Understanding Diagnostic Measurements PDF
Document Details
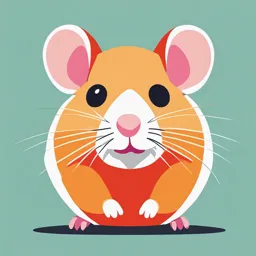
Uploaded by MagicBixbite
Tags
Summary
This document discusses different types of measurements used in diagnostics, including direct and indirect measurements. It explains how standard curves are used to determine analyte concentrations and provides examples of clinical applications, such as diagnosing anemia and cholesterol levels.
Full Transcript
PROPRIETARY. DO NOT SHARE. Transcript: Understanding Diagnostic Measurements Section 1: Introduction to Measurements Welcome Welcome to our course Understanding Diagnostic Measurements. This course will be divided into 3 sections: Types of Measurements, Variability of Measurements, and Accuracy of...
PROPRIETARY. DO NOT SHARE. Transcript: Understanding Diagnostic Measurements Section 1: Introduction to Measurements Welcome Welcome to our course Understanding Diagnostic Measurements. This course will be divided into 3 sections: Types of Measurements, Variability of Measurements, and Accuracy of Measurements. In this first section, we will introduce you to the concept of direct and indirect measurements. These measures are used to determine if a new diagnostic is better, worse, or just as good as the “Gold Standard diagnostic” currently being used. Let’s learn more. Section 1: Introduction to Measurements Objectives By the end of this section, you should be able to: • Define the term Gold Standard diagnostic and explain its importance. • Compare and contrast direct and indirect measurements and give an example of each. • Discuss why standard curves are used as a diagnostic measurement. • Give an example of when a standard curve is used in a diagnostic, and explain what results it provides. The Gold Standard A diagnostic test is only as good as its value in telling the truth about a person's medical condition. In practice, this value is measured against a gold standard, a diagnostic test, or a benchmark that is the best available under reasonable conditions. In some cases, this may mean that the gold standard test is an indirect measurement. For example, in medicine, dealing with conditions that require an autopsy to have a perfect diagnosis, the gold standard test is an indirect measurement that is less accurate than an autopsy. In clinical studies, direct measures are often referred to as clinical endpoints, whereas indirect measures are referred to as surrogate endpoints. Both types of measurements can be useful when properly applied. When new technologies introduce new diagnostic tests, they must be compared to an existing gold standard to be accepted. For example, molecular diagnostics such as PCR, which is now frequently used to identify or confirm the presence of a particular infectious agent became accepted only after being compared favorably to the gold standard tests of bacterial culture and ELISA assays. Examples of indirect measurements used as diagnostics include the arm 1 Copyright 2023 Biotech Primer, Inc. PROPRIETARY. DO NOT SHARE. pressure cuff, which is used instead of an invasive arterial pressure catheter, an abnormal EKG, which measures myocardial infarction, mammogram density test to predict abnormal biopsy, and urine tests to detect elevated levels of pregnancy hormone as the pregnancy test. Measures In a direct measurement, the desired information is assessed directly. Examples include measurements of height, weight, and waist circumference. In these examples, our measuring device, a meter stick, scale, and flexible measuring tape, respectively, provide us with quantitative data that directly answers the question we are asking. Indirect measurements are used when direct measurement is not possible or is deemed too invasive. In an indirect measurement, the measurement enables a diagnostic practitioner to make an inference about the presence or absence of a particular condition. For example, a high cholesterol level in and of itself is not a disease state. However, decades of clinical research have informed physicians that high cholesterol levels lead to atherosclerosis, or the narrowing of blood vessels due to the accumulation of cholesterol and other fatty materials. This in turn can lead to heart attacks and strokes. Accordingly, high cholesterol levels are now accepted as an indirect measurement or predictor of cardiac disease. Anemia is a disorder that develops when there is not enough oxygen-carrying protein hemoglobin, present in a patient's blood. Symptoms include feeling tired, difficulty breathing, dizziness, headache, and feeling cold. Since hemoglobin is found in red blood cells, a patient presenting with these symptoms would most likely have a red blood cell count taken. If the number is lower than average, a diagnosis of anemia may be appropriate. In this case, red blood cell count is an indirect measurement of hemoglobin levels. Likewise, an increase or decrease in tumor size can be used to infer cancer progression or response to treatment. Determining Unknowns Almost everyone has had the experience of going to the doctor and having a tube of blood drawn. The purpose of a blood draw is often to determine the concentration of a specific substance in the patient's blood, such as glucose or a particular hormone. But how can we determine this from such a small sample? It turns out that it's not all that different from guessing the number of jellybeans in a jar, if we know the average weight of a jellybean, and are allowed to weigh the jar. 2 Copyright 2023 Biotech Primer, Inc. PROPRIETARY. DO NOT SHARE. Constructing A Standard Curve How many jellybeans are in this jar? With no background knowledge, it can be challenging to guess. However, if we can construct a standard curve, the task becomes more manageable. To construct a standard curve, we add increasing numbers of jellybeans, 10, 20, 30 and so on to a series of jars. We then weigh each jar and graph the results to determine the relationship between jellybean weight and the number of jellybeans. This information could then be used to predict the number of jellybeans in a jar containing an unknown quantity. Standard Curve The graph that we construct would look something like this and is called a standard curve. There is a linear relationship between jellybean weight and the number of jellybeans. Suppose that someone now hands us a jar of jellybeans and asks us to determine the number of beans contained in the jar. We can quite accurately estimate the correct number if we are allowed to weigh the jar. Suppose that our jar weighs 55 grams, we then simply need to look at our standard curve and note that the weight corresponds to 42 jellybeans. This is certainly a much easier technique than emptying the jar and counting the jellybeans. Colorimetric Unknown This idea of a standard curve is very important for a whole range of diagnostics. One of the most important applications is in the use of colorimetric assays. A colorimetric assay is a method of determining the concentration of a chemical element, chemical compound, or protein in a solution with the aid of a color reagent. Here's the basic idea, the detection reagent is added to a solution containing the specific chemical or protein known as the analyte that we are interested in detecting. If the chemical or protein is present, a color change occurs. If it is not present, no color change occurs. Furthermore, as the concentration of the analyte increases, so too will the intensity of the color change observed. Standard curves are constructed from samples containing a known amount of analyte to correlate the degree of color change with a known analyte concentration. The color reagent is then added to samples of unknown concentration. We can then use the standard curve to infer analyte concentration in an unknown sample, based on the degree of color change. Again, the substance that is being detected in the sample is known as the analyte. For example, cholesterol is an analyte in the blood, as is iron. 3 Copyright 2023 Biotech Primer, Inc. PROPRIETARY. DO NOT SHARE. Section 1: Introduction to Measurements Summary In this section, we learned: • A diagnostic gold standard is the best available test for determining if a patient does or does not have a disease. Any new diagnostic must meet or exceed the capability of the Gold Standard to achieve regulatory approval. • In a direct measurement, the desired information is assessed directly. Examples include measurements of height and weight. • In an indirect measurement, the desired information is inferred and not directly measured. For example, a high cholesterol level correlates to stroke due to the accumulation of cholesterol in the arteries. • A standard curve allows researchers to accurately estimate the concentration of analytes in solution. This same curve can be used to identify unknowns. • One of the most important applications is in the use of colorimetric assays. A colorimetric assay is a method of determining the concentration of a chemical element, chemical compound, or protein in a solution with the aid of a color reagent. 4 Copyright 2023 Biotech Primer, Inc. PROPRIETARY. DO NOT SHARE. Section 2: Variability of Measurements In this section, we will learn how test distributions directly impact the chances of novel diagnostics receiving regulatory approval. Let’s take a closer look. Section 2: Variability of Measurements Objectives By the end of this section, you should be able to: • List causes of variability in diagnostic measurement; • Discuss what happens if there is not a bi-model curve; • Explain why analytes associated with disease follow a bi-model distribution; and • Describe the advantage of a bi-model curve. Variability of Measurements The heart of diagnostics can be considered to be measuring key health variables and determining if they fall within the normal range of a given population or not. It is important to keep in mind that there are a number of factors, which could cause variations in measurement. The instrument itself could be inaccurate. Even something as simple as a ruler or a scale may be slightly off, resulting in variations from measurement to measurement. Certainly, more complex machinery such as photometers used to measure light may also be prone to slight variations. Whenever we are talking about human populations, there will be some significant degree of variation on most variables, all within the normal range. Another common source of variation is sampling. Suppose you want to determine the average height of people in the United States. If you only measured NBA players, your results would be much different than if you measured anyone who passed through New York Central Station. Measurement & Distribution These variations in measurement result in a distribution of values. Good diagnostic design of both equipment and test will ensure that measurement variation is very small compared to variation in what is being measured. But this will still often leave us with some variation due to inherent variation of measurement, population, and sampling. 5 Copyright 2023 Biotech Primer, Inc. PROPRIETARY. DO NOT SHARE. Graphical Display of Distributions Each of these graphs shows a distribution of height for a given sample of men. The sample size for each graph differs. The first graph samples only 100 people, while the sample size of each subsequent graph increases. As we increase the total number of subjects measured, we get a more even distribution of heights, a more representative reflection of the population. If our scientists measure enough people, the graph becomes a smooth curve, showing the normal distribution of height in the population. Looking more closely at the last graph, we see that 68% of the men measured fall within a relatively narrow height range, with 95% falling within a broader range. The outliers, those whose height corresponds to less than 5% of the population are at the tail end of the curve on either side. Extreme outliers correspond to less than 0.3% of the population. In the next several slides, we will be looking at similar curves, representing variation in different disease-associated measurements across the population. Measurement & Distribution In the previous slide, we saw that 95% of the population fell within a certain range of height measurements. 99.7% fell within a broader range. Depending on our criteria, we might consider someone who falls outside of that 95% range as being either abnormally tall or abnormally short. Or we may decide to classify only those beyond the 99.7% cutoff as abnormal. Where we draw that line, shown here as a green cut-off mark, will determine what we consider abnormal or not. Bi-Modal Distribution One challenge of population measurements is a bimodal distribution. In statistics, the mode for a set of data is the value that appears most often. In this curve of analyte concentration versus percent of the population, the mode is simply the peak of the curve. The peak of the curve corresponds to the analyte concentration that appears most often in a given population. Recall that an analyte is simply the biomarker, such as blood cholesterol, that is being measured. Many analytes associated with disease follow a bimodal distribution. That is, they have two different modes in a population sample with one mode corresponding to healthy people, and the other mode corresponding to people with a particular disease. This can introduce challenges in differentiating the diseased population from the healthy population. 6 Copyright 2023 Biotech Primer, Inc. PROPRIETARY. DO NOT SHARE. Cholesterol Cholesterol is an analyte strongly correlated with cardiac disease. However, in any population, there will be a wide range of values for milligrams of cholesterol per deciliter of blood, as shown in this graph. Cholesterol in The Prediction of Atherosclerotic Disease The question then becomes, where do we draw the line between healthy individuals and those who are at risk for coronary disease? Decades of clinical data give us some guidance as to where that line should be, but the distinction is still not necessarily clear-cut. Cholesterol exhibits bimodal distribution, just as we saw for blood pressure. The mode for the diseased population, shown here in red, corresponds to a higher level of blood cholesterol than the mode for the non-diseased population, shown in blue. However, there is a significant overlap in cholesterol levels between the two populations, making it difficult once again to draw a clear cutoff between diseased and non-diseased. Bi-Modal Distribution To review, what we often see is a bimodal distribution with a great deal of overlap between healthy and diseased populations. Such bimodal distributions make it challenging to accurately differentiate diseased from healthy. Ideal Distribution of a Test What we would rather see is something with a clear separation of the diseased group from the healthy group with no overlap between the two curves of analyte concentration. Unfortunately, this type of perfect separation rarely exists. Nearly Ideal Distribution of a Test Typically, the best that we can hope for is this nearly ideal distribution, where there is minimal overlap in analyte values between the diseased and healthy groups. Even in this nearly ideal case, however, we see that our cutoff line between healthy and diseased will misclassify some people, a concept that we will expand upon in the next section of the course. 7 Copyright 2023 Biotech Primer, Inc. PROPRIETARY. DO NOT SHARE. Section 2: Variability of Measurements Summary In summary: • Several factors create variability that must be taken into account when developing a diagnostic, including variation in instrument results, variation in measurements, variation among patients, and variation in population sampling. • If the modes overlap, the diagnostic will not be able to determine who is healthy and who has the disease. • Many analytes associated with disease follow a bi-modal distribution because they have two different modes in a population sample. One mode corresponds to healthy people, and the other mode corresponds to people with a particular disease. • The advantage of a bi-model curve, that does not overlap, is that a diagnostic can determine who is healthy and who has the disease. 8 Copyright 2023 Biotech Primer, Inc. PROPRIETARY. DO NOT SHARE. Section 3: Accuracy of Measurements In this last section, we will use our knowledge of standard curves, distribution, and modes to understand diagnostic concepts such as false positives and false negative results. We will explore the concepts of sensitivity, specificity, precision, and positive predictive value. All of these measurements are used to determine if a new diagnostic is worthy of regulatory approval. Section 3: Accuracy of Measurements Objectives At the end of this section, you should be able to: • Distinguish between diagnostics accuracy and precision. • Define false positive and false negative diagnostic results. • Explain why false positives and false negatives occur. • Compare and contrast specificity with sensitivity. • Explain why the positive predictive value of a diagnostic is a critical measurement. Measurement Considerations Ideally, the result of the analyte measurement will be a number. This makes the values between groups or individuals, or between the same individual at different times much easier to compare. All measurements will have sample and instrument variability. The goal is to minimize that variability as much as possible. Finally, we must compare a measurement's accuracy, the degree of closeness of measurements of the quantity to that quantity's actual value with the value that is achieved from measurements obtained using a gold standard diagnostic. For clinical practice, we need a yes or no answer to remove as much ambiguity as possible from the diagnostic process. A big part of diagnostic development will simply be ensuring that we are measuring the right analyte, something that will give us a clear-cut indication as to whether or not a diseased state is present. Again, minimizing variability in measurement will be critical to clinical use, as will selecting the optimum cutoff to differentiate between diseased versus healthy patients. What Is The “Accuracy” Of A Test? Let's take a moment here to more clearly define the accuracy of a test. This is a useful concept for any test that gives a numerical result and can be defined as the statistical difference between 9 Copyright 2023 Biotech Primer, Inc. PROPRIETARY. DO NOT SHARE. the result and the reference value, or the result and the gold standard. Concepts related to accuracy include precision and bias. Precision simply means, how repeatable are your results. If we test the same sample many times, how much do the results vary? Bias is a measure of how close our measurements are to the gold standard. A significant degree of variance between test results is usually due to random error. Bias, on the other hand, can be traced to a system error, indicating that we need a better method of measurement. To visualize these concepts, we can liken our diagnostic to throwing darts at a target. The best diagnostic would be both unbiased and precise. We would hit the bullseye, the gold standard, every time. We may, however, have a test that is very precise, all the darts are close together but biased, and they are not on the bullseye. Or, we could have a test that is unbiased, the darts are evenly distributed around the bullseye, but imprecise, their spread is quite wide. Worst of all is a test that is biased, all the darts are land ow, and imprecise, they are not close together. False Positive & Negative For many diagnostic tests, there may be issues of either false positives or false negatives. A false positive occurs when someone who doesn't have a disease or condition tests positive for that disease or condition. A false negative then is the opposite, someone who does have the disease, but tests negative for it. If we go back to our graph of analyte concentration, we can see why these issues occur. Here we have the peak illustrating the distribution concentrations of a particular analyte in a normal, non-diseased population. If we overlay our graph of the same analyte concentration in a diseased population, we see that there is some small overlap of the two curves, with the cutoff for the diagnosis occurring in the middle of the overlap. It is in this range then that false positives and false negatives may occur, with false positives appearing when someone without the disease has an analyte concentration that falls to the right of the cutoff point, and a false negative occurring when someone with the disease has an analyte concentration that falls to the left of the cutoff point. Thinking back to our blood pressure and cholesterol curves, we can see that if either of those parameters were our sole measure of cardiac disease diagnosis, we could have significant numbers of false positives and false negatives. 10 Copyright 2023 Biotech Primer, Inc. PROPRIETARY. DO NOT SHARE. Sensitivity and Specificity Two very important measures of the worth of a diagnostic test are its sensitivity and specificity. A test’s sensitivity is essentially a measure of its ability to correctly identify all individuals who have a disease. Are sick people being correctly diagnosed as sick? We can liken this to finding a needle in a haystack. Specificity is the flip side of sensitivity. Specificity is the test's ability to correctly identify healthy people. In other words, to reject the wrong answer. For most diagnostic tests, there is inevitably a trade-off between sensitivity and specificity, depending on where we draw the cutoff line between diseased and healthy. Only in the case where the analyte peaks are separated with no overlap between the diseased versus healthy curves, would one be able to achieve both very high specificity and very high sensitivity. For most diagnostic tests, 99% sensitivity, in other words, the test can correctly identify 99 out of 100 individuals who have the disease, is considered very good. Sensitivity - HIGH Once again, we see here our curves representing analyte concentrations in diseased and healthy populations. Remember, the area under each curve represents all people with, or without, a particular disease and the blue line indicates the cutoff point for diagnosis. This can also be represented in a chart, where A represents the people who have the disease and test positive, B represents the people who don't have the disease and test positive, essentially the false negatives. C represents people who have the disease but test negative, also known as false negatives, and finally, D represents people who don't have the disease and correctly test negative. If we want to calculate the sensitivity of a particular test, we simply take the number of people who have the disease and were correctly diagnosed as having the disease, A, and divide that by all the people who have the disease, A plus C. This calculation will give us the percentage of people with the disease, who were correctly diagnosed as having the disease. 99% specificity, rejecting 99 out of 100 of the people who do not have a disease, is also considered very good. In order to calculate specificity, we simply take the number of people who do not have the disease and who also received a negative test result, D, and divide that by the total number of people who do not have the disease, B plus D. 11 Copyright 2023 Biotech Primer, Inc. PROPRIETARY. DO NOT SHARE. For many tests, it may be nearly impossible to have both high sensitivity and high specificity, although, of course, that is ideal. Moderately good sensitivity and specificity are considered to be in the range of 60%. The analyte concentration curves shown in this slide illustrate the degree of overlap between healthy and diseased populations for this level of sensitivity and specificity. To review, sensitivity is determined by dividing all the people who have the disease and test positive for it, A, by all the people who have the disease, A plus C. The goal of sensitivity is to find everyone with the disease. Specificity is calculated by dividing all the people who don't have the disease and test negative, D, by all the people who don't have the disease, B plus D. Specificity is a measure of how well the test rejects everyone who does not have the disease. Example: Airport Screening An example of a high-sensitivity, low-specificity type of screening that most of us are all too familiar with is airport security. To potentially find the needle in the haystack, the one individual out of millions who may potentially be attempting to bring a weapon onboard an airplane, many, many people are needlessly searched. The result is that most weapons are found, but many people are subjected to unnecessary searches. Example: Drunk Driving Arrests The opposite scenario is true for the low sensitivity, high specificity drunk driving test. Because DUI checkpoints are relatively rare, people are usually not subjected to a sobriety test, unless their driving is erratic, and even erratic driving may not be flagged if there are no official observers present. Thus, many people who drive with a blood alcohol concentration over the legal limit will not get arrested. However, almost all who are arrested are intoxicated, illustrating high specificity and low sensitivity. Review of Sensitivity & Specificity To summarize these important concepts, sensitivity means identifying everyone who has the disease, while specificity means rejecting everyone who does not have the disease. If a diagnostic achieves 99% in either of these measures, it is considered very good. 12 Copyright 2023 Biotech Primer, Inc. PROPRIETARY. DO NOT SHARE. True Positive, True Negative Very often we hear the terms true positive and true negative. What do these mean? True positives are the patients who have the disease and are correctly identified as having the disease. Going back once again to our analyte concentration curves, we see that we can identify the true positives as A, everyone who has the disease and was diagnosed as having the disease, divided by A plus B. Or everyone who has the disease and was diagnosed as having the disease, plus everyone who was healthy, but was misdiagnosed as having the disease. So, we are identifying the percentage of people who were correctly identified as having the disease. True negatives then are essentially the inverse, people who were correctly identified as not having the disease. On our graph, we can represent that group by dividing D, the number of healthy people not diagnosed with the disease by D plus C, the number of healthy people not diagnosed with the disease, combined with the number of sick people not diagnosed. True positives and true negatives are usually expressed as a number of true positives or true negatives, per a specific number of clinical samples. False Negatives, False Positives A related concept is that of false negatives and false positives. A false negative means that someone has the disease, but the diagnostic test indicates that they don't. False negatives are also known as type two errors and can be calculated by dividing the number of diseased people identified as healthy, C, by the number of diseased people identified as healthy plus the number of truly healthy people, C plus D. Finally, a false positive is simply a healthy person who was identified as having the disease. Also known as a type one error, false positive rates can be calculated by dividing the number of incorrectly diagnosed people, B, by the number of incorrectly diagnose people plus the number of correctly identified sick people, B plus A. Positive Predictive Value, Negative Predictive Value Another important statistical concept is predictive value. The positive predictive value of a test is the proportion of positive test results that are true positives, i.e., the correct diagnosis. It is a critical measurement of the performance of a diagnostic method, as it relates to the probability that a positive test reflects the underlying condition being tested for. In order to calculate the positive predictive value as a percentage, the number of true positives A is divided by the 13 Copyright 2023 Biotech Primer, Inc. PROPRIETARY. DO NOT SHARE. number of total positives, A plus B. This is a measurement of how predictive a positive test is of the patient actually having the disease. If a test has a low positive predictive value, for example, 10%, this indicates that many of the positive results from this testing procedure are false positives. Thus, it will be necessary to follow up any positive result with a more reliable test to obtain a more accurate assessment as to whether the disease is present. Nevertheless, such a test may be useful if it is inexpensive and convenient. Negative predictive value is defined as the proportion of subjects with a negative test result who are correctly diagnosed. The negative predictive value of a particular test can be calculated by dividing the number of true negatives, D, by the number of total negatives, C plus D. Negative predictive value is a measurement of how predictive a negative test is, of the patient actually not having the disease. A high negative predictive value for a given test means that when the test yields a negative result, it is most likely correct in its assessment. In the context of medical testing, a high negative predictive value means that the test only rarely misclassifies a healthy person as being sick. Issues with Screening Tests Diagnostic Screening is not without risk. This is especially true in screening for low-prevalence diseases. Low-prevalence disease Screening typically has poor predictive values, as will be discussed in the upcoming slides, and therefore lead to unnecessary invasive procedures to confirm the diagnosis. Let's return to our analyte concentration curves of a healthy and diseased population. Low Prevalence Even in an ideal test that very effectively separates healthy from diseased, there is still some overlap. At any given cutoff, some people with the disease will have low analyte levels, and some people without the disease will have high analyte levels. If the disease is fairly common in the population, as shown here, then the number of false positives relative to the number of true positives remains relatively low. 14 Copyright 2023 Biotech Primer, Inc. PROPRIETARY. DO NOT SHARE. As the disease prevalence decreases further, we see an even greater percentage of false positives during diagnostic screening. If the disease prevalence gets low enough, we can see that the number of false positives is actually larger than the number of true positives. In this low-prevalence disease example, the number of true positives is roughly equal to the number of false positives. Therefore, a person with a positive test result only has about a 50% chance of having the disease. This is a low positive predictive value. Many Diseases Are Much Worse Even if the test is fairly specific and sensitive, the positive predictive value is poor, and therefore the use of this test for screening the general population should be considered carefully. For diseases that are even less prevalent, the predictive value may be as low as 5%. In general, we can conclude that the diagnostic tests for low-prevalence diseases are likely to have a low positive predictive value. Low Prevalence Diseases This then means that for diseases that have a low prevalence in the general population, there is a high chance of false positives in any type of generalized screening. This low predictive value means that even with a positive result, there could still be a chance that the patient does not have the disease. Why is this a problem? Isn't it better to err on the side of caution by identifying anyone who might have a disease rather than miss a chance at early diagnosis of those who do? The problem lies in the reality that false positives lead to unnecessary, often invasive procedures which are not only expensive but can cause real duress to patients, both psychological and physical. In order to minimize these potential negative results of low prevalence disease screening, guidelines typically recommend limiting the test to higher prevalence populations, such as mammograms only for women over 40 and prostate-specific antigen only for men over 50. Mammography What exactly is mammography and how good is it? Mammography is quite simply the X-ray examination of breast tissue for the early detection of cancer. It does this by detecting abnormal 15 Copyright 2023 Biotech Primer, Inc. PROPRIETARY. DO NOT SHARE. density within the tissue. On average, a mammogram has a sensitivity of 85%, meaning that it correctly identifies on average, 85% of patients who do have breast cancer. The average specificity is 90%, meaning that 90% of the time on average, it correctly classifies patients who do not have breast cancer. This translates to an approximate false positive, false negative rate of 7% and 20%, meaning that 7% of women will have a mammogram indicating cancer when they do not have cancer, and 20% of women will have a mammogram indicating no cancer when they do have breast cancer. This equates to a positive predictive value of only 6% to 8%. Mammogram Example What do these rates mean in terms of actual patient numbers? Looking first at false positives, we see that if 1,000 women are screened, on average 930 will be deemed healthy and 70 referred for further examination. Of the 70 referred for further testing, on average 60 will turn out to have a benign explanation for the tissue density observed in the mammogram, while 10 will be referred for biopsy. Of the 10 referred for biopsy, on average, only 3.5 will actually have cancer. Of the 3.5 who have cancer, two of the patients will have cancer that can be treated and cured due to early Looking next to false negatives, we see that the numbers here are not great either. On average, 350 out of 100,000 women have breast cancer. Mammography typically misses between 10% to 30% of those cases. Mammogram: Annual Exams Let's look at published results for annual mammogram screening. If 1,000 women in their 50s are Screened annually for 10 years, the following results would be expected. One woman's life will be extended due to early detection, and two to 10 women will be over-diagnosed and needlessly treated for slow-growing cancer that would not have harmed the patient. Five to 15 women will be treated for breast cancer with no change in outcome, in comparison to waiting for symptoms to appear. 250 to 500 women will have a false positive, being told that they might have breast cancer and therefore need further screening. 125 to 250 of the false positives will undergo breast biopsy. The numbers vary slightly according to the age of the population being screened. As women age, the positive predictive value of mammograms becomes better, but it's still a relatively low prevalence disease with correspondingly low predictive values and resulting 16 Copyright 2023 Biotech Primer, Inc. PROPRIETARY. DO NOT SHARE. needless biopsies. To prevent one death from breast cancer, 2,000 women in their 40s would need to be screened, resulting in 1,000 false positives, and 250 unnecessary biopsies. 1,339 women in their 50s would need to be screened, with 670 false positives and 300 unneeded biopsies. 377 women in their 60s would need to be screened, with 180 false positives and 150 needless biopsies. Of course, these numbers reflect the general population. Women with a family history of breast cancer are more likely to benefit from annual Screening. Current guidelines on mammography screenings are contradictory. US Preventive Services Task Force mammogram guidelines recommend women begin screening at age 50 and repeat the test every two years. The American Cancer Society and other organizations recommend screenings begin at 40 and continue annually. Ideally, a better diagnostic that results in a better predictive value will be developed. PSA Testing for Prostate Cancer Let's now turn our attention to another type of common cancer screening, PSA testing for prostate cancer. Prostate cancer is the second most common cancer in men and the second leading cause of cancer death. Annually, one in six men is diagnosed with the disease, and one in 36 will die from it. PSA stands for prostate-specific antigen. PSA is an enzyme that is secreted by all prostates, however, when cancer is present, it is secreted at higher levels. It is these higher levels that PSA Screening is meant to detect. There are currently 26 different PSA tests on the market. However, the US Preventive Services Task Force now recommends that healthy men avoid getting regular PSA tests. Why is that? PSA Screening Controversy PSA testing has a high false positive rate due to the poor specificity of the test. Remember, all men secrete PSA. It is just a question of how much and as we saw with our earlier analyte curves, determining where to draw that cutoff line between diseased and non-diseased populations can be tricky. PSA levels may also be elevated in men with prostatitis, or benign prostatic hyperplasia. Elevated levels of PSA lead to prostate biopsies. Four out of five biopsies are negative, and there is a significant risk of secondary issues, including rectal bleeding, which can lead to potentially a drug-resistant infection as a result of the biopsy. And of the one in five cancerous 17 Copyright 2023 Biotech Primer, Inc. PROPRIETARY. DO NOT SHARE. biopsies, most turn out to be low-grade, nonaggressive cancer with the patient likely to survive another 10 to 20 years with no ill effect due to the disease. New Prostate Cancer Biomarkers Needed Scientists have been searching for a different prostate cancer biomarker to replace PSA, but today none has been found. There is some hope that further analysis of PSA can increase the test’s predictive value. New research shows that the PSA story is more complex than previously thought. Most PSA in the blood is bound to proteins. A small amount is not protein bound, and it's called free PSA. In men with prostate cancer, the ratio of free, unbound PSA to total PSA is decreased. The risk of cancer increases if the free-to-total ratio is less than 25%. The lower the ratio is, the greater the probability of prostate cancer. Measuring the ratio of free to total PSA appears to be particularly promising for eliminating unnecessary biopsies. Other variations include the activity level of the enzyme, with an increased risk of cancer associated with decreased enzyme activity. As these stratifications are increasingly incorporated into new PSA tests, we can expect to see their predictive value increase. While a replacement for PSA has not been found, researchers have discovered an additional biomarker that can be used in conjunction with PSA testing for Main Topic for prostate cancer. Gen-Probe's PCA3 test detects increased levels of the non-coding RNA PCA3. PCA3 is highly over-expressed in prostate cancer, meaning there is too much of it in the cell, and thus is a more specific biomarker than PSA. Currently, the PCA3 test is only approved to help determine the need for repeat prostate biopsies in men who have had a previously negative biopsy. Eventually, however, widespread use of the PCA3 test in combination with PSA testing may do much to improve the specificity of prostate cancer screening. Invasive Procedures A September 2011 issue of Consumer Reports included a piece on diagnostic testing. An interview with Dr. Steven Nissen highlighted some of the issues involved. These include wasted resources, invasive procedures, and potentially inappropriate treatments. A worst-case scenario highlighted the story of a 52-year-old nurse whose false positive results on a heart scan led to unnecessary surgeries and a result in complications leading to a heart transplant. Despite these potential pitfalls, public support for diagnostic screening remains strong. 87% of the 8,000 18 Copyright 2023 Biotech Primer, Inc. PROPRIETARY. DO NOT SHARE. subscribers surveyed agreed that they would rather have a diagnostic scare that turns out to be nothing than forego testing. Section 3: Accuracy of Measurements Summary Let’s review the main points in this section. • Accuracy is the statistical difference between the result of the new diagnostic and the result of the gold standard diagnostic. Precision is when the diagnostic results received are repeatable. • A false positive occurs when someone doesn't have a disease, but tests positive for that disease. A false negative is when someone has the disease, but tests negative for it. • False positives and false negatives occur when there is an overlap of the healthy and disease standard curves. • Sensitivity means identifying everyone who has the disease. Specificity means rejecting everyone who does not have the disease. • The positive predictive value of a diagnostic test is the proportion of positive test results that are true positives, meaning the diagnostic made the correct diagnosis. It is a critical measurement of the performance of a diagnostic method. 19 Copyright 2023 Biotech Primer, Inc.