Brainard - 1967 - Uncertainty and the Effectiveness of Policy.pdf
Document Details
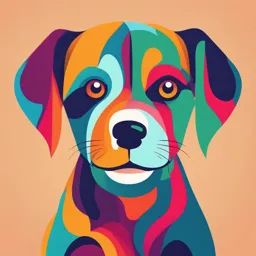
Uploaded by GlisteningMedusa
Yale University
1967
Tags
Full Transcript
Uncertainty and the Effectiveness of Policy Author(s): William C. Brainard Source: The American Economic Review , May, 1967, Vol. 57, No. 2, Papers and Proceedings of the Seventy-ninth Annual Meeting of the American Economic Association (May, 1967), pp. 411-425 Published by: American Economic Associ...
Uncertainty and the Effectiveness of Policy Author(s): William C. Brainard Source: The American Economic Review , May, 1967, Vol. 57, No. 2, Papers and Proceedings of the Seventy-ninth Annual Meeting of the American Economic Association (May, 1967), pp. 411-425 Published by: American Economic Association Stable URL: https://www.jstor.org/stable/1821642 JSTOR is a not-for-profit service that helps scholars, researchers, and students discover, use, and build upon a wide range of content in a trusted digital archive. We use information technology and tools to increase productivity and facilitate new forms of scholarship. For more information about JSTOR, please contact [email protected]. Your use of the JSTOR archive indicates your acceptance of the Terms & Conditions of Use, available at https://about.jstor.org/terms is collaborating with JSTOR to digitize, preserve and extend access to The American Economic Review This content downloaded from 92.202.12.4 on Thu, 02 May 2024 06:25:29 +00:00 All use subject to https://about.jstor.org/terms UNCERTAINTY AND THE EFFECTIVENESS OF POLICY* By WILLIAM BRAINARD Yale University Economists concerned with aggregative policy spend a great deal of their time discussing the implications of various structural changes for the effectiveness of economic policy. In recent years, for example, monetary economists have debated at great length whether the rapid growth of nonbank financial intermediaries has lessened the effectiveness of conventional instruments of monetary control. Similarly, in discussions of the desirability of the addition or removal of specific financial regulations the consequences for the effectiveness of policy play an important role. One of the striking features of many of these discussions is the absence of any clear notion of what "effectiveness" is. At times it appears to be simply "bang per buck"-how large a change in some crucial variable (e.g., the long-term bond rate) results from a given change in a policy variable (e.g., open market operation). A natural question to ask is why a halving of effectiveness in this sense should not be met simply by doubling the dose of policy, with equivalent results. It seems reasonable to suppose that the consequences of a structural change for the effectiveness of policy should be related to how it affects the policy-maker's performance in meeting his objectives. Suppose, for example, that the policy-maker wants to maximize a utility function which depends on the values of "target" variables. If, after some structural change, the policy-maker finds he is able to score higher on his utility function, then presumably the structural change has improved the effectiveness of policy and vice versa. One of the implications of the "theory of policy" in a world of certainty or "certainty equivalence" is that structural changes which simply alter the magnitude of the response to policy do not alter the attainable utility level.' Hence such structural changes do not alter effectiveness in the above sense. Another feature of the theory of policy in a world of certainty is that a policy-maker with more instruments than targets is free to discard the excess instruments, and it makes no difference to his performance which ones he discards. These results are crucially dependent on the assumption that the response of target variables to policy in* I am indebted to Samuel Chase, Jr., Arthur Okun and James Tobin for many useful suggestions. A version of Parts I and II of this paper was presented at the Conference on Targets and Indicators of Monetary Policy held at U.C.L.A. in April, 1966. 1 Assuming that the levels of instruments do not enter directly into the utility function. 411 This content downloaded from 92.202.12.4 on Thu, 02 May 2024 06:25:29 +00:00 All use subject to https://about.jstor.org/terms 412 AMERICAN ECONOMIC ASSOCIATION struments is known for certain. Since it is difficult to imagine a real world policy-maker in such an enviable position, it would seem worth- while to explore the implications of relaxing that assumption. The first two sections of this paper discuss the implications of uncertainty in the response to policy actions for the selection of optimal policy. Optimal policy in the presence of this type of uncertainty is found to differ sig- nificantly from optimal policy in a world of certainty. For example, in general all instruments are used, even if there is only one target variable. Analysis of the optimality question also provides some insight into what constitutes effectiveness. In the third section it is shown that, not surprisingly, the way a structural change alters the effectiveness of policy depends on how it affects both the expected magnitude and the predictability of response to policy actions. The third section goes on to discuss briefly some of the problems involved in assessing the consequences of structural change for the effectiveness of policy when there are several instruments and several targets, and where the structural change affects the response of the system to disturbances as well as policy instruments. I. One Target-One Instrument It is instructive to discuss the complications uncertainty creates in a world of one target and one instrument before discussing the problem of optimal use of policy instruments when there are many instruments and targets. Suppose that the policy-maker is concerned with one target variable (y). Assume that y depends linearly on a policy instrument (P)-for example, government expenditures-and various exogenous variables-for example, autonomous investment demand. For our present purposes the impact of exogenous variables may be summarized in a single variable (u). (1) y-aP + u where a determines the response of y to policy action. The policy-maker faces two kinds of uncertainty. First, at the time he must make a policy decision he is uncertain about the impact of the exogenous variables (u) which affect y. This may reflect his inability to forecast perfectly either the value of exogenous variables or the response of y to them. Second, the policy-maker is uncertain about the response of y to any given policy action. He may have an estimate a of the expected value of the response coefficient a in (1) above, but he is aware that the actual response of y to policy action may differ substantially from the expected value. At the time of the 1964 tax cut, for example, there was considerable uncertainty over the magnitude of the tax multiplier. Both types of uncertainty imply that the policy-maker cannot guar- This content downloaded from 92.202.12.4 on Thu, 02 May 2024 06:25:29 +00:00 All use subject to https://about.jstor.org/terms TOPICS IN MONEY 413 antee that y will assume its t ent implications for policy ac ent by itself, has nothing to is "in the system" independent of any action he takes. The assumption that all of the uncertainties are of this type is one of the reasons Theil and others are able to prescribe "certainty equivalence" behavior; that is, that the policy-maker should act on the basis of expected values as if he were certain they would actually occur. Since in this case the variance and higher moments of the distribution of y do not depend on the policy action taken, the policy-maker's actions only shift the location of y's distribution. In the presence of uncertainty about the response of y to policy actions, however, the shape as well as the location of the distribution of y depends on the policy action. In this case the policy-maker should take into account his influence on the variability of y.2 We will assume that the policy-maker chooses policy on the basis of "expected utility." In particular, we will follow Theil in assuming that the policy-maker maximizes the expected value of a quadratic utility function. In the one-target case this is simply: (2) U = - (y YT where y* is the target value of The assumption of the quadratic enables us to restrict our attention to the mean and variance of y and to compare our findings directly with the familiar certainty equivalence results. The assumption of a quadratic is, of course, subject to the objection that it treats positive and negative deviations from target as equally important. The use of a fancier utility function would provide additional reasons for departing from y certainty equivalence. The precise relationship between policy actions and the variance of y is not obvious. In (1) above, for example, the policy-maker may believe that the response coefficient a is a random variable depending on some unobserved variables, and that it is correlated with u. In that case the y is a random variable with a variance given by: (3) ~~ ~~~2 2 2 2 (3) O'r = 01auP + au + 2PoTaouP where 0- and o.2 are the variances of u and a, respectiv correlation coefficient between u and a. On the other hand, it is possible to conceive of part of the uncertainty of y as the consequence of estimation error. Even if the policy-maker 2 Theil is of course aware that certainty equivalence behavior is not optimal in this case. In fact, he suggests that the sampling errors in the response coefficients are the most "dangerous" ones for a policy-maker who acts as if all random coefficient matrices coincide with their expectations [4, p. 74]. This content downloaded from 92.202.12.4 on Thu, 02 May 2024 06:25:29 +00:00 All use subject to https://about.jstor.org/terms 414 AMERICAN ECONOMIC ASSOCIATION regards the "true" population response coefficient a as nonrandom, he may have to base his actions on an estimate of it obtained by fitting equation (1) to sample data. The estimate he uses will be a random variable, and its variance will affect the "variance" of y around its forecast value.3 As in the case where the population response coefficient is random, the magnitude of the policy action affects the contribution of this type of uncertainty to the variance of y. In this case, however, the contribution depends on the difference between the policy taken in the forecast period and the average level of policy pursued in the sample period used in estimating a. Assuming the u's are independent over time, a will be uncorrelated with the u for the forecast period and equation (3) may be rewritten: (3') - 2 2 p)2 + 2 where P is the average P for the sample period on which the estimate of a is based. Although we will use the first formulation to illustrate the significance of uncertainty in the response of y to policy actions, our results can be translated easily for use in the forecast error case. Assuming the response coefficient is a random variable, we may find the expected utility associated with a given policy action by substituting (1) in (2): (4) E(U) = - y y*) + 2] [Q~P ?j~ - 2 2 2 2 [(-P + U - y*)2 + caP + 0u + 2pJaOuPj where y and ui are the expected values of y and u, respectively. There is no reason to suppose that ut equals zero. By differentiating (4) with respect to P and setting the derivative equal to zero, the optimal value of P is easily found to be: (5) p* *(y* - ul) -Pa0au = a2 + ?a2 The optimal policy indicated by equation (5) clearly differs from the policy which would be pursued in a world of certainty or of certainty equivalence. The policy-maker should make use of more information than the expected value of the exogenous variables and of the response coefficient of a. Even when a and u are independently distributed, he 3Hooper and Zellner provide a discussion of the error of forecast for multivariate regression models. In genera] the variance of the forecast error is F2 =O(xXlXu)[1+XF'-1XF] where x is the matrix of sample observations on the independent variables, and XF is the vector of deviations of the independent variables from their sample means for the forecast period. This content downloaded from 92.202.12.4 on Thu, 02 May 2024 06:25:29 +00:00 All use subject to https://about.jstor.org/terms TOPICS IN MONEY 415 should make use of information about the variance of a as well as its mean. If a and u are not independent, he also needs to know their correlation. The assumption of a quadratic utility function does not lead to certainty equivalence except when the policy-maker is certain about the effects of his actions. Another interesting implication of (5) is that it does not in general pay to aim directly at the target. If a and u are inde- pendent, for example, equation (5) can be rewritten to show that the fraction of the expected "gap" between a and y* which should be filled by policy action depends only on the coefficient of variation of a: (5') P* = 9g/(1 + V? ) where g= (y*-u)/a, the expected gap, and V equals O-a/d, the coefficient of variation of a.4 Only if the policy-maker is absolutely certain about a (V= 0) will he close the entire gap; so long as V is finite, he will partially fill the gap. Some care must be used in interpreting this result. The gap in this context is not the difference between what policy was "last period" and what would be required to make the expected value of y equal to y*. In the example we have used, the gap is the difference between P equal to zero-the point where the variance of y is least-and the P required to give an expected value of y equal to y*. If the expected value of a and u and the standard deviation of a remained unchanged for several periods, the optimal policy would also remain unchanged-the policymaker would not reduce the gap in successive periods. In the case of "forecasting error" the gap is the difference between the average value of policy in the sample used to estimate a and the P which gives y equal to y*. In this case, if the parameter a were reestimated each period and the expected value of u remained the same, policy would be continually revised, making the expected value of y closer and closer to y* in successive periods. A natural question to ask is to what extent uncertainty about a affects optimal behavior. Equation (5) indicates that "moderate" uncertainty about a may have a substantial effect. Suppose, for illustrative purposes, that the monetary authority believes that the equation Y=aM+u correctly specifies the relationship between the stock of money (M), an exogenous variable (u), and money income (Y). It puts its staff to work estimating the relationship and obtains an estimate of 5 for the value of a, significant at a "t" level of 2. Further suppose that for the sample of observations on Y and M used to estimate a, the average level of M was $100. Now suppose that for the next period the desired level of Y is $650 and the expected value of u is taken to be $50. In a 4 (5') can be used for the estimation error case if P* is interpreted as the deviation of optimal policy from the mean of P during the sample period, and u is defined to include a times that mean. This content downloaded from 92.202.12.4 on Thu, 02 May 2024 06:25:29 +00:00 All use subject to https://about.jstor.org/terms 416 AMERICAN ECONOMIC ASSOCIATION world of certainty M would simply be set at $600/5 or $120. Optimal policy in the uncertain situation confronting the policy-maker is most easily found by consulting equation (5'). If M were set at its average value during the sample period the expected value of Y would be $550, leaving a gap of $20 ($10015). According to (5'), however, only 80 percent of this gap should be closed with a coefficient of variation of a equal to one-half. Hence it is optimal to set M at $116 (=$100+.8X$20). When a and u are not independent, the results are slightly more complicated. The policy-malk.er must now take into account the covariation 9\~~~~~~~P A N~~~~~~~~~P cr \N y FIGUIRE 1 between the impact of his policy action and exogenous variables. If there is a positive correlation, it will pay him to shoot for a lower value of y than otherwise; if negative, higher. One interesting implication of this is that the fraction of the gap that the policy-maker should close will depend on which side of the target he is on. Perhaps more surprising, if a and u have sufficiently large positive correlation and there is a positive gap or sufficiently large negative correlation and there is a negative gap, it may actually pay for the policy-maker to go the "wrong" way! That is, at the initial point it may actually pay to reduce the variance of y at the expense of increasing the difference between expected y and y*. These results can be seen diagrammatically by consulting Figure 1 which shows the expected value of y on the vertical axis and the standard deviation of y on the horizontal axis. Indifference curves, showing various combinations of y and o-r, which have the same expected utility, are drawn "around" y*, the target value of y. These curves have the This content downloaded from 92.202.12.4 on Thu, 02 May 2024 06:25:29 +00:00 All use subject to https://about.jstor.org/terms TOPICS form (y*- IN MONEY y)2+ 417 '-=const around y*. In order to focus on the effects of uncertainty concerning the impact of policy, let us assume for the moment that o, is zero. Referring to Figure 1, suppose that in the absence of policy action, y is certain to be u, which is well below y*. By increasing P by "1 unit," the policy-maker may close the expected gap between y and y by an amount d, but in so doing he also increases the standard deviation of y by ca.5 The line P shows the possibilities open to the policy-maker. Optimal policy corresponds to point A. Because the indifference curves are horizontal where they leave y axis, and vertical at a value of y-y*, while the slope of the line P is d/ora, it always pays to do something, but it never pays to aim for y*. It is also apparent that reductions in OJa for a given a increase the optimal amount of policy. These results are not altered for a. not equal to zero, so long as the correlation between u and a is 0. In Figure 1 the point (ui', a.,) indicates the expected value and variance of y in the absence of policy action and the line drawn through that point labeled P' indicates the opportunities available to the policy-maker. This "opportunity locus" is curved, reflecting the absence of perfect correlation between a and u. This independence guarantees that unless ui = y* it will be optimal to pursue some policy action, for the opportunity locus is vertical at the point (u', -,) indicating that the first little bit of policy can be undertaken without increasing the variance of y.6 In Figure 1 the line P" shows the way correlation between a and u alters the opportunity locus available to the policy-maker. In the example shown, a zero level of policy would leave the economy at the point (u", o-u"). The positive correlation between a and u tilts the locus clockwise through that point so that a small decrease in P will decrease the variance of Y. Optimal policy involves choosing point C, which in this case involves going the "wrong" way. If by chance a(y* j1) - PauOa, the policy-maker should do nothing. Figure 2 shows the way in which the appropriate level of policy action depends on the size of the gap. For given values of d, p, O-a) 0-u2 the larger the gap, the more the policy-maker should do. With independence of a and u this relationship is linear-homogeneous. When a and u are correlated the relationship is linear, but a zero level of policy is optimal for some nonzero gap. I As a convention, the sign of policy is always chosen so that "positive" policy increases y 6 For correlation between u and a equal to 0, de,~ O[(ou + Pto)II] l = _. io O dP dP (0fu + pY fa) /2 P-0 This content downloaded from 92.202.12.4 on Thu, 02 May 2024 06:25:29 +00:00 All use subject to https://about.jstor.org/terms 418 AMERICAN ECONOMIC ASSOCIATION P ~~~~~~~~~~1 + V2 < a~~~~~~~~~~c FIGURE 2 II. Multiple Instruments One of the lessons of the theory of policy under certainty is that, in general, the attainment of n targets requires n instruments. If more than n instruments are available, the policy-maker is free to choose n arbi- trarily in order to achieve his objectives. It should already be obvious that this rule breaks down under uncertainty. We will first show that with one target and two instruments it will generally be optimal to use some combination of both instruments.7 It can similarly be shown that in general all instruments available should be used in pursuing one target. It follows that the addition of an objective requires some sacrifice in performance vis-a-vis objectives already being considered. Suppose that: (6) y = a1P1 + a2P2 + u where again it is desired to maximize the expected value of the utility function given in (2). It will be convenient to define units of policy such that the di associated with each policy instrument is exactly 1. For simplicity let us assume that correlation between each ai and u is 0. Then by differentiating the expected value of (2) with respect to P1 and P2 we find the conditions for optimal policy to be: (7a) 0 = (P1 + P2 + U - y*) + P10-al + Pl20TaiUa2P2 (7b) 0 = (P1 + P2 + u-y*) + P20a2 ?+ P12?TaiTa2Pl where P12 is the correlation between a1 and a2. 7The idea of looking at the problem of optimal policy behavior in an uncertain world as essentially a problem of portfolio choice arose in discussions with Arthur Okun around 1962. This content downloaded from 92.202.12.4 on Thu, 02 May 2024 06:25:29 +00:00 All use subject to https://about.jstor.org/terms TOPICS Solving for IN the MONEY 419 ratio of P1 t 2 (8) P1/(Pl + P2) = 2 P120a1(7a2 ((Ja - 2p12(JaiOa2 + Sal) Equation (8) indicates the proportions in which the policy-maker should use the two policy instruments. The optimal policy portfolio as shown in (8) combines the instruments so as to minimize the coefficient of variation of their combined impact. Under the assumption of inde- pendence between the ai's and the se, this policy portfolio can be treated as a single instrument and its optimal level determined as in Section I. 16.a {K, F2 y FIGURE 3 The coefficient of variation of the optimal policy package is, of course, less than or equal to the coefficient of variation of any single instru- ment. Figure 3 shows, for the special case where a, and p equal zero and where the ratio of the coefficient of variation of a2 to that of a, is.8, the locus for the optimal combination of two policies compared to the loci available for each of the instruments used separately. Figure 3 assumes that the correlation between the impacts of the two instruments is zero. The presence of correlation between the a's complicates the computa- tion of the optimal policy portfolio but does not alter the basic conclusion that several instruments are better than one in the pursuit of one goal. The optimal amount of P1 per "unit" of combined policy action 8 By virtue of the normalization of ai, of course, the standard deviations are coefficien variation. This content downloaded from 92.202.12.4 on Thu, 02 May 2024 06:25:29 +00:00 All use subject to https://about.jstor.org/terms 420 AMERICAN ECONOMIC ASSOCIATION 1 12 2 ('al aa2 2 _1P cya + a i Ca2 caICYa2 a 81~~~~~ ~~~~~~~ 2 1 \ FIGuRE 4 P1+P2 is shown in Figure 4 as a function of the correlation between a, and a2. In the example shown it is assumed that the coefficient of varia- tion of a, is greater than that of a2. From Figure 4 it can be seen that for sufficiently high positive correlation it is optimal to use P1 (the "less efficient" ilistrument) in the "wrong" direction. Table 1 shows the coefficients of variation for the optimal policy TABLE 1 COEFFICIENT OF VARIATION FOR P12 OPTIMAL POLICY PACKAGE -9/10 -3/4 -1/2 -1/4 0 1/4 1/2 3/4 9/10 1 1.0 1.1 1.2 1.6.22.23.24.27.35.37.39.43.50.61.71.79.87.94.97 1.0.52.64.74.83.90.97 1.00 0.54.67.77.86.93.99.99 0.61.74.85.93.99.98.85 0 2.4.31.49.69 0a 2.0.30.47.65.79.89.97 1.00.94.74 0 --.82.92.99 1.00.89.67 0 aa 2.8.33.51.71.85.94.99.99.86.63 0 2 3.2.34.53.73.86.95 1.00.98.83.60 0 4.0.35.55.76.89.97 1.00.96.80.56 0 8.0.39.60.81.93.99.99.92.73.49 0 1.4 2= This content downloaded from 92.202.12.4 on Thu, 02 May 2024 06:25:29 +00:00 All use subject to https://about.jstor.org/terms TOPICS package for IN MONEY various 421 values the variance of a2 normalized at one and with o-a2