Backchannel Behavior Is Idiosyncratic PDF
Document Details
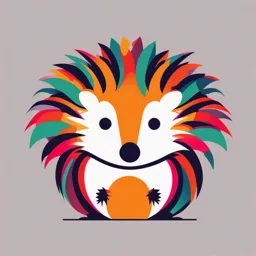
Uploaded by HonorableAnecdote
ZHAW - Zürcher Hochschule für Angewandte Wissenschaften
Peter Blomsma, Julija Vaitonyté, Gabriel Skantze, Marc Swerts
Tags
Summary
This article investigates the variability in feedback behavior of 14 participants during spoken interactions. The study, conducted with a controlled paradigm, reveals that backchannel behaviors vary between listeners. The research examines the role of backchannels in human communication.
Full Transcript
Language and Cognition (2024), 1–24 doi:10.1017/langcog.2024.1 ARTICLE Backchannel behavior is idiosyncratic Peter Blomsma1, Julija Vaitonyté1, Gabriel Skantze2 and Marc Swerts1 1 Tilburg University, Tilburg, The Netherlands...
Language and Cognition (2024), 1–24 doi:10.1017/langcog.2024.1 ARTICLE Backchannel behavior is idiosyncratic Peter Blomsma1, Julija Vaitonyté1, Gabriel Skantze2 and Marc Swerts1 1 Tilburg University, Tilburg, The Netherlands 2 KTH Royal Institute of Technology, Stockholm, Sweden Corresponding author: Peter Blomsma; Email: [email protected] (Received 04 November 2022; Revised 31 October 2023; Accepted 04 January 2024) Abstract In spoken conversations, speakers and their addressees constantly seek and provide different forms of audiovisual feedback, also known as backchannels, which include nodding, vocaliza- tions and facial expressions. It has previously been shown that addressees backchannel at specific points during an interaction, namely after a speaker provided a cue to elicit feedback from the addressee. However, addressees may differ in the frequency and type of feedback that they provide, and likewise, speakers may vary the type of cues they generate to signal the backchannel opportunity points (BOPs). Research on the extent to which backchanneling is idiosyncratic is scant. In this article, we quantify and analyze the variability in feedback behavior of 14 addressees who all interacted with the same speaker stimulus. We conducted this research by means of a previously developed experimental paradigm that generates spontaneous interactions in a controlled manner. Our results show that (1) backchanneling behavior varies between listeners (some addressees are more active than others) and (2) backchanneling behavior varies between BOPs (some points trigger more responses than others). We discuss the relevance of these results for models of human–human and human–machine interactions. Keywords: backchannels; consensus sampling; head nod; listener feedback; multimodal; O-Cam paradigm 1. Introduction A spoken conversation can be operationalized as a highly interactive form of cooperative activity between at least two individuals. In that sense, it is more than an exact data transfer process, whereby a sender simply transmits information to a receiver, who then decodes the incoming message. The latter characterization of a spoken interaction does not do justice to the observation that an addressee is often more than a passive listener and is, in fact, co-responsible for a successful exchange of information (Clark, 1996). Indeed, communication via speech can sometimes be a fuzzy endeavor, for example, because of a noisy channel or the fact that a speaker may not correctly estimate a listener’s prior knowledge about a specific state of affairs. As a result, it is typically the case that speakers and addressees seek and provide feedback © The Author(s), 2024. Published by Cambridge University Press. This is an Open Access article, distributed under the terms of the Creative Commons Attribution licence (http://creativecommons.org/licenses/by/4.0), which permits unrestricted re-use, distribution and reproduction, provided the original article is properly cited. https://doi.org/10.1017/langcog.2024.1 Published online by Cambridge University Press 2 Peter Blomsma et al. on the smoothness of the interaction, to check whether information has successfully arrived at the other end of the communication chain. Accordingly, there is a growing interest in current models of spoken interaction regarding the systematicity of various types of feedback behavior. In this article, we are specifically interested in the brief responses, called back- channels (Yngve, 1970), that addressees return during an interaction. Such back- channels, which can be verbal and non-verbal, serve as cues to show a speaker that an addressee is engaged and listening. Backchannels thus convey attention and interest to the speaker, and they can also regulate turn-taking (Gravano & Hirschberg, 2011). While verbal backchannels include vocalizations (laugh, sigh, etc.), paraverbals (‘mm-hmm’, ‘uh-huh’, etc.) and short utterances (‘really’, ‘yeah’, ‘okay’), non-verbal backchannels consist of facial expressions, nodding, eye gaze and gestures. It has been shown that there is a marked difference between signals that serve as ‘go-on’ cues, that is, to make clear that the addressee has correctly processed the incoming message, and signals that highlight a possible communication problem so that a speaker–sender may have to repair a potential error (Granström et al., 2002; Krahmer et al., 2002; Shimojima et al., 2002). In the literature, backchannels are distinguished from turn-taking cues. The intention of a speaker, when backchanneling, is to signal that the current speaker is still in charge of the turn, while the intention of a turn-taking cue is to interrupt the speaker and to take the speaking turn. Thus, backchannels can be viewed as a form of cooperative overlap or, from a turn-taking perspective, as a turn-yielding cue (Bertrand et al., 2007). 1.1. Backchannel-inviting cues It has been shown that the timing of backchannels is crucial to guarantee a smooth interaction (Gratch et al., 2006; Poppe et al., 2011). For instance, Gratch et al. (2006) demonstrated that a wrongly timed head nod from a listener can disrupt a speaker, which suggests that addressees typically are efficient at producing backchannels at the right points in an interaction. Indeed, research shows that backchannels occur at specific points in a conversation, for example, after the speaker gives a so-called backchannel-inviting cue (Gravano & Hirschberg, 2011), also called backchannel- preceding cues (Levitan et al., 2011). The specific behaviors that the speaker produces to transmit backchannel-inviting cues to elicit backchannel behavior from an addressee come in different forms, including the usage of specific prosodic patterns. Gravano and Hirschberg (2009) found that speakers use rising and falling intonations to elicit feedback. Similarly, Cathcart et al. (2003) and Ward and Tsukahara (2000) showed that listeners often provide a backchannel after speakers have lowered their pitch for at least 110 ms, and Cathcart et al. (2003) showed that pauses in the speaker’s speech and also certain parts of speech are predictive of backchannels. Furthermore, Duncan (1972) observed that backchannels occur after syntactically complete sentences, while Bavelas et al. (2002) revealed that mutual gaze often occurs prior to a backchannel being produced. In line with this, Hjalmarsson and Oertel (2012) found that listeners were more likely to identify a backchannel-inviting cue when the speaker (an embodied conversational agent (ECA) in this case) made direct eye contact with the camera, as opposed to gazing away. https://doi.org/10.1017/langcog.2024.1 Published online by Cambridge University Press Language and Cognition 3 The probability that a listener will backchannel after a cue will increase when backchannel-inviting cues are stapled to form more complex signals (Gravano & Hirschberg, 2011). In a similar vein, Hjalmarsson (2011) showed that it appears to be the case with turn-taking and turn-yielding signals (signals closely related to backchannel-inviting cues, yet distinct) that the more cues are used to comprise the signal, the faster the reaction time of the interlocutor becomes. Speakers may not be aware of sending out backchannel-inviting cues, but listeners and observers are capable of picking up on those signals. Bavelas et al. (2000) showed that listeners are even able to provide backchannels at the right moment when not attending to the content of the speech. 1.2. Backchannel opportunity points Although speakers provide backchannel-inviting cues, it is up to the addressee to pick up on these cues and identify relevant moments in a conversation to produce backchannels. Those moments in a conversation, where it is appropriate for an addressee to provide some kind of listener feedback, are referred to as backchannel opportunity points (BOPs) (Gratch et al., 2006). BOPs, which are also known as jump-in points (Morency et al., 2008) and response opportunities (de Kok, 2013), are points in the interaction where an addressee could or would want to provide feedback in reaction to the speaker (de Kok & Heylen, 2010). Prior studies show that not all BOPs are used by addressees to provide a backchannel (Kawahara et al., 2016; Poppe et al., 2011). However, we lack detailed insight into the extent to which there is variability in the way addressees return feedback and regarding the different types of BOPs. 1.3. Current work The goal of this study is to shed light on the variation that exists in backchannel behaviors across addressees and within an individual addressee. Specifically, we ask the following: (1) What types of behaviors are utilized by addressees to give feedback during BOPs? (2) How does feedback behavior differ across different addressees? (3) To what extent differs the behavior of addressees for the same BOP? The fact that we expect there to be variability between and within addressees in their feedback behavior is in line with the previous findings that human beings do not have a fixed communication style. Speakers have been shown to adapt their way of speaking depending on the situational context, such as the type of addressee or the specific environment. Typically, speakers talk differently to children or adults and switch to a different style when they notice that their partner experiences some problems of understanding (e.g., because that person is not a native speaker) (Bortfeld & Brennan, 1997). Along the same lines, there may be differences across addressees, for example, depending on personality traits or the mere fact that some addressees have more developed communicative skills (Williams et al., 2021). It could be expected that addressees may vary in how they produce backchannel behaviors, with some spots in the interaction eliciting stronger or more backchannels than others (e.g., because such a cue is felt to be more needed). Also, some addressees may be more extraverted or engaged so that one could expect differences across addressees as well. https://doi.org/10.1017/langcog.2024.1 Published online by Cambridge University Press 4 Peter Blomsma et al. Furthermore, the characteristics of a BOP can influence the type of behavior it elicits. A BOP placed at the end of a complete syntactically complete phrase is more likely to be seized than a BOP at the end of a syntactically incomplete phrase (Skantze et al., 2013). The dynamics of the interaction could also play a role. Benus et al. (2007) show that the liveliness of an interaction may influence the type of verbal backchannels a participant uses. In their study, mm-hm and uh-huh were more used during lively interactions, while okay and yeah were used more during less animated interactions. Orthogonal to this, the reason why not every BOP is seized could also be due to idiosyncratic differences between listeners. Huang and Gratch (2012) examined the personalities of backchannel coders and explored the connection between these personalities and the frequency of identified BOPs. The results revealed a positive association between a higher number of identified BOPs and elevated levels of agreeableness, conscientiousness and openness. This is in line with the results of an earlier study that showed that different types of backchannel behavior correlate with various impressions of people’s specific personalities (Blomsma et al., 2022). Insight into the variability of audiovisual backchannel behavior is not only informative to understand how human–human communication proceeds, but it is also relevant for practical applications, such as models of human–computer inter- action, specifically social robots and ECAs (Cassell et al., 2000), also known as socially interactive agents (SIAs) (Lugrin et al., 2021). In a similar manner to human–human interaction, it could be useful for ECAs to vary in the extent to which they back- channel, for example, depending on the type of user, context and application. It is also likely that inducing variability may render the interaction style of an ECA more natural and less monotonous, similar to the efforts to synthesize variability in speech and language generation systems (Gatt & Krahmer, 2018). However, modeling natural backchannel behavior for artificial entities is a non-trivial task for at least two reasons. One of the difficulties lies in detecting and appropriately responding to backchannel-inviting cues. Another difficulty is that due to backchannel behavior being idiosyncratic, it is not easy to define what a typical backchannel behavior should consist of for an ECA. To investigate variation in backchannel behaviors and to answer the research questions above, we conducted a computational study based on the data collected in a human experiment that used the so-called O-Cam paradigm (Goodacre & Zadro, 2010). The current study is the first one in which the paradigm is used to examine backchannel behavior. The O-Cam paradigm was set up to allow comparisons between multiple addressees who are exposed to identical conversational data from the same speaker stimulus. The computational study consisted of two analyses. Analysis I examines the speaker stimulus, specifically the identification of BOPs, the categorization of those BOPs and the prosodic properties of the backchannel- inviting cues preceding the BOPs. Analysis II investigates the addressee’s behavior during the BOPs. We compared the behavior of the addressees across multiple channels (i.e., facial expressions, head movement and vocalizations) to examine the degree of variability between and within addressees. 2. Dataset This study employed the materials of a database previously recorded during an experiment conducted by Brugel (2014). The database consisted of (1) one video https://doi.org/10.1017/langcog.2024.1 Published online by Cambridge University Press Language and Cognition 5 recording of the stimulus, henceforth ‘speaker’, and (2) the video recordings of 14 participants who were filmed during the experiment, henceforth ‘addressees’. Each video was 8.42 minutes long and contained 6.25 minutes of conversation, and the remaining time was used for game-related tasks such as preparing and answering questions (see explanation below). The number of participants is comparable to similar backchannel studies, including Krogsager et al. (2014) and Poppe et al. (2010). The recorded experiment was based on the O-Cam paradigm (Goodacre & Zadro, 2010), an experimental design that combines the advantages of online paradigms (i.e., highly controllable environment, easy to run) with the advantages of offline settings (i.e., high ecological validity). The core concept of the O-Cam paradigm is that a participant thinks that he/she is having a computer-mediated conversation with another participant (i.e., an interaction via a video conferencing setting), while, in reality, the other participant is a confederate whose video is pre-recorded. Certain manipulations are used in the setup to make a participant think it is a real-life conversation (Goodacre & Zadro, 2010). The O-Cam paradigm has been previously utilized to, for example, study the relationship between gender and leadership capabilities (Hong et al., 2014) and investigate the influence of smiling behavior (Mui et al., 2018). The experiment reported by Brugel (2014) was aimed to elicit feedback behavior from the participants. Each addressee played a Tangram game with the speaker (who was a pre-recorded confederate) via computer-mediated connection. During the experiment, the addressee was presented with four Tangram figures for 5 seconds, followed by a description of one of those Tangrams provided by the speaker. The participant’s task was to choose the figure from the four Tangram figures based on the description by the speaker. See Figure 1 for a visual illustration of the experiment. The experiment consisted of 11 rounds in which each time a different quadruple of Tangram figures would be used. The participants were told that the experiment was related to abstract thinking and that they were not allowed to ask questions since Figure 1. Visual impression of the o-cam experiment. First, the participant is prepared (A–C); after that, 11 rounds are played: In each round, the participant is shown four figures (D), followed by a description of one of those figures (E) after which the participant indicates which figure is described (F). https://doi.org/10.1017/langcog.2024.1 Published online by Cambridge University Press 6 Peter Blomsma et al. asking questions would make the game too simple. The confederate (the speaker) was not informed about the goal of the study in order to keep the experiment as ecologically valid as possible. Although task success was not measured, the primary objective of the game was to create a challenging experience with a task success rate close to 100%. This was intended to ensure that participants would fully concentrate on the speaker without feeling the need to ask additional questions for clarification, which would have been disruptive to the experimental setting, as the participant would then notice that the recorded confederate would not be responding to his/her questions. After the experiment, participants were asked whether they suspected that instead of a live interaction they were presented with a pre-recorded video of another person. The data of five participants were discarded because they answered positively, whereas one participant asked a question during the experiment, and thus, their data were also discarded. 3. Analysis I: speaker’s behavior The first analysis regards only the speaker’s behavior to identify the BOPs and to analyze the audiovisual behavior of the speaker during the backchannel-inviting cues preceding the BOPs. The identified BOPs are subsequently used in Analysis II to investigate the addressee’s feedback behavior. An obvious approach to identify the BOPs would be to annotate the backchannel behavior for each of the addressee videos separately. However, such an approach comes with at least two disadvantages. As addressees do not necessarily utilize all BOPs to provide feedback, analyzing the addressees would thus not necessarily result in the identification of all BOPs. Furthermore, using the same data for selection and selective analysis would result in a circular analysis also known as ‘double dipping’ (Kriegeskorte et al., 2009). Therefore, we identified the BOPs based on the speaker stimulus. 3.1. Methods 3.1.1. BOP identification We used parasocial consensus sampling (Heldner et al., 2013; Huang et al., 2010), which takes the advantage of the fact that humans, especially as a third-party observer, can aptly point out BOPs in a conversation (de Kok, 2013). The approach consisted of two steps: identification of possible BOPs by a jury of multiple judges, followed by the aggregation of the output of the jury to determine genuine BOPs. Genuine BOPs are those BOPs that are identified by at least a certain percentage of judges. For the identification of BOPs, we used a human jury that consisted of 10 judges. Each judge watched the speaker video and identified each moment that he/she thought was appropriate to backchannel. Each judge was instructed in the same way. First, they were explained what backchanneling behavior is; namely, the listening signals one gives during a conversation include head nods and sounds like ‘uh-uh’, ‘hmm’ and ‘hm-hm’ and combinations of nods and sounds. Next, they were asked to watch the speaker video and to make a sound (e.g., ‘yes’) when he/she thought it was appropriate to backchannel, either verbally, non-verbally or both. The audio of the judge was recorded. https://doi.org/10.1017/langcog.2024.1 Published online by Cambridge University Press Language and Cognition 7 The aggregation of all the recordings of judges allowed us to determine, for each data point in the stimulus, the percentage of judges that thought that a specific moment was a BOP. BOPs that were agreed upon by a minimum percentage of judges were classified as genuine BOPs and selected for further analysis. The minimum percentage is based on the expected number of backchannels in the recording. Poppe et al. (2011) state that one could expect from 6 to 12 back- channels per minute. Since our recording was 6.25 minutes, we therefore expected between 38 and 77 backchannels. The appropriate consensus level is determined as follows. First, the number of BOPs is calculated for each potential consensus level. That is, the number of BOPs that would be marked as genuine BOPs if that consensus level were used. Next, the final consensus level is selected based on the resulting BOP count. In this case, the BOP count should fall within the range of 38 and 77. In general, the relationship between consensus levels and number of BOPs could be seen as a monotonic non-increasing function: When the consensus level increases, the number of genuine BOPs either increases or stays constant; it never decreases. All the recordings of judges were preprocessed with audacity (Audacity Team, 2021): We used a noise gate filter (250 ms attack and 12.50 dB grate threshold) to remove background noise and a 20 dB audio amplification to ensure that a judge was audible. Each recording was then converted to a binary time series with a resolution of 25 frames per second (FPS), in such a way that frames that contained a sound with an amplitude above 0.1 were converted to 1 and, otherwise, to 0. Although Huang et al. (2010) used a resolution of 10 FPS, we decided to use 25 FPS as this matched with the FPS of both our video recording and the FaceReader encodings (as described in the subsequent section). Because judges had to vocally indicate visual backchannels, which start on average 202 ms before a vocal backchannel (Wlodarczak et al., 2012), the onset of each indication was set to 202 ms before the actual onset in order to correct for a potential delay. Each onset of a judge’s indication was converted to a potential BOP of the length of 1000 ms in line with Huang et al. (2010). Finally, a time series was created with a resolution of 25 FPS, where each frame (i.e., sample) contained the number of judges that indicated a BOP for that frame. 3.1.2. BOP types: continuer and end-of-turn To gain further insight into whether specific BOPs or BOP types affect the average addressee’s behavior, we subdivided the BOPs into two categories. Although each BOP functions as a moment for the addressee to acknowledge certain information, we conjecture that the urge to acknowledge is the strongest at the end of each game round. After all, no further information will follow the last BOP of a game round, and thus, the addressee should have enough information to answer the question at that point. And if not, the addressee should indicate that at that last BOP. Therefore, we estimate that the most expressive addressee’s behaviors will be observable at the last BOP of a round. Hence, we have created the following categories: (1) All BOPs that are the last of a round, we called this category the last backchannel of round (LBR), and (2) all other BOPs that are placed during a round, we called this category continuer. Given this categorization, the LBR category contained 11 cues and the continuer category contained 42 cues. https://doi.org/10.1017/langcog.2024.1 Published online by Cambridge University Press 8 Peter Blomsma et al. 3.1.3. Backchannel-inviting cues To verify that indeed the (visual) prosody is different for backchannel-inviting cues compared to the prosody used during the remaining part of the conversation, we analyzed the pitch properties, facial behavior and head movement of the speaker’s backchannel-inviting cues that preceded the identified BOPs. The cues were isolated by selecting the last 1000 ms of the speaker stimulus sound before the start of each BOP. However, there is no consensus on the length of such samples in literature; for example, Skantze (2012) analyzed the last 200 ms of the voiced region for pitch, while Levitan et al. (2011) reported longer sample lengths including 1000 ms. We choose 1000 ms to be on the safe side of finding a voiced part in the sample. The pitch properties were extracted with Praat (Boersma & Weenink, 2022). Of each sample, the F0 values (i.e., the fundamental pitch values) were extracted with a precision of 100 FPS. Trailing and leading frames that did not contain pitch informa- tion were discarded. For each sample, the average, minimum, maximum, amplitude (which is the maximum minus the minimum), average and form were obtained. The form was calculated by subtracting the average pitch of the second half of the sample from the average pitch of the first half of the sample, such that a negative number for form means an increasing pitch and a positive number means a decreasing pitch. The facial behavior and head movements were analyzed based on the output of FaceReader 8 software (Noldus, 2019). The stimulus video was encoded with action units (AUs) based on the Facial Action Coding System (Ekman & Friesen, 1978). Every frame of the videos was encoded with the following AUs: 1, 2, 4, 5, 6, 7, 9, 10, 12, 14, 15, 17, 18, 20, 23, 24, 25, 26, 27 and 45, and X, Y and Z coordinates were extracted for head orientation. Each AU can be scored for intensity on an ordinal scale from 0 (i.e., absence of an AU) to 5 (i.e., maximum intensity). For some frames in the dataset, FaceReader was unable to detect a face and thus was also unable to encode head position and/or AU activations. Head nods were quantified for all backchannel-inviting cues following Otsuka and Tsumori (2020). Specifically, for head nods, we extracted amplitude and frequency. Amplitude equals the maximum tilt angle, that is, the difference between the minimum and maximum X rotation angles. Frequency is the sum of upward and downward peaks per second of the X rotation angle. To prevent that small noise-related changes in elevation direction would influence the frequency, we ignored upward and downward peaks that differed a maximum of 1 degree. In order to verify whether the backchannel- inviting cues differed from non-backchannel-inviting cues, each backchannel-inviting cue was paired with a randomly selected voice sample from the speaker stimulus. Paired t-tests were conducted between the obtained pitch properties, head movements and the average AU activation of the backchannel-inviting cues and the non-backchannel- inviting cues. The Bonferroni correction was applied for the multiple pairwise com- parisons. Subsequently, the analyzed properties of the backchannel-inviting cues of the LBR category were compared with those of the continuer category. The two categories were compared with Welch’s t-test for significance and also corrected with Bonferroni. 3.2. Results 3.2.1. BOP identification The number of identified backchannels per response level is depicted in Figure 2. Genuine (i.e., definite) BOPs were based on a consensus level of 30% (three coders) https://doi.org/10.1017/langcog.2024.1 Published online by Cambridge University Press Language and Cognition 9 Figure 2. Illustration of a part of the speaker stimulus, with at each point in time the number of judges that indicated the presence of a BOP. If three judges or more indicated a BOP at a certain point, then this point is considered as a genuine BOP. such that 53 BOPs were taken into account. The average duration of the 53 genuine BOPs was 934 ms (SD = 403 ms). The duration of a BOP was calculated starting from the initial timepoint with a consensus level of at least 30% and ending at the last timepoint where the consensus level was at least 30%. 3.2.2. Backchannel-inviting cues The backchannel-inviting cues had a higher maximum pitch and a larger F0 range, compared to the randomly selected samples. There were no significant differences for average pitch, minimum and form. The highest pitch observed in backchannel- inviting cues was on average 350.36 Hz (SD = 106.94 Hz), while the highest pitch in the random samples had a lower average of 201.30 Hz (SD = 70.88 Hz). The F0 range for backchannel-inviting cues was on average 156.07 Hz (SD = 111.84 Hz), while the random samples had a lower average F0 range of 102.34 Hz (SD = 71.77 Hz). See Table 1 for all the results. The speaker’s head movements and facial behavior did not differ significantly between cues and non-cues and also not between LBR and continuer-related inviting cues (see Tables 2 and 3). For all comparisons, the Bonferroni correction was applied. The backchannel-inviting cues that preceded BOPs from the LBR category had a significantly lower average pitch, as compared to the cues that preceded the continuer Table 1. Pitch properties of backchannel-inviting cues, compared to those of non-cues Cue (1) Non-cue (2) Diff (1) (2) df Cohen’s d p-value Average 246.95 (37.23) 250.72 (40.63) 3.77 48 0.10.740 Min 194.29 (43.44) 303.64 (54.23) 109.35 48 0.14.443 Max 350.36 (106.94) 201.30 (70.88) 149.06* 48 0.51.006 Amplitude 156.07 (111.84) 102.34 (71.77) 53.73* 48 0.57.006 Form 16.10 (52.87) 16.66 (49.74) 32.76 48 0.45.057 Note: Statistics are based on paired t-test analysis. All values are in Hertz. The Diff score is the result of subtracting the mean cue value from the mean random value. The Bonferroni correction was applied for the multiple pairwise comparisons with an alpha level of 0.01 (0.05/5 = 0.01). *p