ch_12_monetary_policy_and_data_parameter_uncertainties.pdf
Document Details
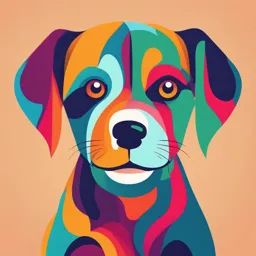
Uploaded by GlisteningMedusa
Tags
Full Transcript
Chapter 12 Monetary policy and data/parameter uncertainties 12.1 Introduction Throughout the Guide we assumed that: the central bank is assumed to know the true model of the economy, the central bank observes accurately all relevant variables timely and accurately, the central bank knows sources a...
Chapter 12 Monetary policy and data/parameter uncertainties 12.1 Introduction Throughout the Guide we assumed that: the central bank is assumed to know the true model of the economy, the central bank observes accurately all relevant variables timely and accurately, the central bank knows sources and properties of economic disturbances. For instance, in Chapter 9, we discussed New Keynesian model of monetary economics. Depending on the nature of the shock (permanent or transitory, demand or supply) the central bank can change the interest rates as soon as the shock is observed. There is no role for caution in this setting. In practice, conducting monetary policy is very difficult. There is tremendous uncertainty about the true structure of the economy, the impact policy actions have on the economy, and even about the state of the economy. Following quote from a prominent former central banker, Alan Blinder (1995), who was speaking at a meeting in Minnesota best describes the challenges a central banker faces: ‘Unfortunately actually to use such a strategy in practice you have to use forecasts knowing that they may be wrong. You have to base your thinking on some kind of monetary theory even though that theory might be wrong. And you have to attach numbers to that theory knowing that your numbers might be wrong. We at the Fed have all these fallible tools and no choice but to use them.’ Blinder continues to suggest the following ‘What can you do to try to guard against failure? First of all be cautious. Dont oversteer the ship. If you yank the steering wheel really hard a year later you may find yourself on the rocks.’ Blinder suggests to exercise caution in monetary policymaking. This effectively translates into a smooth adjustment of interest rates. The reasons for smooth adjustment of interest rates can be due to: data uncertainty (alternatively, additive uncertainty), parameter uncertainty (alternatively, multiplicative uncertainty). 161 12. Monetary policy and data/parameter uncertainties 12.2 Aims This chapter aims to introduce two major challenges, data and parameter uncertainty, that policy makers will face in deciding the monetary policy. 12.3 Learning outcomes By the end of this chapter, and having completed the Essential reading and activities, you should be able to: Discuss the importance of additive uncertainty Distinguish between news and noise in data revisions Discuss the choice of monetary policy instruments under additive uncertainty Discuss the importance of parameter uncertainty Explain the concept of certainty equivalence. Explain optimal policy conduct when the economy subject to parameter uncertainty. 12.4 Reading advice This chapter follows the work by Aruoba (2008) for discussions of data uncertainty and Brainard (1967) for discussions of parameter uncertainty. For empirical evidence see Aruoba (2008) and Sack (2000). For optimal policy instrument choice under data uncertainty you should read Poole (1970). 12.5 Essential reading Aruoba, B. ‘Data revisions are not well-behaved’, Journal of Money, Credit and Banking, 40(2–3) 2008, pp.319–340. Brainard, W. ‘Uncertainty and effectiveness of policy’, American Economic Review (papers and proceedings), 57 (2) 1967, pp.411–425. Poole, W. ‘Optimal choice of monetary policy instrument in a simple stochastic macro model’, Quarterly Journal of Economics, 84 (2) 1970, pp.197–216. 12.6 Further reading Croushore, D. and T. Stark, ‘A real time dataset for macroeconomists: does the data vintage matter?’ Review of Economics and Statistics, 2003 pp.605–17. 162 12.7. Data uncertainty Sack, B. ‘Does the Fed act gradually? A VAR analysis’ Journal of Monetary Economics, 46 2000, pp.229–256. 12.7 Data uncertainty Economic agents (policy makers, financial agents, firms, households) possess information and form their forecast in real time. As it turns out, most macroeconomic data is subject to continuous revisions. We can define data revisions in the following way. Xtf = Xtp + rtf . (12.1) where Xtp denotes the statistical agency’s initial announcement of a variable that was realised at time t, Xtf denotes the final or true value of the same variable and rtf denotes the final revision which may potentially be never observed. These data revisions, rtf , can be due to: short run revisions based on additional source data or benchmark revisions based on structural changes or updating base year. If those revisions are done because of new data arrivals that are not forecastable at the time of the forecast (news), we can refer to ‘well behaved’ revisions since there is nothing economic agents can do about these. If, however, future data revisions are forecastable at the time of the forecast these are not well behaved revisions (noise). By not making use of the forecastablity of future revisions, economic agents would violate one of the main assumption of modern macroeconomics, that is rationality. Well behaved revisions have three properties. Revisions should have a mean zero. i.e. initial announcement of the statistical agency should be an unbiased estimate of the final value of the data. Final revision should be unpredictable given the information set at the time of the initial announcement and The variance of the final revision should be small compared to the variance of the final value of the data. Aruoba (2008) shows vast empirical evidence that none of these properties of well behaved revisions are full-filled in the US data. The measurement problem is more important for output series than it is for inflation or employment/unemployment series. We first look at a case where we assume that the structure of the economy is known with certainty. However, we also assume that the economy may be subject to additive shocks; this creates data uncertainty. Data (additive) uncertainty in Poole’s model Poole (1970) analysed the implication of adding such news shocks to both the IS and LM schedules. Such shocks could come about from for instance changes in consumer tastes or government expenditures shocks on the IS side and stock market crashes and 163 12. Monetary policy and data/parameter uncertainties financial crises such as the collapse of LTCM in 1998 or a change in the central bank behaviour on the LM side. Poole’s model assumes that the parameters and structure of the model are known with certainty, which is an unrealistic assumption, but allows the IS and LM schedules to be subject to zero-mean random errors, again the so called news shocks. Define IS and LM schdules as: Y = a − bR + ε (12.2) M = c − dR + eY + η. ε and η are additive IS and LM shocks whose variances are σε2 and ση2 respectively, and for simplicity we ignore the price level in the LM curve. Alternatively, assume the monetary authorities can control real money balances, denoted by M . The authorities can either set the money supply, M , or the interest rate, R, but not both. With a downward-sloping money demand schedule, either R or M can be set and the other variable will have to change to allow the markets to clear. If the authorities set the interest rate, then from the IS schedule, the expected value of output, Y , given R, denoted E[Y | R] will be: E[Y | R] = a − bR. (12.3) Assume that the goal of the policy makers is to minimise the variance of output. From (12.2) and (12.3), Y − E[Y | R] will simply be ε and so the variance of output given that the authorities set the interest rate will be E[Y − E[Y | R]]2 = σε2 . Alternatively, the monetary authorities could set the money supply, M . In order to calculate the variance of output in this scenario, we need to calculate Y as a function of M only. From the LM schedule, we can calculate R as a function of M , Y and η and then substitute this into the IS curve. Solving for Y will give: Y = bM dε − bη ad − bc + + . d + be d + be d + be (12.4) We can then find E[Y | M ] and the variance of output given that the authorities directly control the money stock. This is given in 12.5. 2 E[Y − E[Y | M ]] = 1 d + be 2 (d2 σε2 + b2 ση2 ). (12.5) We can now examine which policy instrument, when set by the authorities, will result in a lower output variance. Consider the case where there are no IS shocks, σε2 . The variance of output under both interest rate and money targeting regimes is given in the top line of Table 12.1. It is clear that setting the interest rate and allowing money to change to clear the market is the optimal strategy. However, if there are no LM shocks, ση2 , then output variance is smaller under a policy of fixed money supply, see the bottom line of Table 12.1. Therefore, if an economy is prone to IS shocks, the authorities should keep the money supply constant. If the economy is prone to money market, LM, shocks, the interest rate should be the instrument of choice. This is also shown in Figures 12.1a and 12.1b. Figure 12.1a shows the case with IS shocks only. The output variation when the interest rate is fixed at R∗ is shown by |R , in which case the money supply has to change to clear the money markets causing the LM curve to shift so that equilibrium is at points A or B. If the money supply was kept 164 12.8. Parameter (or multiplicative) uncertainty Only LM shocks, σε2 = 0 Var[Y | R] = 0 < Var[Y | M ] = Only IS shocks, ση2 = 0 Var[Y | R] = σε2 > Var[Y | M ] = 2 b d+be ση2 2 d d+be σε2 Table 12.1: fixed then output deviations are shown by |M . Therefore, with IS shocks, in order to keep output variance at a minimum, it is best to keep the money supply fixed. In Figure 12.1b, by keeping the interest rate fixed after LM shocks, equilibrium will be unchanged at point E and output variance will therefore be zero. By keeping the money supply fixed, however, LM shocks will cause equilibrium to move between points A and B and output variance will be positive, equal to ∆Y |M . So, depending on the main source of economic shocks, whether they originate from the goods or money markets, will determine which monetary instrument the authorities should target. Figure 12.1: Poole’s analysis. 12.8 Parameter (or multiplicative) uncertainty Whereas Poole considered the case where shocks were additive in nature, Brainard (1967) examined the case where the values of the parameters in the model were not known with certainty. This is, arguably, more realistic since any model must be estimated from data. An estimated model will not only give point estimates of the parameters but will also give standard errors since there will always be measurement error, model mis-specification and other problems that cause us not to know the exact structure of the economic model. Suppose output, y, depends on a policy mix, X, a 165 12. Monetary policy and data/parameter uncertainties vector containing fiscal and monetary instruments that the government can control. The relationship between y and X is given by: y = gX. (12.6) For simplicity, assume X is a single policy instrument so that g is a scalar parameter estimate with mean gb and variance σg2 . The aim of the authorities is to minimise the variance of y around some target level, y ∗ , full employment level of output for example, subject to the constraint (8.13), i.e. min E[y − y ∗ ]2 s.t. y = gX. (12.7) If y = gX, taking averages will give yb = gbX. The problem can then be written as: min E[(y − yb)X − (y ∗ − yb)]2 s.t. y = gX ⇒ min E[(g − gb)X − (y ∗ − gbX)]2 ⇒ min E[(g − gb)2 X 2 + (y ∗ − gbX)2 − 2(g − gb)X(y ∗ − gbX)]. (12.8) Noting that E[g − gb]2 = σg2 and that E[g − gb] = gb − gb = 0, the problem then becomes: min X 2 σg2 + (y ∗ − gbX)2 . X (12.9) Differentiating this with respect to X, the choice variable, setting equal to zero and solving will give: gby ∗ X= 2 σg + gb2 ⇒ gb2 y ∗ yb = gbX = 2 < y∗. 2 σg + gb (12.10) The implication of the model is that because of the presence of uncertainty, σg2 > 0, the authorities will never push aggressively enough to make average output equal to the target level, y ∗ . To do so will simply cause output variation to increase to an intolerable level. The policy maker would rather have a stable level of output below the full employment level than very volatile output whose average was y ∗ . This is shown in Figure 12.2. From y = gX and yb = gbX, it follows that σy2 = σg2 X 2 which implies X = σy /σg . Substituting into y = gX will give the linear policy constraint in Figure 12.2. The authorities try to reach the indifference curve closest to y ∗ , the target level, subject to the policy constraint and as can be seen in Figure 12.2, the presence of uncertainty, σg2 , causes the authorities to opt for a less aggressive policy stance, causing equilibrium output to be below y ∗ . Activity 12.1 What happens in Figure 12.2 when the uncertainty, shown by σg2 , increases? Do the policy makers become more or less aggressive in reaching the target level of output, y ∗ ? Explain. 166 12.8. Parameter (or multiplicative) uncertainty Figure 12.2: Poole’s analysis. 12.8.1 The New Keynesian model and parameter uncertainty In this section we will apply Brainard’s ideas to a simple macroeconomic model in the New Keynesian spirit. We will show that if there is no parameter uncertainty, and the economy is subject to additive shocks with mean zero and constant variance, the policy maker should behave in a certainty equivalent manner, that is if the economic shocks did not occur. If, however, the economy is subject to structural changes as exemplified in parameter uncertainty, than Brainard conservatism applies. Now, suppose that the economy is characterised by: Ï€t = yt + aÏ€t−1 (12.11) and yt = −bit + εt (12.12) ε ∼ (0, σε2 ) (12.13) with where Ï€ stands for inflation, y for the business cycle component of real output (or income), i for the short term rate that the policy maker can control and ε for the stochastic demand shocks hitting the economy. We assume that the mean value of these shocks are equal to zero and their variance is given by σε2 and these are known by the policy maker. Parameters a and b are constants. By substituting (12.12) into (12.11) we obtain an expression for current inflation as a function of past inflation, the policy rate and shocks hitting the economy. Ï€t = aÏ€t−1 − bit + εt . (12.14) 167 12. Monetary policy and data/parameter uncertainties The policy maker cares about inflation stabilisation. Suppose that the quadratic loss function of the central bank takes the following form: L = (Ï€t − Ï€ ∗ )2 (12.15) where Ï€ ∗ represents the target inflation. In other words, whenever the current inflation deviates form the target inflation, the policy maker has an incentive to bring back the inflation to its target level by manipulating the policy rate, i. The case with additive uncertainty The only source of uncertainty is the presence of stochastic shocks. Given that the policy maker knows the nature of the shocks with a mean of zero (E(ε) = 0) and a constant variance (E(ε2 ) = σε2 ), it will form an expectation about these. Substitute the perceived structure of the economy into the objective function of the central bank: Le = E(aÏ€t−1 − bit + εt − Ï€ ∗ )2 or 2 Le = a2 Ï€t−1 + b2 i2t + E(ε2 ) +Ï€ ∗ 2 − 2aÏ€t−1 bit + 2aÏ€t−1 E(εt ) | {z } | {z } 0 σε2 ∗ ∗ ∗ −2aÏ€t−1 Ï€ − 2bit E(εt ) +2bit Ï€ − 2 E(εt ) Ï€ . | {z } | {z } 0 0 Note that, by setting E(ε) = 0 and E(ε2 ) = σε2 we have inserted policy makers expectations about the shocks. The policy maker’s job is to minimise the loss with the use of the monetary policy instrument it . ∂Le = 2b2 it + 2bÏ€ ∗ − 2aÏ€t−1 b = 0 ∂it it = −π ∗ + aÏ€t−1 . b It is important to notice that policy rate set by the policy maker is the same as the one that it would set if there were no shocks hitting the economy. This is the certainty equivalence result. That means additive shocks do not affect the way monetary policy is conducted. The best the policy maker can do is simply to ignore them. The case with parameter uncertainty Now, we can include a bit of complication. The economic environment is the same as in the previous section except that the parameter b of the real income equation is allowed to vary over time. We capture this by adding a time subscript to parameter b. Specifically: yt = −bt it + εt (12.16) with ε ∼ (0, σε2 ), 168 b ∼ (bb, σb2 ) (12.17) 12.8. Parameter (or multiplicative) uncertainty where the shocks are still additive, however we also see that the parameter b has a certain distribution such that its mean is bb and its variance is σb2 . It is important to note that this information is available to the policy maker such that it can form expectations about the value of the parameter b. By substituting (12.16) into (12.11) we obtain again an expression for current inflation as a function of past inflation, the policy rate, the shocks hitting the economy and the novel element of time varying parameter b. Ï€t = aÏ€t−1 − bt it + εt . (12.18) The problem of the central bank now becomes: Le = E(aÏ€t−1 − bt it + εt − Ï€ ∗ )2 2 = a2 Ï€t−1 + E(b2t )i2t + E(ε2 ) +Ï€ ∗ 2 − 2aÏ€t−1 E(bt )it + 2aÏ€t−1 E(εt ) | {z } | {z } 0 σε2 ∗ ∗ ∗ +2aÏ€t−1 Ï€ + 2E(bt )it E(εt ) −2E(bt )it Ï€ − 2 E(εt ) Ï€ . | {z } | {z } 0 0 Remember that you can write the variance of b as σb2 = E(bt − bb)2 = E(b2t − 2btbb + bb2 ). Given that E(bt ) = bb that is equal to σb2 = E(b2t ) − E(bb2 ). That allows us to rewrite the Le as: 2 Le = a2 Ï€t−1 + E(b2t )i2t + σε2 + Ï€ ∗ 2 − 2aÏ€t−1 E(bt )it + 2aÏ€t−1 Ï€ ∗ + 2E(bt )it Ï€ ∗     2 = a2 Ï€t−1 + ï£sigma2b + E(bb2 ) i2t + σε2 + Ï€ ∗ 2 − 2aÏ€t−1 E(bt ) it + 2aÏ€t−1 Ï€ ∗ + 2 E(bt ) it Ï€ ∗ | {z } | {z } | {z } b b2 b b b b 2 = a2 Ï€t−1 + σb2 i2t + σε2 − 2aÏ€t−1bbit + 2aÏ€t−1 Ï€ ∗ + bb2 i2t + Ï€ ∗ 2 + 2bbit Ï€ ∗ . We can now solve the policy maker’s optimisation problem that is: ∂Le 2 ∗ b b b = 2σb it − 2aÏ€t−1 b + 2 bit + Ï€ b = 0 ∂it it = (aÏ€t−1 − Ï€ ∗ )bb (σ 2 + bb2 ) b = (aÏ€t−1 − Ï€ ∗ ) . (σ 2 + bb2 )/bb b The expression σb /bb refers to the coefficient of variation. It represents the trade-off between returning inflation to target and increasing uncertainty about inflation depends on the variance of the parameter relative to its mean level. A large coefficient of variation means for a small reduction in the inflation bias the central bank induces a large variance into future inflation. Once parameter uncertainty is taken into account inflation variance depends on the interest rate reactions. Policy maker’s decisions affect uncertainty of future inflation. Hence, rather than strong reaction or ‘cold turkey’, gradualism (sustained policy reaction) is preferable (Brainard conservatism). 169 12. Monetary policy and data/parameter uncertainties 12.8.2 Graphical exposition Finally, we can show the effect of data and parameter uncertainties on policymaking in a simple graphical setting. Consider Figure 12.3 following Sack (2000). Suppose that there is a negative relationship between real output (horizontal axis) and policy rates (vertical axis, which characterises the IS curve. Upper panel shows the case without any uncertainty. As is clear whenever the actual output deviates from desired output, the policy maker can adjust policy rates to reach the desired level. Middle panel shows the case with additive uncertainty (only news shocks are considered here). Here, the policy maker is not sure about the quality of the data it receives (as shown by parallel dashed lines). Nevertheless, it acts as if it knows the data with certainty, as there is nothing it can do about the shocks (certainty equivalence). Finally, lower panel shows the case with parameter uncertainty. Since, the nature of the relationship between output and policy rate is time-varying, the slope is changing over time. If the policy makers acts with aggression, that is trying to reach the desired output by increasing the policy rate a lot, the economy may find itself at an equilibrium that is even less desirable than before. What can the policy maker do? Figure 12.3: Poole’s analysis. 170 12.9. Interest rate smoothing In Figure 12.4 Sack (2000) demonstrates a possible alternative to the bad outcome in line with the analytical discussion in the previous section. The policy maker moves in steps (interest smoothing). First, it adjusts the policy rates in the right direction. When the (relatively benign) state of the economy is revealed, it moves again in the same direction. The policy maker thereby exploits arrival of new information and can reduce the size of potential errors it can commit. Figure 12.4: Poole’s analysis. 12.9 Interest rate smoothing As mentioned in the introduction central banks tend to change interest rates i) in small steps and ii) often in the same direction for consecutive periods. Figure 12.5 shows this case for the three major central banks. The Federal Reserve, the European Central Bank and the Bank of England. Policy makers are usually not only uncertain about the state of the economy (data uncertainty) but also on the structural parameters of the economy therefore interest rates are set in a smooth fashion. 171