AppliedAI%206.pdf
Document Details
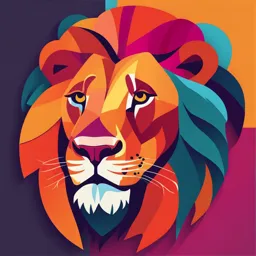
Uploaded by DelightedPolonium
Stanford University
Tags
Related
- 07 Innovation through Artificial Intelligence.pdf
- 07 Innovation through Artificial Intelligence.pdf
- BSIT41A Module 1: Digital Transformation PDF
- Artificial Intelligence and Machine Learning Applications in Smart Production (PDF)
- DST301 Artificial Intelligence Applications Lecture 02 - Machine Learning PDF
- CPCS-335 Introduction to Artificial Intelligence Lecture 8 PDF
Full Transcript
Machine Learning Lifecycle Management AI for Innovation and Entrepreneurship Tristan Post | December 4th, 2023 | TUM Audimax Machine Learning Lifecycle Management ‹#› What Companies Think AI Looks Like ‹#› What Companies Think AI Looks Like ‹#› What Companies Think AI Looks Like ‹#› M...
Machine Learning Lifecycle Management AI for Innovation and Entrepreneurship Tristan Post | December 4th, 2023 | TUM Audimax Machine Learning Lifecycle Management ‹#› What Companies Think AI Looks Like ‹#› What Companies Think AI Looks Like ‹#› What Companies Think AI Looks Like ‹#› Machine Learning Lfiecycle Management ‹#› Lorem Ipsum ‹#› MLOps MLOps unifies machine learning development and operations, emphasizing seamless integration and deployment of ML models in production environments. ‹#› Lorem IMpusm ‹#› Human Centric MLOps Human-Centric MLOps emphasizes how AI fits into human workflows and behaviors, ensuring models align with organizational needs and are adoptable by users. ‹#› Human Centric ML Lifestyle ‹#› Scoping ‹#› Human Centric ML Lifestyle ‹#› Starting From a Real Need ‹#› Starting From a Real Need ‹#› Always ask: what is the right tool for the job? ‹#› Lorem Ipsum ‹#› To begin with theend in mind means to start with a clear understanding of your destination. It means to know where you’re going so that you better understand where you are now and so that the steps you take are always in the right direction Stephen Covey Author ‹#› A Tale of Two Approaches ‹#› Set KPIs ‹#› ‹#› Data ‹#› Human Centric ML Lifestyle ‹#› Lorem Ipsum ‹#› Acquiring and Preparing Representative Data ‹#› Acquiring Representative Data ‹#› Preparing Representative Data ‹#› Acquiring Representative Data ‹#› Deep Dive: Data Cleaning ‹#› Key Steps of Data Preparation ‹#› Training, Test and Validation Sets ‹#› Training, Test and Validation Sets ‹#› Lorem Ipsum ‹#› Data Collection Types of Labels ‹#› Types of Labels ‹#› Modeling ‹#› Human Centric ML Lifecycle ‹#› Algorithm Selection and Model Evaluation ‹#› Data to Trained Model ‹#› Choosing the Right Algorithm ‹#› Undercutting ‹#› Undercutting ‹#› Half knowledge is worse than ignorance Thomas B. Macaulay Historian ‹#› Overfitting ‹#› Overfitting ‹#› Proper Generalisation ‹#› Proper Generalisation ‹#› Model Valuation Generalisation ‹#› Lorem Ipsum ‹#› Lorem Ipsum RetinaNet network architecture composed of four main components: a) Bottom-up pathway; b) top-down pathway; c) classification subnetwork; d) regression subnetwork ‹#› Lorem Ipsum ‹#› Lorem Ipsum ‹#› Lorem Ipsum ‹#› Lorem Ipsum ‹#› Lorem Ipsum ‹#› Classification Confusion Matrix ‹#› Classification Confusion Matrix ‹#› Classification Accuracy ‹#› Classification Accuracy ‹#› Classification Precision ‹#› Classification Precision ‹#› Classification Recall ‹#› Classification Recall ‹#› Mean Squared Error ‹#› Model Selection ‹#› Test Data ‹#› Model Evaluation RetinaNet + ResNet50 RetinaNet + ResNet101 RetinaNet + ResNet152 ‹#› Model Evaluation ‹#› Model Evaluation ‹#› A Day in The Life of (Most) Data Scientists ‹#› Deployment ‹#› Human Centric ML Lifecycle ‹#› Lorem Ipsum ‹#› Lorem Ipsum ‹#› Acquiring and Preparing Representative Data ‹#› From Model to Product ‹#› From Model to Product ‹#› From Model to Product ‹#› How does AI fit into the bigger picture? ‹#› Geographic Information System (GIS) ‹#› From Prediction to Product to Value ‹#› How is the Product/Service going to be used? ‹#› Usage ‹#› Human Centric ML Lifestyle ‹#› People ‹#› People ‹#› People S R E T N E L P E M IM ‹#› People ‹#› Starting with a Pilot ‹#› Importance of a Release Strategy ‹#› Rollout ‹#› Monitoring & Feedback ‹#› Human Centric ML Lifestyle ‹#› Monitoring ‹#› Data Drift ‹#› Data Drift ‹#› (Continuous) Retraining ‹#› System Monitoring 1. Objectives of System Monitoring 2. Key Areas to Monitor - Ensure Reliability - Technical Glitches: Hardware & Software - Maintain Security - User Behavior: Usage Patterns & Feedback - Optimize Performance - Security: Breach Detection & Data Integrity Human Centric MLOps ‹#› Human Centric MLOps ‹#› Human Centric MLOps ‹#› Human Centric MLOps ‹#› • Amazon Web Services. (2019, August 1). How Machine Learning Kept Out the Cat [Video]. YouTube. Retrieved from https://www.youtube.com/watch?v=bW8b1gWMaJk&t=7s • American tank moves along an Italian road with a large explosion in the background, circa 1944-1945. (n.d.). Retrieved from https://www.ww2online.org/image/american-tank-moves-along-italian-road-large-explosionbackground-circa-1944-1945 • A n a r t i f i c i a l i n t e l l i g e n c e l i f e c y c l e : F r o m c o n c e p t i o n t o p r o d u c t i o n . " ( n . d . ) . I n S c i e n c e D i r e c t . R e t r i e v e d f r o m h t t p s : / / w w w. s c i e n c e d i r e c t . c o m / s c i e n c e / a r t i c l e / p i i / S2666389922000745#:~:text=The%20CDAC%20AI%20life%20cycle,context%20of%20AI%20are%20presented • Ani-Tank Dogs. (2018, October 8). In r/OldSchoolSad. Retrieved from https://www.reddit.com/r/OldSchoolSad/comments/9mef63/antitank_dogs/ • Anti-tank dog unit with trainers. (n.d.). In Quora. Retrieved from https://www.quora.com/What-is-a-random-fact-about-WWI • Arfaoui, N. (2023). Comparative Study of Machine Learning Algorithms for Movie Sentiment Analysis. Journal of Information Assurance & Security, 18(1), 25-38. • Baker, J. (2016, August 1). Feature Flag-Driven Releases. Medium. Retrieved from https://medium.com/@justinucd/feature-flag-driven-releases-7bf60d1232b1 • Bunk, T., Varshneya, D., Vlasov, V., and Nichol, A. (2020). Diet: Lightweight language understanding for dialogue systems. • Centrality of AI Quality in MLOPs Lifecycle and Its Impact on the..." (n.d.). In SpringerLink. Retrieved from https://link.springer.com/chapter/10.1007/978-3-031-35510-3_42 • Comparative Study of Machine Learning Algorithms for Movie Sentiment Analysis. (2023). Journal of Information Assurance & Security, 18(1), 25-38. • Covey, S. R. (2020). The 7 Habits of Highly Effective People: 30th Anniversary Edition. • De Mulder, W. (2022). The Use of Partial Order Relations and Measure Theory in Developing Objective Measures of Explainability. In: Calvaresi, D., Najjar, A., Winikoff, M., Främling, K. (eds) Explainable and Transparent AI and Multi-Agent Systems. EXTRAAMAS 2022. Lecture Notes in Computer Science(), vol 13283. Springer, Cham. https://doi.org/10.1007/978-3-031-15565-9_11 • Engineer Makes an AI-Enabled Cat Flap to Stop His Cat from Bringing Home Dead Animals. (2019, July 9). Adafruit. Retrieved from https://blog.adafruit.com/2019/07/09/engineer-makes-an-ai-enabled-cat-flap-to-stop-hiscat-from-bringing-home-dead-animals/ • Forbes Tech Council. (2020, October 14). Council Post: Why Do Most AI Projects Fail? Forbes. Retrieved from https://www.forbes.com/sites/forbestechcouncil/2020/10/14/why-do-most-ai-projects-fail/ • France uses AI to detect more than 20,000 undeclared swimming pools. (2022, August 30) Euronews. Retrieved from https://www.euronews.com/video/2022/08/30/france-uses-artificial-intelligence-to-detect-morethan-20000-undeclared-swimming-pools • French Tax Collectors Use A.I. to Spot Thousands of Undeclared Pools. (2022, August 2022 ). The New York Times. Retrieved from https://www.nytimes.com/2022/08/30/world/europe/france-taxes-pools-artificialintelligence.html • Gomede, E., (2022, November 8). Understanding Data Drift in Machine Learning. Medium. Retrieved from https://medium.com/@evertongomede/understanding-data-drift-in-machine-learning-51bf0022ee14 • Hamm, B. (2019, June 11). Cats, Rats, A.I., Oh My! [Video]. YouTube. Retrieved from https://www.youtube.com/watch?v=1A-Nf3QIJjM&t=178s • How Fast Will AI Automation Arrive? Uncharted Territories. (2023). Retrieved from https://unchartedterritories.tomaspueyo.com/p/how-fast-will-ai-automation-arrive • How Machine Learning Kept Out the Cat. (2019, August 1). [Video]. YouTube. Retrieved from https://www.youtube.com/watch?v=bW8b1gWMaJk&t=7s ‹#› • How TimeXtender Bridges the Data Team Divide: Data Movers vs Data Users. (2022, August 15). Medium. Retrieved from https://medium.com/data-empowerment-with-timextender/how-timextender-bridges-the-data-teamdivide-data-movers-vs-data-users-d9d01d296cf2 • Kiger, P. J. (2021, December 9). Flip Flop: Why Zillow's Algorithmic Home Buying Venture Imploded. Stanford Graduate School of Business. Retrieved from https://www.gsb.stanford.edu/insights/flip-flop-why-zillowsalgorithmic-home-buying-ve • Kumar, S. (2022, April 6). “Staying Ahead of the Game: Using PSI and CSI to Ensure Your Data and Models Remain Relevant”. Medium. Retrieved from https://medium.com/@shubham_kumar318/staying-ahead-of-the-gameusing-psi-and-csi-to-ensure-your-data-and-models-remain-relevant-38f0836824eb • Lakhani M. (2021), Inside a Car with its Own Mind – LiDAR Vs Computer Vision Explained. Medium. Retrieved from https://medium.com/analytics-vidhya/inside-a-car-with-its-own-mind-960d792c3b42 • Li, Y. (2022, June 8). How to Estimate the Time and Cost to Train a Machine Learning Model. Towards Data Science. Retrieved from https://towardsdatascience.com/how-to-estimate-the-time-and-cost-to-train-a-machinelearning-model-eb6c8d433ff7 • Metz, R. (2021, November 9). Zillow's home-buying debacle shows how hard it is to use AI to value real estate | CNN Business. CNN. Retrieved from https://edition.cnn.com/2021/11/09/tech/zillow-ibuying-home-zestimate/ index.html • MLOps: a guide to its adoption in the context of responsible AI." (n.d.). In ACM Digital Library. Retrieved from https://dl.acm.org/doi/ 10.1145/3526073.3527591#:~:text=DevOps%20practices%20have%20increasingly%20been,to%20to%20implement%20it%20effectively • MLOps Spanning Whole Machine Learning Life Cycle: A Survey. (n.d.). Zhengxin, F., & other authors. Retrieved from https://arxiv.org/abs/ 2304.07296#:~:text=Download%20a%20PDF%20of%20the,wider%20adoptions%20in%20various • Pascanean, A. (2022, July 27). The Myth of the Data Science Trap. Vantage AI. Retrieved from https://www.vantage-ai.com/blog/the-myth-of-the-data-science-trap • Publikum. (n.d.). Soviet 'suicide' dogs, 1941. Retrieved from https://publikum.net/wie-hunde-fur-den-krieg-eingesetzt-wurden/ • Pueyo T., (2023), How Fast Will AI Automation Arrive? Uncharted Territories. Retrieved from https://unchartedterritories.tomaspueyo.com/p/how-fast-will-ai-automation-arrive • Quora. (n.d.). Anti-tank dog unit with trainers. Retrieved from https://www.quora.com/What-is-a-random-fact-about-WWI • RAD Industries. (2019, December 5). T-34/76. Retrieved from https://radindustries.wordpress.com/2019/12/05/t-34-76/ • Retail Sales Associate PPT PowerPoint Presentation Professional Graphics Design. SlideTeam. Retrieved from https://www.slideteam.net/retail-sales-associate-ppt-powerpoint-presentation-professional-graphics-designcpb.html • Saturn Cloud. (2020, August 25). MLOps: A complete guide for building, deploying, and managing machine learning models. Data Science Dojo. Retrieved from https://datasciencedojo.com/blog/mlops-guide/ • Scherpenberg, A. (n.d.). What companies think AI looks like [Illustration]. Tangent Works. • ScienceDirect. (n.d.). The pipeline for the continuous development of artificial intelligence models—Current state of research and practice. Retrieved from https://www.sciencedirect.com/science/article/pii/ S0164121223000109#:~:text=Based%20on%20these%20sources%2C%20this,CD4ML • ScienceDirect. (n.d.). Visualization in machine learning lifecycle is critically important and it can be categorized based on the presented functional features. Retrieved from https://link.springer.com/chapter/ 10.1007/978-3-031-35510-3_42#:~:text=Visualization%20in%20machine%20learning%20lifecycle,interesting%20patterns%20in%20the%20data ‹#› • Soviet anti-tank dog. (2023, February 16). Tank Archives. Twitter. Retrieved from https://twitter.com/Tank_Archives/status/1625869809136041985 • Soviet 'suicide' dogs, 1941. (n.d.). Publikum. Retrieved from https://publikum.net/wie-hunde-fur-den-krieg-eingesetzt-wurden/ • Tank Archives. (2023, February 16). Soviet anti-tank dog. Twitter. Retrieved from https://twitter.com/Tank_Archives/status/1625869809136041985 • Tangent Works. (n.d.). What companies think AI looks like [Illustration]. Retrieved from https://www.tangentworks.com/wp-content/uploads/2022/06/Tangent-Works-Illustration-What-companies-think-AI-looks-like.png • The 5 Most Important Stages in Machine Learning Lifecycle. (2021, August 7). Medium. Retrieved from https://medium.com/@amit0388naik/what-are-5-important-stages-in-machine-learning-lifecycle-ae3aecf59581 • The Myth of the Data Science Trap. (2022, July 27). Vantage AI. Retrieved from https://www.vantage-ai.com/blog/the-myth-of-the-data-science-trap • The pipeline for the continuous development of artificial intelligence models—Current state of research and practice. (n.d.). ScienceDirect. Retrieved from https://www.sciencedirect.com/science/article/pii/ S0164121223000109#:~:text=Based%20on%20these%20sources%2C%20this,CD4ML • T-34/76. (2019, December 5). RAD Industries. Retrieved from https://radindustries.wordpress.com/2019/12/05/t-34-76/ • Towards Data Science. (2022, June 8). How to Estimate the Time and Cost to Train a Machine Learning Model. Retrieved from https://towardsdatascience.com/how-to-estimate-the-time-and-cost-to-train-a-machinelearning-model-eb6c8d433ff7 • Uncharted Territories. (2023). How Fast Will AI Automation Arrive? Retrieved from https://unchartedterritories.tomaspueyo.com/p/how-fast-will-ai-automation-arrive • Upadhyay, D. (2022, August 15). How TimeXtender Bridges the Data Team Divide: Data Movers vs Data Users. Retrieved from https://medium.com/data-empowerment-with-timextender/how-timextender-bridges-thedata-team-divide-data-movers-vs-data-users-d9d01d296cf2 • Visualization in machine learning lifecycle is critically important and it can be categorized based on the presented functional features. (n.d.). SpringerLink. Retrieved from https://link.springer.com/chapter/ 10.1007/978-3-031-35510-3_42 • What companies think AI looks like [Illustration]. (n.d.). Tangent Works. Retrieved from https://www.tangentworks.com/wp-content/uploads/2022/06/Tangent-Works-Illustration-What-companies-think-AI-looks-like.png • WIRED UK. (2019, August 1). New LiDAR: Driverless cars are about to get a whole lot better at seeing the world | WIRED Originals [Video]. YouTube. Retrieved from https://www.youtube.com/watch? v=bW8b1gWMaJk&t=7s • Zillow's home-buying debacle shows how hard it is to use AI to value real estate | CNN Business. (2021, November 9). CNN. Retrieved from https://edition.cnn.com/2021/11/09/tech/zillow-ibuying-home-zestimate/ index.html ‹#›