Advanced Statistical Analysis Lecture Notes
Document Details
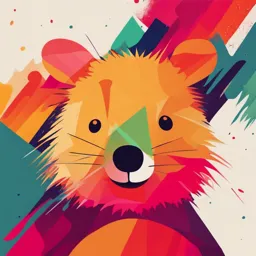
Uploaded by ClearerKoala
University of Groningen
2023
Mark van Duijn
Tags
Related
Summary
These lecture notes from the University of Groningen cover advanced statistical analysis, specifically focusing on linear regression models, transformations of variables, how to handle multicollinearity issues, and interpreting and building models with considerations for data quality and ethical use.
Full Transcript
Advanced Statistical Analysis Week 2 Lecture 4 Dr. Mark van Duijn Department of Economic Geography Department of DemographyUniversity of Groningen [email protected] 16 Feb, 2023 Introduction Interpretation Functional form Collinearity issues Conclusions Last lecture OLS properties and assumptio...
Advanced Statistical Analysis Week 2 Lecture 4 Dr. Mark van Duijn Department of Economic Geography Department of DemographyUniversity of Groningen [email protected] 16 Feb, 2023 Introduction Interpretation Functional form Collinearity issues Conclusions Last lecture OLS properties and assumptions Interpretation and model fit Data transformation Data problems: Measurement error, omitted variables and missing values Schafer & Graham (2002) - Missing Data: Our View of the State of the Art Mehmetoglu & Jakobsen (2017) - Chapter 7 Dr. Mark van Duijn (RUG) Advanced Statistical Analysis 16 Feb 20232 / 28 Introduction Interpretation Functional form Collinearity issues Conclusions Agenda Part I: Interpretation Part II: Functional form Part III: (Multi)collinearity Part IV: Room for Q&A Finalizing OLS Mehmetoglu & Jakobsen (2017) - Chapter 7,15.3-15.6 Dr. Mark van Duijn (RUG) Advanced Statistical Analysis 16 Feb 20233 / 28 Introduction Interpretation Functional form Collinearity issues Conclusions Intercept and slope If x increases by 1, y increases with . . . Dr. Mark van Duijn (RUG) Advanced Statistical Analysis 16 Feb 20234 / 28 Introduction Interpretation Functional form Collinearity issues Conclusions Transformations Continuous, categorical, binary, . . . Independent variable Dependent variable(income) Continuous: X can be any number (age) Categorical: C=1 or 2, or 3, or 4, etc. (high school, college-degree, university-degree, doctor-degree) Binary/Dummy: D=0 or D=1 (male or female) Which type of variable is easiest to transform? And do you know why? Remember, that data transformation is notunethical data manipulation! Dr. Mark van Duijn (RUG) Advanced Statistical Analysis 16 Feb 20235 / 28 Introduction Interpretation Functional form Collinearity issues Conclusions Different types of variables Continuous, categorical, binary, . . . Independent variable Dependent variable (income) Continuous: X can be any number (age) Categorical: C=0 or 1, or 2, or 3, or 4, etc. (none=0, high school=1, college-degree=2, university-degree=3, doctor-degree=4) Binary/Dummy: D=0 or D=1 (male or female) inc i= b 0 + b 1x 1,i + b 2C i+ b 3D i+ e i If C increases by 1, y increases with . . . If D=1=female, y increases with . . . compared to D=0=male Dr. Mark van Duijn (RUG) Advanced Statistical Analysis 16 Feb 20236 / 28 Introduction Interpretation Functional form Collinearity issues Conclusions Dummy variables If D=1=female, y increases with . . . compared to D=0=male Or, the effect of being a women compared to being a man is . . . Dr. Mark van Duijn (RUG) Advanced Statistical Analysis 16 Feb 20237 / 28 Introduction Interpretation Functional form Collinearity issues Conclusions Transformation + Interpretation What changes if we take the natural logarithm of income? Continuous: X can be any number (age) Categorical: C=0 or 1, or 2, or 3, or 4, etc. (none=0, high school=1, college-degree=2, university-degree=3, doctor-degree=4) Binary/Dummy: D=0 or D=1 (male or female) ln (inc i) = b 0 + b 1x 1,i + b 2C i+ b 3D i+ e i If C increases by 1, y increases with . . . If D=1=female, y increases with . . . compared to D=0=male Dr. Mark van Duijn (RUG) Advanced Statistical Analysis 16 Feb 20238 / 28 Introduction Interpretation Functional form Collinearity issues Conclusions Example of a ln-transformation Dr. Mark van Duijn (RUG) Advanced Statistical Analysis 16 Feb 20239 / 28 Introduction Interpretation Functional form Collinearity issues Conclusions Transformations and interpretation Linear: y= b 0 + b 1x + . . . +e b 1 : level-effect +1 x increases y with b 1 Log-linear: ln(y ) = b 0 + b 1x + . . . +e b 1 : growth rate +1 x increases y with exp( b 1) times or +1 x increases y with (( exp( b 1) − 1) ∗100) % Log-log: ln(y ) = b 0 + b 1ln (x ) + . . .+e b 1 : elasticity +1% in x, increases y with ( b 1)% Assuming that x is a continuous variable! Dr. Mark van Duijn (RUG) Advanced Statistical Analysis 16 Feb 202310 / 28 Introduction Interpretation Functional form Collinearity issues Conclusions Functional form Dr. Mark van Duijn (RUG) Advanced Statistical Analysis 16 Feb 202311 / 28 Introduction Interpretation Functional form Collinearity issues Conclusions Functional form Functional form refers to the form of a relationship between a dependent variable and regressors So, what to do when we do not observe a linear relationship between y and x? Dr. Mark van Duijn (RUG) Advanced Statistical Analysis 16 Feb 202312 / 28 Introduction Interpretation Functional form Collinearity issues Conclusions Last example How to get from line 1 to line 2? What kind of transformation is needed? Dr. Mark van Duijn (RUG) Advanced Statistical Analysis 16 Feb 202313 / 28 Introduction Interpretation Functional form Collinearity issues Conclusions Last example Polynomials y = b 0 + b 1x + b 2x 2 + e If we plot this formula it looks much like LINE 2 First derivative gives us the interpretation (if x increases with 1, y increases with . . . ) ∂ y ∂ x = . . . ∂ y ∂ x = b 1 + b 22 x Note: Slope is a linear line increasing in x Dr. Mark van Duijn (RUG) Advanced Statistical Analysis 16 Feb 202314 / 28 Introduction Interpretation Functional form Collinearity issues Conclusions Last example Polynomials y = b 0 + b 1x + b 2x 2 + e If we plot this formula it looks much like LINE 2 First derivative gives us the interpretation (if x increases with 1, y increases with . . . ) ∂ y ∂ x = . . . ∂ y ∂ x = b 1 + b 22 x Note: Slope is a linear line increasing in x Dr. Mark van Duijn (RUG) Advanced Statistical Analysis 16 Feb 202314 / 28 Introduction Interpretation Functional form Collinearity issues Conclusions Remember: Multivariate analysis y= b 0 + b 1x 1 + b 2x 2 + ... +ϵ (1)Corrects for associations between two (or more) independent variables Coefficients measures the effect of each independent variable in the presence of other independent variables Example: Income and level of education affect the transition to home-ownership But what if the associations are too strong? Example: Length and weight of babies Dr. Mark van Duijn (RUG) Advanced Statistical Analysis 16 Feb 202315 / 28 Introduction Interpretation Functional form Collinearity issues Conclusions What is (multi)collinearity Strong association between two (or more) variables: one variable (almost) perfectly predicts the other Example of perfect multicollinearity: Suppose: y= β 0 + β 1x 1 + β 2x 2 + ϵwith x 1 = 2 x 2 + 4 Problem: No unique solution to the least squares minimization problem In other words: β 1 measures the effect of x 1 on y, holding x 2 constant. However, . . . Variables ’take over’ each others’ effects: unclear what causes what High correlation (above 0.9? or 0.8??) Dr. Mark van Duijn (RUG) Advanced Statistical Analysis 16 Feb 202316 / 28 Introduction Interpretation Functional form Collinearity issues Conclusions Detect (multi)collinearity Rule of thumb: Sample correlation >0.8 is evidence of severe collinearity However, if the collinear relationship involves more than 2 independent variables, you may not detect it using simple correlations Look at Variance Inflation Factors (VIFs): 1 Regress each independent variable, ( x k ), on all the other independent variables 2 Collect the R squared of each of the regressions: ( R2 k ) 3 Compute the VIF: VIF(x k ) = 1 (1 −R2 j ) 4 Rule of thumb: VIF(x k ) > 5 is evidence of severe multicollinearity Dr. Mark van Duijn (RUG) Advanced Statistical Analysis 16 Feb 202317 / 28 Introduction Interpretation Functional form Collinearity issues Conclusions Solution for perfect (multi)collinearity Delete one of the (irrelevant) variables from the model For categorical variables: leave out one whole variable OR a category; rearrange variables or categories Usually, this is not very difficult Dr. Mark van Duijn (RUG) Advanced Statistical Analysis 16 Feb 202318 / 28 Introduction Interpretation Functional form Collinearity issues Conclusions Solution for imperfect (multi)collinearity Usually, this is more difficult Do nothing: face the consequences Delete one of the (irrelevant) variables from the model For categorical variables: leave out one whole variable OR a category; rearrange variables or categories Transform one of the variables - only if it is consistent with theory and common sense Principal component analysis Dr. Mark van Duijn (RUG) Advanced Statistical Analysis 16 Feb 202319 / 28 Introduction Interpretation Functional form Collinearity issues Conclusions Example Dependent variable: realising the wish to become a homeowner (categories: did not move, moved to owner-occupied market, moved to rental market) Theoretical reasons to expect an effect of: Absolute local house price Ratio of average local house price to average local rent Correlation matrix: Both variables are collinear (apparently too little regional variation in average rents) Solution? Dr. Mark van Duijn (RUG) Advanced Statistical Analysis 16 Feb 202320 / 28 Introduction Interpretation Functional form Collinearity issues Conclusions Choice that was made in the paper Present the model with price-to-rent ratio only (theoretical reason) Discuss absolute local house prices in the text and report the existence of collinearity Dr. Mark van Duijn (RUG) Advanced Statistical Analysis 16 Feb 202321 / 28 Introduction Interpretation Functional form Collinearity issues Conclusions Last example: xand x2 Very strong association. . . but no perfect collinearity Neat way of relaxing the assumption of linear effect Compute in STATA: gen agesq = age*age Include age and agesq as independent variables (remember our discussion on polynomials in week 1) Remember how to interpret the coefficients (increase of one unit in X . . . ) Dr. Mark van Duijn (RUG) Advanced Statistical Analysis 16 Feb 202322 / 28 Introduction Interpretation Functional form Collinearity issues Conclusions Other example Dr. Mark van Duijn (RUG) Advanced Statistical Analysis 16 Feb 202323 / 28 Introduction Interpretation Functional form Collinearity issues Conclusions General lessons: Always. . . Explore your data carefully Look at your data Run frequencies, crosstabs, correlations, . . . Build your models carefully Start with few variables, then add more and see what changes in the results Only add variables that make sense! Preferably, derive them from theory and/or literature It is never a bad thing to run 20+ regressions and see how robust your results are to small changes (adding/removing variables, adding/removing groups, . . . ) Dr. Mark van Duijn (RUG) Advanced Statistical Analysis 16 Feb 202324 / 28 Introduction Interpretation Functional form Collinearity issues Conclusions Data management Where to save the data? Open source vs. Non-disclosure agreements What is a ’safe’ environment? When to delete data? How to deal with data in research? Data quality + ethics: What am I allowed to do with the raw data obtained? Report on data management: How do you go from raw data to data used for analysis (in main text + details in the Appendix) Syntax files: Transparancy + reproducability of analyses (add to the Appendix) Dr. Mark van Duijn (RUG) Advanced Statistical Analysis 16 Feb 202325 / 28 Introduction Interpretation Functional form Collinearity issues Conclusions Any remaining questions about OLS? Any remaining questions about OLS? Estimation technique? Tranforming data vs. data manipulation? Ethical considerations? Model building? Interpretation coefficients (of various types of independent (x) variables)? Model fit statistics? . . . We move to discrete models (e.g. logistic regression) on Monday! Dr. Mark van Duijn (RUG) Advanced Statistical Analysis 16 Feb 202326 / 28 Introduction Interpretation Functional form Collinearity issues Conclusions What did we learn? Make judgements on when to transform variables (and when not) Being able to interpret estimated coefficients of different kind of independent variables Exploring non-linear relationships between y and x using OLS Identify and deal with (multi)collinearity How to deal with data in a responsible way Dr. Mark van Duijn (RUG) Advanced Statistical Analysis 16 Feb 202327 / 28 Introduction Interpretation Functional form Collinearity issues Conclusions Next Computer lab sessions: Today 15h00-17h00 Lecture: Next Monday from 11h00-13h00 Prepare Mehmetoglu & Jakobsen (2022) - Chapter 8 DeMaris (1995) - Tutorial on logistic regression Dr. Mark van Duijn (RUG) Advanced Statistical Analysis 16 Feb 202328 / 28