Biol 208 Lab 7: Population Dynamics PDF
Document Details
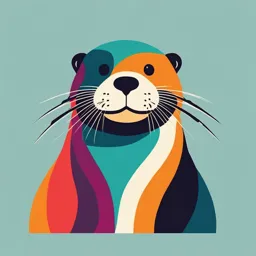
Uploaded by HardWorkingLute
null
Tags
Summary
This document describes a lab for a biology course on population dynamics. The lab covers creating life tables, survivorship curves, and exponential and logistic growth models using modelling and simulations.
Full Transcript
## Lab 7: Population Dynamics using Modelling and Simulations ### Overview - Part 1: Create a static life table and survivorship curve using online cemetery data. - Part 2: Use an online simulator to predict population dynamics in exponential and logistic growth models. - Part 3: Complete the "Lio...
## Lab 7: Population Dynamics using Modelling and Simulations ### Overview - Part 1: Create a static life table and survivorship curve using online cemetery data. - Part 2: Use an online simulator to predict population dynamics in exponential and logistic growth models. - Part 3: Complete the "Lionfish Invasion: Density-Dependent Population Dynamics" Click-and-Learn and discuss the case studies as a group. ### Objectives At the conclusion of this lab, participants will be able to: - Calculate a static life table - Identify and interpret three types of survivorship curves - Identify and interpret growth curves for exponential and logistic growth - Compare the assumptions of the exponential and logistic growth models - Predict how changing model parameters will affect population growth in the exponential and logistic models - Interpret mathematical models and graphs to draw scientific conclusions ### Connections to the lecture material: - What is a population? - How are populations structured? - How do we model populations? - What factors affect population growth? ### Population Ecology A population is a group of individuals from the same species that co-occur in space and time. Population ecology is a stepping-stone between individual ecology (how a single organism interacts with its environment) and community ecology (how multiple different species of organisms interact between themselves and their environments). Population ecology is an important field for resource-based economies, such as forestry or fisheries, where knowledge of the dynamics of that population and the factors that affect it are crucial to the success and protection of the resource. ### Modelling Population Dynamics Scientists use modelling to help explain and predict how complex systems work. Population ecologists use modelling to investigate how changes in the population characteristics affect overall population size. Models are useful not only for scientists, but these models are often used as tools in the "real-world" to predict outcomes and to guide decision making in fields such as resource management and conservation. One of the most simplified mathematic equations for population dynamics is the BIDE equation. This principle is shown in the following equation: $N_{t+1} = N_t + B + I - D - E$ BIDE dynamics state that the size of a population $N_t$ (number of individuals at a time t) will change over time depending on four main factors: the number of individuals born (B), died (D), immigrated (I), and emigrated (E). The number of individuals born and immigrated (arrived into the population) will increase the population size. The number of individuals that died or emigrated (exited the population) will cause a decrease in the population size. If the total number of individuals that were born or immigrated is greater than the number of individuals that died or emigrated, then the population will grow. If the total number of individuals that died or emigrated is greater than the combined number of individuals that were born or immigrated, then the population size will decrease over that time interval. ### Patterns of Survival The BIDE equation shows that the numbers of births and deaths will affect the growth of a population over time. Different species have adopted different reproductive strategies, resulting in different patterns of survival over time. For example, some species (including humans) produce few numbers of offspring, but invest highly in their care, resulting in a high survival rate for young offspring. This results in an overall survivorship curve where the mortality rate is highest at old ages. Other species, including many marine fish, produce a high number of offspring, but invest little in securing their survivability, thus resulting in a high mortality rate in young offspring. Other species, such as many types of birds, have an equal death rate throughout all stages of their life. These three different life strategies can be plotted by graphing the number of survivors on the y-axis and age on the x-axis. This type of graph is called a survivorship curve, and these three life strategies can be represented by differently shaped survivorship curves. ### Cohort and Static Life Tables One way to gather data on patterns of survival in a population is by creating a cohort life table. A cohort is a group of individuals that are born around the same time and move through life within the same period as a "cohort". To create a cohort life table you need to follow each member of this cohort for its entire lifetime and track the age at which each individual dies. Since following a cohort through their entire lifetime can be difficult or in some cases even impossible, there are other options: create an age distribution, or create a static life table. In a static life table, the age at time of death is recorded for a group of individuals that were born at different times. You will use online cemetery data to create a static life tables from two different periods in time and plot their corresponding survivorship curves. You collect the data for age (x) and number of individuals surviving to day x (nx), and then you will calculate the proportion of individuals surviving until day x (lx). ### Exponential and Logistic Models of Population Growth In the second and third parts of this lab, you will use the Population Modelling tools from the HHMI Biolnteractive website. First, you will use the "Population Dynamics" online simulator to explore the exponential and logistic models of population growth. Second, you will used the "Lionfish Invasion: Density-Dependent Population Dynamics" Click-and-Learn to practice using the logistic growth equations and work through the provided case studies. Exponential population growth occurs when a population size is small or when there is little to no competition for resources. There are two equations associated with exponential population growth. The first equation can be used to calculate the rate of population growth (N) under exponential conditions, where *r* is the per capita rate of increase, and *N* is the population size. $\frac{dN}{dt}$ = $rN$ When rearranged to solve for $N_t$, the equation becomes: $N_t = N_0e^{rt}$ Where *Nt* = number of individuals at a given time (t), *No* = initial number of individuals, *r* = per capita rate of increase, *t* = number of time intervals, and *e* = is a constant that represents the base for natural logs. Because there is no limitation on growth, the per capita rate of increase (r) remains constant, and the population size (N) gets successively larger and larger with each time interval. A graph of population size plotted against time for an exponentially growing population will look like \ Figure 7-2 (A). In contrast to exponential growth, logistic population growth occurs when there is an upper limit to population growth, meaning that factors such as resource availability, space, and inter- or intra-specific competition can put a limit on the maximum size of a population. The factors that put an upper limit on growth are called "density dependent" factors because their effect depends on the density of the population. The upper limit of the logistic growth curve is defined by the carrying capacity (K) of the population. K is the theoretical maximum size of the population at a particular location. The "K" is quantified on the graph above (Figure 7-2B) as the population size where the logistic curve flattens out. The equation for logistic population growth is: $\frac{dN}{dt}$ = $r_{max}N(1 - \frac{N}{K})$ Note that the difference for this equation when compared to the equation for exponential growth is the subscript "max" on the per capita rate of increase (r), representing the maximum per capita rate of increase under ideal conditions, and the term $(1 - \frac{N}{K})$, which accounts for limiting resources. In order to solve for population size in logistically growing population, the equation becomes: $N_t = \frac{K}{1 + (K - 1)e^{-r_{max}t}}$ Notice how this equation contains all the same variables as the $N_t = N_0e^{rt}$ equation for exponential growth, except that it also factors in the effect of carrying capacity (K) and $r_{max}$ on population growth. In Part 2 of the lab, you will use the Population Dynamics simulator to compare the two types of population growth. You will then investigate how these models behave and what they can tell you about population growth. Finally, in Part 3 will end the lab with a discussion of the case studies outlined in the "Lionfish Invasion: Density-Dependent Population Dynamics" Click-and-Learn ### Part 1: Static Life Tables and Survivorship Curves using online cemetery demographics Your task is to create a static life table and survivorship curve for a population of humans. 1. There are two sample data sets provided on eClass. Choose one to work on. 2. The data set has already pre-calculated the "Age at death" for the entire data set. This is calculated by subtracting the year of birth from the year of death on the cemetery records. 3. Count the number of individuals whose age at death were within each age range shown in Table 7-1. You can fill in the table manually or on the electronic copy on eClass. 4. Calculate the number of individuals surviving to day x ($n_x$) and put the values in the corresponding column in Table 7-1. To do this, first, sum the total number of all individuals in your population. Next, subtract the number of individuals who died in the first age range from your total and record the number as $n_x$ for that age range. For each successive age group, continue to subtract the number dying in each age class from the previous number remaining alive. 5. Calculate the probability of surviving to the start of a given age class ($l_x$) by dividing $n_x$ by the total number of people in the population. Place those values in the column labelled $l_x$. By normalizing your data into proportions ($l_x$) you can compare between populations that had different numbers of individuals. 6. Draw a survivorship curve for each group. This is done by plotting the $l_x$ over time. The y-axis ($l_x$) needs to be expressed on a logarithmic scale. The excel sheet on eClass is set up to do this for you automatically.