Industrial-Organizational Psychology: Research Methods in the Workplace (2017)
Document Details
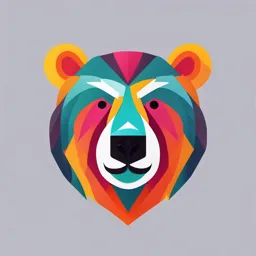
Uploaded by RelaxedPeninsula
2017
Paul E. Levy
Tags
Related
- Chapter 2: Research Methods in I/O Psychology (2017) PDF
- Industrial and Organizational Psychology (6th Edition) PDF
- I/O Psychology Reviewer PDF
- Introduction to Industrial-Organizational Psychology Lesson-1-PDF
- Lesson 1: Introduction to Industrial/Organizational Psychology PDF
- Week 3-7 Industrial-Organizational Psy Notes PDF
Summary
This is a chapter on research methods in industrial-organizational psychology, discussing the scientific method, goals, and basic concepts. It explores internal and external validity, different research designs, data collection techniques, and applies the concepts to investigating organizational issues like absenteeism. Includes a section on measurement and ethics.
Full Transcript
CHAPTER Research Methods in I/O Psychology CHAPTER OUTLINE What Is Science? Goals of Science Assumptions of Science Theories Research Terminology and Basic Concepts Independent and Dependent Variables Control PRACTITIONER FORUM: Douglas Klein Internal and External Validity A Model of the Research...
CHAPTER Research Methods in I/O Psychology CHAPTER OUTLINE What Is Science? Goals of Science Assumptions of Science Theories Research Terminology and Basic Concepts Independent and Dependent Variables Control PRACTITIONER FORUM: Douglas Klein Internal and External Validity A Model of the Research Process Types of Research Designs Experimental Methods Observational Methods Data Collection Techniques Naturalistic Observation Case Studies Archival Research Surveys Technological Advances in Survey Methodology Measurement Reliability Validity of Tests, Measures, and Scales Ethics 2 Statistics Measures of Central Tendency Measures of Dispersion Shapes of Distributions Correlation and Regression Meta-Analysis I/O TODAY Big Data Summary LEARNING OBJECTIVES This chapter should help you understand: The scientific method and its goals and assumptions The importance of theory to science and psychology Internal and external validity The complex interplay among experimental variables How research is conducted, with an emphasis on induction and deduction as well as the five steps involved in the process A variety of measurement issues such as reliability and validity Basic-level statistics ranging from descriptive statistics to correlation and regression Suppose that an organization is having a problem with absenteeism such that, on average, its employees are missing about 15 days of work per year. Absenteeism is a very expensive problem for organizations because it slows down production and it costs additional dollars to replace employees who are missing. Suppose further that this company has hired you as an I/O psychologist to help diagnose the problem, provide a potential solution, and implement and evaluate that solution. First, then, you have to figure out what the problem is. You might do this by interviewing current plant employees, by talking to managers, and by administering surveys. In addition, you might need to look at the organization’s absence data and do some statistical analysis to uncover how frequent the absences are, when they tend to occur (e.g., Mondays and Fridays versus days in the middle of the week), and whether they are occurring in certain departments more than in others. Second, you might need to collect some historical data about the company and its absence policy (usually a written policy in the employee handbook) because this may help you to understand the problem better. Third, you might use this information and your expertise in I/O psychology to develop and implement an approach to remedy the problem. Furthermore, you will need to look at the absence data again a few months after the new approach is under way to see whether absence has decreased. In addition, you might want to survey and interview employees to see if they have noticed any differences since (and as a result of) the implementation of the new approach. At each of these steps in the process, you will need to gather data in order to understand the problem and to evaluate the solution. In this chapter, I will talk about how I/O psychologists gather data and use it to improve their understanding of organizational functioning. WHAT IS SCIENCE? Science is a process or method for generating a body of knowledge. It’s important to note that this term refers not to a body of knowledge but, rather, to the process or method used in producing that body of knowledge. Science represents a logic of inquiry—a way of going about doing things to increase our understanding of concepts, processes, and relationships. What makes psychology scientific is that we rely on formal, systematic observation to help us find answers to questions about behavior. Science is not about people in white coats in a laboratory, or brilliant teachers in the thought-provoking world of academia, or mind-boggling technological advances; science is about understanding the world in which we live. But scientists are not satisfied with a superficial understanding; they strive for a complete understanding. For instance, the nonscientist may note that employees seem to be more productive when they are allowed to participate in important organizational decisions. The scientist, on the other hand, works at understanding why this is the case, what kind of participation is most important for employees, when participation might not benefit the organization, why it is that participation seems important for some employees but not for others, and so on. In the next section, we will examine the four major goals of science. science A process or method for generating a body of knowledge. Goals of Science There are many different lists of the goals or objectives of science, but I will use the list presented by Larry Christensen (1994) because it is most consistent with my thinking on the subject. First, there is description, the accurate portrayal or depiction of the phenomenon of interest. In our example, this is the focus of the nonscientist’s approach to understanding— simply noting and describing the phenomenon whereby employees are more productive when given some voice by the organization. But the scientist goes further than this—which brings us to the second goal. In an attempt to better understand the phenomenon, the scientist focuses on an explanation—that is, on gathering knowledge about why the phenomenon exists or what causes it. This usually requires the identification of one or more antecedents, which are conditions that occur prior to the phenomenon. Again, in our example, it may be that giving employees the opportunity to participate in decisions makes them feel needed and important, which leads to or causes increased effort. Of course, as scientists, we realize that almost all behaviors are complex and that there tend to be many causes or antecedents for any given behavior. The third goal of science is prediction, which refers to the ability to anticipate an event prior to its actual occurrence. Accurate prediction requires that we have some understanding of the explanation of the phenomenon so that we can anticipate when the phenomenon will occur. In our example, we may be able to predict an increase in effort on the part of the employees if we know that the company just recently sent out a survey asking for their input on important decisions about medical benefits. Without the ability to predict, we have a gap in our knowledge and are left with very little helpful information that we can provide to the company. In other words, if we have described and explained the phenomenon but cannot help the company predict this behavior in the future, our usefulness is somewhat limited. The last goal of science is control of the phenomenon—specifically, the manipulation of antecedent conditions to affect behavior. Ideally, we’d like to have a sufficiently complete understanding of the phenomenon so that we can manipulate or control variables to increase or decrease the occurrences of the phenomenon. In other words, we’d like to be able to help the company provide the appropriate antecedents so that employees will work harder and perform better. Indeed, by providing employees with opportunities for participation throughout the year, the company should be able to maintain high levels of effort and performance. In sum, we have attained some degree of scientific understanding only when a phenomenon is accurately described, explained, predicted, and able to be controlled. Assumptions of Science In order for scientists to do their work and to make sense of the world, they must have some basic assumptions about the world. First, scientists must believe in empiricism, which is the notion that the best way to understand behavior is to generate predictions based on theory, gather data, and use the data to test these predictions. Second, scientists must believe in determinism, which suggests that behavior is orderly and systematic and doesn’t just happen by chance. Imagine what psychology would be like if its basic assumption was that behavior was a chance occurrence. It would counter what all psychologists believe because it would suggest that we can neither explain nor predict behavior. In effect, if we didn’t believe that behavior is orderly, psychology would have nothing to offer society. The third basic assumption of scientists is discoverability, which suggests not only that behavior is orderly but also that this orderliness can be discovered. I’m not suggesting that behavior is so orderly that a psychologist can easily predict what an individual will do at any given moment; if it was that easy, think about how it would change the way you interact with others. For instance, if you knew exactly how a classmate would react to being asked out on a date, you would feel much less stress and be certain that you would never hear the answer “no” (since, in that event, you wouldn’t ask the question!). Psychologists do not suggest that behavior is that orderly, only that there is some order to it; so the last major assumption here is that we can experience, examine, and discover—to some extent—that orderliness. Theories A theory is a set of interrelated constructs (concepts), definitions, and propositions that present a systematic view of a phenomenon by specifying relations among variables, with the purpose of explaining and predicting the phenomenon (Kerlinger, 1986). Fred Kerlinger argues that without an emphasis on theory, science would become nothing more than a simple search for answers, lacking any framework or carefully executed process. It is theory and its accompanying propositions, according to Kerlinger, that allow us to describe, explain, predict, and control the phenomenon under investigation. Even though, as I/O practitioners, our focus may be on conducting research that will help us solve an applied problem, that research should be based on a theory, or its results should be used to help develop new theories and alter existing theories. It’s important to realize that theories are as important to applied scientific disciplines like I/O psychology as they are to less applied scientific disciplines like microbiology. theory A set of interrelated constructs (concepts), definitions, and propositions that present a systematic view of a phenomenon by specifying relations among variables, with the purpose of explaining and predicting the phenomenon. What Makes a Good Theory? As with everything else in science, there are good theories and bad theories. Scientists generally agree that a good theory should meet certain criteria or standards. First, a theory is of little use if it is not parsimonious. In other words, the theory should be able to explain a lot as simply as possible. The fewer statements in a theory, the better. If a theory has to have a proposition or statement for every phenomenon it tries to explain, it will become so big as to be unmanageable. Generally, if two theories make the same predictions, the more parsimonious one is the better theory. After all, if people can’t understand the theory or use it in their research, the theory will not help advance science by improving their understanding. The second criterion for a good theory is precision. A theory should be specific and accurate in its wording and conceptual statements so that everyone knows what its propositions and predictions are. You might notice a trade-off here between parsimony and precision, but, in fact, scientists try to develop theories that are both simple and precise: Too much of either is a potential weakness of any theory. If the theory is not very precise but instead is wordy and unclear, scientists will not know how to use the theory or be able to make predictions based on it. They would be left in the position of having to try to interpret the theory based on what they think it tries to say. Testability is the third criterion for a theory: If it can’t be tested, it can’t be useful. Testability means that the propositions presented in the theory must be verifiable by some sort of experimentation. If you have developed a theory that predicts rainfall in inches as a function of the number of Martians that land on the earth per day, you have developed a theory that is untestable (unless you know something I don’t know!) and that, therefore, is not a good theory. Notice that I have not said that the theory must be capable of being proven. The reason is that theories are not proven but, rather, are either supported or not supported by the data. We can never prove a theory; we can only gather information that makes us more confident that the theory is accurate or that it is misspecified. One of the great scientific philosophers, Karl Popper, argued that science is really about ruling out alternative explanations, leaving just one explanation or theory that seems to fit the data (Popper, 1959). He further said that the ultimate goal of a scientific theory is to be “not yet disconfirmed.” In making this statement, Popper seemed to realize that most theories are likely to be disconfirmed at some point but that it is in the disconfirmation that science advances. A CLOSER LOOK A slightly different list of the stages involved in the research process. Why are the steps of the research process so important? What would be the impact of not following these steps? Of course, a theory must also be useful, such that it is practical and helps in describing, explaining, and predicting an important phenomenon. You may be able to generate a theory about how often and when your psychology instructor is likely to walk across the room while lecturing; but I would wonder, even if your theory describes, explains, and predicts this phenomenon pretty well and is testable, whether anyone really cares. The behavior itself is not important enough to make the theory useful. By this criterion of usefulness, it would be a bad theory. Finally, a theory should possess the quality of generativity; it should stimulate research that attempts to support or refute its propositions. We may develop a theory that seems to meet all the other criteria, but if no one ever tests the theory or uses it in any way, then the theory itself would be of little value. In sum, science is logic of inquiry, and the primary objective of science is theory building. As a set of propositions about relationships among variables, a theory is developed to describe, explain, predict, and control important phenomena. Theories can be evaluated as to their parsimony, precision, testability, usefulness, and generativity. See Table 2.1 for a useful summary of the characteristics of a good theory. But how do we put all of this together and start to conduct research? That will be the focus of the rest of the chapter. First, however, we need to clarify the relationship between theories and data. Which Comes First—Data or Theory? Theory and data are of the utmost importance to science and can’t be overemphasized. Science uses both theory and data, but individual scientists have disagreed about which should come first and which is more important. Empirical observation, referred to as induction, involves working from data to theory. Basically, the argument here is that we must collect data, data, and more data until we have enough data to develop a theory. Others, however, take the opposite approach, known as deduction, which involves starting with a theory and propositions and then collecting data to test those propositions. In this case, reasoning proceeds from a general theory to data that test particular elements of that theory— working from theory to data. induction An approach to science that consists of working from data to theory. deduction An approach to science in which we start with theory and propositions and then collect data to test those propositions—working from theory to data. In their purest forms, there are problems with both the inductive and deductive approaches to research. Collecting data and then generating theories (i.e., induction) is useless unless those theories are tested and modified as a result of additional data. But generating a theory and collecting data to test its propositions (i.e., deduction) is only a partial process, too, unless those data are used to alter or refute those propositions. The approach taken by most distinguished scientists is one that combines inductive and deductive processes. TABLE 2.1 Characteristics of a Good Theory Characteristic What Does It Mean? Why Does It Matter? Parsimony The theory explains as much as possible, as simply as possible. Theories that are too complex are unmanageable and, therefore, not very useful. Precision The theory should be as specific and accurate in its language as possible. If a theory is wordy and unclear, scientists won’t know how to test it. Testability The propositions in the theory must be verifiable with experimentation. An untestable theory can’t be supported or disconfirmed and is of little use to science. Usefulness The theory should be practical A theory that only predicts or explains and describe or predict an something as trivial as the color of the pen important phenomenon. in your drawer isn’t of any worth. Generativity The theory should stimulate research aimed at testing it. If a theory never gets tested—that is, if no research follows from it—the theory has no purpose. Both approaches are depicted in Figure 2.1, which illustrates the cyclical inductive–deductive model of research. Note that it doesn’t really matter whether one starts with data (induction) or with a theory (deduction) —neither is used exclusively at the expense of the other. If one starts with a general theory (inside path of the figure), data are collected to test the theory; the data are used to make changes to the theory if necessary; more data are collected; the theory is amended again; and the process continues. If one begins with data collection (outside path of the figure), the data are used to develop a theory; additional data are collected to test this theory; the theory is amended if necessary; even more data are collected; and the process continues. FIGURE 2.1 The Cyclical Inductive–Deductive Model of Research At least initially, most research tends to be driven by inductive processes. Think about it. Even in research using the deductive approach— theory to data—the original development of the theory was probably based on some data. Hence, induction is an initial part of the process, with an ensuing emphasis on deductive methods. So here, too, we see the cyclical model in action. By now it should be obvious that there is no perfect way to “do science.” However, being aware of the goals of science, the assumptions of scientists, the criteria for good theories, and how induction and deduction feed into each other should help you understand the ways in which the scientific process is best applied. The specific types of research designs and data collection techniques will be discussed soon, but first we need to consider some basic terminology and concepts. RESEARCH TERMINOLOGY AND BASIC CONCEPTS To begin to understand what is involved in conducting experiments, you have to know what is meant by drawing a causal inference because this is what we typically want to be able to do at the completion of an experiment. Recall my earlier point that we can never prove that a theory is right or wrong; we can only gain confidence in the theory through collecting data that support it. With causality, we have a similar situation. We can never prove a causal relationship between two variables because there may be some other variable, of which we aren’t even aware, that’s causing the relationship. Thus, our greatest hope is that we have conducted our experiment carefully enough to feel confident about inferring causality from it. We make a causal inference when we determine that our data indicate that a causal relationship between two variables is likely. This inability to prove causality explains why psychologists spend a great deal of time working to design their experiments very carefully. Being able to confidently draw a causal inference depends on careful experimental design, which in turn begins with the two major types of variables. causal inference A conclusion, drawn from research data, about the likelihood of a causal relationship between two variables. Independent and Dependent Variables An independent variable is anything that is systematically manipulated by the experimenter or, at the least, measured by the experimenter as an antecedent to other variables. The intention is to determine whether the independent variable causes changes in whatever behavior we are interested in. For example, we might vary the amount of participation that we allow employees in deciding how to do their work: One group might be given no input and simply told how to do its work, a second group might be given some input in deciding how to accomplish the tasks, and a third group might be given complete control over how to do the job. In this experiment, our independent variable would be the degree of participation granted to employees. independent variable A variable that is systematically manipulated by the experimenter or, at the least, measured by the experimenter as an antecedent to other variables. The dependent variable is the variable of interest—what we design our experiment to assess. In short, we are usually interested in the effect of the independent variable on the dependent variable. For example, we might predict that participation (the independent variable) will influence employees’ job satisfaction (the dependent variable). In other words, the more opportunities employees are given to decide how to do their own work, the more satisfied they will be with their jobs. If we were to find that this is indeed the case, we would conclude that participation causes job satisfaction. Keep in mind, however, that when I say causes, I mean that we are making a causal inference, because we can’t be certain that participation causes satisfaction. This shortcut in terminology is typically used by psychologists: We talk about causation when we really mean causal inference. In I/O psychology, common dependent variables include performance, profits, costs, job attitudes, salary, promotion, attendance behaviors, and motivation. Usually, we manipulate the independent variable and measure its effect on the dependent variable. dependent variable The variable of interest, or what we design experiments to assess. Another type of variable is called an extraneous variable. An extraneous variable—also called a confounding variable—is anything other than the independent variable that can contaminate the results or be thought of as an alternative to our causal explanation. Extraneous variables get in the way and prevent us from being confident that our independent variable is affecting our dependent variable. In our participation–job satisfaction experiment, for example, performance might be an extraneous variable if better performers were given more opportunity for participation. In this case, they may be more satisfied not because of their participation but, rather, because they are getting more organizational rewards as a result of being better performers. extraneous variable Anything other than the independent variable that can contaminate our results or be thought of as an alternative to our causal explanation; also called a confounding variable. In I/O psychology, we often refer to independent and dependent variables by other, more specific names. Independent variables are frequently called predictors, precursors, or antecedents, and dependent variables are often called criteria, outcomes, or consequences. In the selection context, we often talk of predictors and criteria. We use a predictor variable to forecast an individual’s score on a criterion variable. For instance, we might say that intelligence is an important predictor of successful job performance. Predictors and criteria are discussed in much more detail later in the book, but for now it’s important to realize that these terms are often used interchangeably with independent variable and dependent variable, respectively. Control Control is a very important element of experimental design. To ensure that we can make a causal inference about the effect of our independent variable on our dependent variable—a matter of internal validity—we need to be able to exercise control over the experiment. In order to confidently draw a causal inference, we must eliminate other potential explanations of the effect. One major way of doing this is to control extraneous variables. Let’s say we’re interested in the effect of leadership style on employee performance. To examine this relationship, we could use a survey of employees to measure their leaders’ style and then match that up with the performance of individuals who work for each leader. We could then look to see if a particular leadership style results in better employee performance. However, there are many potential extraneous variables in this situation, such as employees’ ability or experience. It may be that all the employees who work for the leader with the most favorable style also happen to be the employees with the most ability. Therefore, although it looks as though leadership style leads to a particular level of performance, employees who work for a certain leader may actually be performing well because of their own ability rather than because of the leader’s style. There are various ways in which we can attempt to control for extraneous variables. First, the potentially extraneous variable can be held constant in our experiment. For instance, if we think that employees’ experience might be an extraneous variable in our leadership–job performance study, we could control for this by using as participants only those employees who have the same amount of job experience—exactly 10 years. In other words, rather than allowing experience to confound our results, we hold it constant. Then, if we find that leadership style and employee performance are related, although we cannot say for sure that style causes performance, we can be certain that employee experience is not an alternative explanation. Because all the participants in our study have the same amount of experience, any differences in performance must be due to something else. A second way in which we try to control for extraneous variables is by systematically manipulating different levels of the variable. For example, if we think that gender might be a confounding variable in our leadership–job performance study, we might treat gender as an independent variable and use a more complicated experimental design in which we look at the relationship between leadership style and job performance among female leaders versus male leaders. This would be the opposite of holding the variable constant; here, we make the variable part of the experimental design and examine whether it plays a role in affecting the dependent variable. For instance, if we find the same relationship between leadership style and job performance among male leaders as we find among female leaders, then we have successfully ruled out gender as an alternative explanation of our effect. A third way, though it’s somewhat complicated, is to use statistical control (see Pedhazur & Schmelkin, 1991). With statistical techniques such as the analysis of covariance, we can remove or control the variability in our dependent variable that is due to the extraneous variable. For instance, if we wanted to test the hypothesis that older workers outperform younger workers on some task because of their greater experience, we would need to control for variables that might be related to age. We could use statistical approaches to make sure that the older group and younger group do not differ on intelligence, quality of supervision, training opportunities, and so on. This would allow us to be more certain that any differences in performance between the two groups are due to experience, because we have controlled for the other potential explanations. PRACTITIONER FORUM Douglas Klein MA, 1989, I/O Psychology, New York University Former Principal Member and Chief Leadership Advisor at Sirota Survey Intelligence As a practitioner helping companies uncover and overcome obstacles to performance, I have been using field-based research (primarily surveys, but also unobtrusive research methods) for over 20 years. Moreover, reliable, valid, data-driven insights have been fueling strategic decision making in organizations for some time now (although there are still unfortunate examples to the contrary). Gone are the days when successful companies made million-dollar decisions based on unreliable information or management hunches. This is as true for deciding whether to build a new plant as it is for implementing a new leadership development program. My clients routinely focus on the independent, dependent, and control variables impacting business effectiveness. Recently, a major retailer wanted to understand the relationship between how it led and managed and business results. The hypothesis was that certain leadership and management practices (independent variables) had direct and measurable impacts on business outcomes such as customer satisfaction, employee engagement, operational efficiency, and financial performance (the dependent variables). The company’s employee survey data (collected through self-administered surveys) were correlated with existing archival data available for each retail location (e.g., customer ratings, performance metrics, financial performance, turnover, etc.) using a quasi-experimental design. However, we knew that locations in rural areas would have lower financial performance and urban areas would have lower customer service ratings (due to increased volumes), so location-type was used as a control variable. The findings helped this retailer understand why certain locations performed better than others (after controlling for confounding variables) and helped it anticipate future returns on investment in people (and helped it prioritize upcoming spending across a number of potential asset classes). I/O psychologists help companies recognize the importance of and manage their intangible assets (e.g., people, practices, values, etc.). It is the people who convert a company’s tangible resources into business results, so how they are led, valued, managed, and so on plays a huge role in the ultimate success of any organization. Most executives would agree that a weak culture will undermine a sound business strategy every time. In order to become a successful I/O psychologist providing consultative services, you must understand research theory/methods and statistics and be able to translate learned insights into actions to be taken. A good first step on that path is mastery of the concepts expressed in this chapter on research. Applying Your Knowledge 1. The first paragraph states that reliable, valid, data-driven insights fuel strategic decision making in large organizations. How do the goals of science align with this business practice? 2. Explain how field-based research, such as surveys and unobtrusive methods, could be helpful in gathering information for business decisions. 3. Toward the end of this feature, the difference between the tangible versus intangible assets of an organization is discussed. How would I/O psychologists be particularly suited to understanding and synthesizing both assets to increase productivity? Internal and External Validity With any experiment, the first thing we are interested in is whether our results have internal validity, which is the extent to which we can draw causal inferences about our variables. In other words, can we be confident that the result of our experiment is due to the independent variable that we manipulated, not to some confounding or extraneous variable? Returning to our participation–job satisfaction study, we would have high internal validity if we were confident that participation was the variable that was affecting employees’ satisfaction levels. If, however, it was actually the rewards associated with better performance that led to high satisfaction (as I suggested, these rewards might be an extraneous variable), then we couldn’t rule out rewards as an alternative explanation for differences in satisfaction; we would thus have internal validity problems. internal validity The extent to which we can draw causal inferences about our variables. The other major type of validity that is important when designing experiments is external validity, the extent to which the results obtained in our experiment generalize to other people, settings, and times. Suppose we conduct a study in which we find that those employees who are given specific, difficult goals to work toward perform better than those who are simply told to do their best. We want to be able to say that this effect will generalize to other employees in other companies at other times. Obviously, external validity is extremely important to the development of science; to the extent that we devise studies that are low in external validity, we are not moving science forward. For instance, some criticize I/O research done with student participants as not being generalizable to “real-world” employees. If the work that is done with students does not generalize to employees, it is not very useful for increasing our understanding of the phenomenon under study. (For a very thoughtful discussion on this topic, see Landers and Behrend, 2015.) external validity The extent to which the results obtained in an experiment generalize to other people, settings, and times. However, external validity is always an empirical question—that is, a question that can be answered only through experimentation, experience, or observation. We can’t simply conduct a study and argue that it is or is not externally valid. Rather, the external validity of this one study is demonstrated by replicating it with different participants, in different settings, at different times. We can also try to use representative samples of the population to which we want to generalize and argue that our results probably generalize to others. However, to be sure, we have to collect additional data. There is an important trade-off between internal and external validity that often demands the researcher’s attention (Cook & Campbell, 1979). As we rule out various alternative explanations by controlling more and more elements of the study to successfully increase our internal validity, what happens to the generalizability of our results? It may decline because, now, a one-of-a-kind situation has been created that is so artificial it is not likely to be externally valid. Put more simply, the findings may not generalize to other situations because no other situation is likely to be very similar to the one we’ve created. So, as we gain control for internal validity purposes, we may lose the potential for external validity. Of course, if we don’t control and rule out alternative explanations, we will not be confident about our causal explanation (internal validity), even if the results have the potential to generalize to other settings and participants. And in any case, if we aren’t sure what the results mean, what good is external validity? My point here is that we have to start with internal validity because, without it, our research really can’t make much of a contribution to the knowledge in the field. But we also need to balance the two types of validity, as too much control can reduce the extent to which the results are likely to generalize. A Model of the Research Process There are various ways to go about conducting a research project, but in this section we will discuss a general approach that should act as a guide for you in developing and conducting your own research. It should also help you understand how research in psychology progresses through various stages. The model is presented in Figure 2.2. Usually, the first step in any research project is to formulate testable hypotheses. A hypothesis is a tentative statement about the relationship between two or more variables. Hypotheses are most often generated as a result of a thorough review of the literature. Sometimes, however, they arise from the experimenter’s own experiences or from a question that has not yet been answered in the published literature. Regardless of how one gets there, hypothesis generation is the first formal step in this process. hypothesis A tentative statement about the relationship between two or more variables. The second step involves the actual designing of the study, which we’ve talked about previously with respect to control, internal validity, and external validity. The two basic choices that need to be made at this stage are who the participants in your study are going to be and what is going to be measured. Although research participants in general can include children, older adults, animals, and people suffering from various disorders, participants in most I/O psychology experiments are either college undergraduates or organizational employees. As for measures, we typically have one or more independent variables and dependent variables. After the study is designed, it’s time to actually go about collecting the data. This can be done in many different ways. Some studies require data collection over more than one time period, whereas others may require the use of more than one group of participants. Some data are collected through face-to-face interactions, other data are collected by mail, and still other data are collected via e-mail or the Internet. We’ll talk briefly about some of the more common approaches to data collection in the following sections. FIGURE 2.2 Stage Model of the Research Process Once the data are collected, the researcher needs to make sense out of them. This is usually done through some sort of statistical analysis, an area in which I/O psychologists are well schooled because the field of I/O is one of the more quantitative fields in psychology. I/O psychologists make great use of the various analytic methods that are available. At this stage of the process, the researcher goes back to the original hypotheses and research questions and uses statistical techniques to test those hypotheses and answer those research questions. The last step in the research process is writing up the results. This is the point at which the I/O researcher goes back to the original ideas that were used to generate the hypotheses and describes those ideas and the research on which they were based. The researcher will then present the hypotheses and describe in detail the procedures that were used to carry out the research. The last two sections of the write-up include a presentation of the results and a discussion of what they mean, how they fit into the existing literature, how they help to solve a problem, and what implications they have for organizational functioning. Ideally, this paper can be published in a journal that is an outlet for research of this type and that allows researchers to communicate their findings to other scholars and practitioners. Papers like this one provide important information that benefits employers, employees, and organizations as they all work to be more productive and effective. Sometimes these research reports are presented at national conferences, serve as work toward a student’s master’s or doctoral degree, or meet the requirements of a psychology course or an honors curriculum. Whether one is writing up the results of an honors project, a small study done for an experimental psychology class, or a major project to be published in one of the top I/O journals, the process is the same in terms of the steps involved in the research, as well as in the actual written presentation of the research project. For guidelines, see the Publication Manual of the American Psychological Association (American Psychological Association, 2009). TYPES OF RESEARCH DESIGNS There are various ways in which studies are designed to answer a particular research question. In this section, we will consider the most frequently employed methods and look at some examples to further your understanding. Experimental Methods We have already examined the inductive–deductive cycle of research, as well as the five major steps in the research process. Now we turn to the experimental methods that the researcher can employ in order to answer particular research questions. Experimental methods are characterized by two factors: random assignment and manipulation. Table 2.2 presents a learning aid to help you better understand how different methodologies and data collection techniques can be applied to the same research problem—in this case, the effect of training on individual employee performance. experimental methods Research procedures that are distinguished by random assignment of participants to conditions and the manipulation of independent variables. Random assignment refers to the procedure by which research participants, once selected, are assigned to conditions such that each one has an equally likely chance of being assigned to each condition. For instance, if we were interested in measuring the effect of training on job performance, we would want to randomly assign participants to training and no-training conditions so as to provide a fair test of our hypothesis. If we assigned all of the good performers to the training condition and found that their performance was better than that of the no-training group, the reason could be that the training group was composed of better performers than the notraining group even before training. In other words, pretraining performance could be an extraneous variable. To control for this and other potential extraneous variables, we use random assignment to conditions. Of course, it is possible that even with random assignment we could end up with all of the good performers in one condition and the poor performers in the other condition, but random assignment makes this very unlikely. To make sure our random assignment “worked” we might measure performance at the beginning of the research to confirm that there are no significant differences on performance across groups. random assignment The procedure by which research participants, once selected, are assigned to conditions such that each one has an equally likely chance of being assigned to each condition. TABLE 2.2 Research Designs and Methodologies The effect of training on individual employee performance can be investigated in many different ways, such as the following: Research Design Experimental Methodology Example Laboratory experiment Student participants are randomly assigned to the training condition (experimental) or the no-training condition (control); then performance is measured. Field experiment Employees in a manufacturing organization are randomly assigned to the training condition (experimental) or the notraining condition (control); then performance is measured. Quasiexperiment All the employees in one department of a manufacturing organization are assigned to the training condition (experimental), and all the employees in another department of the same organization are assigned to the no-training condition (control); then performance is measured. Observational Naturalistic observation The researcher watches individuals as they receive or do not receive training and records what they experience as well as the individuals’ performance behaviors. Archival research The researcher identifies an existing data set that includes whether or not individuals experienced training and also individuals’ performance levels. Survey Employees are given a questionnaire that asks them how much training they have received at their organization and what their most recent performance appraisal ratings were. The second necessary factor associated with experimental methods is the manipulation of one or more independent variables. With experimental designs, we don’t just measure our independent variables; we systematically control, vary, or apply them to different groups of participants. In our training–job performance example, we systematically provide training to one group of participants and no training to another. Random assignment and manipulation of independent variables are the keys to controlling extraneous variables, ruling out alternative explanations, and being able to draw causal inferences. In other words, these two techniques increase the internal validity of our experiment. They are the reason that experimental designs almost always have higher internal validity than observational designs. manipulation The systematic control, variation, or application of independent variables to different groups of participants. Laboratory Experiments One type of experimental methodology is the laboratory experiment. Random assignment and manipulation of independent variables are used in a laboratory experiment to increase control, rule out alternative explanations, and increase internal validity. Most laboratory experiments take place in a somewhat contrived setting rather than a realworld work setting. For instance, two of my former students and I conducted a study in which undergraduates were randomly assigned to one of four conditions (Medvedeff, Gregory, & Levy, 2008). The four conditions varied in the type of feedback given (positive or negative) and the nature of the feedback (process based or outcome based). We were interested in whether these differences influenced how likely participants were to request more feedback. We found that the feedback manipulations (positive vs. negative and process vs. outcome) mattered, and participants did seek more feedback in some conditions than in others. The findings revealed that participants paid more attention to the additional peer information than to the additional self-rating information. Because of our random assignment of participants to experimental conditions and our careful manipulation of the additional information (i.e., the selfratings and peer ratings), we were able to rule out other plausible alternatives and argue that the difference in final judgments was due to different degrees of trust in the sources of the additional information. Recall the internal validity–external validity trade-off that we discussed earlier. Laboratory experiments give rise to that very problem. Although they are typically very high in internal validity because of the extent to which researchers have control of the laboratory context, their external validity, or generalizability, is often questioned because of the contrived nature of the laboratory setting. In an attempt to counter this criticism in the study described above, my colleague and I invited as participants only those students who were also employed at least part time. In addition, the majority of our participants had real-life experience in conducting performance appraisals. This factor improved the external validity of our study because our participants were much like the population to whom we wanted to generalize. That they had actual real-world performance appraisal experience made the task we asked them to do (i.e., make performance judgments) appear more reasonable, thus increasing external validity. Imposing enough control to maintain high levels of internal validity, having realistic scenarios, and using participants who are representative of the working world for external validity are important issues in experimental designs. Field Experiments and Quasi-Experiments To overcome the problems associated with the artificiality of laboratory settings, I/O researchers sometimes take advantage of the realism of field settings and do field experiments. Here, we still use random assignment and manipulations of independent variables to control for extraneous variables, but we do so within a naturally occurring real-world setting. Admittedly, these experiments are not used a great deal in I/O research because it is difficult to find real-world settings in which random assignment and manipulations are reasonable. Organizations are typically not willing to allow a researcher to come in and randomly assign employees to a training or no-training group because such arrangements could disrupt productivity and organizational efficiency. field experiment An approach to research that employs the random assignment and manipulation of an experiment, but does so outside the laboratory. A more viable alternative is a quasi-experiment, which resembles a field experiment but does not include random assignment (Cook & Campbell, 1979). Quasi-experiments usually involve some manipulation of an independent variable, but instead of random assignment of participants to conditions, they often use intact groups. In our training–job performance study, for example, it would be difficult to find an organization that would allow random assignment of individuals to conditions, but it might be possible to assign departments, work groups, or plants to conditions. In this case, perhaps four work groups could be assigned to the training condition and four others to the no-training condition. And to control for potential extraneous variables, we could measure and control for any preexisting differences between the two experimental conditions in terms of experience, gender, performance, attitudes, and so on. Quasi-experiments are very common in I/O psychology because they are more feasible to conduct than field experiments but still allow for reasonable levels of internal validity. quasi-experiment A research design that resembles an experimental design but does not include random assignment. Observational Methods Studies that employ observational methods are sometimes called correlational designs because their results are usually analyzed by correlational statistics, which we will talk about a bit later in this chapter. This kind of research is also often described as descriptive because we focus exclusively on the relationships among variables and thus can only describe the situation. Descriptive studies are not true experiments because they don’t involve random assignment or the manipulation of independent variables. In these designs, we make use of what is available to us, and we are very limited in drawing causal inferences. All we can conclude from such studies is that our results either do or do not indicate a relationship between the variables of interest. Although we can use this approach in either the laboratory or the field, it is much more common in the field because it is here that we are usually limited in terms of what we can control. observational methods Research procedures that make use of data gathered from observation of behaviors and processes in order to describe a relationship or pattern of relationships. In consulting with one company, we were interested in the relationship between job satisfaction and performance of the company’s employees. To examine this issue, we administered a job satisfaction survey to 200 employees at a company and then measured their performance using their supervisor’s rating. Through statistical analysis, we concluded that job satisfaction was related to performance, but we could not conclude that satisfaction causes performance because we had not manipulated satisfaction or randomly assigned employees to satisfaction conditions. Indeed, it might be that performance causes job satisfaction, as the better performers are rewarded for their performance and, therefore, are more satisfied (Lawler & Porter, 1967). The only way we could have actually examined causality in this situation would have been to manipulate job satisfaction—an option that seems both nonsensical and potentially unethical. Can you think of a company that would allow you to come in and make some employees satisfied with their jobs and others dissatisfied so that you could examine the effects of these conditions on performance? Certainly, the company that hired me would not have allowed me to do that. In such situations, we typically use observational methods and draw conclusions about relationships rather than about causality. A CLOSER LOOK Why can’t we infer causality using observational methods? Descriptive research can be very important in a couple of ways. First, although we can’t infer causality from such research, we can often gather data that describe a relationship or pattern of relationships. These data can then be used to generate more causal hypotheses, which in turn can be examined with experimental designs. Second, in some cases, there is a great deal to be gained by description alone. Recall that description is one of the basic goals of science. Indeed, some important scientific findings are entirely descriptive, such as the Periodic Table of Elements in chemistry. Descriptive research in I/O psychology can be very important as well, such as when we use surveys to measure the work-related attitudes of organizational employees. This information is certainly useful for employers who may be trying to decide if it is worth implementing a new reward program for their employees. In addition, it can be integral to us as researchers who are trying to enhance our understanding of work processes. Prediction, you may remember, is another goal of science—and such studies can be very useful in predicting behavior in certain situations. So, although observational research is not as strong as experimental research with respect to inferring causality, it is important for other reasons. DATA COLLECTION TECHNIQUES There are various ways in which data can be collected for a research study. In this section, we will focus on some methods of data collection in I/O psychology, proceeding from the least to the most common approaches. Naturalistic Observation Perhaps the most obvious way to gather data to answer a research question about a behavior is to watch individuals exhibiting that behavior. We use observation in both laboratory and field settings in which we are interested in some behavior that can be observed, counted, or measured. Naturalistic observation refers to the observation of someone or something in its natural environment. One type of naturalistic observation that is commonly used in sociology and anthropology, though not so much in I/O psychology, is called participant observation; here, the observer tries to “blend in” with those who are to be observed. The observational technique more often used by I/O psychologists is called unobtrusive naturalistic observation, in which the researcher tries not to draw attention to himself or herself and objectively observes individuals. unobtrusive naturalistic observation An observational technique whereby the researcher unobtrusively and objectively observes individuals but does not try to blend in with them. For example, a consultant interested in the effect of leadership style on the functioning of staff meetings might measure leadership style through a questionnaire and then observe the behaviors and interactions that take place during a series of staff meetings conducted by various leaders. Note that, although unobtrusive naturalistic observation can be a very fruitful approach for gathering data, the researcher needs to be aware of the possibility that she is affecting behaviors and interactions through her observation. No matter how unobtrusive she tries to be, some people may react differently when an observer is present. Case Studies Somewhat similar to naturalistic observation, case studies are best defined as examinations of a single individual, group, company, or society (Babbie, 1998). A case study might involve interviews, historical analysis, or research into the writings or policies of an individual or organization. The main purpose of case studies (as with other observational methods) is description, although explanation is a reasonable goal of case studies, too. Sigmund Freud is well known for his use of case studies in the evaluation of his clients, but an I/O psychologist might use a case study to analyze the organizational structure of a modern company or to describe the professional life of a Fortune 500 CEO. I/O consulting firms often use case studies for PR purposes to showcase the good work that they do and to increase business for the firm. Case studies are not typically used to test hypotheses, but they can be very beneficial in terms of describing and providing details about a typical or exceptional firm or individual. Of course, a major concern with this approach is that the description is based on a single individual or organization, thus limiting the external validity of the description. case studies Examinations of a single individual, group, company, or society. Archival Research Sometimes social scientists can answer important research questions through the use of existing, or “secondary,” data sets. Archival research relies on such data sets, which have been collected for either general or specific purposes identified by an individual or organization (Zaitzow & Fields, 1996). One implication is immediately clear: The quality of research using an archival data set is strongly affected by the quality of that original study. In other words, garbage in–garbage out. Researchers cannot use a weak data set for their study and expect to fix any problems inherent in it. Indeed, lack of control over the quality of the data is the chief concern with archival research. archival research Research relying on secondary data sets that were collected either for general or specific purposes identified by an individual or organization. This issue aside, archival data sets can be very helpful and, in fact, are used a great deal by I/O psychologists. Available to them are data sets that have been collected by market researchers, news organizations, behavioral scientists, and government researchers. One of the largest and most extensively used in the employment area is the data set containing scores on the General Aptitude Test Battery (GATB), which was developed by the U.S. Employment Service in 1947. It includes information on personal characteristics, ability, occupation, and work performance for over 36,000 individuals. The GATB has received a good deal of attention for many years, and these data were employed in many research studies as well (Doverspike, Cober, & Arthur, 2004). Certainly, the use of secondary data sets can be very beneficial to researchers in that they do not have to spend huge amounts of time developing measures and collecting data. Going back to Figure 2.2, we find that steps 2 and 3 are already completed for the researcher who embarks on an archival study. In addition, many of the archival data sets available for use were collected by organizations with a great deal more resources than any one researcher would tend to have. Such data may also be richer in that many more variables may be involved than a single researcher would be able to include. A final strength of archival data sets is that they often include both cross-sectional data, which are collected at one point in time from a single group of respondents, and longitudinal data, which are collected over multiple time periods so that changes in attitudes and behaviors can be examined. Again, given the limited resources of most researchers, these types of dat