Chapter 2 - 03 - Understand Network-level Attacks - 09_ocred.pdf
Document Details
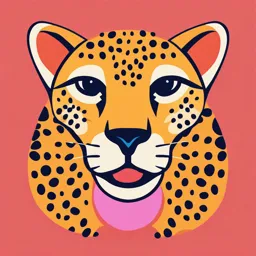
Uploaded by barrejamesteacher
null
EC
Tags
Full Transcript
Certified Cybersecurity Technician Information Security Attacks Exam 212-82 Adversarial Artificial Intelligence (AI) @ T QO Adversarial artificial intelligence is a new technology attack vector designed by attackers with malicious intent to mislead ML models O Attackers leverage the flaws in ML syst...
Certified Cybersecurity Technician Information Security Attacks Exam 212-82 Adversarial Artificial Intelligence (AI) @ T QO Adversarial artificial intelligence is a new technology attack vector designed by attackers with malicious intent to mislead ML models O Attackers leverage the flaws in ML systems and introduce malicious traffic into the target ML systems to gain control over the network Tainted Training Data for Machine Learning +*+ Tainted training data means infecting or contaminating the training data of machine learning models * «* Attackers can contaminate the data with malicious inputs that can disrupt the system performance and cause disturbances in retraining Security of Machine Learning Algorithms %+ The primary task in securing ML algorithms involves securing the dataset used for training the ML system. The potential ML security risks include: v’ Confidentiality of Data v Manipulating the Online System v Making False Predictions v’ Poisoning the Data v’ Transfer Learning Attack Copyright © by EC L All Rights Reserved. Reproductionis Strictly Prohibited. 1 Adversarial Artificial Intelligence (AI) Adversarial artificial intelligence (Al) is a new technology attack vector designed by attackers with malicious intent to mislead machine learning (ML) models. It can be implemented by changing the system inputs and converting the system behavior to favor the attackers. Attackers can also leverage the flaws in ML systems and inject malicious traffic into legitimate ones for holding persistence on the network. To trigger such attacks on ML models, attackers use custom Al resources as weapons, referred to as adversarial Al. Al is a crucial component to defend against the latest cyberattacks. It automates most of the tasks while securing the infrastructure from cyberattacks with deep learning capabilities and expedites data processing. However, attackers can misuse the capabilities of Al by creating adversarial examples and giving false inputs that resemble normal inputs, which change the behavior of the security model and deteriorates its performance. * Tainted Training Data for Machine Learning Tainted training data implies infecting or contaminating the training data of ML models. ML systems utilize the operational data aggregated during retraining operations. For example, security solutions such as intrusion detection systems use operational data to learn and retrain to defend against future cyberattacks. Attackers can also contaminate the data with malicious inputs that can disrupt the system performance and cause disturbances in retraining. When the training data are tainted, the machine learning algorithm is retrained with the malicious data and acts according to the instructions of the attacker. Module 02 Page 219 Certified Cybersecurity Technician Copyright © by EC-Gouncil All Rights Reserved. Reproduction is Strictly Prohibited. Certified Cybersecurity Technician Information Security Attacks = Exam 212-82 Security of Machine Learning Algorithms It is important to ensure the security of ML algorithms, similar to software applications. The primary task in securing ML algorithms involves securing the dataset used for training the ML system. Researchers have stated that 60% of the risks associated with ML algorithms and systems can be attributed to their training dataset. The potential ML security risks are listed below: o] Confidentiality of Data It is difficult to maintain data confidentiality, especially for the data used by ML systems for training. Attackers might perform sophisticated attacks to exfiltrate confidential data from ML systems while training. To overcome this risk, it is essential to build security protocols from the initial phase of an ML life cycle. Manipulating the Online System ML systems are generally built online, especially when learning and updating their behavior during operational use. can mislead an ML system by providing wrong inputs. To security team must select the right algorithm and secure systems. they are continuously A highly skilled attacker alleviate this issue, the the operations of ML Making False Predictions Attackers can fool ML system models with malicious inputs that resemble genuine inputs, thus corrupting the ML system. Attackers can send deceptive images to systems that enable incorrect learning processes. Such attacks are associated with high risks that can lead to system malfunction. Poisoning the Data ML systems usually rely on operational data for learning and retraining. If attackers can alter the operational data, then they can compromise the entire ML system. Therefore, ML engineers must secure all training data sources and focus on those sources with high potential risks. Transfer Learning Attack Transfer learning attacks are most common in ML systems if the system is fine-tuned with some common or pre-trained capabilities. If a pre-trained model is stored on a public repository, an attacker can use it to conceal the behavior with their malicious ML. Hence, while using transfer models, users must check the functions of the trained model and the controls that can be implemented by developers to mitigate risks. Module 02 Page 220 Certified Cybersecurity Technician Copyright © by EG-Council All Rights Reserved. Reproduction is Strictly Prohibited.