord, K., Sjo Stockholm. Sydsvenskan. Retrieved on April 20, 2014 from. http://www. sydsvenskan.se. OECD., 2013. StatExtracts. Retrieved on April 20, 2013 from. http://www.stats.oecd. org. €r o €ver lagrådet, 2005, October 4. Dagens Nyheter. Retrieved on April Regeringen ko 20, 2014 from. http://www.dn.se. € jning efter Gudrun o €kade antalet do €da i arbetsolyckor, 2006, March 24. SydsRo venskan. Retrieved on April 20, 2014 from. http://www.sydsvenskan.se. SCB., 2013. Statistics Sweden's Database. Retrieved on April 20, 2014 from. http:// I would like to thank the anonymous reviewers of this paper, Kåre Vernby, Charles Parker, Jens Hainmueller, Sarah J. CormackPatton, Sven Oskarsson, Katrin Uba, Anders Lindbom, Li Bennich€rkman, Pa €r Nyman, Johanna So € derstro € m, Ari Tryggvason, Tito Bjo Maldonado, Patrick S. Roberts and Sebastian Lundmark for helpful comments on previous drafts of this paper. I also thank Sven Halldin, Adam Dingwell, Gunnar Berglund, Stina Haraldsson, Frederike Albrecht and Berit Eriksson for helpful assistance with data collection. This research has been funded by Uppsala University, Center for Natural Disaster Science (CNDS), the Fulbright Commission and Skytteanska. Smaller travel grants for presenting earlier drafts of this paper at workshops and conferences have generously been provided by the Erik Borbos Hansson scholarship. Appendix A. Supplementary data 142 L.M. Eriksson / Electoral Studies 41 (2016) 129e142 www.scb.se. SMHI., 2013. Gudrun e januaristormen 2005. Retrieved on April 20, 2013 from. http://www.smhi.se. Sprickor i stormf€ allt virke, 2006, January 14. Dagens Nyheter. Retrieved on April 20, 2014 from. http://www.dn.se. SvD, 2015. Svenska Dagbladet's online archive. Retreived on January 5, 2015 from. http://www.svd.se. SVT., 2015, January 7. Rapport, 7.30pm. Retrived on January 8, 2015 from. http:// www.svtplay.se. Sweden Democrats, 2013. Vårt parti: Valresultat. Retrieved on April 20, 2013 from. http://sverigedemokraterna.se. Swedish Forest Agency, 2013. Statistics. Retrieved on April 20, 2013 from. http:// www.skogsstyrelsen.se. SYD., 2015. Sydsvenskan's online archive. Retreived on January 5, 2015 from. http:// www.sydsvenskan.se. €rbring, G., 2006, March 6. All skog ska återplanteras efter stormen “Gudrun”. So Dagens Nyheter. Retrieved on April 20, 2014 from. http://www.dn.se. €stlo € st en månad efter stormen, 2005, February 7. Dagens Nyheter. Retrieved on Tro April 20, 2014 from. http://www.dn.se. €rnberg, U., 2005a, May 9. Elupproret sa €tter press på Sydkraft. Sydsvenskan. To Retrieved on April 20, 2014 from. http://www.sydsvenskan.se. €rnberg, U., 2005b, December 28. Ny skogsplantering får kritik. Sydsvenskan. To Retrieved on April 20, 2014 from. http://www.sydsvenskan.se. UI., 2012. Landguiden: Sverige, Modern historia. Retrieved on April 20, 2012 from. http://www.landguiden.se. €s, M., 2006, January 4. Stor sorg efter stormens ha €rjningar. Dagens Nyheter. Ålsna Retrieved on April 20, 2014 from. http://www.dn.se. €nder, 2005, January 30. Dagens Nyheter. Retrieved on 500 mil elkabel fortfarande so April 20, 2014 from. http://www.dn.se.