DBSCAN Clustering Slides PDF
Document Details
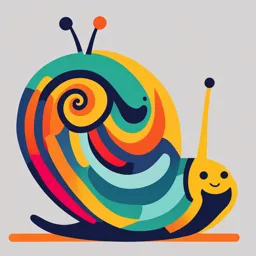
Uploaded by MonumentalTropicalIsland728
Kent State University
Tags
Summary
These slides explain the DBSCAN algorithm for clustering data points. DBSCAN is a density-based clustering algorithm that groups closely packed points together while identifying outliers. The algorithm relies on two main parameters, epsilon (ε) and minPoints. The slides provide examples and details on the algorithm.
Full Transcript
Let us now look at a non-parametric density clustering algorithm DBSCAN. It was proposed by Martin Ester, Hans-Peter Kriegel, Jörg Sander and Xiaowei Xu in 1996. 1 Given a set of points in some space, the algori...
Let us now look at a non-parametric density clustering algorithm DBSCAN. It was proposed by Martin Ester, Hans-Peter Kriegel, Jörg Sander and Xiaowei Xu in 1996. 1 Given a set of points in some space, the algorithm groups points that are closely packed, i.e., neighbors, together, while marking as outliers points that lie alone in low-density regions (whose nearest neighbors are too far away). The amount of density, and the closeness of the neighbors are values that are set by the modeler. 2 This dataset cannot be adequately clustered with k-means, but DBSCAN can find non- linearly separable clusters. Notice that the density of points within clusters is much higher than those outside of the clusters. 3 There are two main parameters that need to be defined. The first is epsilon, the parameter specifying the radius of a neighborhood with respect to some point, and minPoints, the number of points that should be contained within the neighborhood. 4 Points are classified as core, border, or outlier as follows. A point p is a core point if at least minPts points are within distance ε of it (including p). A point q is directly reachable from p if point q is within distance ε from core point p. Points are only said to be directly reachable from core points. A point q is reachable from p if there is a path p1,..., pn with p1 = p and pn = q, where each pi+1 is directly reachable from pi. Note that this implies that all points on the path must be core points, with the possible exception of q. These points we label as border points. All points not reachable from any other point are outliers or noise points. Now if p is a core point, then it forms a cluster together with all points (core or non- core) that are reachable from it. Each cluster contains at least one core point; non- core points can be part of a cluster, but they form its "edge", since they cannot be used to reach more points. 5 In this diagram, minPts = 4. Point A and the other red points are core points, because the area surrounding these points in an ε radius contain at least 4 points (including the point itself). Because they are all reachable from one another, they form a single cluster. Points B and C are not core points, but are reachable from A (via other core points) and thus belong to the cluster as well. Point N is a noise point that is neither a core point nor directly-reachable. 6 Reachability is not a symmetric relation since, by definition, no point may be reachable from a non-core point, regardless of distance (so a non-core point may be reachable, but nothing can be reached from it). In the diagram, p is a core point, but q is not. DBSCAN requires two parameters: ε (eps) and the minimum number of points required to form a dense region[a] (minPts). It starts with an arbitrary starting point that has not been visited. This point's ε-neighborhood is retrieved, and if it contains sufficiently many points, a cluster is started. Otherwise, the point is labeled as noise. Note that this point might later be found in a sufficiently sized ε-environment of a different point and hence be made part of a cluster. If a point is found to be a dense part of a cluster, its ε-neighborhood is also part of that cluster. Hence, all points that are found within the ε-neighborhood are added, as is their own ε-neighborhood when they are also dense. This process continues until the density-connected cluster is completely found. Then, a new unvisited point is retrieved and processed, leading to the discovery of a further cluster or noise. Here is an example of DBSCAN in action. Note that it is good at identifying non-linear regions, something that other clustering algorithms have problem doing. We will explore this further in the next module.