DRL - DnA Revised Framework 18 July PDF
Document Details
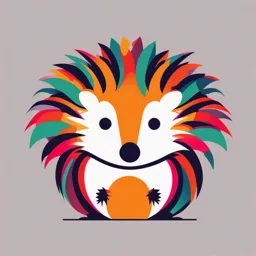
Uploaded by IntuitiveRiver
Praxis Business School
2023
Dr. Ravi Shankar
Tags
Related
- QbD_LSSYB_DOE_PHARMA DEVELOPMENT OPTIMISATION_MCS_STUDY MATERIAL_156933 PDF
- Instrumental Methods of Analysis B Pharmacy PDF
- UE4-Hématologie-Immunologie-Microbiologie Hémogramme PDF 2024/2025
- Introduction to Pharma and Healthcare Industries 2024 PDF
- Unit 5, Instrumental Methods of Analysis, B Pharmacy 7th Sem PDF
- Pharmaceutical Analysis PDF
Summary
DnA Champ – Business Translator Framework, curated and customized for Dr. Reddy's. A presentation on the revised Pharma Analytics curriculum and course content, covering topics such as motivation, data management, advanced analytics, and the data science research process.
Full Transcript
DnA Champ – Business Translator Framework Curated & Customized for: Dr. Ravi Shankar Prof – Data Science (AI & ML) Dean – Enterprise Solutions https://www.linkedin.com/in/ravi-shankar-70584b1/ [email protected] Revised Pharma Analytics curriculum & Course content 18th July , 2023 DnA Champ Learn...
DnA Champ – Business Translator Framework Curated & Customized for: Dr. Ravi Shankar Prof – Data Science (AI & ML) Dean – Enterprise Solutions https://www.linkedin.com/in/ravi-shankar-70584b1/ [email protected] Revised Pharma Analytics curriculum & Course content 18th July , 2023 DnA Champ Learning Journey Day 1 Day 1 Day 2 Session 1 Day 2 Session 2 Session 3 Session 4 1. Motivation 1. Leadership connect with 1. Data Management 1. Introduction 2. Data Governance 3. Removing Bias 4. Getting the right talent 5. Breaking Data Silos 6. Streamlining electronic 1. Execution Framework 1. Project Management 2. Agile Framework vs Venkat / Alpesh 2. Introduction 1. 4IR 2. High level real world examples of Pharma 3. Business Translator 1. Definition 2. Skills 3. Importance 4. Data Science Lifecycle 5. Descriptive Vs Prescriptive Vs Predictive 4. An executive’s guide to AI 1. What is AI? 2. Trends – New developments 3. Major types of ML Algorithms – Supervised, Unsupervised, Reinforcement with examples records 7. Performance Management 2. Advanced Analytics 1. Introduction 2. Challenges & Trends 3. Benefits & Applications 4. Real world examples 3. The Data Science Research Process 1. 2. 3. 4. 5. Problem Definition Data Cleaning Feature Engineering Evaluation Deployment Waterfall 2. Digital Twin 1. Digital Twin Overview 2. Digital Twin Use Cases in Manufacturing and R&D 3. Simulations Overview 4. Simulations examples in Research and Manufacturing 1. Robotics, RPA and IPA 1. Definition & Benefits 2. Use Cases & Best Practices 2. IoT 1. 4Cs of IoT –Connect, Collect, Compute, Communicate 2. IoT Use cases 3. AR/VR/ER - Overview 1. Definition 2. Augmented Reality Devices & Players 3. Use cases 4. Dashboarding 1. Basic Principles 2. Examples 5. Other technologies 1. Additive manufacturing, Blockchain etc. 6. Connect with Business Translator Profile : Dr. Ravi Shankar INTRODUCTION Background: PhD (Econometrics)Analytics Led Consulting, Data Science Thought Leader, Entrepreneur, Start-up Mentor, Social Impact Investor Senior Venture Partner, Visiting Prof Current Focus: AI adoption in Business, Deep Learning, XAI, ML Operations, Design Thinking, Agile Framework, OB & Strategy Sectoral Exposure: multiple sectors straddling BFSI / Pharma / Manufacturing / Retail / DeepTech – AgTech |InsurTech |FinTech | Healthnet Overall Experience : 30 Yrs & still counting / learning Organizational Experience Approach Executive’s Guide Interactive Business Treatment with Real World Relevant Examples “Tell me and I forget, teach me and I remember, involve me and I learn.” BEN FRANKLIN Motivation 1.Leadership connect with Venkat / Alpesh 2.Vision 3.Digital Initiatives 1. Digital Science 2. Digitalize the core 3. Transform with Digital 4.Examples @ DRL 5.Lighthouse status IR 4.0 Industry 4.0—also called the Fourth Industrial Revolution or 4IR—is the next phase in the digitization of the manufacturing sector, driven by disruptive trends including the rise of data and connectivity, analytics, human-machine interaction, and improvements in robotics. Pharma 4.0 is a framework for adapting digital strategies to the unique context of pharmaceutical manufacturing. It introduces more connectivity, increased productivity, simplified compliance, and the ability to leverage production information to respond to problems as they emerge1. The emerging technologies that characterize Industry 4.0—from connectivity to advanced analytics, robotics and automation—have the potential to revolutionize every element of pharmamanufacturing labs within the next five to ten years2. 4 IR Connectivity, data, and Analytics and computational power: cloud technology, the Internet, blockchain, sensors intelligence: advanced analytics, machine learning, artificial intelligence Human–machine Advanced interaction: virtual reality (VR) and augmented reality (AR), robotics and automation, autonomous guided vehicles engineering: additive manufacturing (such as, 3-D printing), renewable energy, nanoparticles 4IR and Pharma 4.0 • Implementing new Industry 4.0-based manufacturing concepts in Pharma 4.0™ requires alignment of expectations, definitions, and interpretation, with global pharmaceutical regulations. • While Industry 4.0 has been called a new industrial revolution, Pharma 4.0™ implementation will more likely resemble an evolution in which digitalization and automation meet very complex product portfolios and cycles • Digitalization, an important component of Pharma 4.0™, will connect everything, creating new levels of transparency and adaptivity for a “smart” plant floor. This will enable faster decision-making, and provide in-line and on-time control over business, operations, quality, and regulatory compliance. Notably, this new connectedness will require higher levels of security, since linked systems heighten vulnerability. Key Success Factors for the introduction of Data Science in the Pharma Industry “AI isn’t just a new set of tools. It’s the new world” From automation to augmentation, generative AI and beyond, AI is changing everything. $15.7 trillion—that’s the global economic growth that AI will provide by 2030, according to PwC research. Challenges in Pharma Manufacturing 1 Lack of visibility 2 Too much data Without real-time visibility, Pharma manufacturing production teams struggle to creates an overwhelming make data-driven decisions amount of data. Without in a timely manner. proper organization and visualization, it can be difficult to identify trends or patterns. 3 Competitive pressure Innovative companies constantly look for ways to cut costs while increasing production quality. 13 AI-ML Driven PSC or Digital PSCTRUST AI-ML provides the wherewithal for building Trust, Visibility & Control VISIBILITY CONTROL Digital transformation and digitalization are on the agenda for all organizations in the Pharmaceutical industry. But what are the main enablers of intelligent manufacturing? We hypothesize that data science–derived manufacturing process and product understanding is the main driver of digitalization in the bioprocessing industry for biologics manufacturing. Companies that use advanced analytics to improve operations have the potential to transform the Pharmaceutical manufacturing industry Pharma supply chains in a post-Covid-19 world will consist of resilient systems and processes infused with reliability, transparency and intelligence, at every stage. To achieve operational excellence, Pharma manufacturers must develop capabilities in advanced analytics. McKinsey & Pharma and Healthcare Logistics - Services | Maersk AI-ML-DS : Pre-requisites & Use From “Molecule to Market” there is no spectrum of the Pharma value chain cases that AI-ML-DS cannot impact including Manufacturing. However, KSF’s include : Data Driven Culture Team work Appropriate Skills Search | Pharma Manufacturing https://youtu.be/xJSapAmgMRU https://youtu.be/wcnXPnPV64g https://youtu.be/EoYvUx1v8vY AI in Pharma and Life Sciences | Deloitte US https://youtu.be/WRUliRQ2MHA Design Thinking AI-ML Infrastructure Pharma Supply Chain • Pharmaceutical supply chains have become global and complex. With more outsourcing, new modalities, and novel ways to reach patients, it’s critical to ensure that they can withstand shocks. • Leading pharma companies have succeeded in shifting their supply chains to drive growth and manage costs. But unless they assess and plan for the risks that come with these changes, they can suffer huge losses. • Pharma executives say supply-chain risk is a significant reason for their companies’ susceptibility to disruption, according to a recent McKinsey Global Institute survey. Nearly 50 percent of respondents cite sole sourcing of inputs as a critical vulnerability, and 25 percent point to a lack of visibility into supplier risks. Pharma Supply-chain resilience requires four elements End-to-end transparency Routine stresstesting and reassessment, Reduced exposure to shocks Supply-chain resilience on the executive agenda 70% of leaders in major pharmaceutical and MedTech corporations believe that their supply chains are vulnerable to the ongoing problems that are caused by the pandemic. Most pressing PSC issues include: Low Data, Rising Costs, Supply Shortage AI-ML in Pharma Use Cases across the Value Spectrum in general and Manufacturing in particular AI Value Creation for Pharma Case study : Optimizing manufacturing activities using AI To improve current manufacturing processes, a Pharma company applied analytics and neural networks technologies to manufacturing data at one of its sites in the United Kingdom. This improved manufacturing line speeds by 21%, cut downtime, and delivered an overall equipment effectiveness improvement of 10%. Additionally, the company was able to improve detection of quality defects and optimize machine throughput. The company is planning to apply deep-learning image recognition to detect quality defects at the site Use case : A top five Vaccine maker used advanced analytics to significantly increase its yield in vaccine production while incurring no additional capital expenditures Process Segmentation: clusters of closely related production activities; for each cluster, it took far-flung data about process steps and the materials used and gathered them in a central database. Hypothesis formulation : One team “on the ground” ran workshops and focus groups with local experts to draw an initial issue tree hypothesizing which parameters had the greatest influence on performance. A second advanced analytics team then tested that hypothesis using the company’s centralized and cleansed data. Through iterative loops between the two teams, nine parameters were identified as the most influential. Time to inoculate cells and conductivity measures associated with one of the chromatography steps were proven to be particularly important The manufacturer was able to increase its vaccine yield by more than 50 percent — worth between $5 million and $10 million in yearly savings for a single substance, one of hundreds it produces globally Predictive Monitoring for APIs Production Process Improving batch production process based on an AI model that analyzes real-time data streams from reactors? Problem Solution • Client XXX, a producer of Active Pharmaceutical Ingredients, was struggling with the manufacturing efficiency of their current repeatable batch production processes. Over 50% of produced batches went to waste due to final product quality deterioration. • A model that analyzes real-time data streams from the production process and identifies potential outliers that may lead to quality deterioration based on process data. Critical regions that accounted for process alterations were identified and applied anomaly detection using various deep learning techniques (RNNs, CNNs, and Transformers architectures). • Predictive data analytics has been applied to track batches of products as they go through the entire manufacturing process. This allows for real-time adjustments when issues arise during the operation so that corrective actions taken will minimize disruption while maximizing efficiency. • The benefits are improved efficiency, predictability, and quality assurance of manufacturing operations and yields. AI- AND ML-DRIVEN INSIGHTS ON AWS CLOUD DELIVERS 15% REDUCTION IN FORECASTING ERROR FOR THE WORLD’S LARGEST PHARMACEUTICAL COMPANY TO ACHIEVE USD 600 MN INCREMENTAL SALES Clinical trials analysis with NLP at Boehringer Ingelheim • • • The problem is that each protocol is up to 200 pages long and the structure can vary. Solution: Developing and training a deep learning tool using natural language processing (NLP) to predict more than 50 output variables from a clinical trial protocol. This allows pharma companies and regulators to analyze and quantify large numbers of clinical trial protocols, allowing more accurate cost estimation. The technique can be extended to other industries where large unstructured or semi-structured documents are the norm. Natural language processing can analyze clinical trial protocols. Pharma companies write a 200-page protocol at the planning stage of a clinical trial, and the NLP model is able to ‘read’ the document and output a number of complexity metrics. AI in Action for Manufacturing Process Improvement A success case study of AI application to improve the granulation process in drug manufacturing RESULTS The results were reviewed with the subject matter experts who validated that the parameters individually were significant. All the parameters were within normal operating ranges so looking at the parameters individually would not have raised any alarms. AI was able to identify three parameters of interest that the subject matter expert team can now monitor more closely as the team continually learns about the process “We have now entered a new phase of AI implementation where AI-based technology is expected to be foundational and not just a novel technology,” Abid Rahman, vice president, innovation, Intouch Group AI Advances Pharmaceutical Packaging Inspection Stevanato Group, has launched an artificial intelligence platform for its pharmaceutical inspection systems. Using deep learning models to overcome the traditional trade-off between detection and false reject rates, the company says the solution improves detection rates and offers a “tenfold” reduction in false reject rates for up to 99.9% accuracy, both for particle inspection and cosmetic defects detection. Raffaele Pace, engineering vice president of operations at Stevanato Group, says the AI-and-cloud enhanced platform can “substantially increase defect detection accuracy even with the most challenging drugs.” In particular, drugs in the form of suspensions or lyophilized cakes frequently challenge available vision tools, causing misinterpretations of supposed defects. Traditional systems can, for instance, misclassify cosmetic defects or air bubbles as particles. Artificial Intelligence mitigates misclassification and reduces costly re-inspection. Cloudbased Security Assistance Monitoring Get more from your pharma commercial spend using advanced analytics Predictive analytics and data visualization offer pharma business leaders the opportunity to transform commercial-spend optimization and lift returns by 10 to 25 percent. The ability to rapidly create and manage broad data sets. The leap in processing power offered by cloud computing. The emergence of advancedanalytics platforms that use an ensemble of approaches New optimization algorithms and predictive analytics that guide spend reallocation Machine Learning with Statistical Imputation for Predicting Drug Approvals 1. 2. 3. Machine-learning techniques to predict drug approvals using drug-development and clinical-trial data from 2003 to 2015 involving several thousand drug-indication pairs with over 140 features across 15 disease groups. The most important features for predicting success are trial outcomes, trial status, trial accrual rates, duration, prior approval for another indication, and sponsor track records. Provide estimates of the probability of success for all drugs in the current pipeline. – To deal with missing data, we use imputation methods that allow us to fully exploit the entire dataset, the largest of its kind. – ML approach outperforms complete-case analysis, which typically yields biased inferences. – Achieved a predictive measures of 0.78 and 0.81 AUC (“area under the receiver operating characteristic curve,” the estimated probability that a classifier will rank a positive outcome higher than a negative outcome) for predicting transitions from phase 2 to approval and phase 3 to approval, respectively. – Using five-year rolling windows, we document an increasing trend in the predictive power of these models, a consequence of improving data quality and quantity. Source : https://hdsr.mitpress.mit.edu/pub/ct67j043 41 Intelligent drug supply chain Creating value from AI – Use cases Inventory prediction in vaccine supply chains Challenges • Inventory prediction, to meet regulatory standards Solution • A new type of data tracking that could handle GPRS signal functionality, and send • Reduce Risk large amounts of up-to-date information at • Improving decision-making once via a monitoring device • Improving partnership selection • Improve CX Results • Cloud capabilities, to track conditions of vaccine components in real time •99.999% of Pfizer-BioNTech vaccines successfully reaching their receiving sites Cas Optimizing Demand Forecasting Challenges • Improving demand forecasting across its product portfolio Solution Results • ML and cloud-based software that enables a holistic and actionable view of a company’s supply network • Continuously comb through enterprise • Improved the forecast accuracy of 90 per cent of its products. • Quickly and accurately forecast the systems to collect, harmonize and demand for its products in terms of refine data, and to consequently both quantity and location provide real-time analytics and end-toend • Improve visibility of the company’s supply chain operation and performance • Pilot runs show the technology is 80% more effective than humans in demand planning processes Predictive & Prescriptive Maintenance Solution Challenges • Improve robustness of pharma supply • Leverage AI, machine learning, predictive chains by preventing production and prescriptive capabilities to monitor the downtime that affects the delivery of smallest changes in the behavior of the lifesaving medicines equipment (such as temperature, flow or • Production failures due to unexpected asset repairs and replacements • Costly downtime power) and data from previous failures to alert operators proactively when issues start to surface • Identifying the root cause in the deionizer equipment and determining the early signs of the asset failing using machine learning from late-stage deterioration patterns • Identifying early coil saturation using predictive capabilities, operators now have the lead time needed to remove the batch from the chamber and ensure that the coil s stable before reintroducing the batch. Results • 35 days’ advance warning of potential issues • Tens of millions of USD in lost batches avoided • 50% reduction in lifecycle maintenance costs • 30 sites across 18 countries covered, multiplying value gained Inventory Management Challenges Solution Results • Leverage AI, machine learning, predictive • The client pharma company has • Inefficient inventory management and prescriptive capabilities to monitor the • Random & uncertain delays in inbound smallest changes in the behavior of the complex formulations and works with equipment (such as temperature, flow or hundreds of global suppliers; using power) and data from previous failures to machine learning enables it to better alert operators proactively when issues start assess shopping orders to surface • ATP* dates from suppliers and make sure to balance inventory appropriately • multiplying value gained Procurement spend Optimization Challenges • . Information asymmetry on the vendor products coupled with inconsistent buying behaviors resulted in overspending, inventory mismanagement, in addition to Results Solution • Develop a “Buying Engine” by leveraging AI, machine learning • 1. Procurement products from third-party vendors are ingested in the form of product catalogs • 2. The catalogs are analyzed, enriched, research development, and and categorized by utilizing state-of-the-art manufacturing delays machine learning • techniques • 3. The processed products data is ingested in a set of databases that form the Novartis Product Knowledge Base • Enable 5% yearly cost saving on procurement spending with progressively increasing the total addressable spend • Search and recommendation, letting users search for products within the catalog • using free text. • make faster data-driven decisions, plan better, negotiate contracts and discounts, and save costs. Analytics/ Business Translator The Art of Analytics Business Translation Analytics business translation is the process of interpreting complex data into actionable insights that guide data-driven decisions. What is an Analytics Business Translator? 1 What They Do 2 Why We Need Them 3 Essential Skills An analytics business translator Without an analytics business Strong analytical skills, business bridges the gap between translator, businesses risk acumen, and the ability to business stakeholders and making decisions based on communicate insights to non- technical experts to ensure data- incomplete or inaccurate data. technical stakeholders are key to driven decision making. being an effective translator. The Importance of Analytics Business Translation Clarity Insight Impact Analytics business translation ensures Translations can uncover insights that Analytics business translation can drive clear and accurate communication of would otherwise remain hidden in profit growth and operational efficiency insights that guide decision making. complex data sets. by guiding smarter decision making. Key Skills Required to be an Analytics Business Translator Communication Visual storytelling Industry Knowledge Effectively communicate findings Present complex data in a way that Stay up-to-date with advancements and insights to both technical and is visually appealing and easy to in the industry to be able to non-technical stakeholders. understand. recognize opportunities and challenges. Data Analysis Analyze data sets to identify trends and patterns that can be turned into actionable insights. Business (Analytics) Translator The core of the Analytics Translator function is the second result area, Translating analytics to business. We use the 5 step Data Science process as defined by McKinsey: 1. In identifying and prioritizing business use cases, as an Analytics Translator, you work with business-unit leaders to identify and prioritize problems that analytics is suited to solve. 2. In collecting and preparing data, as an Analytics Translator, you help identify the business data needed to produce the most useful insights. 3. In building the analytics engine , as an Analytics Translator, you ensure that the solution solves the business problem in the most efficient and interpretable form for business users. 4. In validating and deriving business implications, as an Analytics Translator, you synthesize complex analytics-derived insights into easy-to-understand, actionable recommendations that business users can easily extract and execute on. 5. In implementing the solution and executing on insights, as an Analytics Translator, you drive adoption among business users. An Executives’ Guide to AI-ML The term ‘AI’ encompasses many different technologies and capabilities Jargon Demystified in AI and ML Artificial Intelligence Statistics & BI Machine Learning Data Science Deep Learning AI- ML What is AI-ML? AI-ML stands for Artificial Intelligence and Machine Learning. AI is a technique of building machines that can learn and understand from experience, while ML is an application of AI that allows machines to automatically learn and improve from experience without being explicitly programmed. What is Machine Learning? Before we dive deeper into the applications of ML in pharma manufacturing, let's define what this technology actually is. Machine learning is a subset of artificial intelligence that allows machines to automatically learn from data, without being explicitly programmed. Supervised ML Unsupervised ML Reinforcement ML Trained on labeled data to predict Trained on unlabeled data to find Trained through trial and error to new similar data. patterns and relationships. maximize rewards for certain actions. In the bioprocessing industry, parts or all stages of the data analytics continuum are applicable. In earlystage development, advanced data analytics is used to compare batches, for example, to figure out how to modify cell expansion steps so that they lead to higher cell densities and product titers. In latestage development, advanced data analytics is used when scaling-up manufacturing processes to verify comparable performance at different scales. And in full production, advanced data analytics is used for real-time bioprocess monitoring and early fault detection of batches deviating from good, normal operating conditions Humans Vs AI-ML models COMPUTER VISION NLP Key factors driving AI-ML BIG DATA GPU Another technology that helped modern day ML Data Management in Pharma Manufacturing Pharmaceutical production is a highly complex process requiring extensive data management. This session will cover challenges, trends, best practices and more. Structured data Can be displayed in rows, columns and relational databases Numbers, dates, strings Estimated 20% of enterprise data (Gartner) Requires less storage Easier to manage and protect with legacy solutions Unstructured data Cannot be displayed in rows, columns and relational databases Images, audio, video, word processing files, emails, spreadsheets Estimated 80% of enterprise data (Gartner) Requires more storage More difficult to manage and protect Data Types • Internal versus external • Structured versus unstructured • Machine generated versu s human generated •Static versus dynamic •Raw versus pre-processed •Transactional or not •Sensor data or not Nano data problems need tender care Medium data problems are playful and most enjoyable Big data problems require engineering brute force Nano, medium and big data problems AI-ML is data hungry & sensitive Challenges 1 4 Volume 2 Diversity 3 Quality The sheer volume of data generated Data generated in pharmaceutical Data quality is paramount in in pharmaceutical manufacturing manufacturing is highly diverse, pharmaceutical manufacturing, where processes is increasing, leading to ranging from chemical and biological errors in data collection, processing challenges of data storage, sharing data to manufacturing and clinical or analysis can lead to costly quality and analysis. data. Managing diversity is a major issues, product recalls, and loss of challenge. reputation. Compliance Data management is subject to stringent regulatory requirements, both in terms of data privacy and product quality. Compliance with regulations remains a challenge. Trends Smart manufacturing Big data analytics Cloud computing Cybersecurity Pharma manufacturing facilities are Data analytics and machine learning Cloud computing is increasingly being used Pharmaceutical manufacturers are focusing increasingly adopting smart technologies, algorithms are being extensively used in in pharmaceutical manufacturing to store, more on cybersecurity measures to protect including automation, the internet of things pharmaceutical manufacturing to analyze share, and analyze data in a cost-effective sensitive data from sophisticated cyber (IoT), and artificial intelligence (AI), leading complex data sets, identify patterns, and and scalable manner, enabling easy access threats, including data breaches, to more data generation and higher predict outcomes, leading to more effective to data from anywhere and anytime. ransomware attacks, and other malicious efficiency in data management. data-driven decision making. activities. Best Practices 1 Data Governance Framework A data governance framework should be established to define Master Data Management 2 policies, standards, roles, and responsibilities for data 3 Data Analytics management, ensuring data quality, security and compliance. A master data management system should be established to maintain a central repository of master data, harmonizing data across functions and systems, thereby ensuring data integrity and consistency. Data analytics should be integrated with the manufacturing process to facilitate real-time monitoring, predictive analytics Data Security Data security measures should be established to protect sensitive data from unauthorized access, including encryption, access controls, backup, and disaster recovery. 4 and prescriptive analytics for more informed decision making. Real World Examples Sanofi Cipla Pfizer Sanofi deployed a data integration Cipla established a master data Pfizer developed a data analytics platform to integrate data from management system to harmonize platform to automate data analysis, various sources into a unified view, data across systems, reducing data enabling real-time decision making facilitating decision making and redundancy and improving the and reducing the risk of errors. improving operational efficiency. quality of data. The Novartis data lifecycle Data Biases ML carries with it all the biases that data carries Types of Bias in Statistics and the Affect Data Bias Has on Your Business | Mailchimp Fairness • What do you see? • Bananas • Stickers • Bananas on shelves What do you see? What do you see? • Green Bananas • Unripe Bananas • Overripe Bananas • Good for Banana Bread Yellow Bananas Yellow is prototypical for bananas Designing for Fairness Consider the problem Ask experts Trainthemodelstoaccountforbias Interpret outcomes Publish with context Fairness: Identifying Bias Missing Feature Values If your data set has one or more features that have missing values for a large number of examples, that could be an indicator that certain key characteristics of your data set are under-represented. Unexpected Feature Values When exploring data, you should also look for examples that contain feature values that stand out as especially uncharacteristic or unusual. These unexpected feature values could indicate problems that occurred during data collection or other inaccuracies that could introduce bias. Data Skew Any sort of skew in your data, where certain groups or characteristics may be under- or over-represented relative to their real-world prevalence, can introduce bias into your model. If you can’t measure it, you can’t manage it If it’s easy, it’s probably wrong. A classifier is only as good as the metric used to evaluate it. Types of metrics for evaluating ML models Probability Ranking Threshold Common Metrics That Are Used To Evaluate Predictive Models 1. Confusion Matrix 5. AUC – ROC 2. F1 Score 6. Log Loss 3. Gain and Lift Charts 7. Gini Coecient 9. Root Mean Squared Error 4. Kolmogorov Smirnov Chart 8. Concordant – Discorda nt Ratio Performance Metrics for Machine Learning Models Performance Metrics for Classification Problems Accuracy Confusion Matrix ROC Log Probability Performance Metrics for Regression Problems R Square Adjusted R Square MSE RMSE MAE MADE MAPE “The unexamined life is not worth living.” Performance MeasurementBasics with examples “The unexamined ML model is not worth-production.” Problems with Accuracy • Assumes equal cost for both kinds of errors – cost(b-type-error) = cost (c-type-error) • is 99% accuracy good? – can be excellent, good, mediocre, poor, terrible – depends on problem • is 10% accuracy bad? – information retrieval • BaseRate = accuracy of predicting predominant class (on most problems obtaining BaseRate accuracy is easy) Percent Reduction in Error • 80% accuracy = 20% error • suppose learning increases accuracy from 80% to 90% • error reduced from 20% to 10% • 50% reduction in error • 99.90% to 99.99% = 90% reduction in error • 50% to 75% = 50% reduction in error • can be applied to many other measures Conclusion Data management is an essential component of pharmaceutical manufacturing. By implementing best practices and adopting emerging trends, manufacturers can effectively manage data, improve operational efficiency, and drive business growth. References Sanofi. “Using Data Integration to Improve Collaboration and Efficiency.” Cipla. “Harmonizing Data Across Systems for Improved Data Quality.” Pfizer. “Automated Data Analysis: A Game Changer for Pharmaceutical Manufacturing.” Advanced Analytics in Pharma An Introduction Advanced Analytics in Pharma Manufacturing Pharma manufacturing is a complex process with a lot of moving parts. Advanced Analytics is a game changer for this industry, solving problems and providing insights that would otherwise be impossible. How Advanced Analytics are benefiting Pharma Manufacturing ? Reducing PQV Benefits Lowering Costs Increasing Sales Pharma Manufacturing Overview What is Pharma Manufacturing? Key Challenges Industry Trends Pharma manufacturing involves Increased demand for The process of producing complex processes, strict personalized medicine and new pharmaceutical products for regulations and high quality innovative drugs requiring more human or animal consumption in a standards. flexible manufacturing processes. safe and cost-effective manner. The Challenges of Pharma Manufacturing Regulatory Compliance Quality Control Resource Allocation Production schedules need to be Production runs need to meet Optimizing manufacturing meticulously tracked and strict quality standards and meet processes requires juggling the documented to ensure pharmaceutical testing competing priorities of time, cost, compliance with FDA regulations. requirements. and quality. Introduction to Advanced Analytics What is it? How does it work? Why is it important? Advanced Analytics is a broad term These tools use machine learning This approach allows manufacturers that encompasses a variety of tools algorithms to identify patterns and to identify problems and optimize and techniques used to analyze insights in large amounts of data. processes in ways that are simply not complex data sets. possible with traditional analysis tools. Benefits of Advanced Analytics • Optimized production processes • Improved product quality • Reduced manufacturing costs • Lower risk of product recalls • Real-time monitoring and feedback • Better use of resources • Increased efficiency • Improved decision making Applications of Advanced Analytics 1 Drug Discovery & Development Advanced Analytics can help manufacturers Manufacturing Process Monitoring 2 Real-time monitoring of manufacturing data, identify new target molecules and predict the effectiveness of drug compounds. This helps them select the most promising candidates for collected from various sensors and processes, clinical trials. provides valuable insights. Manufacturers can continuously monitor processes to ensure consistent quality. 3 Sales & Marketing Analytics Pharmaceutical manufacturers can analyze their sales and marketing data to better understand Supply Chain Optimization Using analytics to optimize logistics and reduce costs, manufacturers can ensure that products are delivered on time and with fewer interruptions. 4 consumer behavior. They can also track market trends and uncover new opportunities. Implementation of Advanced Analytics Data Collection & Management Modeling & Machine Learning Integrated Systems & Partnerships Pharmaceutical manufacturers Manufacturers must develop and Finally, the most successful must collect data from multiple train models to analyze the implementations of advanced sources, including sensors, collected data. This requires the analytics in pharma equipment, and people. Collecting use of sophisticated machine manufacturing typically involve an data is only the first step, learning algorithms that can integrated suite of systems and however; they also need to identify patterns and insights in partnerships with vendors. manage it in a scalable way. large amounts of data. Future Trends in Analytics • Increase in predictive analytics • Growth in real-time monitoring • Increased adoption of cloud-based analytics • Greater use of artificial intelligence and machine learning • More emphasis on data privacy and security Real World Examples GlaxoSmithKline Eli Lilly and Co. Merck GSK is using advanced analytics to Eli Lilly and Co. used advanced analytics Merck used advanced analytics to predict and manage quality issues to optimize waste disposal in their improve their drug discovery process. before they occur. This has resulted in manufacturing processes. This resulted By analyzing large amounts of data, they improved compliance, lower costs, and in significant cost savings and a reduced were able to accelerate the discovery of increased efficiency. environmental impact. new drugs and reduce costs. AI-ML in Pharma Manufacturing Join us on a journey to explore the benefits, challenges and applications of AI-ML technology in the field of pharma manufacturing. Applications of AI-ML in Pharma Manufacturing Manufacturing Processes Drug Discovery Data Analytics Automating various steps of Using machine learning to speed up the AI-ML algorithms help pharma manufacturing processes such as drug discovery process and develop manufacturers make sense of large assembly, inspection, and packaging to new treatments with greater accuracy. amounts of data and provide insights increase efficiency and quality into how to optimize their operations. Benefits of AI-ML in Pharma Manufacturing Predictive Maintenance Using AI-ML can help detect and predict equipment failures and maintenance needs, reducing downtime and costs. 1 2 3 Process Optimization Quality Control AI-ML enables manufacturing processes to be more AI-ML can improve detection of defects, quality issues efficient, flexible and reduces errors. and product failures, allowing for more accurate and timely responses. Successful Implementation of AI-ML 1 GlaxoSmithKline 2 Pfizer Used AI-ML to decrease Implemented predictive drug discovery time from maintenance systems in four years to one in a manufacturing plants to project that looked into decrease breakdowns and pharmaceutical lead increase uptime. optimization. 3 Merck Implemented AI-ML to analyze large amounts of data for the development of both small and large molecules in the manufacturing process. Future Possibilities and Developments 1 Predictive Analytics Using machine learning to predict a variety of Smart Manufacturing 2 outcomes such as demand for drugs and supply 3 Advanced Analytics chain inefficiencies. AI-ML allows companies to achieve smart manufacturing capabilities in the pharma industry, to enable a fully connected workforce and supply chain. Using AI and big data analytics to create a predictive maintenance roadmap that optimizes manufacturing efficiency based on insights obtained from analyses of infrastructure and product performance. Considerations for Companies Regulatory Compliance Data Management and Security Employee Training and Education pharma industry and AI-ML Ensure that robust data Training and education are key to systems should be auditable, management systems are put in successful integration of AI-ML transparent and in compliance place to protect sensitive data systems, including developing with FDA or EMEA regulations. and ensure data privacy and necessary skills among employees security. and encouraing data-driven Compliance is critical in the decision making. Conclusion and Key Takeaways AI-ML is a game changer in Pharma Manufacturing Preparation is Key Future is Bright Companies must properly prepare The future for AI-ML in pharma for the adoption of AI-ML solutions manufacturing is very promising and The benefits of AI-ML in pharma to avoid and overcome the it will continue to transform the manufacturing are significant, and challenges. industry in ways we cannot yet more and more companies are embracing this technology. imagine. Applications of Machine Learning in Pharma Manufacturing Machine learning (ML) is becoming widely implemented in the pharmaceutical manufacturing industry. Here, we will explore some of the ways in which this technology is transforming the field. Applications of ML in Pharma Manufacturing Quality Control Drug Discovery Packaging Optimization Machine learning algorithms can be used ML can accelerate the drug discovery By analyzing market trends and consumer to analyze product quality, detect defects, process by identifying key features in preferences, ML can optimize packaging and predict quality issues on the chemical compounds, predicting drug design and materials to improve efficiency production line. efficacy, and simulating cellular and reduce waste. responses. Benefits of ML in Pharma Manufacturing 1 3 Increased Efficiency 2 Improved Quality ML can automate routine ML can identify defects and tasks, reduce errors, and quality issues earlier in the optimize production production cycle, reducing processes, resulting in product recalls and significant cost and time improving customer savings. satisfaction. Enhanced Innovation ML can help identify new opportunities for drug development, optimize clinical trials, and drive continuous process improvement. Real-World Examples of ML in Pharma Manufacturing 1 Amgen Developed ML models to optimize upstream and downstream processes in bioreactors, resulting in faster and more efficient drug development. 2 Novartis Uses predictive ML algorithms to forecast product demand, optimizing inventory management and reducing waste. 3 Pfizer Implemented a predictive maintenance system for manufacturing equipment, reducing unplanned downtime and maintenance costs. 4 Roche Used ML to analyze patient data and predict which patients would benefit most from cancer immunotherapy drugs, improving patient outcomes. Potential Impact of ML on the Pharma Industry The adoption of ML in pharma has the potential to transform the industry, resulting in faster drug development, improved clinical outcomes, and reduced costs. However, the implementation of this technology also raises ethical and regulatory concerns that need to be addressed. Improved Patient Outcomes Greater Efficiency New Business Models By leveraging ML to optimize drug ML can streamline manufacturing The implementation of ML could lead development and clinical trials, we processes, reduce waste, and lower to new and disruptive business could see more personalized, overall costs. models, such as outcome-based effective, and targeted treatments. pricing and personalized medicine. Conclusion and Future Directions Overall, machine learning has the potential to revolutionize the pharmaceutical industry by improving efficiency, reducing costs, and increasing innovation. As this technology continues to evolve, it is important to carefully consider the ethical and regulatory implications of its use. Data Science Research Process / Life Cycle Learn about the different phases in the data science life cycle and how to create a successful data-driven strategy. The Data Science Research Process Problem Definition Data Governance Deployment Evaluation Data Collection 1 Identify Data Sources Determine which sources of data are relevant for Extract Data 2 your research project. Use APIs and web scraping tools to collect data from various sources. 3 Validate Data Quality Ensure that the collected data is accurate and free of errors or duplicates. Data Preparation Data Cleaning Data Transformation Data Integration Remove incomplete or irrelevant data to Convert data into a format that can be Combine data from multiple sources to improve the quality of your dataset. analyzed and processed efficiently. build a comprehensive and accurate dataset. Data Analysis Exploratory Data Analysis Descriptive Analytics Predictive Analytics Visualize data distributions and Calculate summary statistics and Create predictive models based on relationships to discover patterns metrics to describe data historical data to forecast future and insights. characteristics. trends. Prescriptive Analytics Recommend optimal decisions or actions based on analytical insights. Data Modeling 1 2 3 Choose Algorithm Train Model Evaluate Model Select an appropriate machine Use a training dataset to build and Test your model's ability to predict learning algorithm for your research refine your model. new data accurately. project. Model Deployment Choose Deployment Method Implement Deployment Decide how to deploy your model: cloud, server, or on- Configure your deployment infrastructure and publish your premises. model as an API or web service. Model Maintenance 1 2 Monitor Model Performance Update Model Track your model's performance and adjust as needed to Retrain your model as new data becomes available, or as ensure its accuracy and reliability. your business requirements evolve. Conclusion The data science life cycle is a continuous process that requires expertise in statistics, programming, and domain knowledge. By following these Best Practices, you can create a data-driven culture and obtain valuable insights from data to drive your business goals forward. Project Management in AI, Machine Learning, and Data Science Effective project management is critical to ensuring the success of AI and data science initiatives. In this presentation, we'll explore the unique challenges of managing these projects and discuss best practices for achieving your goals. What is Project Management? Project Planning Team Management Risk Management Developing a detailed project plan Ensuring that all team members Identifying potential risks and that outlines the scope, timeline, understand their roles and developing strategies for mitigating budget, and resources required to responsibilities and are working them to minimize potential impacts complete the project successfully. effectively towards the project on the project. goals. Project Monitoring and Control Monitoring project progress and adjusting the project plan as needed to ensure successful completion. Why Data Science Initiatives Require Effective Project Management? Data Analysis Complexity Cross-functional Collaboration Data science projects involve analyzing large Many data science projects involve developing Data science projects often require collaboration volumes of complex data, requiring careful complex machine learning algorithms requiring across multiple teams, making it important to planning and management to ensure accurate extensive technical expertise, so effective project have clear goals, deadlines, and processes in and meaningful insights. management is essential to ensure the place to manage these relationships effectively. successful development of these algorithms. How to Project Manage AI Engagements? 1 Define Project Objectives Clearly define and prioritize project goals based on business needs, technological capabilities, and feasibility. 2 Develop a Detailed Project Plan Create a comprehensive project plan that outlines the project scope, timeline, budget, and resources required. 3 Building the Team Assemble a team with the required expertise and skills, including data scientists, developers, business analysts, and project managers. 4 Execution and Monitoring Execute the project plan, monitor progress, and adjust the plan as needed to ensure successful completion of the project. Data Science Project Management Approaches 1 Waterfall 2 Agile 3 Scrum A linear approach to project An iterative approach that A subset of Agile that focuses on management that involves emphasizes flexibility, teamwork, accountability, and sequential stages, each collaboration, and customer iterative progress delivery completed before moving to the feedback to deliver the final through sprints. next one. product. The ML Blueprint Mathematics Algorithms Languages Libraries Essential skills and technologies for developers to embrace machine learning Tools Conclusion and Next Steps Partnership Plan Your Next Project Beyond Project Management Whatever the size of your AI and Data Take your next step by outlining your AI and Data Science presents limitless Science initiatives, effective project project scope, deliverables, and timeline. opportunities, let's put on our thinking hats management is essential to their success. Document intended resources, develop and brainstorm! Let's work together to make your project a risk management strategies and explore success! your approach to project management! Agile Framework for Data Science Agile methodology has revolutionized the way software development works. In this presentation, we will see how Agile principles can be applied in Data Science. What is Agile? 1 A Customer-Centric Approach 🎯 2 Iterative Development 🔄 Agile development is based on the In Agile methodology, the focus is idea of breaking down a project into on delivering value to the customer smaller, more manageable chunks, through continuous iteration and and delivering them incrementally. improvement. 3 Collaboration 💬 Agile methodology requires close 4 Continuous Improvement 📈 collaboration between cross- The Agile framework focuses on functional teams, including continuous learning and developers, data scientists, and improvement, so that the team can stakeholders. adapt to changing requirements and improve their processes with each iteration. Agile Data Science Best Practices Start Small Continuously Test and Refine Stakeholder Involvement into smaller, more manageable chunks, Tests should be conducted step of the way, from project kickoff, and deliver them incrementally. continuously to ensure that each standups to project delivery. Break down your data science projects iteration or module delivers as planned and meets the requirements. Stakeholders should be involved every Agile Data Science Principles Embrace Change 🌪️ Instead of resisting change, embrace it, and adapt as fast as possible to changing requirements. 1 2 3 Satisfy the Customer 🤝 Collaborate 🎤 Deliver value to the customer through continuous delivery. Cross-functional teams must collaborate closely to improve the development process ongoing. Key Concepts…. Scrum Stand-up Kanban A methodology for project A short daily meeting where team A visual workflow management tool, management that emphasizes members discuss their progress and which allows teams to visualize teamwork, accountability and any problems they're facing. work, optimize resources and iterative progress toward a well- improve delivery times. defined goal. DevOps A set of practices that automates the processes between software development and operations teams, allowing for faster delivery and shorter cycle times. Agile Roles in Data Science 1 2 3 4 Product Owner 🧑💼 Data Scientist 👩💻 Scrum Master 👨✈️ Responsible for the Applies statistical and Responsible for ensuring Analytics Engineer 👨💻 success of the product, machine learning that the Scrum team is Responsible for building working with the techniques to vast adhering to Scrum and maintaining the data stakeholders to define quantities of data to theory, practices, and infrastructure and data the product goals, and extract insights and rules throughout the pipeline used by the data coordinating its generate value for the project lifecycle. scientists in their analysis development. customer. work. Agile Data Science Framework Kickoff 🎬 Data Analysis 📊 Code Development 🖥️ Testing & Delivery 🚀 The first step is to define the project goals, Once the data is available, data scientists use Once the insights are found, the next step is The testing phase is crucial to ensure that the scope, and timeline with stakeholders and their skills and expertise to explore patterns to develop the code or algorithm to achieve software works as intended and delivers the align everyone's expectations. and extract insights. the project goals. value the customers are expecting. Benefits of Agile Framework in Data Science Flexibility Collaboration Iterative Process Offers flexibility to adjust the project Promotes cross-functional Delivers a working model of the scope and requirements based on collaboration, customer feedback, and product at the end of each iteration, changing business needs and market team member engagement, improving enabling stakeholders to provide conditions. project outcomes. feedback and adjust project requirements accordingly. Agile Data Science Challenges Data Quality Technical Debt Managing Stakeholders One of the biggest challenges in Agile Technical debt is the accrual of Managing all the stakeholders involved Data Science is also one of the most development work that is put off in can be a significant challenge, as many fundamental - the quality of data itself. favor of faster delivery to maintain the projects are held up waiting on pace of Agile. information from the stakeholders. Digital Twin in Pharma Manufacturing A digital twin is a virtual replica of a physical system that can be used to simulate, predict, and optimize real-world processes. In pharma manufacturing, digital twins can help optimize the production process and improve quality by providing a real-time visualization of the process. Why Digital Twin is Important in Pharma Manufacturing 1 Reduce production costs 2 Enhanced product quality 3 Improved production efficiency Digital twin technology enables Digital twins are used to detect tracking and optimizing of anomalies and prevent errors in With real-time condition processes, which leads to better the production cycle which monitoring, manufacturers can resource management and, in ultimately leads to the improved identify inefficiencies and address turn, reduced production costs. product quality. them before they become bottlenecks, leading to improved production efficiency. German manufacturer Bausch + Ströbel plans to make engineering at least 30% more efficient by 2020 (source: Siemens). Digital representation of a chemical API process Benefits of Using Digital Twin in Pharma Manufacturing Enhanced Process Control Reduced Time to Market Better Collaboration Digital twins can predict the process Digital twins are a shared platform that Digital Twin provides real-time insights performance beforehand thus is accessible by everyone involved in that help to monitor the process and streamline the production pipeline. This the manufacturing process. This predict deviations. This promotes ensures that the product reaches the promotes collaboration among cross- decision-making and helps in achieving market in a timely manner, improving functional teams which helps to improve greater control over the process. the ROI. the quality and accuracy of the product. Implementation Challenges of Digital Twin in Pharma Manufacturing 1 Data Quality 2 Integration Complexity 3 Privacy and Security Digital Twins are data-driven and This is a concern when Digital Digital Twins gather data by hence require high-quality data Twin technologies are introduced connecting multiple systems that is accurate and reliable to into existing facilities. It may together, This may increase improve quality control. Poor data require the systems to be rebuilt vulnerability to cyber threats. quality leads to suboptimal from scratch which may disrupt the Regulatory policies need to be decision-making strategy. ongoing operations. followed to safeguard the security and privacy of the information. Real-Life Examples of Digital Twin in Pharma Manufacturing Vaccine Manufacturing Medical Tablet Production Manufacturing Operations Monitoring Digital twins provide manufacturers with insights into Digital twins are used to monitor the process flow, Digital twins can capture the performance of the the performance of individual systems, which, when detect deviations, and predict process performance. manufacturing process in real-time, predict the combined, provide a holistic view of the supply chain. This leads to a reduction in scrapped products and, in behavior of machines and the manufacturing This helps to diagnose and correct anomalies in real- turn, improved quality. process. Based on this analysis, steps can be taken time. to optimize the process leading to higher benefits, reduced costs, and improved efficiency. Future of Digital Twin in Pharma Manufacturing Innovations in Process Technology IoT-based Solutions Collaborative Partnerships The Internet of Things (IoT) is Companies in the pharma industry As the pharma industry expands, new growing in popularity across many can leverage partnerships with Big process technology is being industries, and the pharma industry is Tech corporations to develop digital introduced which would leverage no exception. Manufacturers are twins that extend from R&D to the digital twins to better control and exploring ways of combining IoT manufacturing process, improving the optimize the manufacturing process technology with digital twins to quality and speed of product by detecting anomalies quickly. improve performance, reduce costs development. and ensures a streamlined production pipeline. Pharma Digital Twin Solutions: Real Impact • • • • • • Save $2 Mn by Improving the Golden Batch Yield A global pharma manufacturer, wanted to predict the quality of their products by gaining more visibility from their plant operations. Solution : build a Chemical Synthesis Process Monitor Digital Twin Application. Which helped the operator choose the right process parameters to optimize yield. Based on pre-determined parameters or conditions, our digital twin solution can predict the quality of the drug yield. It can also monitor operations and alert the plant operators during a sudden failure. If the quality drops, floor managers will receive alerts on their mobiles. Operators can also track drops in quality in real-time through a large screen display on the plant floor. Operators can simulate the production of a batch using the digital twin solution and predict its quality even before manufacture. They can also change the parameters in the simulations to improve the quality of the successive batches. This helped save $2 Mn by improving the Golden Batch yield. Pharma Digital Twin Solutions: Real Impact • • • • • • • • 67% Reduction in Machine Set Up Time Manually setting up machines for drug production is time-consuming. It can also lead to monthly wastage of more than 10,000 tablets. Solution : develop a Pharma Digital Twin solution to automate the process for a global drug manufacturer. Operators can ensure correct tablet weight, thickness, and hardness using a data-driven approach. They can also set up the compressor correctly. Save $2 Mn by Improving the Golden Batch Yield 2.6% Increase in Golden Batch Yield A pharma major wanted to increase the yield quantity of their product. S