More on Experiments: Confounding and Obscuring Variables PDF
Document Details
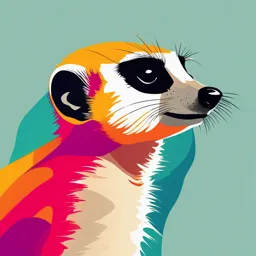
Uploaded by MagicBoron
Tags
Summary
This document discusses internal validity threats in experimental research. It examines various threats, including design confounds, selection effects, order effects, and maturation threats, illustrating how these factors can obscure the impact of an independent variable. It also details how to avoid these threats and interpret null results in experiments.
Full Transcript
11 More on Experiments: Confounding and Obscuring Variables More Information “Was it really the intervention, or something else, that caused things to improve?” More Information “How should we interpret a null result?” LEARNING OBJECTIVES A year from now, you should still be able to...
11 More on Experiments: Confounding and Obscuring Variables More Information “Was it really the intervention, or something else, that caused things to improve?” More Information “How should we interpret a null result?” LEARNING OBJECTIVES A year from now, you should still be able to: 1. Interrogate a study and decide whether it rules out twelve potential threats to internal validity. 2. Describe how researchers can design studies to prevent internal validity threats. 3. Interrogate an experiment with a null result to decide whether the study design obscured an effect or whether there is truly no effect to find. 4. Describe how researchers can design studies to minimize possible obscuring factors. CHAPTER 10 COVERED THE basic structure of an experiment, and this chapter addresses a number of questions about experimental design. Why is it so important to use a comparison group? Why do many experimenters create a standardized, controlled, seemingly artificial environment? Why do researchers use so many participants? Why do they often use special technologies to measure their variables? Why do they insist on double- blind study designs? For the clearest possible results, responsible researchers specifically design their experiments with many factors in mind. They want to correctly estimate real effects, and they want to determine conclusively when their predictions are wrong. The first section of this chapter describes potential internal validity problems and how researchers usually avoid them. The second section discusses some of the reasons experiments may yield null results. THREATS TO INTERNAL VALIDITY: DID THE INDEPENDENT VARIABLE REALLY CAUSE THE DIFFERENCE? When you interrogate an experiment, internal validity is the priority. As discussed in Chapter 10, three possible threats to internal validity include design confounds, selection effects, and order effects. All three of these threats involve an alternative explanation for the results. With a design confound, there is an alternative explanation because the experiment was poorly designed; another variable happened to vary systematically along with the intended independent variable. Chapter 10 presented the study on pen versus laptop notetaking and comprehension. If the test questions assigned to the laptop condition were more difficult than the those assigned to the pen condition, that would have been a design confound (see Figure 10.5). It would not be clear whether the notetaking format or the difficulty of the questions caused the handwritten notes group to score better. With a selection effect, a confound exists because the different independent variable groups have systematically different types of participants. In Chapter 10, the example was a study of an intensive therapy for autism, in which children who received the intensive treatment did improve over time. However, we are not sure if their improvement was caused by the therapy or by greater overall involvement on the part of the parents who elected to be in the intensive-treatment group. Those parents’ greater motivation could have been an alternative explanation for the improvement of children in the intensive-treatment group. With an order effect (in a within-groups design), there is an alternative explanation because the outcome might be caused by the independent variable, but it might also be caused by the order in which the levels of the variable are presented. When there is an order effect, we do not know whether the independent variable is really having an effect, or whether the participants are just getting tired, bored, or well-practiced. These types of threats are just the beginning. There are other ways—about twelve in total—in which a study might be at risk for a confound. Experimenters think about all of them, and they plan studies to avoid them. Normally, a well-designed experiment can prevent these threats and make strong causal statements (Shadish et al., 2002). The Really Bad Experiment (A Cautionary Tale) Previous chapters have used examples of published studies to illustrate the material. In contrast, this chapter presents three fictional experiments. You will rarely encounter published studies like these because, unlike the designs in Chapter 10, the basic design behind these examples has so many internal validity problems. Nikhil, a summer camp counselor and psychology major, has noticed that his current cabin of 15 boys is an especially rowdy bunch. He’s heard a change in diet might help them calm down, so he eliminates the sugary snacks and desserts from their meals for 2 days. As he expected, the boys are much quieter and calmer by the end of the week, after refined sugar has been eliminated from their diets. Dr. Yuki has recruited a sample of 40 depressed women, all of whom are interested in receiving psychotherapy to treat their depression. She measures their level of depression using a standard depression inventory at the start of therapy. For 12 weeks, all the women participate in Dr. Yuki’s style of cognitive therapy. At the end of the 12-week session, she measures the women again and finds that, on the whole, their levels of depression have significantly decreased. A dormitory on a university campus has started a Go Green social media campaign, focused on persuading students to turn out the lights in their rooms when they’re not needed. Dorm residents receive emails and see posts on social media that encourage energy-saving behaviors. At the start of the campaign, the head resident noted how many kilowatt hours the dorm was using by checking the electric meters on the building. At the end of the 2-month campaign, the head resident checks the meters again and finds that the usage has dropped. He compares the two measures (pretest and posttest) and finds they are significantly different. Notice that all three of these examples fit the same template, as shown in Figure 11.1. If you graphed the data of the first two studies, they would look something like the two graphs in Figure 11.2. Consider the three examples: What alternative explanations can you think of for the results of each one? More Information Figure 11.1 The really bad experiment. (A) A general diagram of the really bad experiment, or the one-group, pretest/posttest design. Unlike the pretest/posttest design, it has only one group: no comparison condition. (B, C) Possible ways to diagram two of the examples given in the text. Using these as a model, try sketching a diagram of the Go Green example. More Information Figure 11.2 Graphing the really bad experiment. The first two examples can be graphed this way. Using these as a model, try sketching a graph of the Go Green example. The formal name for this kind of design is the one-group, pretest/posttest design. A researcher recruits one group of participants; measures them on a pretest; exposes them to a treatment, intervention, or change; and then measures them on a posttest. This design differs from the true pretest/posttest design you learned in Chapter 10 because it has only one group, not two. There is no comparison group. Therefore, a better name for this design might be “the really bad experiment.” Understanding why this design is problematic can help you learn about threats to internal validity and how to avoid them with better designs. Six Potential Internal Validity Threats in One- Group, Pretest/Posttest Designs By the end of this chapter, you will have learned a total of 12 internal validity threats. Three of them we just reviewed: design confounds, selection effects, and order effects. Several of the internal validity threats apply especially to the really bad experiment, but they can be prevented with a good experimental design. These include maturation threats, history threats, regression threats, attrition threats, testing threats, and instrumentation threats. The final three threats (observer bias, demand characteristics, and placebo effects) potentially apply to any study. MATURATION THREATS TO INTERNAL VALIDITY Why did the boys in Nikhil’s cabin start behaving better? Was it truly because they had eaten less sugar? Perhaps. An alternative explanation, however, is that most of them simply settled in, or “matured into,” the camp setting after they got used to the place. The boys’ behavior improved on its own; the low-sugar diet may have had nothing to do with it. Such an effect is called a maturation threat, a change in behavior that emerges more or less spontaneously over time. People adapt to changed environments; children get better at walking and talking; plants grow taller —but not because of any outside intervention. It just happens. Similarly, the depressed women may have improved because the cognitive therapy was effective, but an alternative explanation is that a systematically high portion of them simply improved on their own. Sometimes the symptoms of depression or other disorders disappear, for no known reason, with time. This phenomenon, known as spontaneous remission, is a specific type of maturation. Preventing Maturation Threats. Because both Nikhil and Dr. Yuki conducted studies following the model of the really bad experiment, there is no way of knowing whether the improvements they noticed were caused by maturation or by the treatments they administered. In contrast, if the two researchers had conducted true experiments (such as a pretest/posttest design, which, as you learned in Chapter 10, has at least two groups, not one), they would also have included an appropriate comparison group. Nikhil would have observed a comparison group of equally lively campers who did not switch to a low-sugar diet. Dr. Yuki would have studied a comparison group of women who started out equally depressed but did not receive the cognitive therapy. If the treatment groups improved significantly more than the comparison groups did, these researchers could essentially subtract out the effect of maturation when they interpret their results. Figure 11.3 illustrates the benefits of a comparison group in preventing a maturation threat for the depression study. More Information Figure 11.3 Maturation threats. A pretest/posttest design would help control for the maturation threat in Dr. Yuki’s depression study. HISTORY THREATS TO INTERNAL VALIDITY Sometimes a threat to internal validity occurs not just because time has passed, but because something specific has happened between the pretest and posttest. In the third example, why did the dorm residents use less electricity? Was it the Go Green campaign? Perhaps. But a plausible alternative explanation is that the weather got cooler and most residents did not use air conditioning as much. Why did the campers’ behavior improve? It could have been the low-sugar diet, but maybe they all started a difficult swimming course in the middle of the week and the exercise tired most of them out. These alternative explanations are examples of history threats, which result from a “historical” or external factor that systematically affects most members of the treatment group at the same time as the treatment itself, making it unclear whether the change is caused by the treatment received. To be a history threat, the external factor must affect most people in the group in the same direction (systematically), not just a few people (unsystematically). ❯❯ For more on pretest/posttest design, see Chapter 10, pp. 293–294. Preventing History Threats. As with maturation threats, a comparison group can help control for history threats. In the Go Green study, the students would need to measure the kilowatt usage in another, comparable dormitory during the same 2 months, but not give the students in the second dorm the Go Green campaign materials. (This would be a pretest/posttest design rather than a one-group, pretest/posttest design.) If both groups decreased their electricity usage about the same over time (Figure 11.4A), the decrease probably resulted from the change of seasons, not from the Go Green campaign. However, if the treatment group decreased its usage more than the comparison group did (Figure 11.4B), you can rule out the history threat. Both the comparison group and the treatment group experienced the same seasonal “historical” changes, so including the comparison group controls for this threat. More Information Figure 11.4 History threats. A comparison group would help control for the history threat of seasonal differences in electricity usage. REGRESSION THREATS TO INTERNAL VALIDITY ❯❯ For more detail on arithmetic mean, see Statistics Review: Descriptive Statistics, p. 471. A regression threat refers to a statistical concept called regression to the mean. When a group average (mean) is unusually extreme at Time 1, the next time that group is measured (Time 2), it is likely to be less extreme— closer to its typical or average performance. Everyday Regression to the Mean. Real-world situations can help illustrate regression to the mean. For example, during an early round of the 2019 Women’s World Cup, the team from Italy outscored the team from Jamaica 5–0. That’s a big score; soccer (football) teams hardly ever score 5 points in a game. Without being familiar with either team, people who know about soccer would predict that in their next game, Italy would score fewer than 5 goals. Why? Simply because most people have an intuitive understanding of regression to the mean. Here’s the statistical explanation. The Italian team’s score was exceptionally high partly because of the team’s talent, and partly because of a unique combination of random factors that happened to come out in their favor. It was an early-round game, and the players felt confident because they were higher seeded. The team’s injury level was, just by chance, much lower than usual. The European setting may have favored Italy. Therefore, despite Italy’s legitimate talent as a team, they also benefited from randomness—a chance combination of lucky events that would probably never happen in the same combination again, like flipping a coin and getting eight heads in a row. Overall, the team’s score in the subsequent game would almost necessarily be worse than in this game— not all eight flips will turn out in their favor again. Indeed, the team did regress: In their next game, they lost to Brazil, 0–1. In other words, Italy finished closer to an average level of performance. Here’s another example. Suppose you’re normally cheerful and happy, but on any given day your usual upbeat mood can be affected by random factors, such as the weather, your friends’ moods, and even parking problems. Every once in a while, just by chance, several of these random factors will affect you negatively: It will pour rain, your friends will be grumpy, and you won’t be able to find a parking space. Your day is terrible! The good news is that tomorrow will almost certainly be better because all three of those random factors are unlikely to occur in that same, unlucky combination again. It might still be raining, but your friends won’t be grumpy, and you’ll quickly find a good parking space. If even one of these factors is different, your day will go better and you will regress toward your average, happy mean. Regression works at both extremes. An unusually good performance or outcome is likely to regress downward (toward its mean) the next time. And an unusually bad performance or outcome is likely to regress upward (toward its mean) the next time. Either extreme is explainable by an unusually lucky, or an unusually unlucky, combination of random events. Regression and Internal Validity. Regression threats occur only when a group is measured twice, and only when the group has an extreme score at pretest. If the group has been selected because of its unusually high or low group mean at pretest, you can expect them to regress toward the mean somewhat when it comes time for the posttest. You might suspect that the 40 depressed women Dr. Yuki studied were, as a group, quite depressed. Their group average at pretest was partly due to their true, baseline level of depression, but it’s also true that people seek treatment when they are especially low. In this group, a proportion are feeling especially depressed partly because of random events (e.g., the winter blues, a recent illness, family or relationship problems, job loss, divorce). At the posttest, the same unlucky combination of random effects on the group mean probably would not be the same as they were at pretest (maybe some saw their relationships get better, or the job situation improved for a few), so the posttest depression average would go down. The change would not occur because of the treatment, but simply because of regression to the mean, so in this case there would be an internal validity threat. Preventing Regression Threats. Once again, comparison groups can help researchers prevent regression threats, along with a careful inspection of the pattern of results. If the comparison group and the experimental group are equally extreme at pretest, the researchers can account for any regression effects in their results. In Figure 11.5A, you can rule out regression and conclude that the therapy really does work: If regression played a role, it would have done so for both groups because they were equally at risk for regression at the start. In contrast, if you saw the pattern of results shown in Figure 11.5B, you would suspect that regression had occurred. Regression is a particular threat in exactly this situation—when one group has been selected for its extreme mean. In Figure 11.5C, in contrast, the therapy group started out more extreme on depression, and therefore probably regressed to the mean. However, regression alone can’t make a group cross over the comparison group, so the pattern shows an effect of therapy, in addition to a little help from regression effects. More Information Figure 11.5 Regression threats to internal validity. Regression to the mean can be analyzed by inspecting different patterns of results. ATTRITION THREATS TO INTERNAL VALIDITY Why did the average level of rambunctious behavior in Nikhil’s campers decrease over the course of the week? It could have been because of the low-sugar diet, but maybe it was because the most unruly camper had to leave camp early. Similarly, the level of depression among Dr. Yuki’s patients might have decreased because of the cognitive therapy, but it might have been because three of the most depressed women in the study could not maintain the treatment regimen and dropped out of the study. The posttest average is lower only because these extra-high scores are not included. In studies that have a pretest and a posttest, attrition (sometimes referred to as mortality) is a reduction in participant numbers that occurs when people drop out before the end. Attrition can happen when a pretest and posttest are administered on separate days and some participants are not available on the second day. An attrition threat becomes a problem for internal validity when attrition is systematic; that is, when only a certain kind of participant drops out. If any random camper leaves midweek, it might not be a problem for Nikhil’s research, but it is a problem when the most rambunctious camper leaves early. His departure creates an alternative explanation for Nikhil’s results: Was the posttest average lower because the low-sugar diet worked, or because one extreme score is gone? Similarly, as shown in Figure 11.6, it would not be unusual if two of 40 women in the depression therapy study dropped out over time. However, if the two most depressed women systematically drop out, the mean for the posttest is going to be lower only because it does not include these two extreme scores (not because of the therapy). Therefore, if the depression score goes down from pretest to posttest, you wouldn’t know whether the decrease occurred because of the therapy or because of the alternative explanation—that the highest-scoring women had dropped out. More Information Figure 11.6 Attrition threats. (A) If two people (noted by blue dots) drop out of a study, both of whom scored at the high end of the distribution on the pretest, the group mean changes substantially when their scores are omitted, even if all other scores stay the same. (B) If the dropouts’ scores on the pretest are close to the group mean, removing their scores does not change the group mean as much. Preventing Attrition Threats. An attrition threat is fairly easy for researchers to identify and correct. When participants drop out of a study, most researchers will remove those participants’ scores from the pretest average too. That way, they look only at the scores of those who completed both parts of the study. Another approach is to check the pretest scores of the dropouts. If they have extreme scores on the pretest, their attrition is more of a threat to internal validity than if their scores are closer to the group average. TESTING THREATS TO INTERNAL VALIDITY A testing threat, a specific kind of order effect, refers to a change in the participants as a result of taking a test (dependent measure) more than once. People might have become more practiced at taking the test, leading to improved scores, or they may become fatigued or bored, which could lead to worse scores over time. Therefore, testing threats include practice effects (see Chapter 10). In an educational setting, for example, students might perform better on a posttest than on a pretest, but not because of any educational intervention. Instead, perhaps they were inexperienced the first time they took the test, and they did better on the posttest simply because they had more practice the second time around. Preventing Testing Threats. To avoid testing threats, researchers might abandon a pretest altogether and use a posttest-only design (see Chapter 10). If they do use a pretest, they might opt to use alternative forms of the test for the two measurements. The two forms might both measure depression, for example, but use different items to do so. A comparison group will also help. If the comparison group takes the same pretest and posttest but the treatment group shows an even larger change, testing threats can be ruled out (Figure 11.7). More Information Figure 11.7 Testing threats. (A) If there is no comparison group, it’s hard to know whether the improvement from pretest to posttest is caused by the treatment or simply by practice. (B) The results from a comparison group can help rule out testing threats. Both groups might improve, but the treatment group improves even more, suggesting that both practice and a true effect of the treatment are causing the improvement. INSTRUMENTATION THREATS TO INTERNAL VALIDITY An instrumentation threat occurs when a measuring instrument changes over time. In observational research, the people who are coding behaviors are the measuring instrument, and over a period of time, they might change their standards for judging behavior by becoming stricter or more lenient. Thus, maybe Nikhil’s campers did not really become less disruptive; instead, the people judging the campers’ behavior became more tolerant of loud voices and rough-and-tumble play. Another case of an instrumentation threat would be when a researcher uses different forms for the pretest and posttest, but the two forms are not sufficiently equivalent. Dr. Yuki might have used a measure of depression at pretest on which people tend to score a little higher, and another measure of depression at posttest that tends to yield lower scores. As a result, the pattern she observed was not a sign of how good the cognitive therapy is, but merely reflected the way the alternative forms of the test are calibrated. Preventing Instrumentation Threats. To prevent instrumentation threats, researchers can switch to a posttest-only design, or they can take steps to ensure that the pretest and posttest measures are equivalent. To do so, they might collect data from each instrument to be sure the two are calibrated the same. To avoid shifting standards of behavioral coders, researchers might retrain their coders throughout the experiment, establishing their reliability and validity at both pretest and posttest. Using clear coding manuals would be an important part of this process. Another simple way to prevent an instrumentation threat is to use a posttest-only design (in which behavior is measured only once). Finally, to control for the problem of different forms, Dr. Yuki could also counterbalance the versions of the test, giving some participants version A at pretest and version B at posttest, and giving other participants version B, and then version A. Instrumentation Versus Testing Threats. These two threats are pretty similar, but here’s the difference: An instrumentation threat means the measuring instrument has changed from Time 1 to Time 2, whereas a testing threat means the participants change over time from having been tested before. COMBINED THREATS You have learned throughout this discussion that true pretest/posttest designs (those with two or more groups) normally take care of many internal validity threats. However, in some cases, a study with a pretest/posttest design might combine selection threats with history or attrition threats. In a selection-history threat, an outside event or factor affects only those at one level of the independent variable. For example, perhaps the dorm that was used as a comparison group was undergoing construction, and the construction crew used electric tools that drew on only that dorm’s power supply. Therefore, the researcher won’t be sure: Was it because the Go Green campaign reduced student energy usage? Or was it only because the comparison group dorm used so many power tools? Similarly, in a selection-attrition threat, only one of the experimental groups experiences attrition. If Dr. Yuki conducted her depression therapy experiment as a pretest/posttest design, it might be the case that the most severely depressed people dropped out—but only from the treatment group, not the control group. The treatment might have been especially arduous for the most depressed people, so they dropped out of the study. Because the control group was not undergoing treatment, they are not susceptible to the same level of attrition. Therefore, selection and attrition can combine to make Dr. Yuki unsure: Did the cognitive therapy really work, compared with the control group? Or is it just that the most severely depressed people dropped out of the treatment group? Three Potential Internal Validity Threats in Any Study Many internal validity threats are likely to occur in the one-group, pretest/posttest design, and these threats can often be examined simply by adding a comparison group. Doing so would result in a pretest/posttest design. The posttest-only design is another option (see Chapter 10). However, three more threats to internal validity—observer bias, demand characteristics, and placebo effects—might apply even for designs with a clear comparison group. OBSERVER BIAS Observer bias can be a threat to internal validity in almost any study in which there is a behavioral dependent variable. Observer bias occurs when researchers’ expectations influence their interpretation of the results. For example, Dr. Yuki might be a biased observer of her patients’ depression: She expects to see her patients improve, whether they do or do not. Nikhil may be a biased observer of his campers: He may expect the low-sugar diet to work, so he views the boys’ posttest behavior more positively. Although comparison groups can prevent many threats to internal validity, they do not necessarily control for observer bias. Even if Dr. Yuki used a no-therapy comparison group, observer bias could still occur: If she knew which participants were in which group, her biases could lead her to see more improvement in the therapy group than in the comparison group. ❯❯ For more on observer bias, see Chapter 6, p. 170. Observer bias can threaten two kinds of validity in an experiment. It threatens internal validity because an alternative explanation exists for the results. Did the therapy work, or was Dr. Yuki biased? It can also threaten the construct validity of the dependent variable because it means the depression ratings given by Dr. Yuki do not represent the true levels of depression of her participants. DEMAND CHARACTERISTICS ❯❯ For more on demand characteristics, see Chapter 10, p. 302. Demand characteristics are a problem when participants guess what the study is supposed to be about and change their behavior in the expected direction. For example, Dr. Yuki’s patients know they are getting therapy. If they think Dr. Yuki expects them to get better, they might change their self-reports of symptoms in the expected direction. Nikhil’s campers, too, might realize something fishy is going on when they’re not given their usual snacks. Their awareness of a menu change could certainly change the way they behave. Controlling for Observer Bias and Demand Characteristics. To avoid observer bias and demand characteristics, researchers must do more than add a comparison group to their studies. The most appropriate way to avoid such problems is to conduct a double-blind study, in which neither the participants nor the researchers who evaluate them know who is in the treatment group and who is in the comparison group. Suppose Nikhil decides to test his hypothesis as a double-blind study. He could arrange to have two cabins of equally lively campers and replace the sugary snacks with good-tasting low-sugar versions for only one group. The boys would not know which kind of snacks they were eating, and the people observing their behavior would also be blind to which boys were in which group. When a double-blind study is not possible, a variation might be an acceptable alternative. In some studies, participants know which group they are in, but the observers do not; this is called a masked design, or blind design (see Chapter 6). The students exposed to the Go Green campaign would certainly be aware that someone was trying to influence their behavior. Ideally, however, the raters who were recording their electrical energy usage should not know which dorm was exposed to the campaign and which was not. Of course, keeping observers unaware is even more important when they are rating behaviors that are more difficult to code, such as symptoms of depression or behavior problems at camp. Recall the study by Mueller and Oppenheimer (2014) from Chapter 10, in which people took notes in longhand or on laptops. The research assistants in that study were blind to the condition each participant was in when they graded their tests on the lectures. The participants themselves were not blind to their notetaking method. However, since the test-takers participated in only one condition (an independent-groups design), they were not aware that the form of notetaking was an important feature of the experiment. Therefore, they were blind to the reason they were taking notes in longhand or on a laptop. PLACEBO EFFECTS The women who received Dr. Yuki’s cognitive therapy may have improved because her therapeutic approach really works. An alternative explanation is that there was a placebo effect. A placebo effect occurs when people receive a treatment and really improve—but only because the recipients believe they are receiving a valid treatment. In most studies on the effectiveness of medications, for example, one group receives a pill or an injection with the real drug, while another group receives a pill or an injection with no active ingredients—a sugar pill or a saline solution. People can even receive placebo psychotherapy, in which they simply talk to a friendly listener about their problems, but these placebo conversations have no therapeutic structure. The inert pill, injection, or therapy is the placebo. Often people who receive the placebo see their symptoms improve because they believe the treatment they are receiving is supposed to be effective. In fact, the placebo effect can occur whenever any kind of treatment is used to control symptoms, such as an herbal remedy to enhance wellness (Figure 11.8). More Information Figure 11.8 Are herbal remedies placebos? It is possible that perceived improvements in mood, joint pain, or wellness promised by herbal supplements are simply due to the belief that they will work, not because of the specific ingredients they contain. Placebo effects are not imaginary. Placebos have been shown to reduce real symptoms and side effects, both psychological and physical, including depression (Kirsch & Sapirstein, 1998), postoperative pain or anxiety (Benedetti et al., 2006), terminal cancer pain, and epilepsy (Beecher, 1955). They are not always beneficial or harmless; physical side effects, including skin rashes and headaches, can be caused by placebos, too. People’s symptoms appear to respond not just to the active ingredients in medications or to psychotherapy, but also to their belief in what the treatment can do to alter their situation. A placebo can be strong medicine. Kirsch and Sapirstein (1998) reviewed studies that gave either antidepressant medication, such as Prozac, or a placebo to depressed patients, and concluded that the placebo groups improved almost as much as groups that received real medicine. In fact, up to 75% of the depression improvement in the Prozac groups was also achieved in placebo groups. Designing Studies to Rule Out the Placebo Effect. To determine whether an effect is caused by a therapeutic treatment or by placebo effects, the standard approach is to include a special kind of comparison group. As usual, one group receives the real drug or real therapy, and the second group receives the placebo drug or placebo therapy. Crucially, however, neither the people treating the patients nor the patients themselves know whether they are in the real group or the placebo group. This experimental design is called a double-blind placebo control study. The results of such a study might look like the graph in Figure 11.9. Notice that both groups improved, but the group receiving the real drug improved even more, showing placebo effects plus the effects of the real drug. If the results turn out like this, the researchers can conclude that the treatment they are testing does cause improvement above and beyond a placebo effect. Once again, an internal validity threat—a placebo effect—can be avoided with a careful research design. More Information Figure 11.9 A double-blind placebo control study. Adding a placebo comparison group can help researchers separate a potential placebo effect from the true effect of a particular therapy. Is That Really a Placebo Effect? If you thought about it carefully, you probably noticed that the results in Figure 11.9 do not definitively show a placebo effect pattern. Both the group receiving the real drug and the group receiving the placebo improved over time. However, some of the improvement in both groups could have been caused by maturation, history, regression, testing, or instrumentation threats (Kienle & Kiene, 1997). If you were interested in showing a placebo effect specifically, you would have to include a no-treatment comparison group—one that receives neither drug nor placebo. Suppose your results looked something like those in Figure 11.10. Because the placebo group improved over time, even more than the no-therapy/no- placebo group, you can attribute the improvement to placebo and not just to maturation, history, regression, testing, or instrumentation. More Information Figure 11.10 Identifying a placebo effect. Definitively showing a placebo effect requires three groups: one receiving the true therapy, one receiving the placebo, and one receiving no therapy. If there is a placebo effect, the pattern of results will show that the no-therapy group does not improve as much as the placebo group. With So Many Threats, Are Experiments Still Useful? After reading about a dozen ways a good experiment can go wrong, you might be tempted to assume that most experiments you read about are faulty. However, responsible researchers consciously avoid internal validity threats when they design and interpret their work. Many of the threats discussed in this chapter are a problem only in one-group, pretest/posttest studies—those with no comparison group. As shown in the Working It Through section (p. 340), a carefully designed comparison group will correct for many of these threats. The section analyzes the study on mindfulness (Mrazek et al., 2013) discussed in Chapter 10 and presented again here in Figure 11.11. Table 11.1 summarizes the internal validity threats in Chapters 10 and 11 and suggests ways to find out whether a particular study is vulnerable. STRAIGHT FROM THE SOURCE More Information Figure 11.11 Mindfulness study results. This study showed that mindfulness classes, but not nutrition classes, were associated with an increase in GRE scores. Can the study rule out all twelve internal validity threats and support a causal claim? (Source: Mrazek et al., 2013, Fig. 1A.) TABLE 11.1 Asking About Internal Validity Threats in Experiments Questions to Name Definition Example Ask From Chapter Did the 10: If people researchers A second variable who take turn potential that notes on third variables unintentionally laptops into control Design varies answer harder variables—for confound systematically questions example, with the than those keeping independent who take question variable. notes difficulty longhand. constant? From Chapter 10: In the In an independent- autism study, groups design, some parents Did the when the two insisted they researchers use independent wanted their random variable groups Selection effect children to be assignment or have in the matched groups systematically intensive- to equalize different kinds of treatment groups? participants in group rather them. than the control group. From Chapter In a repeated- 10: People measures design, rated the Did the when the effect of shared researchers the independent chocolate counterbalance variable is higher only the orders of Order effect confounded with because the presentation of carryover from first taste of the levels of one level to the chocolate is the independent other, or with always more variable? practice, fatigue, delicious than or boredom. the second one. Did the researchers use An experimental Disruptive a comparison group improves boys settle group of boys over time only down as they who had an Maturation because of natural get used to equal amount development or the camp of time to spontaneous setting. mature but who improvement. did not receive the treatment? Did the Dorm researchers An experimental residents use include a group changes less air comparison over time because conditioning group that had of an external in November History an equal factor that affects than exposure to the all or most September external factor members of the because the but did not group. weather is receive the cooler. treatment? A group’s An experimental average is group whose extremely average is Did the depressed at extremely low (or researchers pretest, in high) at pretest include a part because will get better (or comparison some Regression to worse) over time group that was members the mean because the equally volunteered random events extreme at for therapy that caused the pretest but did when they extreme pretest not receive the were feeling scores do not therapy? much more recur the same depressed way at posttest. than usual. Because the Did the An experimental most researchers group changes rambunctious compute the over time, but boy in the pretest and only because the cabin leaves posttest scores most extreme camp early, with only the Attrition cases have his unruly final sample systematically behavior included, dropped out and affects the removing any their scores are pretest mean dropouts’ data not included in the but not the from the posttest. posttest pretest group mean. average? Did the GRE verbal researchers A type of order scores have a effect: An improve only comparison experimental because group take the group changes students take same two tests? over time because the same Did they use a Testing repeated testing version of the posttest-only has affected the test both design, or did participants. times and they use Practice effects therefore are alternative (fatigue effects) more forms of the are one subtype. practiced at measure for the posttest. pretest and posttest? Did the Coders get researchers An experimental more lenient train coders to group changes over time, so use the same over time, but the same standards when Instrumentation only because the behavior is coding? Are measurement coded as less pretest and instrument has disruptive at posttest changed. posttest than measures at pretest. demonstrably equivalent? The researcher expects a An experimental Were the low-sugar group’s ratings observers of diet to differ from a the dependent decrease the comparison variable campers’ Observer bias group’s, but only unaware of unruly because the which behavior, so researcher expects condition he notices the groups’ participants only calm ratings to differ. were in? behavior and ignores wild behavior. Were the participants Campers kept unaware guess that the of the purpose Participants guess low-sugar of the study? what the study’s diet is Was it an purpose is and Demand supposed to independent- change their characteristic make them groups design, behavior in the calmer, so which makes expected they change participants direction. their behavior less able to accordingly. guess the study’s purpose? Women receiving Participants in an cognitive Did a experimental therapy comparison group improve improve group receive a only because they Placebo effect simply placebo (inert) believe in the because they drug or a efficacy of the believe the placebo therapy or drug therapy will therapy? they receive. work for them. CHECK YOUR UNDERSTANDING 1. How does a one-group, pretest/posttest design differ from a pretest/posttest design, and which threats to internal validity are especially applicable to this design? 2. Using Table 11.1 as a guide, indicate which of the internal validity threats would be relevant even to a (two-group) posttest-only design. 1. See p. 325. 2. See pp. 338–339. Glossary one-group, pretest/posttest design An experiment in which a researcher recruits one group of participants; measures them on a pretest; exposes them to a treatment, intervention, or change; and then measures them on a posttest. maturation threat A threat to internal validity that occurs when an observed change in an experimental group could have emerged more or less spontaneously over time. history threat A threat to internal validity that occurs when it is unclear whether a change in the treatment group is caused by the treatment itself or by an external or historical factor that affects most members of the group. regression threat A threat to internal validity related to regression to the mean, a phenomenon in which any extreme finding is likely to be closer to its own typical, or mean, level the next time it is measured (with or without the experimental treatment or intervention). See also regression to the mean. regression to the mean A phenomenon in which an extreme finding is likely to be closer to its own typical, or mean, level the next time it is measured, because the same combination of chance factors that made the finding extreme are not present the second time. See also regression threat. attrition threat In a pretest/posttest, repeated-measures, or quasi-experimental study, a threat to internal validity that occurs when a systematic type of participant drops out of the study before it ends. testing threat In a repeated-measures experiment or quasi-experiment, a kind of order effect in which scores change over time just because participants have taken the test more than once; includes practice effects. instrumentation threat A threat to internal validity that occurs when a measuring instrument changes over time. selection-history threat A threat to internal validity in which a historical or seasonal event systematically affects only the participants in the treatment group or only those in the comparison group, not both. selection-attrition threat A threat to internal validity in which participants are likely to drop out of either the treatment group or the comparison group, not both. observer bias A bias that occurs when observer expectations influence the interpretation of participant behaviors or the outcome of the study. demand characteristic A cue that leads participants to guess a study’s hypotheses or goals; a threat to internal validity. Also called experimental demand. double-blind study A study in which neither the participants nor the researchers who evaluate them know who is in the treatment group and who is in the comparison group. masked design A study design in which the observers are unaware of the experimental conditions to which participants have been assigned. Also called blind design. placebo effect A response or effect that occurs when people receiving an experimental treatment experience a change only because they believe they are receiving a valid treatment. double-blind placebo control study A study that uses a treatment group and a placebo group and in which neither the researchers nor the participants know who is in which group. WORKING IT THROUGH Did Mindfulness Training Really Cause GRE Scores to Improve? Three male students work at desks arranged in a line, one in front of the other. Each student holds a pencil and writes on paper. In Chapter 10, you read about a pretest/posttest design in which students were randomly assigned to a mindfulness training course or to a nutrition course (Mrazek et al., 2013). Students took GRE verbal tests both before and after their assigned training course. Those assigned to the mindfulness course scored significantly higher on the GRE posttest than pretest. The authors would like to claim that the mindfulness course caused the improvement in GRE scores. Does this study rule out internal validity threats? QUESTIONS CLAIMS, QUOTES, OR INTERPRETATION TO ASK DATA AND EVALUATION QUESTIONS CLAIMS, QUOTES, OR INTERPRETATION TO ASK DATA AND EVALUATION The paper reports that classes met for 45 minutes These passages four times a week for 2 indicate that the weeks and were taught by classes were equal in professionals with Is the study their time extensive teaching susceptible to commitment, the experience in their any of these quality of the respective fields. “Both internal instructors used, and classes were taught by validity other factors, so these expert instructors, were threats? are not design composed of similar confounds. It appears numbers of students, were Design the two classes did not held in comparable confound accidentally vary on classrooms during the late anything besides their afternoon, and used a mindfulness versus similar class format, nutrition content. including both lectures and group discussions” (p. 778). The article reports that Random assignment “students... were controls for selection Selection effect randomly assigned to either effects, so selection is a mindfulness class... or a not a threat in the nutrition class” (p. 777). study. QUESTIONS CLAIMS, QUOTES, OR INTERPRETATION TO ASK DATA AND EVALUATION Order effects are relevant only for repeated-measures Order effect designs, not independent-groups designs like this one. While it’s possible that people could simply get better at the GRE over time, maturation Maturation would have happened threat to the nutrition group as well (but it did not). We can rule out maturation. QUESTIONS CLAIMS, QUOTES, OR INTERPRETATION TO ASK DATA AND EVALUATION Could some outside event, such as a free GRE prep course on campus, have improved people’s GRE scores? We can rule out such a history History threat threat because of the comparison group: It’s unlikely a campus GRE program would just happen to be offered only to students in the mindfulness group. QUESTIONS CLAIMS, QUOTES, OR INTERPRETATION TO ASK DATA AND EVALUATION A regression threat is unlikely here. First, the students were randomly assigned to the mindfulness group, not selected on the basis of extremely low GRE scores. Second, Regression the mindfulness group threat and the nutrition group had the same pretest means. They were equally extreme, so if regression had affected one group, it would also have affected the other. There’s no indication in the Because all paper that any participants participants apparently Attrition threat dropped out between pretest completed the study, and posttest. attrition is not a threat. QUESTIONS CLAIMS, QUOTES, OR INTERPRETATION TO ASK DATA AND EVALUATION Participants did take the verbal GRE two times, but if their improvement was Testing threat simply due to practice, we would see a similar increase in the nutrition group, and we do not. The study reports, “We used The described two versions of the verbal procedure controls for Instrumentation GRE measure that were any difference in test threat matched for difficulty and difficulty from pretest counterbalanced within to posttest. each condition” (p. 777). QUESTIONS CLAIMS, QUOTES, OR INTERPRETATION TO ASK DATA AND EVALUATION Experimenter expectancy is another “We minimized name for observer experimenter expectancy bias. These procedures effects by testing seem to be reasonable participants in mixed- ways to prevent an Observer bias condition groups in which experimenter from nearly all task instructions leading participants in were provided by one group to be more computers” (p. 778). motivated to do well on the dependent measure. “All participants were This statement argues recruited under the pretense that all students that the study was a direct expected their Demand comparison of two equally assigned program to be characteristics viable programs for effective. If true, then or placebo improving cognitive placebo effects and effects performance, which demand characteristics minimized motivation and were equal in both placebo effects” (p. 778). conditions. QUESTIONS CLAIMS, QUOTES, OR INTERPRETATION TO ASK DATA AND EVALUATION This study’s design and results have controlled for virtually all the internal validity threats in Table 11.1, so we can conclude its internal validity is strong and the study supports the claim that mindfulness training improved students’ GRE verbal scores. (Next you could interrogate this study’s construct, statistical, and external validity!) INTERROGATING NULL EFFECTS: WHAT IF THE INDEPENDENT VARIABLE DOES NOT MAKE A DIFFERENCE? So far, this chapter has discussed cases in which a researcher works to ensure that any covariance found in an experiment was caused by the independent variable, not by a threat to internal validity. What if the independent variable did not make much difference in the dependent variable? What if the 95% CI for the effect includes zero, such as a 95% CI of [–.21,.18]? Such an outcome may be called a null result, or null effect. Research that finds null effects are surprisingly common—something many students learn when they start to conduct their own studies. If researchers expected to find a large difference but obtained a 95% CI that contained zero instead, they sometimes say their study “didn’t work.” What might null effects mean? Here are three hypothetical examples: Many people believe having more money will make them happy. But will it? A group of researchers designed an experiment in which they randomly assigned people to three groups. They gave one group nothing, gave the second group a little money, and gave the third group a lot of money. The next day, they asked each group to report their happiness on a mood scale. The groups who received cash (either a little or a lot) were not significantly happier, or in a better mood, than the group who received nothing. The 95% CIs for the groups overlapped completely. Do online reading games make kids better readers? An educational psychologist recruited a sample of 5-year-olds, all of whom did not yet know how to read. She randomly assigned the children to two groups. One group played with a commercially available online reading game for 1 week (about 30 minutes per day), and the other group continued “treatment as usual,” attending their normal kindergarten classes. Afterward, the children were tested on their reading ability. The reading game group’s scores were a little higher than those of the kindergarten-as-usual group, but the 95% CI for the estimated difference between two groups included zero. Researchers have hypothesized that feeling anxious can cause people to reason less carefully and logically. To test this hypothesis, a research team randomly assigned people to three groups: low, medium, and high anxiety. After a few minutes of being exposed to the anxiety manipulation, the participants solved problems requiring logic, rather than emotional reasoning. Although the researchers had predicted the anxious people would do worse on the problems, participants in the three groups scored roughly the same. These three examples of null effects, shown as graphs in Figure 11.12, are all posttest-only designs. However, a null effect can happen in a within- groups design or a pretest/posttest design, too (and even in a correlational study). In all three of these cases, the independent variable manipulated by the experimenters did not result in a change in the dependent variable. Why didn’t these experiments show covariance between the independent and dependent variables? More Information Figure 11.12 Results of three hypothetical experiments showing a null effect. (A) Why might cash not have made people significantly happy? (B) Why might the online reading games not have worked? (C) Why might anxiety not have affected logical reasoning? Error bars represent fabricated 95% Cis. Any time an experiment gives a null result, it might be the case that the independent variable has virtually no effect on the dependent variable. In the real world, perhaps money does not make people any happier, online reading games improve kids’ reading skill only a tiny amount, and being anxious has virtually no effect on logical reasoning. In other words, the experiment gave an accurate estimate, showing that the manipulation the researchers used caused hardly any change in the dependent variable. Therefore, when we obtain a null result, it can tell us something valid and valuable: The independent variable does not cause much of a difference. ❯❯ For more details on confidence intervals, see Statistics Review: Inferential Statistics, pp. 493–495, and Figure S2.9, p. 505. A different possibility is that there is a true effect, but this particular study did not detect it. Research takes place over the long run, and scientists conduct multiple replication studies testing the same theory. Because of chance variations from study to study, we should expect slight variations in the 95% CIs. Even if there’s a true effect, some studies will produce a 95% CI that includes zero just by chance. Researchers can increase their ability to detect a true effect by planning the best study possible. That is, sometimes an obscuring factor in the study prevents the researchers from detecting the true difference. Such obscuring factors can take two general forms: There might not have been enough between-groups difference, or there might have been too much within- groups variability. To illustrate these two obscuring factors, suppose you prepared two bowls of salsa: one containing two shakes of hot sauce and the other containing four shakes of hot sauce. People might not taste any difference between the two bowls (a null effect!). One reason is that four shakes is not that different from two: There’s not enough between-groups difference. A second reason is that each bowl contains many other ingredients (tomatoes, onions, jalapeños, cilantro, lime juice), so it’s hard to detect any change in hot sauce intensity with all those other flavors getting in the way. That’s a problem of too much within-groups variability. Now let’s see how this analogy plays out in psychological research. Perhaps There Is Not Enough Between- Groups Difference When a study returns a null result, sometimes the culprit is not enough between-groups difference. Weak manipulations, insensitive measures, ceiling and floor effects, and reverse design confounds might prevent study results from revealing a true difference that exists between two or more experimental groups. WEAK MANIPULATIONS Why did the study show that money had little effect on people’s moods? You might ask how much money the researchers gave each group. What if the amounts were $0.00, $0.25, and $1.00? In that case, it might be no surprise that the manipulation didn’t have a strong effect. A dollar doesn’t seem like enough money to affect most people’s mood. Like the difference between two shakes and four shakes of hot sauce, it’s not enough of an increase to matter. Similarly, perhaps a 1-week exposure to reading games is not sufficient to cause any change in reading scores. Both of these would be examples of weak manipulations, which can obscure a true causal relationship. When you interrogate a null result, then, it’s important to ask how the researchers operationalized the independent variable. In other words, you have to ask about construct validity. The money and mood researchers might have obtained a very different pattern of results if they had given $0.00, $5.00, and $150.00 to the three groups. The educational psychologist might have found that reading games improve scores if played daily for 3 months rather than just a week. INSENSITIVE MEASURES ❮❮ For more on scales of measurement, see Chapter 5, pp. 122–124. Sometimes a study finds a null result because the researchers have not used an operationalization of the dependent variable with enough sensitivity. If a medication reduces fever by a tenth of a degree, you wouldn’t be able to detect it with a thermometer that was calibrated in one- degree increments—it wouldn’t be sensitive enough. Similarly, if online reading games improve reading scores by about 2 points, you wouldn’t be able to detect the improvement with a simple pass/fail reading test (either passing or failing, nothing in between). When it comes to dependent measures, it’s smart to use ones that have detailed, quantitative increments —not just two or three levels. CEILING AND FLOOR EFFECTS In a ceiling effect, all the scores are squeezed together at the high end. In a floor effect, all the scores cluster at the low end. As special cases of weak manipulations and insensitive measures, ceiling and floor effects can cause independent variable groups to score almost the same on the dependent variable. Ceilings, Floors, and Independent Variables. Ceiling and floor effects can be the result of a problematic independent variable. For example, if the researchers really did manipulate the independent variable by giving people $0.00, $0.25, or $1.00, that would be a floor effect because these three amounts are all low—they’re squeezed close to a floor of $0.00. Consider the example of the anxiety and reasoning study. Suppose the researcher manipulated anxiety by telling the groups they were about to receive an electric shock. The low-anxiety group was told to expect a 10- volt shock, the medium-anxiety group a 50-volt shock, and the high- anxiety group a 100-volt shock. This manipulation would probably result in a ceiling effect because expecting any amount of shock would cause anxiety, regardless of the shock’s intensity. As a result, the various levels of the independent variable would appear to make no difference. ❮❮ Ceiling and floor effects are examples of restriction of range; see Chapter 8, pp. 216–218. Ceilings, Floors, and Dependent Variables. Poorly designed dependent variables can also lead to ceiling and floor effects. Imagine if the logical reasoning test in the anxiety study was so difficult that nobody could solve the problems. That would cause a floor effect: The three anxiety groups would score the same, but only because the measure for the dependent variable results in low scores in all groups. Or suppose the reading test used in the online game study asked the children to point to the first letter of their own name. Almost all 5-year- olds can do this, so the measure would result in a ceiling effect. All children would get a perfect score, and there would be no room for between-groups variability on this measure. Similarly, if the reading test asked children to analyze a passage of Tolstoy, almost all children would fail, creating a floor effect (Figure 11.13). More Information Figure 11.13 Ceiling and floor effects. A ceiling or floor effect on the dependent variable can obscure a true difference between groups. If all the questions on a test are too easy, everyone will get a perfect score. If the questions are too hard, everyone will score low. MANIPULATION CHECKS HELP DETECT WEAK MANIPULATIONS, CEILINGS, AND FLOORS When you interrogate a study with a null effect, it is important to ask how the independent and dependent variables were operationalized. Was the independent variable manipulation strong enough to cause a difference between groups? And was the dependent variable measure sensitive enough to detect that difference? Recall from Chapter 10 that a manipulation check is a separate dependent variable that experimenters include in a study, specifically to make sure the manipulation worked. For example, in the anxiety study, after telling people they were going to receive a 10-volt, 50-volt, or 100-volt shock, the researchers might have asked: How anxious are you right now, on a scale of 1 to 10? If the manipulation check showed that participants in all three groups felt nearly the same level of anxiety (Figure 11.14A), you’d know the researchers did not effectively manipulate what they intended to manipulate. If the manipulation check showed that the independent variable levels differed in an expected way—participants in the high- anxiety group really felt more anxious than those in the other two groups (Figure 11.14B)—then you’d know the researchers did effectively manipulate anxiety. If the manipulation check worked, the researchers could look for another reason for the null effect of anxiety on logical reasoning. Perhaps the dependent measure has a floor effect; that is, the logical reasoning test might be too difficult, so everyone scores low (see Figure 11.13). Or perhaps the effect of anxiety on logical reasoning is truly negligible. More Information Figure 11.14 Possible results of a manipulation check. (A) These results suggest the anxiety manipulation did not work because people at all three levels of the independent variable reported being equally anxious. (B) These results suggest the manipulation did work because the anxiety of people in the three independent variable groups did vary in the expected way. The error bars depict fabricated 95% Cis. DESIGN CONFOUNDS ACTING IN REVERSE Confounds are usually considered to be internal validity threats— alternative explanations for some observed difference in a study. However, they can apply to null effects, too. A study might be designed in such a way that a design confound actually counteracts, or reverses, some true effect of an independent variable. In the money and happiness study, for example, perhaps the students who received the most money happened to be given the money by a grouchy experimenter, while those who received the least money were exposed to a more cheerful person. This confound would have worked against any true effect of money on mood. Perhaps Within-Groups Variability Obscured the Group Differences Another reason a study might return a null effect is that there is too much unsystematic variability within each group. This is referred to as noise (also known as error variance or unsystematic variance). In our salsa analogy, noise refers to the great number of the other flavors in the two bowls. Noisy within-groups variability can get in the way of detecting a true difference between groups. Consider the sets of scores in Figure 11.15. The bar graphs and scatterplots depict the same data, but in two graphing formats. In each case, the mean difference between the two groups is the same. However, the variability within each groups is much larger in part A than part B. You can see that when there is more variability within groups, it obscures the differences between the groups because more overlap exists between the members of the two groups. It’s a statistical validity concern: The greater the overlap, the less precisely the two group means are estimated and the smaller the standardized effect size. More Information Figure 11.15 Within-groups variability can obscure group differences. Notice that the group averages are the same in both versions, but the variability within each group is greater in part A than part B. Part B is the situation researchers prefer because it enables them to better detect true differences in the independent variable (error bars represent fabricated standard errors). ❮❮ For more on statistical significance, see Chapter 8, pp. 213–214; and Statistics Review: Inferential Statistics. When the data show less variability within the groups (see Figure 11.15B), the 95% CI will be narrower and the standardized effect size will be larger. With less within-groups variability, our estimate of the group difference is more precise. If the two bowls of salsa contained nothing but tomatoes, the difference between two and four shakes of hot sauce would be more easily detectable because there would be fewer competing, “noisy” flavors within bowls. In sum, the more unsystematic variability there is within each group, the more the scores in the two groups overlap with each other. The greater the overlap, the less apparent the average difference. As described next, most researchers try to keep within-groups variability to a minimum by attending to measurement error, irrelevant individual differences, and situation noise. MEASUREMENT ERROR One reason for high within-groups variability is measurement error, a human or instrument factor that can randomly inflate or deflate a person’s true score on the dependent variable. For example, a person who is 160 centimeters tall might be measured at 160.25 cm because of the angle of vision of the person using the meter stick, or they might be recorded as 159.75 cm because they slouched a bit. All dependent variables involve a certain amount of measurement error, but researchers try to keep those errors as small as possible. For example, the reading test used as a dependent variable in the educational psychologist’s study is not perfect. Indeed, a group’s score on the reading test represents the group’s “true” reading ability—that is, the actual level of the construct in a group—plus or minus some random measurement error. Maybe one child’s batch of questions happened to be more difficult than average. Perhaps another student just happened to be exposed to the tested words at home. Maybe one child was especially distracted during the test, and another was especially focused. When these distortions of measurement are random, they cancel each other out across a sample of people and will not affect the group’s average, or mean. Nevertheless, an operationalization with a lot of measurement error will result in a set of scores that are more spread out around the group mean (see Figure 11.15A). A child’s score on the reading measure can be represented with the following formula: child’s reading score = child’s true reading ability +/- random error of measurement Or, more generally: dependent variable score = participant’s true score +/- random error of measurement The more sources of random error there are in a dependent variable’s measurement, the more variability there will be within each group in an experiment (see Figure 11.15A). In contrast, the more precisely and carefully a dependent variable is measured, the less variability there will be within each group (see Figure 11.15B). And lower within-groups variability is better, making it easier to detect a difference (if one exists) between the different independent variable groups. Solution 1: Use Reliable, Precise Tools. When researchers use measurement tools that have excellent reliability (internal, interrater, and test-retest), they can reduce measurement error (see Chapter 5). When such tools also have good construct validity, there will be a lower error rate as well. More precise and accurate measurements have less error. Solution 2: Measure More Instances. A precise, reliable measurement tool is sometimes impossible to find. What then? In this case, the best alternative is to use a larger sample (e.g., more people, more animals) or take multiple measurements on the sample you have. In other words, one solution to measuring badly is to take more measurements. When a tool potentially causes a great deal of random error, the researcher can cancel out many errors simply by including more people in the sample or measuring multiple observations. Is one person’s score 2 points too high because of a random measurement error? If so, it’s not a problem, as long as another participant’s score is 2 points too low because of a random measurement error. The more participants or items there are, the better the chances of having a full representation of all the possible errors. The random errors cancel each other out, and the result is a better estimate of the “true” average for that group. INDIVIDUAL DIFFERENCES Individual differences can be another source of within-groups variability. They can be a problem in independent-groups designs. In the experiment on money and mood, for example, the normal mood of the participants must have varied. Some people are naturally more cheerful than others, and these individual differences have the effect of spreading out the scores of the students within each group, as Figure 11.16 shows. In the $1.00 condition is Candace, who is typically unhappy. The $1.00 gift might have made her happier, but her mood would still be relatively low because of her normal level of saltiness. Michael, a cheerful guy, was in the no-money control condition, but he still scored high on the mood measure. More Information Figure 11.16 Individual differences. Overall, students who received money were slightly more cheerful than students in the control group, but the scores in the two groups overlapped a great deal. Looking over the data, you’ll notice that, on average, the participants in the experimental condition did score a little higher than those in the control condition. But the data are mixed and far from consistent; there’s a lot of overlap between the scores in the money group and the control group. Because of this overlap, any effect of a money gift is swamped by these individual differences in mood. The effect of the gift would be small compared to the variability within each group. Solution 1: Change the Design. One way to accommodate individual differences is to use a within-groups design instead of an independent- groups design. In Figure 11.17, each pair of points, connected by a line, represents a single person whose mood was measured under both conditions. The top pair of points represents Michael’s mood after a money gift and after no gift. Another pair of points represents Candace’s mood after a money gift and after no gift. Do you see what happens? The individual data points are exactly where they were in Figure 11.16, but the pairing process has turned a scrambled set of data into a clear and very consistent finding: Every participant was a little happier after receiving a money gift than after no gift. This included Michael, who is always cheerful, and Candace, who is usually unhappy, as well as others in between. More Information Figure 11.17 Within-groups designs control for individual differences. When each person participates in both levels of the independent variable, the individual differences are controlled for, and it is easier to see the effect of the independent variable. A within-groups design, in which all participants are compared with themselves, controls for irrelevant individual differences. Finally, notice that the study required only half as many participants as the original independent-groups experiment. You can see again the two strengths of within-groups designs (introduced in Chapter 10): They control for irrelevant individual differences, and they require fewer participants than independent-groups designs. Solution 2: Add More Participants. If within-groups or matched-groups designs are inappropriate (and sometimes they are, because of order effects, demand characteristics, or other practical concerns), another solution to individual difference variability is to measure more people. The principle is the same as it is for measurement error: When a great deal of variability exists because of individual differences, a simple solution is to increase the sample size. The more people you measure, the less impact any single person will have on the group’s average. Adding more participants reduces the influence of individual differences within groups, thereby enhancing the study’s ability to detect differences between groups. ❮❮ For more on computing confidence intervals, see Statistical Review: Inferential Statistics, pp. 493–505. Another reason to use a larger sample is that it leads to a more precise estimate. Computing the 95% CI for a set of data requires three elements: a variability component (based on the standard deviation), a sample size component (where sample size goes in the denominator), and a constant. The larger the sample size, the more precise our estimate is and the narrower our CI is (see Table 11.2). TABLE 11.2 The 95% CI represents the precision of our statistical estimates. This table summarizes the relationship between the 95% CI and measurement error, irrelevant individual differences, and situation noise. TO Increase the Precision of the Components Role in the 95% 95% CI (Make it Narrower), of a 95% CI CI Researchers Can: As error variability Reduce error variability in the decreases, the 95% study by using precise Variability CI will become measurements, reducing situation component narrower (more noise, or studying only one type of precise). person or animal. As sample size increases, the 95% Sample size Increase the number of CI will become component participants studied. narrower (more precise). Constant In a 95% CI, the We have no real control over the (such as a z constant is at least constant when we estimate a 95% or t value) 1.96. CI. SITUATION NOISE Besides measurement error and irrelevant individual differences, situation noise—external distractions—is a third factor that could cause variability within groups and obscure true group differences. Suppose the money and mood researchers had conducted their study in the middle of the student union on campus. The sheer number of distractions in this setting would make a mess of the data. The smell of the nearby coffee shop might make some participants feel cozy, seeing friends at the next table might make some feel extra happy, and seeing the cute person from sociology class might make some feel nervous or self-conscious. The kind and amount of distractions in the student union would vary from participant to participant and from moment to moment. The result, once again, would be unsystematic variability within each group. Unsystematic variability, like that caused by random measurement error or irrelevant individual differences, will obscure true differences between groups. Researchers may attempt to minimize situation noise by carefully controlling the surroundings of an experiment. The investigators might choose to distribute money and measure people’s moods in a consistently undistracting laboratory room, far from coffee shops and classmates. Similarly, the researcher studying anxiety and logical reasoning might reduce unsystematic situation noise by administering the logical reasoning test on a computer in a standardized classroom environment. The educational psychologist might avoid unsystematic variability in the dependent variable, reading performance, by limiting children’s exposure to alternative reading activities. Table 11.3 summarizes the possible reasons for a null result in an experiment. TABLE 11.3 Reasons for a Null Result Obscuring Factor Example Questions to Ask Not enough variability between levels One week of reading games How did the researchers might not manipulate the independent Ineffective improve reading variable? Was the manipulation of skill (compared manipulation strong? Do independent with a control manipulation checks suggest variable group), but 3 the manipulation did what it months might was intended to do? improve scores. Researchers used a pass/fail measure, when How did the researchers Insufficiently the improvement measure the dependent sensitive was detectable variable? Was the measure measurement of only by using a sensitive enough to detect dependent variable finer-grained group differences? measurement scale. Researchers manipulated three Are there meaningful levels of anxiety differences between the Ceiling or floor by threatening levels of the independent effects on people with 10- variable? Do manipulation independent volt, 50-volt, or checks suggest the variable 100-volt shocks manipulation did what it was (all of which intended to do? make people very anxious). Researchers How did the researchers measured logical measure the dependent Ceiling or floor reasoning ability variable? Do participants effects on with a very hard cluster near the top or near dependent variable test (a floor effect the bottom of the on logical distribution? reasoning ability). Too much variability within levels Logical reasoning Is the dependent variable test scores are measured precisely and affected by reliably? Does the measure multiple sources have good construct of random error, validity? If measurements Measurement error such as item are imprecise, did the selection, experiment include enough participant’s participants or observations mood, fatigue, to counteract this obscuring etc. effect? Did the researchers use a Reading scores within-groups design to are affected by better control for individual irrelevant Individual differences? If an individual differences independent-groups design is differences in used, larger sample size can motivation and reduce the impact of ability. individual differences. The money and happiness study was run in a Did the researchers attempt distracting to control any situational location, which influences on the dependent Situation noise introduced variable? Did they run the several external study in a standardized influences on the setting? participants’ mood. If the study was sound, place it in context of the body of evidence: Did the researchers use a If so, it’s useful evidence very large sample that the independent variable The independent and take has little effect on the variable could, in precautions to dependent variable. truth, have almost maximize Additional studies will no effect on the between-groups strengthen this conclusion: dependent variable. variability and What does the body of minimize within- evidence (including meta- groups analyses) say? variability? The independent When several variable could have Again, additional studies studies are a true effect on the help us evaluate this conducted on the dependent variable, possibility. What does the same but because of body of evidence (including phenomenon, the random errors of meta-analyses) say? If most 95% CIs will measurement or studies show an effect, this differ from each sampling, this one one null result might be a other just by study didn’t detect fluke. chance. it. The Opposite of Obscuring: Power and Precision When researchers use a within-groups design, employ a strong manipulation, carefully control the experimental situation, or add more participants to a study, they are increasing the precision of their estimates and increasing the study’s power. Power is an aspect of statistical validity; it is the likelihood that a study will return an accurate result when the independent variable really has an effect. If online reading games cause even a small improvement or if anxiety affects problem solving even by a small amount, will the experiment estimate that effect precisely? Will the 95% CI be reasonably narrow? A within-groups design, a strong manipulation, a larger number of participants, and less situation noise are all things that can improve the precision of our estimates. Of these, the easiest way to increase precision and power is to add more participants. Studies with large samples have two major advantages. First, as already discussed, large samples make the CI narrow—they lead to a more precise estimate of any statistic, whether it’s a mean, a correlation, or a difference between groups. Large samples are more likely to lead to statistically significant results (CIs that do not include zero) when an effect is real. Second, effects detected from small samples sometimes can’t be repeated. Imagine a study on online reading games that tested only 10 children. Even if reading games don’t work, it’s possible that just by chance, three children show a terrific improvement in reading after using them. Those children would have a disproportionate effect on the results because the sample was so small. And because the result was due primarily to three exceptional children, researchers may not be able to replicate it. Indeed, the CI for such a small sample will be very wide, reflecting how difficult it is to predict what the next study will show. In contrast, in a larger sample (say, 100 children), three exceptional kids would have much less impact on the overall pattern. In short, large samples have a better chance of estimating real effects. Null Effects Should Be Reported Transparently When an experiment results in a null effect (the CI includes zero), what should you conclude? The study might have an obscuring factor, so you might first ask whether it was designed to elicit and det