7.3 Correlation PDF
Document Details
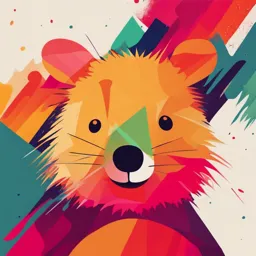
Uploaded by ObservantEuphemism2980
Technological Institute of the Philippines
CPE
Tags
Related
- Data Analytics (Digital) for Decision Making - Les Roches PDF
- Data Analytics (Digital) for Decision Making PDF
- Lecture 14 & 15_Regression and correlation_2022.pdf
- Correlation and Regression PDF
- Descriptive Statistics - Correlation and Regression PDF
- Cours Traitement Numérique du Signal et Ses Applications 2024-2025 - esiea PDF
Summary
This document discusses the concept of correlation, which is a mathematical operation similar to convolution. It uses two signals to produce a third signal, and is a key element of radar systems for signal detection. It explains how correlation works and how it's used to determine the presence of a specific signal in noisy data.
Full Transcript
10/15/24, 11:01 PM 7.3 Correlation: CPE 027-CPE41S8 - Digital Signal Processing and Application 7.3 Correlation The concept of correlation can best be presented with an example. Figure 7-13 shows the key elements of a radar system. A specially designed ante...
10/15/24, 11:01 PM 7.3 Correlation: CPE 027-CPE41S8 - Digital Signal Processing and Application 7.3 Correlation The concept of correlation can best be presented with an example. Figure 7-13 shows the key elements of a radar system. A specially designed antenna transmits a short burst of radio wave energy in a selected direction. If the propagating wave strikes an object, such as the helicopter in this illustration, a small fraction of the energy is reflected back toward a radio receiver located near the transmitter. The transmitted pulse is a specific shape that we have selected, such as the triangle shown in this example. The received signal will consist of two parts: (1) a shifted and scaled version of the transmitted pulse, and (2) random noise, resulting from interfering radio waves, thermal noise in the electronics, etc. Since radio signals travel at a known rate, the speed of light, the shift between the transmitted and received pulse is a direct measure of the distance to the object being detected. This is the problem: given a signal of some known shape, what is the best way to determine where (or if) the signal occurs in another signal. Correlation is the answer. Correlation is a mathematical operation that is very similar to convolution. Just as with convolution, correlation uses two signals to produce a third signal. This third signal is called the cross- correlation of the two input signals. If a signal is correlated with itself, the resulting signal is instead called the autocorrelation. The convolution machine was presented in the last chapter to show how convolution is performed. Figure 7-14 is a similar https://tip.instructure.com/courses/58221/pages/7-dot-3-correlation?module_item_id=5979184 1/4 10/15/24, 11:01 PM 7.3 Correlation: CPE 027-CPE41S8 - Digital Signal Processing and Application illustration of a correlation machine. The received signal, x[n], and the cross-correlation signal, y[n], are fixed on the page. The waveform we are looking for, t[n], commonly called the target signal, is contained within the correlation machine. Each sample in y[n] is calculated by moving the correlation machine left or right until it points to the sample being worked on. Next, the indicated samples from the received signal fall into the correlation machine, and are multiplied by the corresponding points in the target signal. The sum of these products then moves into the proper sample in the cross- correlation signal. The amplitude of each sample in the cross-correlation signal is a measure of how much the received signal resembles the target signal, at that location. This means that a peak will occur in the cross- correlation signal for every target signal that is present in the received signal. In other words, the value of the cross-correlation is maximized when the target signal is aligned with the same features in the received signal. What if the target signal contains samples with a negative value? Nothing changes. Imagine that the correlation machine is positioned such that the target signal is perfectly aligned with the matching https://tip.instructure.com/courses/58221/pages/7-dot-3-correlation?module_item_id=5979184 2/4 10/15/24, 11:01 PM 7.3 Correlation: CPE 027-CPE41S8 - Digital Signal Processing and Application waveform in the received signal. As samples from the received signal fall into the correlation machine, they are multiplied by their matching samples in the target signal. Neglecting noise, a positive sample will be multiplied by itself, resulting in a positive number. Likewise, a negative sample will be multiplied by itself, also resulting in a positive number. Even if the target signal is completely negative, the peak in the cross-correlation will still be positive. If there is noise on the received signal, there will also be noise on the cross-correlation signal. It is an unavoidable fact that random noise looks a certain amount like any target signal you can choose. The noise on the cross-correlation signal is simply measuring this similarity. Except for this noise, the peak generated in the cross-correlation signal is symmetrical between its left and right. This is true even if the target signal isn't symmetrical. In addition, the width of the peak is twice the width of the target signal. Remember, the cross-correlation is trying to detect the target signal, not recreate it. There is no reason to expect that the peak will even look like the target signal. Correlation is the optimal technique for detecting a known waveform in random noise. That is, the peak is higher above the noise using correlation than can be produced by any other linear system. (To be perfectly correct, it is only optimal for random white noise). Using correlation to detect a known waveform is frequently called matched filtering. More on this in Chapter 17. The correlation machine and convolution machine are identical, except for one small difference. As discussed in the last chapter, the signal inside of the convolution machine is flipped left-for-right. This means that samples numbers: 1, 2, 3 … run from the right to the left. In the correlation machine this flip doesn't take place, and the samples run in the normal direction. https://tip.instructure.com/courses/58221/pages/7-dot-3-correlation?module_item_id=5979184 3/4 10/15/24, 11:01 PM 7.3 Correlation: CPE 027-CPE41S8 - Digital Signal Processing and Application Since this signal reversal is the only difference between the two operations, it is possible to represent correlation using the same mathematics as convolution. This requires preflipping one of the two signals being correlated, so that the left-for-right flip inherent in convolution is canceled. For instance, when a[n] and b[n], are convolved to produce c[n], the equation is written: a[n] * b[n] = c[n]. In comparison, the cross-correlation of a[n] and b[n] can be written: a[n] * b[-n] = c[n]. That is, flipping b[n] left-for-right is accomplished by reversing the sign of the index, i.e., b[-n]. Don't let the mathematical similarity between convolution and correlation fool you; they represent very different DSP procedures. Convolution is the relationship between a system's input signal, output signal, and impulse response. Correlation is a way to detect a known waveform in a noisy background. The similar mathematics is only a convenient coincidence. https://tip.instructure.com/courses/58221/pages/7-dot-3-correlation?module_item_id=5979184 4/4 10/15/24, 11:00 PM 7.2 Mathematical Properties: CPE 027-CPE41S8 - Digital Signal Processing and Application 7.2 Mathematical Properties Commutative Property The commutative property for convolution is expressed in mathematical form: In words, the order in which two signals are convolved makes no difference; the results are identical. As shown in Fig. 7-8, this has a strange meaning for system theory. In any linear system, the input signal and the system's impulse response can be exchanged without changing the output signal. This is interesting, but usually doesn't have any physical meaning. The input signal and the impulse response are very different things. Just because the mathematics allows you to do something, doesn't mean that it makes sense to do it. For example, suppose you make: $10/hour ? 2,000 hours/year = $20,000/year. The commutative property for multiplication provides that you can make the same annual salary by only working 10 hours/year at $2000/hour. Let's see you convince your boss that this is meaningful! In spite of this, the commutative property sees great use in DSP for manipulating equations, just as in ordinary algebra. Associative Property Is it possible to convolve three or more signals? The answer is yes, and the associative property describes how: convolve two of the signals to produce an intermediate signal, then convolve the intermediate signal with the third signal. The associative property provides that the order of the convolutions doesn't matter. As an equation: https://tip.instructure.com/courses/58221/pages/7-dot-2-mathematical-properties?module_item_id=5979183 1/5 10/15/24, 11:00 PM 7.2 Mathematical Properties: CPE 027-CPE41S8 - Digital Signal Processing and Application The associative property is used in system theory to describe how cascaded systems behave. As shown in Fig. 7-9, two or more systems are said to be in a cascade if the output of one system is used as the input for the next system. From the associative property, the order of the systems can be rearranged without changing the overall response of the cascade. Further, any number of cascaded systems can be replaced with a single system. The impulse response of the replacement system is found by convolving the impulse responses of all of the original systems. Distributive Property In equation form, the distributive property is written: The distributive property describes the operation of parallel systems with added outputs. As shown in Fig. 7-10, two or more systems can share the same input, x[n], and have their outputs added to produce y[n]. The distributive property allows this combination of systems to be replaced with a single https://tip.instructure.com/courses/58221/pages/7-dot-2-mathematical-properties?module_item_id=5979183 2/5 10/15/24, 11:00 PM 7.2 Mathematical Properties: CPE 027-CPE41S8 - Digital Signal Processing and Application system, having an impulse response equal to the sum of the impulse responses of the original systems. Transference between the Input and Output Rather than being a formal mathematical property, this is a way of thinking about a common situation in signal processing. As illustrated in Fig. 7-11, imagine a linear system receiving an input signal, x[n], and generating an output signal, y[n]. Now suppose that the input signal is changed in some linear way, resulting in a new input signal, which we will call x´[n]. This results in a new output signal, y?[n]. The question is, how does the change in the input signal relate to the change in the output signal? The answer is: the output signal is changed in exactly the same linear way that the input signal was changed. For example, if the input signal is amplified by a factor of two, the output signal will also be amplified by a factor of two. If the derivative is taken of the input signal, the derivative will also be taken of the output signal. If the input is filtered in some way, the output will be filtered in an identical manner. This can easily be proven by using the associative property. https://tip.instructure.com/courses/58221/pages/7-dot-2-mathematical-properties?module_item_id=5979183 3/5 10/15/24, 11:00 PM 7.2 Mathematical Properties: CPE 027-CPE41S8 - Digital Signal Processing and Application The Central Limit Theorem The Central Limit Theorem is an important tool in probability theory because it mathematically explains why the Gaussian probability distribution is observed so commonly in nature. For example: the amplitude of thermal noise in electronic circuits follows a Gaussian distribution; the cross- sectional intensity of a laser beam is Gaussian; even the pattern of holes around a dart board bull's eye is Gaussian. In its simplest form, the Central Limit Theorem states that a Gaussian distribution results when the observed variable is the sum of many random processes. Even if the component processes do not have a Gaussian distribution, the sum of them will. The Central Limit Theorem has an interesting implication for convolution. If a pulse-like signal is convolved with itself many times, a Gaussian is produced. Figure 7-12 shows an example of this. The signal in (a) is an https://tip.instructure.com/courses/58221/pages/7-dot-2-mathematical-properties?module_item_id=5979183 4/5 10/15/24, 11:00 PM 7.2 Mathematical Properties: CPE 027-CPE41S8 - Digital Signal Processing and Application irregular pulse, purposely chosen to be very unlike a Gaussian. Figure (b) shows the result of convolving this signal with itself one time. Figure (c) shows the result of convolving this signal with itself three times. Even with only three convolutions, the waveform looks very much like a Gaussian. In mathematics jargon, the procedure converges to a Gaussian very quickly. The width of the resulting Gaussian (i.e., σ in Eq. 2-7 or 2-8) is equal to the width of the original pulse (expressed as σ in Eq. 2-7) multiplied by the square root of the number of convolutions. https://tip.instructure.com/courses/58221/pages/7-dot-2-mathematical-properties?module_item_id=5979183 5/5 10/15/24, 11:00 PM 7.1 Common Impulse Responses: CPE 027-CPE41S8 - Digital Signal Processing and Application 7.1 Common Impulse Responses A linear system's characteristics are completely specified by the system's impulse response, as governed by the mathematics of convolution. This is the basis of many signal processing techniques. For example: Digital filters are created by designing an appropriate impulse response. Enemy aircraft are detected with radar by analyzing a measured impulse response. Echo suppression in long distance telephone calls is accomplished by creating an impulse response that counteracts the impulse response of the reverberation. The list goes on and on. This chapter expands on the properties and usage of convolution in several areas. First, several common impulse responses are discussed. Second, methods are presented for dealing with cascade and parallel combinations of linear systems. Third, the technique of correlation is introduced. Forth, a nasty problem with convolution is examined, the computation time can be unacceptably long using conventional algorithms and computers. Delta Function The simplest impulse response is nothing more that a delta function, as shown in Fig. 7-1a. That is, an impulse on the input produces an identical impulse on the output. This means that all signals are passed through the system without change. Convolving any signal with a delta function results in exactly the same signal. Mathematically, this is written: This property makes the delta function the identity for convolution. This is analogous to zero being the identity for addition (a + 0 = a), and one being the identity for multiplication (a×1 = a). At first glance, this type of system may seem trivial and uninteresting. Not so! Such systems are the ideal for data storage, communication and measurement. Much of DSP is concerned with passing information through systems without change or degradation. Figure 7-1b shows a slight modification to the delta function impulse response. If the delta function is made larger or smaller in amplitude, the resulting system is an amplifier or attenuator, respectively. In equation form, amplification results if k is greater than one, and attenuation results if k is less than one: https://tip.instructure.com/courses/58221/pages/7-dot-1-common-impulse-responses?module_item_id=5979182 1/9 10/15/24, 11:00 PM 7.1 Common Impulse Responses: CPE 027-CPE41S8 - Digital Signal Processing and Application The impulse response in Fig. 7-1c is a delta function with a shift. This results in a system that introduces an identical shift between the input and output signals. This could be described as a signal delay, or a signal advance, depending on the direction of the shift. Letting the shift be represented by the parameter, s, this can be written as the equation: Science and engineering are filled with cases where one signal is a shifted version of another. For example, consider a radio signal transmitted from a remote space probe, and the corresponding signal received on the earth. The time it takes the radio wave to propagate over the distance causes a delay between the two signals. In biology, the electrical signals in adjacent nerve cells are shifted versions of each other, as determined by the time it takes an action potential to cross the synaptic junction that connects the two. Figure 7-1d shows an impulse response composed of a delta function plus a shifted and scaled delta function. By superposition, the output of this system is the input signal plus a delayed version of the input signal, i.e., an echo. Echoes are important in many DSP applications. The addition of echoes is a key part in making audio recordings sound natural and pleasant. Radar and sonar analyze echoes to detect aircraft and submarines. Geophysicists use echoes to find oil. Echoes are also very important in telephone networks, because you want to avoid them. https://tip.instructure.com/courses/58221/pages/7-dot-1-common-impulse-responses?module_item_id=5979182 2/9 10/15/24, 11:00 PM 7.1 Common Impulse Responses: CPE 027-CPE41S8 - Digital Signal Processing and Application Calculus-like Operations Convolution can change discrete signals in ways that resemble integration and differentiation. Since the terms "derivative" and "integral" specifically refer to operations on continuous signals, other names are given to their discrete counterparts. The discrete operation that mimics the first derivative is called the first difference. Likewise, the discrete form of the integral is called the running sum. It is also common to hear these operations called the discrete derivative and the discrete integral, although mathematicians frown when they hear these informal terms used. Figure 7-2 shows the impulse responses that implement the first difference and the running sum. Figure 7-3 shows an example using these operations. In 7-3a, the original signal is composed of several sections with varying slopes. Convolving this signal with the first difference impulse response produces the signal in Fig. 7-3b. Just as with the first derivative, the amplitude of each point in the https://tip.instructure.com/courses/58221/pages/7-dot-1-common-impulse-responses?module_item_id=5979182 3/9 10/15/24, 11:00 PM 7.1 Common Impulse Responses: CPE 027-CPE41S8 - Digital Signal Processing and Application first difference signal is equal to the slope at the corresponding location in the original signal. The running sum is the inverse operation of the first difference. That is, convolving the signal in (b), with the running sum's impulse response, produces the signal in (a). These impulse responses are simple enough that a full convolution program is usually not needed to implement them. Rather, think of them in the alternative mode: each sample in the output signal is a sum of weighted samples from the input. For instance, the first difference can be calculated: That is, each sample in the output signal is equal to the difference between two adjacent samples in the input signal. For instance, y = x - x. It should be mentioned that this is not the only way to define a discrete derivative. Another common method is to define the slope symmetrically around the point being examined, such as: y[n] = (x[n+1] - x[n-1])/2. https://tip.instructure.com/courses/58221/pages/7-dot-1-common-impulse-responses?module_item_id=5979182 4/9 10/15/24, 11:00 PM 7.1 Common Impulse Responses: CPE 027-CPE41S8 - Digital Signal Processing and Application Using this same approach, each sample in the running sum can be calculated by summing all points in the original signal to the left of the sample's location. For instance, if y[n] is the running sum of x[n], then sample y is found by adding samples x through x. Likewise, sample y is found by adding samples x through x. Of course, it would be very inefficient to calculate the running sum in this manner. For example, if y has already been calculated, y can be calculated with only a single addition: y = x + y. In equation form: Relations of this type are called recursion equations or difference equations. We will revisit them in Chapter 19. For now, the important idea to understand is that these relations are identical to convolution using the impulse responses of Fig. 7-2. Table 7-1 provides computer programs that implement these calculus-like operations. https://tip.instructure.com/courses/58221/pages/7-dot-1-common-impulse-responses?module_item_id=5979182 5/9 10/15/24, 11:00 PM 7.1 Common Impulse Responses: CPE 027-CPE41S8 - Digital Signal Processing and Application Low-pass and High-pass Filters The design of digital filters is covered in detail in later chapters. For now, be satisfied to understand the general shape of low-pass and high-pass filter kernels (another name for a filter's impulse response). Figure 7-4 shows several common low-pass filter kernels. In general, low-pass filter kernels are composed of a group of adjacent positive points. This results in each sample in the output signal being a weighted average of many adjacent points from the input signal. This averaging smoothes the signal, thereby removing high-frequency components. As shown by the sinc function in (c), some low-pass filter kernels include a few negative valued samples in the tails. Just as in analog electronics, digital low-pass filters are used for noise reduction, signal separation, wave shaping, etc. https://tip.instructure.com/courses/58221/pages/7-dot-1-common-impulse-responses?module_item_id=5979182 6/9 10/15/24, 11:00 PM 7.1 Common Impulse Responses: CPE 027-CPE41S8 - Digital Signal Processing and Application The cutoff frequency of the filter is changed by making filter kernel wider or narrower. If a low-pass filter has a gain of one at DC (zero frequency), then the sum of all of the points in the impulse response must be equal to one. As illustrated in (a) and (c), some filter kernels theoretically extend to infinity without dropping to a value of zero. In actual practice, the tails are truncated after a certain number of samples, allowing it to be represented by a finite number of points. How else could it be stored in a computer? Figure 7-5 shows three common high-pass filter kernels, derived from the corresponding low-pass filter kernels in Fig. 7-4. This is a common strategy in filter design: first devise a low-pass filter and then transform it to what you need, high-pass, band-pass, band-reject, etc. To understand the low- pass to high-pass transform, remember that a delta function impulse response passes the entire signal, while a low-pass impulse response passes only the low-frequency components. By superposition, a filter kernel consisting of a delta function minus the low-pass filter kernel will pass the entire signal minus the low-frequency components. A high-pass filter is born! As shown in Fig. 7- 5, the delta function is usually added at the center of symmetry, or sample zero if the filter kernel is not symmetrical. High-pass filters have zero gain at DC (zero frequency), achieved by making the sum of all the points in the filter kernel equal to zero. https://tip.instructure.com/courses/58221/pages/7-dot-1-common-impulse-responses?module_item_id=5979182 7/9 10/15/24, 11:00 PM 7.1 Common Impulse Responses: CPE 027-CPE41S8 - Digital Signal Processing and Application Causal and Noncausal Signals Imagine a simple analog electronic circuit. If you apply a short pulse to the input, you will see a response on the output. This is the kind of cause and effect that our universe is based on. One thing we definitely know: any effect must happen after the cause. This is a basic characteristic of what we call time. Now compare this to a DSP system that changes an input signal into an output signal, both stored in arrays in a computer. If this mimics a real world system, it must follow the same principle of causality as the real world does. For example, the value at sample number eight in the input signal can only affect sample number eight or greater in the output signal. Systems that operate in this manner are said to be causal. Of course, digital processing doesn't necessarily have to function this way. Since both the input and output signals are arrays of numbers stored in a computer, any of the input signal values can affect any of the output signal values. As shown by the examples in Fig. 7-6, the impulse response of a causal system must have a value of zero for all negative numbered samples. Think of this from the input side view of convolution. To be causal, an impulse in the input signal at sample number n must only affect those points in the output signal with a sample number of n or greater. In common usage, the term causal is applied to any signal where all the negative numbered samples have a value of zero, whether it is an impulse response or not. https://tip.instructure.com/courses/58221/pages/7-dot-1-common-impulse-responses?module_item_id=5979182 8/9 10/15/24, 11:00 PM 7.1 Common Impulse Responses: CPE 027-CPE41S8 - Digital Signal Processing and Application Zero Phase, Linear Phase, and Nonlinear Phase As shown in Fig. 7-7, a signal is said to be zero phase if it has left-right symmetry around sample number zero. A signal is said to be linear phase if it has left-right symmetry, but around some point other than zero. This means that any linear phase signal can be changed into a zero phase signal simply by shifting left or right. Lastly, a signal is said to be nonlinear phase if it does not have left-right symmetry. You are probably thinking that these names don't seem to follow from their definitions. What does phase have to do with symmetry? The answer lies in the frequency spectrum, and will be discussed in more detail in later chapters. Briefly, the frequency spectrum of any signal is composed of two parts, the magnitude and the phase. The frequency spectrum of a signal that is symmetrical around zero has a phase that is zero. Likewise, the frequency spectrum of a signal that is symmetrical around some nonzero point has a phase that is a straight line, i.e., a linear phase. Lastly, the frequency spectrum of a signal that is not symmetrical has a phase that is not a straight line, i.e., it has a nonlinear phase. A special note about the potentially confusing terms: linear and nonlinear phase. What does this have to do the concept of system linearity discussed in previous chapters? Absolutely nothing! System linearity is the broad concept that nearly all of DSP is based on (superposition, homogeneity, additivity, etc). Linear and nonlinear phase mean that the phase is, or is not, a straight line. In fact, a system must be linear even to say that the phase is zero, linear, or nonlinear. https://tip.instructure.com/courses/58221/pages/7-dot-1-common-impulse-responses?module_item_id=5979182 9/9 10/15/24, 11:00 PM 6.5 Sum of Weighted Inputs: CPE 027-CPE41S8 - Digital Signal Processing and Application 6.5 Sum of Weighted Inputs The characteristics of a linear system are completely described by its impulse response. This is the basis of the input side algorithm: each point in the input signal contributes a scaled and shifted version of the impulse response to the output signal. The mathematical consequences of this lead to the output side algorithm: each point in the output signal receives a contribution from many points in the input signal, multiplied by a flipped impulse response. While this is all true, it doesn't provide the full story on why convolution is important in signal processing. Look back at the convolution machine in Fig. 6-8, and ignore that the signal inside the dotted box is an impulse response. Think of it as a set of weighing coefficients that happen to be embedded in the flow diagram. In this view, each sample in the output signal is equal to a sum of weighted inputs. Each sample in the output is influenced by a region of samples in the input signal, as determined by what the weighing coefficients are chosen to be. For example, imagine there are ten weighing coefficients, each with a value of one-tenth. This makes each sample in the output signal the average of ten samples from the input. Taking this further, the weighing coefficients do not need to be restricted to the left side of the output sample being calculated. For instance, Fig. 6-8 shows y being calculated from: x, x, x and x. Viewing the convolution machine as a sum of weighted inputs, the weighing coefficients could be chosen symmetrically around the output sample. For example, y might receive contributions from: x, x, x, x and x. Using the same indexing notation as in Fig. 6-8, the weighing coefficients for these five inputs would be held in: h, h, h, h[-1] and h[-2]. In other words, the impulse response that corresponds to our selection of symmetrical weighing coefficients requires the use of negative indexes. We will return to this in the next chapter. Mathematically, there is only one concept here: convolution as defined by Eq. 6-1. However, science and engineering problems approach this single concept from two distinct directions. Sometimes you will want to think of a system in terms of what its impulse response looks like. Other times you will understand the system as a set of weighing coefficients. You need to become familiar with both views, and how to toggle between them. https://tip.instructure.com/courses/58221/pages/6-dot-5-sum-of-weighted-inputs?module_item_id=5979177 1/1 10/15/24, 11:00 PM 6.4 Output Side Algorithm: CPE 027-CPE41S8 - Digital Signal Processing and Application 6.4 Output Side Algorithm The first viewpoint of convolution analyzes how each sample in the input signal affects many samples in the output signal. In this second viewpoint, we reverse this by looking at individual samples in the output signal, and finding the contributing points from the input. This is important from both mathematical and practical standpoints. Suppose that we are given some input signal and impulse response, and want to find the convolution of the two. The most straightforward method would be to write a program that loops through the output signal, calculating one sample on each loop cycle. Likewise, equations are written in the form: y[n] = some combination of other variables. That is, sample n in the output signal is equal to some combination of the many values in the input signal and impulse response. This requires a knowledge of how each sample in the output signal can be calculated independently of all other samples in the output signal. The output side algorithm provides this information. Let's look an example of how a single point in the output signal is influenced by several points from the input. The example point we will use is y in Fig. 6-5. This point is equal to the sum of all the sixth points in the nine output components, shown in Fig. 6-6. Now, look closely at these nine output components and identify which can affect y. That is, find which of these nine signals contains a nonzero sample at the sixth position. Five of the output components only have added zeros (the diamond markers) at the sixth sample, and can therefore be ignored. Only four of the output components are capable of having a nonzero value in the sixth position. These are the output components generated from the input samples: x, x, x and x. By adding the sixth sample from each of these output components, y is determined as: y = xh + xh + xh + xh. That is, four samples from the input signal are multiplied by the four samples in the impulse response, and the products added. Figure 6-8 illustrates the output side algorithm as a convolution machine, a flow diagram of how convolution occurs. Think of the input signal, x[n], and the output signal, y[n], as fixed on the page. The convolution machine, everything inside the dashed box, is free to move left and right as needed. The convolution machine is positioned so that its output is aligned with the output sample being calculated. Four samples from the input signal fall into the inputs of the convolution machine. These values are multiplied by the indicated samples in the impulse response, and the products are added. This produces the value for the output signal, which drops into its proper place. For example, y[n] is shown being calculated from the four input samples: x, x, x, and x. To calculate y, the convolution machine moves one sample to the right. This results in another four samples entering the machine, x through x, and the value for y dropping into the proper place. This process is repeated for all points in the output signal needing to be calculated. https://tip.instructure.com/courses/58221/pages/6-dot-4-output-side-algorithm?module_item_id=5979176 1/6 10/15/24, 11:00 PM 6.4 Output Side Algorithm: CPE 027-CPE41S8 - Digital Signal Processing and Application The arrangement of the impulse response inside the convolution machine is very important. The impulse response is flipped left-for-right. This places sample number zero on the right, and increasingly positive sample numbers running to the left. Compare this to the normal impulse response in Fig. 6-5 to understand the geometry of this flip. Why is this flip needed? It simply falls out of the mathematics. The impulse response describes how each point in the input signal affects the output signal. This results in each point in the output signal being affected by points in the input signal weighted by a flipped impulse response. https://tip.instructure.com/courses/58221/pages/6-dot-4-output-side-algorithm?module_item_id=5979176 2/6 10/15/24, 11:00 PM 6.4 Output Side Algorithm: CPE 027-CPE41S8 - Digital Signal Processing and Application Figure 6-9 shows the convolution machine being used to calculate several samples in the output signal. This diagram also illustrates a real nuisance in convolution. In (a), the convolution machine is located fully to the left with its output aimed at. In this position, it is trying to receive input from samples: x[-3], x[-2], x[-1] and x. The problem is, three of these samples: x[-3], x[-2] and x[-1] do not exist! This same dilemma arises in (d), where the convolution machine tries to accept samples to the right of the defined input signal, points x, x and x. One way to handle this problem is by inventing the nonexistent samples. This involves adding samples to the ends of the input signal, with each of the added samples having a value of zero. This is called padding the signal with zeros. Instead of trying to access a nonexistent value, the convolution machine receives a sample that has a value of zero. Since this zero is eliminated during the multiplication, the result is mathematically the same as ignoring the nonexistent inputs. https://tip.instructure.com/courses/58221/pages/6-dot-4-output-side-algorithm?module_item_id=5979176 3/6 10/15/24, 11:00 PM 6.4 Output Side Algorithm: CPE 027-CPE41S8 - Digital Signal Processing and Application The important part is that the far left and far right samples in the output signal are based on incomplete information. In DSP jargon, the impulse response is not fully immersed in the input signal. If the impulse response is M points in length, the first and last M-1 samples in the output signal are based on less information than the samples between. This is analogous to an electronic circuit requiring a certain amount of time to stabilize after the power is applied. The difference is that this transient is easy to ignore in electronics, but very prominent in DSP. Figure 6-10 shows an example of the trouble these end effects can cause. The input signal is a sine wave plus a DC component. The desire is to remove the DC part of the signal, while leaving the sine wave intact. This calls for a high-pass filter, such as the impulse response shown in the figure. The problem is, the first and last 30 points are a mess! The shape of these end regions can be understood by imagining the input signal padded with 30 zeros on the left side, samples x[-1] through x[-30], and 30 zeros on the right, samples x through x. The output signal can then be viewed as a filtered version of this longer waveform. These "end effect" problems are widespread in DSP. As a general rule, expect that the beginning and ending samples in processed signals will be quite useless. Now the math. Using the convolution machine as a guideline, we can write the standard equation for convolution. If x[n] is an N point signal running from 0 to N-1, and h[n] is an M point signal running https://tip.instructure.com/courses/58221/pages/6-dot-4-output-side-algorithm?module_item_id=5979176 4/6 10/15/24, 11:00 PM 6.4 Output Side Algorithm: CPE 027-CPE41S8 - Digital Signal Processing and Application from 0 to M-1, the convolution of the two: y[n] = x[n] * h[n], is an N+M-1 point signal running from 0 to N+M-2, given by: This equation is called the convolution sum. It allows each point in the output signal to be calculated independently of all other points in the output signal. The index, i, determines which sample in the output signal is being calculated, and therefore corresponds to the left-right position of the convolution machine. In computer programs performing convolution, a loop makes this index run through each sample in the output signal. To calculate one of the output samples, the index, j, is used inside of the convolution machine. As j runs through 0 to M-1, each sample in the impulse response, h[j], is multiplied by the proper sample from the input signal, x[i-j]. All these products are added to produce the output sample being calculated. Study Eq. 6-1 until you fully understand how it is implemented by the convolution machine. Much of DSP is based on this equation. (Don't be confused by the n in y[n] = x[n] * h[n]. This is merely a place holder to indicate that some variable is the index into the array. Sometimes the equations are written: y[] = x[] * h[], just to avoid having to bring in a meaningless symbol). Table 6-2 shows a program for performing convolutions using the output side algorithm, a direct use of Eq. 6-1. This program produces the same output signal as the program for the input side algorithm, shown previously in Table 6-1. Notice the main difference between these two programs: the input side algorithm loops through each sample in the input signal (line 220 of Table 6-1), while the output side algorithm loops through each sample in the output signal (line 180 of Table 6-2). Here is a detailed operation of this program. The FOR-NEXT loop in lines 180 to 250 steps through each sample in the output signal, using I% as the index. For each of these values, an inner loop, composed of lines 200 to 230, calculates the value of the output sample, Y[I%]. The value of Y[I%] is set to zero in line 190, allowing it to accumulate the products inside of the convolution machine. The FOR-NEXT loop in lines 200 to 240 provide a direct implementation of Eq. 6-2. The index, J%, steps through each https://tip.instructure.com/courses/58221/pages/6-dot-4-output-side-algorithm?module_item_id=5979176 5/6 10/15/24, 11:00 PM 6.4 Output Side Algorithm: CPE 027-CPE41S8 - Digital Signal Processing and Application sample in the impulse response. Line 230 provides the multiplication of each sample in the impulse response, H[J%], with the appropriate sample from the input signal, X[I%-J%], and adds the result to the accumulator. In line 230, the sample taken from the input signal is: X[I%-J%]. Lines 210 and 220 prevent this from being outside the defined array, X to X. In other words, this program handles undefined samples in the input signal by ignoring them. Another alternative would be to define the input signal's array from X[-30] to X, allowing 30 zeros to be padded on each side of the true data. As a third alternative, the FOR-NEXT loop in line 180 could be changed to run from 30 to 80, rather than 0 to 110. That is, the program would only calculate the samples in the output signal where the impulse response is fully immersed in the input signal. The important thing is that you must use one of these three techniques. If you don't, the program will crash when it tries to read the out-of-bounds data. https://tip.instructure.com/courses/58221/pages/6-dot-4-output-side-algorithm?module_item_id=5979176 6/6 10/15/24, 10:59 PM 6.3 Input Side Algorithm: CPE 027-CPE41S8 - Digital Signal Processing and Application 6.3 Input Side Algorithm Figure 6-5 shows a simple convolution problem: a 9 point input signal, x[n], is passed through a system with a 4 point impulse response, h[n], resulting in a 9 + 4 - 1 = 12 point output signal, y[n]. In mathematical terms, x[n] is convolved with h[n] to produce y[n]. This first viewpoint of convolution is based on the fundamental concept of DSP: decompose the input, pass the components through the system, and synthesize the output. In this example, each of the nine samples in the input signal will contribute a scaled and shifted version of the impulse response to the output signal. These nine signals are shown in Fig. 6-6. Adding these nine signals produces the output signal, y[n]. Let's look at several of these nine signals in detail. We will start with sample number four in the input signal, i.e., x. This sample is at index number four, and has a value of 1.4. When the signal is decomposed, this turns into an impulse represented as: 1.4δ[n-4]. After passing through the system, the resulting output component will be: 1.4h[n-4]. This signal is shown in the center box of the nine signals in Fig. 6-6. Notice that this is the impulse response, h[n], multiplied by 1.4, and shifted four samples to the right. Zeros have been added at samples 0-3 and at samples 8-11 to serve as place holders. To make this more clear, Fig. 6-6 uses squares to represent the data points that come from the shifted and scaled impulse response, and diamonds for the added zeros. Now examine sample x, the last point in the input signal. This sample is at index number eight, and has a value of -0.5. As shown in the lower-right graph of Fig. 6-6, x results in an impulse response that has been shifted to the right by eight points and multiplied by -0.5. Place holding zeros have been added at points 0-7. Lastly, examine the effect of points x and x. Both these samples have a value of zero, and therefore produce output components consisting of all zeros. https://tip.instructure.com/courses/58221/pages/6-dot-3-input-side-algorithm?module_item_id=5979175 1/4 10/15/24, 10:59 PM 6.3 Input Side Algorithm: CPE 027-CPE41S8 - Digital Signal Processing and Application In this example, is a nine point signal and is a four point signal. In our next example, shown in Fig. 6- 7, we will reverse the situation by making a four point signal, and a nine point signal. The same two waveforms are used, they are just swapped. As shown by the output signal components, the four samples in result in four shifted and scaled versions of the nine point impulse response. Just as before, leading and trailing zeros are added as place holders. But wait just one moment! The output signal in Fig. 6-7 is identical to the output signal in Fig. 6-5. This isn't a mistake, but an important property. Convolution is commutative: a[n]*b[n] = b[n]*a[n]. The mathematics does not care which is the input signal and which is the impulse response, only that two signals are convolved with each other. Although the mathematics may allow it, exchanging the two signals has no physical meaning in system theory. The input signal and impulse response are two totally different things and exchanging them doesn't make sense. What the commutative property provides is a mathematical tool for manipulating equations to achieve various results. A program for calculating convolutions using the input side algorithm is shown in Table 6-1. Remember, the programs in this book are meant to convey algorithms in the simplest form, even at the expense of good programming style. For instance, all of the input and output is handled in mythical subroutines (lines 160 and 280), meaning we do not define how these operations are conducted. Do not skip over these programs; they are a key part of the material and you need to understand them in detail. https://tip.instructure.com/courses/58221/pages/6-dot-3-input-side-algorithm?module_item_id=5979175 2/4 10/15/24, 10:59 PM 6.3 Input Side Algorithm: CPE 027-CPE41S8 - Digital Signal Processing and Application The program convolves an 81 point input signal, held in array X[ ], with a 31 point impulse response, held in array H[ ], resulting in a 111 point output signal, held in array Y[ ]. These are the same lengths shown in Figs. 6-3 and 6-4. Notice that the names of these arrays use upper case letters. This is a violation of the naming conventions previously discussed, because upper case letters are reserved for frequency domain signals. Unfortunately, the simple BASIC used in this book does not allow lower case variable names. Also notice that line 240 uses a star for multiplication. Remember, a star in a program means multiplication, while a star in an equation means convolution. A star in text (such as documentation or program comments) can mean either. The mythical subroutine in line 160 places the input signal into X[ ] and the impulse response into H[ ]. Lines 180-200 set all of the values in Y[ ] to zero. This is necessary because Y[ ] is used as an accumulator to sum the output components as they are calculated. Lines 220 to 260 are the heart of the program. The FOR statement in line 220 controls a loop that steps through each point in the input signal, X[ ]. For each sample in the input signal, an inner loop (lines 230-250) calculates a scaled and shifted version of the impulse response, and adds it to the array accumulating the output signal, Y[ ]. This nested loop structure (one loop within another loop) is a key characteristic of convolution programs; become familiar with it. https://tip.instructure.com/courses/58221/pages/6-dot-3-input-side-algorithm?module_item_id=5979175 3/4 10/15/24, 10:59 PM 6.3 Input Side Algorithm: CPE 027-CPE41S8 - Digital Signal Processing and Application Keeping the indexing straight in line 240 can drive you crazy! Let's say we are halfway through the execution of this program, so that we have just begun action on sample X, i.e., I% = 40. The inner loop runs through each point in the impulse response doing three things. First, the impulse response is scaled by multiplying it by the value of the input sample. If this were the only action taken by the inner loop, line 240 could be written, Y[J%] = X✳H[J%]. Second, the scaled impulse is shifted 40 samples to the right by adding this number to the index used in the output signal. This second action would change line 240 to: Y[40+J%] = X*H[J%]. Third, Y[ ] must accumulate (synthesize) all the signals resulting from each sample in the input signal. Therefore, the new information must be added to the information that is already in the array. This results in the final command: Y[40+J%] = Y[40+J%] + X*H[J%]. Study this carefully; it is very confusing, but very important. https://tip.instructure.com/courses/58221/pages/6-dot-3-input-side-algorithm?module_item_id=5979175 4/4 10/15/24, 10:48 PM 6.2 Convolution: CPE 027-CPE41S8 - Digital Signal Processing and Application 6.2 Convolution Let's summarize this way of understanding how a system changes an input signal into an output signal. First, the input signal can be decomposed into a set of impulses, each of which can be viewed as a scaled and shifted delta function. Second, the output resulting from each impulse is a scaled and shifted version of the impulse response. Third, the overall output signal can be found by adding these scaled and shifted impulse responses. In other words, if we know a system's impulse response, then we can calculate what the output will be for any possible input signal. This means we know everything about the system. There is nothing more that can be learned about a linear system's characteristics. (However, in later chapters we will show that this information can be represented in different forms). The impulse response goes by a different name in some applications. If the system being considered is a filter, the impulse response is called the filter kernel, the convolution kernel, or simply, the kernel. In image processing, the impulse response is called the point spread function. While these terms are used in slightly different ways, they all mean the same thing, the signal produced by a system when the input is a delta function. Convolution is a formal mathematical operation, just as multiplication, addition, and integration. Addition takes two numbers and produces a third number, while convolution takes two signals and produces a third signal. Convolution is used in the mathematics of many fields, such as probability and statistics. In linear systems, convolution is used to describe the relationship between three signals of interest: the input signal, the impulse response, and the output signal. Figure 6-2 shows the notation when convolution is used with linear systems. An input signal, x[n], enters a linear system with an impulse response, h[n], resulting in an output signal, y[n]. In equation https://tip.instructure.com/courses/58221/pages/6-dot-2-convolution?module_item_id=5979174 1/4 10/15/24, 10:48 PM 6.2 Convolution: CPE 027-CPE41S8 - Digital Signal Processing and Application form: x[n] * h[n] = y[n]. Expressed in words, the input signal convolved with the impulse response is equal to the output signal. Just as addition is represented by the plus, +, and multiplication by the cross, ×, convolution is represented by the star, *. It is unfortunate that most programming languages also use the star to indicate multiplication. A star in a computer program means multiplication, while a star in an equation means convolution. Figure 6-3 shows convolution being used for low-pass and high-pass filtering. The example input signal is the sum of two components: three cycles of a sine wave (representing a high frequency), plus a slowly rising ramp (composed of low frequencies). In (a), the impulse response for the low- pass filter is a smooth arch, resulting in only the slowly changing ramp waveform being passed to the output. Similarly, the high-pass filter, (b), allows only the more rapidly changing sinusoid to pass. Figure 6-4 illustrates two additional examples of how convolution is used to process signals. The inverting attenuator, (a), flips the signal top-for-bottom, and reduces its amplitude. The discrete https://tip.instructure.com/courses/58221/pages/6-dot-2-convolution?module_item_id=5979174 2/4 10/15/24, 10:48 PM 6.2 Convolution: CPE 027-CPE41S8 - Digital Signal Processing and Application derivative (also called the first difference), shown in (b), results in an output signal related to the slope of the input signal. Notice the lengths of the signals in Figs. 6-3 and 6-4. The input signals are 81 samples long, while each impulse response is composed of 31 samples. In most DSP applications, the input signal is hundreds, thousands, or even millions of samples in length. The impulse response is usually much shorter, say, a few points to a few hundred points. The mathematics behind convolution doesn't restrict how long these signals are. It does, however, specify the length of the output signal. The length of the output signal is equal to the length of the input signal, plus the length of the impulse response, minus one. For the signals in Figs. 6-3 and 6-4, each output signal is: 81 + 31 - 1 = 111 samples long. The input signal runs from sample 0 to 80, the impulse response from sample 0 to 30, and the output signal from sample 0 to 110. Now we come to the detailed mathematics of convolution. As used in Digital Signal Processing, convolution can be understood in two separate ways. The first looks at convolution from the viewpoint of the input signal. This involves analyzing how each sample in the input signal contributes to many points in the output signal. The second way looks at convolution from the viewpoint of the output signal. This examines how each sample in the output signal has received information from many points in the input signal. https://tip.instructure.com/courses/58221/pages/6-dot-2-convolution?module_item_id=5979174 3/4 10/15/24, 10:48 PM 6.2 Convolution: CPE 027-CPE41S8 - Digital Signal Processing and Application Keep in mind that these two perspectives are different ways of thinking about the same mathematical operation. The first viewpoint is important because it provides a conceptual understanding of how convolution pertains to DSP. The second viewpoint describes the mathematics of convolution. This typifies one of the most difficult tasks you will encounter in DSP: making your conceptual understanding fit with the jumble of mathematics used to communicate the ideas. https://tip.instructure.com/courses/58221/pages/6-dot-2-convolution?module_item_id=5979174 4/4 10/15/24, 10:47 PM 6.1 Delta Function and Impulse Response: CPE 027-CPE41S8 - Digital Signal Processing and Application 6.1 Delta Function and Impulse Response Convolution is a mathematical way of combining two signals to form a third signal. It is the single most important technique in Digital Signal Processing. Using the strategy of impulse decomposition, systems are described by a signal called the impulse response. Convolution is important because it relates the three signals of interest: the input signal, the output signal, and the impulse response. This chapter presents convolution from two different viewpoints, called the input side algorithm and the output side algorithm. Convolution provides the mathematical framework for DSP; there is nothing more important in this book. The previous chapter describes how a signal can be decomposed into a group of components called impulses. An impulse is a signal composed of all zeros, except a single nonzero point. In effect, impulse decomposition provides a way to analyze signals one sample at a time. The previous chapter also presented the fundamental concept of DSP: the input signal is decomposed into simple additive components, each of these components is passed through a linear system, and the resulting output components are synthesized (added). The signal resulting from this divide-and-conquer procedure is identical to that obtained by directly passing the original signal through the system. While many different decompositions are possible, two form the backbone of signal processing: impulse decomposition and Fourier decomposition. When impulse decomposition is used, the procedure can be described by a mathematical operation called convolution. In this chapter (and most of the following ones) we will only be dealing with discrete signals. Convolution also applies to continuous signals, but the mathematics is more complicated. We will look at how continious signals are processed in Chapter 13. Figure 6-1 defines two important terms used in DSP. The first is the delta function, symbolized by the Greek letter delta, δ[n]. The delta function is a normalized impulse, that is, sample number zero has a value of one, while all other samples have a value of zero. For this reason, the delta function is frequently called the unit impulse. https://tip.instructure.com/courses/58221/pages/6-dot-1-delta-function-and-impulse-response 1/2 10/15/24, 10:47 PM 6.1 Delta Function and Impulse Response: CPE 027-CPE41S8 - Digital Signal Processing and Application The second term defined in Fig. 6-1 is the impulse response. As the name suggests, the impulse response is the signal that exits a system when a delta function (unit impulse) is the input. If two systems are different in any way, they will have different impulse responses. Just as the input and output signals are often called x[n] and y[n], the impulse response is usually given the symbol, h[n]. Of course, this can be changed if a more descriptive name is available, for instance, f[n] might be used to identify the impulse response of a filter. Any impulse can be represented as a shifted and scaled delta function. Consider a signal, a[n], composed of all zeros except sample number 8, which has a value of -3. This is the same as a delta function shifted to the right by 8 samples, and multiplied by -3. In equation form: a[n] = -3δ[n-8]. Make sure you understand this notation, it is used in nearly all DSP equations. If the input to a system is an impulse, such as -3δ[n-8], what is the system's output? This is where the properties of homogeneity and shift invariance are used. Scaling and shifting the input results in an identical scaling and shifting of the output. If δ[n] results in h[n], it follows that -3δ[n-8] results in -3h[n- 8]. In words, the output is a version of the impulse response that has been shifted and scaled by the same amount as the delta function on the input. If you know a system's impulse response, you immediately know how it will react to any impulse. https://tip.instructure.com/courses/58221/pages/6-dot-1-delta-function-and-impulse-response 2/2 Chapter 4 - Linear system reacts when the Superposition - break down then add; signals arent changing divide and conquer - It passes to 0 from the origin - Solve individually before combining - All linear system is static but not all SIgnal vs System static is linear Signal - Parameter to another parameter Sinusoidal Fidelity System - Process produces output signal in - Sine waves = sine waves response to input signal - Sine input/output Continuos system - i/o continuous signal - Clipping (limiting) system is not Discrete system - i/o discrete signal linear Convention Attenuation system - downscaling CS - uses parenthesis = x(t) Commutative Property DS - use brackets = x[n] - Commute; can be arranged; can be FD - use upper case letters shift as long as system a and b are Signal - use lower case linear Linear System - Can be commutative even a lot of ECG, radio transmitter systems as long as the systems are When to know if the signal is linear? linear - Homogeneity - change in input Multiplication result in the output; same input/out - If you multiply by a constant; if not ;v(r) and the output it’s not linear even if your system is ;P = IV - LS linear ;P = V^2 - NLS Superposition ;P = V^2/R - Identical to the one produced by - Additivity - input of x1[n] output of directly passing y2[n] equals the summation of input - Y[n] = x[n] - LS and output - Y[n] = x^2[n] - NLS - Shift invariance - additional - Y[n] = 2x[n-2]+5 - NLS property; will result nothing more - Y[n] = x[n+1] - x[n-1] - LS than an identical shift; any value that Decomposition - breaking down of signals you shift, it should be the value of output signal; Synthesis - Indiv to another signal As long there’s 1 property the signal is STatic - no memory linear - No input depends to output Linear Time Invariance - N = n0 - Time shifting Causality - depicts only on the present and - If and only if there's an advance or past value delay - Both y[n]=x[n]-x[n-1] Another Systems When you compare the input to - Differentiator - delayed output it must be the same - Time multiplier - amplitude scaling y[n]=nx[n] NOT LTI Static Linearity Stability Convolution - mathematical way of combining two signals to form a third signal -impulse decomposition - all 0s except to non 0 ; breakdown of signal Delta function ;normalized impulse Impulse response ;input = impulse Convolution Identity property - siya pa rin x[n]*delta[n] = x[n] Amplification and attenuation