DWDM Unit 1.pdf
Document Details
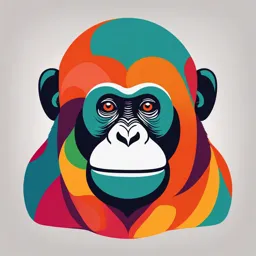
Uploaded by EffectualErudition587
Tags
Full Transcript
UNIT 1 The Data-Information-Knowledge-Wisdom (DIKW) pyramid illustrates the progression of raw data to valuable insights. It gives you a framework to discuss the level of meaning and utility within data. Each level of the pyramid builds on lower levels, and to effec...
UNIT 1 The Data-Information-Knowledge-Wisdom (DIKW) pyramid illustrates the progression of raw data to valuable insights. It gives you a framework to discuss the level of meaning and utility within data. Each level of the pyramid builds on lower levels, and to effectively make data-driven decisions, you need all four levels. Wisdom is the ability to make well-informed decisions and take effective action based on understanding of the underlying knowledge. Knowledge is the result of analyzing and interpreting information to uncover patterns, trends, and relationships. It provides an understanding of "how" and "why" certain phenomena occur. Information is organized, structured, and contextualized data. Information is useful for answering basic questions like "who," "what," "where," and "when." Data refers to raw, unprocessed facts and figures without context. It is the foundation for all subsequent layers but holds limited value in isolation. Everyday Life Example: Fitness tracking Fitness tracking devices collect your health and activity data, but your end goal is to use that to make decisions about how to train or how to manage your health. Wisdom: Understanding these patterns lets you make informed decisions about adjusting your exercise routine, sleep habits, and other lifestyle factors to improve your health and fitness. Knowledge: Analyzing and interpreting the information may reveal patterns, such as increased step count leading to improved sleep quality or a correlation between heart rate and workout intensity. Information: The smartwatch app organizes and structures the data, displaying it in a comprehensible format, such as daily step count, average heart rate, and hours of sleep per night. Data: The smartwatch collects raw data such as the number of steps taken, heart rate, and sleep duration. Product Example: Mobile App Development Mobile apps collect user data, and you can get additional feedback data from users, but the product manager's end goal is to make decisions to improve the app. Wisdom: Understanding user needs, preferences, and pain points lets the product manager make informed decisions to prioritize feature development, enhance user experience, and allocate resources effectively to maximize user satisfaction and retention. Knowledge: Analyzing and interpreting information from app usage and user feedback uncovers patterns such as frequently requested features, causes of user frustration, and key factors driving user engagement and loyalty. Information: App usage and user feedback data is organized and structured, providing metrics like average session duration, feature usage frequency, user retention rates, and common feedback themes across user segments. Data: The raw data consists of individual user interactions with the app, such as button clicks, screen views, and time spent in the app, as well as user-submitted feedback through reviews, surveys, and support tickets. What is business intelligence? Business intelligence combines business analytics, data mining, data visualization, data tools and infrastructure, and best practices to help organizations make more data-driven decisions. In practice, you know you’ve got modern business intelligence when you have a comprehensive view of your organization’s data and use that data to drive change, eliminate inefficiencies, and quickly adapt to market or supply changes. Modern BI solutions prioritize flexible self- service analysis, governed data on trusted platforms, empowered business users, and speed to insight. What is a Data Warehouse? A data warehouse, also called an enterprise data warehouse (EDW), is an enterprise data platform used for the analysis and reporting of structured and semi-structured data from multiple data sources, such as point-of-sale transactions, marketing automation, customer relationship management, and more. Data warehouses include an analytical database and critical analytical components and procedures. They support ad hoc analysis and custom reporting, such as data pipelines, queries, and business applications. They can consolidate and integrate massive amounts of current and historical data in one place and are designed to give a long-range view of data over time. These data warehouse capabilities have made data warehousing a primary staple of enterprise analytics that help support informed business decisions. The Architecture of Bl A BI system has four major components: a data warehouse, with its source data; business analytics, a collection of tools for manipulating, mining, and analyzing the data in the data warehouse; business performance management {BPM) for monitoring and analyzing performance; and a user interface (e.g., a dashboard). The relationship among these components is illustrated in Figure 1.4. Benefits and uses of Business Intelligence Generally, business intelligence helps businesses spot problems early on, detect recent sales trends, uncover new market trends, and accelerate business growth. Business benefits will increase manifold, thanks to the technological advancements of BI platforms. Below are a few of the top benefits business intelligence offers: A holistic view of enterprise performance: The BI process allows businesses to aggregate companies’ disparate systems and provide a comprehensive view of their operations. Thus, BI tools help businesses evaluate their performance and optimize their business processes. Access to cloud-based platforms: Cloud analytics solutions and business intelligence will continue to benefit global businesses. As industry data becomes more diverse, timely analytics will become crucial for companies seeking to stay competitive. More control over data access: With the rising sophistication of AI- powered BI platforms, organizations will be better equipped to analyze the sources and types of data. BI will continue to empower business staff by providing them with accessible business data that can be used to make data analytics truly democratic. Data integration and integration data quality tools will also be in high demand, as businesses aim to make sense of their massive amounts of data. As users become more knowledgeable about the various Data Management tools available within BI platforms, they will not need to depend on technical experts for day-to-day analytics. Sharing data easily: BI platforms empower users to easily analyze and share their data, thanks to the wide range of interfaces available. This improves access to data analytics for major industry players who can use these insights to improve their decision-making processes. Improved Data Governance (DG): BI will continue to benefit global businesses by refining the DG policies. This trend will require businesses to adopt a robust governance strategy that includes implementing a DG system to ensure data security and protection. Business users will need to focus on key data consistency and transparency across regions that are adopting general data regulations like the General Data Protection Regulation (GDPR). Data mining and storage analytics: The market growth in this area is expected to continue as more businesses realize the benefits of mining analytics across organizations. Additionally, storage analytics models are becoming more popular among mobile users. This increased adoption of data mining and storage analytics has led to a significant increase in workforce productivity. Adaptable AI tools: These specialized tools will enable ordinary business staff to take on new roles. More employees will need access to these tools as businesses continue to grow, but giving employees the power of data analytics could transform their role in their respective areas of work. Visualizing analytics outcomes through reports and dashboards: Reports and dashboards help businesses to visualize complex data and monitor key performance indicators (KPIs) for further improvement. By uncovering gaps and weaknesses, the visualization tools offer timely data for improving performance. Additionally, dashboards offer real-time data – organizing important information and updating data regularly. This saves management time and helps track information for streamlining overall operations. Knowledge Discovery in Databases (KDD Knowledge Discovery in Databases, commonly referred to as KDD, is a systematic approach to uncovering patterns, relationships, and actionable insights from vast datasets. It involves multiple steps, from selecting and preprocessing data to the actual process of data mining and finally to the interpretation and use of the results. In this article, we will walk through the KDD methodology using a real-world example based on daily household transactions. What is KDD? Knowledge Discovery in Databases (KDD) is a systematic process that seeks to identify valid, novel, potentially useful, and ultimately understandable patterns from large amounts of data. In simpler terms, it’s about transforming raw data into valuable knowledge. The KDD process typically consists of the following steps: 1. Data Cleaning: Removing noise and inconsistent data. 2. Data Integration: Combining data from different sources. 3. Data Selection: Choosing the data relevant for analysis. 4. Data Transformation: Converting data into a suitable format or structure. 5. Data Mining: Applying algorithms to extract patterns. 6. Pattern Evaluation: Identifying the truly interesting patterns. 7. Knowledge Presentation: Visualizing and presenting the findings. Exploring the KDD Steps 1. Data Cleaning in this stage data reliability is enhanced, it includes data cleaning such as handling missing values and removal of noise or outliers. 2. Data Integration Data often comes from multiple sources, each with its own format and structure. This step merges this data into a unified set, ensuring consistency and reducing redundancy. 3. Data Selection this includes finding out what data is available and select a subset on which discovery will be performed, according to the goals of the analysis. 4. Data Transformation in this stage the generation of better data for the data mining is prepared and developed. Methods here include dimension reduction and attribute transformations. 5. Data Mining We are ready to decide on which type of data mining to use, for example, classification, regression or clustering. This mostly depends on the KDD goals: descriptive or predictive. This includes selecting the specific method and algorithm to be used for searching patterns in the data. 6. Pattern Evaluation In this stage we evaluate and interpret the mined patterns with respect to the goals defined in the first step and there’s the possibility to return to any of the previous steps. 7. Knowledge Presentation We are now ready to incorporate the knowledge into another system for further action. The knowledge becomes active in the sense that we may make changes to the system and measure the effects of them. KDD in Action: A Simple Example Scenario: Imagine a bookstore that wants to understand its customers’ buying habits to recommend books more effectively. 1. Data Cleaning: The bookstore starts with sales data. They remove any transactions with errors, like those with missing book titles or negative quantities. 2. Data Integration: They combine the sales data with their online store data, ensuring that the formats match and there are no redundancies. 3. Data Selection: The bookstore is only interested in sales from the last year, so they filter out older transactions. 4. Data Transformation: They summarize the data to see the number of books bought by each customer in different genres. 5. Data Mining: Using clustering algorithms, the bookstore identifies groups of customers with similar buying habits. 6. Pattern Evaluation: Among the patterns, they find a cluster of customers who buy a lot of science fiction and fantasy but rarely buy romance novels. 7. Knowledge Presentation: The bookstore creates a visualization showing the different customer clusters and their preferred genres. They then implement a recommendation system that suggests books based on the identified patterns. What to do to clean data? 1. Handle Missing Values 2. Handle Noise and Outliers 3. Remove Unwanted data Handle Missing Values Missing values cannot be looked over in a data set. They must be handled. Also, a lot of models do not accept missing values. There are several techniques to handle missing data, choosing the right one is of utmost importance. The choice of technique to deal with missing data depends on the problem domain and the goal of the data mining process. The different ways to handle missing data are: 1. Ignore the data row: This method is suggested for records where maximum amount of data is missing, rendering the record meaningless. This method is usually avoided where only less attribute values are missing. If all the rows with missing values are ignored i.e. removed, it will result in poor performance. 2. Fill the missing values manually: This is a very time consuming method and hence infeasible for almost all scenarios. 3. Use a global constant to fill in for missing values: A global constant like “NA” or 0 can be used to fill all the missing data. This method is used when missing values are difficult to predict. 4. Use attribute mean or median: Mean or median of the attribute is used to fill the missing value. 5. Use forward fill or backward fill method: In this, either the previous value or the next value is used to fill the missing value. A mean of the previous and succession values may also be used. 6. Use a data-mining algorithm to predict the most probable value Handle Noise and Outliers Noise in data may be introduced due to fault in data collection, error during data entering or due to data transmission errors, etc. Unknown encoding (Example : Marital Status — Q), out of range values (Example : Age — -10), Inconsistent Data (Example : DoB — 4th Oct 1999, Age — 50), inconsistent formats (Example : DoJ — 13th Jan 2000, DoL — 10/10/2016), etc. are different types of noise and outliers. Noise can be handled using binning. In this technique, sorted data is placed into bins or buckets. Bins can be created by equal-width (distance) or equal-depth (frequency) partitioning. On these bins, smoothing can be applied. Smoothing can be by bin mean, bin median or bin boundaries. Outliers can be smoothed by using binning and then smoothing it. They can be detected using visual analysis or boxplots. Clustering can be used to identify groups of outlier data.The detected outliers may be smoothed or removed. Remove Unwanted Data Unwanted data is duplicate or irrelevant data. Scraping data from different sources and then integrating may lead to some duplicate data if not done efficiently. This redundant data should be removed as it is of no use and will only increase the amount of data and the time to train the model. Also, due to redundant records, the model may not provide accurate results as the duplicate data interferes with the analysis process, giving more importance to the repeated values. Data Integration In this step, a coherent data source is prepared. This is done by collecting and integrating data from multiple sources like databases, legacy systems, flat files, data cubes etc. Data is like garbage. You’d better know what you are going to do with it before you collect it. — Mark Twain Issues in Data Integration 1. Schema Integration: Metadata (i.e. the schema) from different sources may not be compatible. This leads to entity identification problem. Example : Consider two data sources R and S. Customer id in R is represented as cust_id and in S is represented is c_id. They mean the same thing, represent the same thing but have different names which leads to integration problems. Detecting and resolving them is very important to have a coherent data source. 2. Data value conflicts: The values or metrics or representations of the same data maybe different in for the same real world entity in different data sources. This leads to different representations of the same data, different scales etc. Example : Weight in data source R is represented in kilograms and in source S is represented in grams. To resolve this, data representations should be made consistent and conversions should be performed accordingly. 3. Redundant data: Duplicate attributes or tuples may occur as a result of integrating data from various sources. This may also lead to inconsistencies. These redundancies or inconsistencies may be reduced by careful integration of data from multiple sources. This will help in improving the mining speed and quality. Also, co- relational analysis can be performed to detect redundant data. Data Reduction If the data is very large, data reduction is performed. Sometimes, it is also performed to find the most suitable subset of attributes from a large number of attributes. This is known as dimensionality reduction. Data reduction also involves reducing the number of attribute values and/or the number of tuples. Various data reduction techniques are: Data cube aggregation: In this technique the data is reduced by applying OLAP operations like slice, dice or rollup. It uses the smallest level necessary to solve the problem. Dimensionality reduction: The data attributes or dimensions are reduced. Not all attributes are required for data mining. The most suitable subset of attributes are selected by using techniques like forward selection, backward elimination, decision tree induction or a combination of forward selection and backward elimination. Data compression: In this technique. large volumes of data are compressed i.e. the number of bits used to store data is reduced. This can be done by using lossy or lossless compression. In lossy compression, the quality of data is compromised for more compression. In lossless compression, the quality of data is not compromised for higher compression level. Numerosity reduction : This technique reduces the volume of data by choosing smaller forms for data representation. Numerosity reduction can be done using histograms, clustering or sampling of data. Numerosity reduction is necessary as processing the entire data set is expensive and time consuming. Data discretization Data discretization is defined as a process of converting continuous data attribute values into a finite set of intervals with minimal loss of information and associating with each interval some specific data value or conceptual labels. Suppose we have an attribute of Age with the given values Age 1,5,9,4,7,11,14,17,13,18, 19,31,33,36,42,44,46,70,74,78,77 Table before Discretization Attribute Age Age Age Age 1,5,4,9,7 11,14,17,13,18,1 31,33,36,42,44,4 70,74,77,7 9 6 8 After Child Young Mature Old Discretization Another example is analytics, where we gather the static data of website visitors. For example, all visitors who visit the site with the IP address of India are shown under country level. Methods to achieve data discretization: a)Data binning or bucketing is a data pre-processing method used to minimize the effects of small observation errors. The original data values are divided into small intervals known as bins and then they are replaced by a general value calculated for that bin. This has a smoothing effect on the input data and may also reduce the chances of overfitting in the case of small datasets There are 2 methods of dividing data into bins: 1. Equal Frequency Binning: Equal Frequency Binning can be referred as Equal Depth Binning. It is a data binning technique that divides the values into bins having equal number of observations or frequency. This method's major goal is to ensure every bin has a comparable amount of data points. Input:[5, 10, 11, 13, 15, 35, 50, 55, 72, 92, 204, 215] Output: [5, 10, 11, 13] [15, 35, 50, 55] [72, 92, 204, 215] 2. Equal Width Binning : bins have equal width with a range of each bin are defined as [min + w], [min + 2w] …. [min + nw] where w = (max – min) / (no of bins). Equal Width: Input: [5, 10, 11, 13, 15, 35, 50, 55, 72, 92, 204, 215] Output: [5, 10, 11, 13, 15, 35, 50, 55, 72] [204, 215] b)Data Normalization Last but not least, data normalization is the process of scaling the data to a much smaller range, without losing information to help minimize or exclude duplicated data and improve algorithm efficiency and data extraction performance. There are three methods to normalize an attribute: 1. Min-max normalization: Where you perform a linear transformation on the original data. Min-max normalization is one of the most common ways to normalize data. For every feature, the minimum value of that feature gets transformed into a 0, the maximum value gets transformed into a 1, and every other value gets transformed into a decimal between 0 and 1. For example, if the minimum value of a feature was 20, and the maximum value was 40, then 30 would be transformed to about 0.5 since it is halfway between 20 and 40. The formula is as follows: ( − )/( − ) Min-max normalization has one fairly significant downside: it does not handle outliers very well. For example, if you have 99 values between 0 and 40, and one value is 100, then the 99 values will all be transformed to a value between 0 and 0.4. That data is just as squished as before! Take a look at the image below to see an example of this. Normalizing fixed the squishing problem on the y-axis, but the x-axis is still problematic. Now if we were to compare these points, the y-axis would dominate; the y-axis can differ by 1, but the x-axis can only differ by 0.4. 2. Z-score normalization: In z-score normalization (or zero-mean normalization) you are normalizing the value for attribute A(which stands for x or y attribute) using the mean and standard deviation. Z-score normalization is a strategy of normalizing data that avoids this outlier issue. The formula for Z-score normalization is below: ( − )/ Here, μ is the mean value of the feature and σ is the standard deviation of the feature. If a value is exactly equal to the mean of all the values of the feature, it will be normalized to 0. If it is below the mean, it will be a negative number, and if it is above the mean it will be a positive number. The size of those negative and positive numbers is determined by the standard deviation of the original feature. If the unnormalized data had a large standard deviation, the normalized values will be closer to 0. Take a look at the graph below. This is the same data as before, but this time we’re using z-score normalization. While the data still looks squished, notice that the points are now on roughly the same scale for both features — almost all points are between -2 and 2 on both the x-axis and y-axis. The only potential downside is that the features aren’t on the exact same scale. With min-max normalization, we were guaranteed to reshape both of our features to be between 0 and 1. Using z-score normalization, the x- axis now has a range from about -1.5 to 1.5 while the y-axis has a range from about -2 to 2. This is certainly better than before; the x-axis, which previously had a range of 0 to 40, is no longer dominating the y-axis. 3. Decimal scaling: Where you can normalize the value of attribute A by moving the decimal point in the value. Decimal scaling is another technique for normalization in data mining. It functions by converting a number to a decimal point. Normalization by decimal scaling follows the method of standard deviation. In decimal scaling normalization, the decimal point of values of the attributes is moved. The movement of the decimal points in decimal scaling normalization is dependent upon the maximum values amongst all values of the attribute. Decimal Scaling Formula Here: V’ is the new value after applying the decimal scaling V is the respective value of the attribute Now, integer J defines the movement of decimal points. So, how to define it? It is equal to the number of digits present in the maximum value in the data table. Here is an example: Suppose a company wants to compare the salaries of the new joiners. Here are the data values: Employee Salary Arti 10,000 Satish 25,000 Akshay 8,000 Pooja 15,000 Shruti 20,000 Now, look for the maximum value in the data. In this case, it is 25,000. Now count the number of digits in this value. In this case, it is ‘5’. So here ‘j’ is equal to 5, i.e 100,000. This means the V (value of the attribute) needs to be divided by 100,000 here. Employee Salary After Scaling Arti 10,000 0.1 Satish 25,000 0.25 Akshay 8,000 0.08 Pooja 15,000 0.15 Shruti 20,000.20 c)Smoothing Binning method is used for smoothing data or to handle noisy data. In this method, the data is first sorted and then the sorted values are distributed into a number of buckets or bins. As binning methods consult the neighborhood of values, they perform local smoothing. There are three approaches to performing smoothing – Smoothing by bin : each value in a bin is replaced by the mean value of the bin. Smoothing by bin median : In this method each bin value is replaced by its bin median value. Smoothing by bin boundary : In smoothing by bin boundaries, the minimum and maximum values in a given bin are identified as the bin boundaries. Each bin value is then replaced by the closest boundary value. Approach: Sort the array of a given data set. Divide the range into N intervals, each containing the approximately same number of samples(Equal-depth partitioning). Store mean/ median/ boundaries in each row. Examples: Sorted data for price (in dollars): 4, 8, 9, 15, 21, 21, 24, 25, 26, 28, 29, 34 Partition using equal frequency approach: - Bin 1 : 4, 8, 9, 15 - Bin 2 : 21, 21, 24, 25 - Bin 3 : 26, 28, 29, 34 Smoothing by bin means: - Bin 1: 9, 9, 9, 9 - Bin 2: 23, 23, 23, 23 - Bin 3: 29, 29, 29, 29 Smoothing by bin boundaries: - Bin 1: 4, 4, 4, 15 - Bin 2: 21, 21, 25, 25 - Bin 3: 26, 26, 26, 34 Smoothing by bin median: - Bin 1: 9 ,9, 9, 9 - Bin 2: 23, 23, 23, 23 - Bin 3: 29, 29, 29, 29