Applied Analytics for Business – Healthcare PDF
Document Details
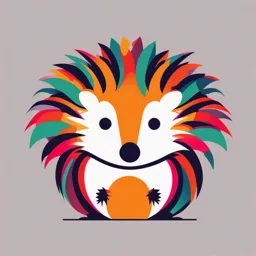
Uploaded by IntuitiveRiver
Praxis Business School
2023
Dr. Ravi Shankar
Tags
Summary
This presentation is on applied analytics for business in healthcare. It covers topics such as Health 4.0, HAAM, and associated technologies, along with an examination of the challenges and solutions in healthcare.
Full Transcript
Applied Analytics for Business – Healthcare Dr. Ravi Shankar Prof – Data Science (AI -ML) Dean – Enterprise Solutions & Academic Collaborations https://www.linkedin.com/in/ravi-shankar-70584b1/ [email protected] Session 2 (Forenoon), • HC 4.0 • HAAM March 22nd , 2023 Faculty Profile : Dr. Ravi...
Applied Analytics for Business – Healthcare Dr. Ravi Shankar Prof – Data Science (AI -ML) Dean – Enterprise Solutions & Academic Collaborations https://www.linkedin.com/in/ravi-shankar-70584b1/ [email protected] Session 2 (Forenoon), • HC 4.0 • HAAM March 22nd , 2023 Faculty Profile : Dr. Ravi Shankar INTRODUCTION • Background: PhD - Econometrics, Entrepreneur, Start-up Mentor, Social Impact Investor, Data Science Thought Leader, Senior Venture Partner • Current Focus: AI adoption in Business, Deep Learning, XAI, ML Operations, Design Thinking, OB & Strategy • Sectoral Exposure: multiple sectors straddling BFSI / Pharma / Manufacturing / Retail / Tech • Overall Experience : 30 Yrs. Applied Analytics for Business - Healthcare: Learning Journey Five sessions of 1.5 hours each First & Second session Introduction Third session Healthcare 4.0 + HC - AMM Fourth session Data Governance Fifth A session Fifth B session AI- ML Real World Applications – I AI- ML Real World Applications – II Mortality Prediction Suicide Rate Trend analysis STRUCTURE HC 4.0 HC-AMM Real World Example Trends in the following six areas will determine organizational success in 2023 and have a lasting impact on providers and patients: Health equity. Patient safety. Staffing. Care delivery. COVID-19 recovery. Payment and payers Driven by networked Electronic Health Record systems, Artificial Intelligence, real-time data from wearable devices with an overlay of invisible user interfaces and improved analytics, Health 4.0 is changing the healthcare industry. The focus on collaboration, coherence, and convergence that will make healthcare more predictive and personalised. Furthermore, Health 4.0 realizes the value of data more consistently and effectively. It can pinpoint areas of improvement and enable more informed decisions. What it also does is help move the entire healthcare industry from a system that is reactive and focused on fee-for-service to a system that is value-based, which measures outcomes and ensures proactive prevention What is Health 4.0? Health 4.0 is defined as “a strategic concept for the health domain derived from the Industry 4.0 concept.” The term is often used synonymously with digital health, m-health, e-health and smart health (Thuemmler and Bai, 2017). Behind this concept exists the aim of virtualisation in healthcare and personalization for patients, professionals and other stakeholders and an overall improvement of the health industry with technology. Briefly, Health 4.0 can be described as a phenomenon to improve healthcare service and improve connectivity between health care stakeholders using technology. Three “pillars” of Health 4.0; Design Technol ogy People Motivation What is Health? What is well being? How are they related? What is HC 4.0 & how it is transforming HC? What is HC – AMM & why is it important? There is no one universal definition of health. Infact, there are four main schools of thought on the definition of health 3 Popular around the 1920s, health is defined as: MEDICAL MODEL OF H E A LT H D E F I N ITION "A state characterized by anatomic, physiologic and psychologic integrity; Ability to perform personally valued family, work and community roles; Ability to deal with physical, biologic, psychologic and social stress." J. Stokes, J. Noren, S. Shindell, Definition of terms and concepts applicable to clinical preventive medicine. J. Community Health 8(1), 33–41 (1928). http://www.ncbi.nlm.nih.gov/pubmed/676 4 HOLISTIC MODEL OF H E A LT H D E F I N ITION In 1946, World Health Organization (WHO) definition, "A state of complete physical, ▪ mental and ▪ social ▪ well-being and not merely the absence of disease or infirmity" This is the most commonly used definition of health. https://www.who.int/governance/eb/who_constitution_en.pdf. 5 Promoted by the World Health Organization (WHO) in 1984 definition was, ▪ “The extent to which an individual or WE L L N E S S MODEL OF H E A LT H DEFINITION group is able to realize aspirations and satisfy needs, and to change or cope with the environment. ▪ Health is a resource for everyday life, not the objective of living; ▪ It is a positive concept, emphasizing social and personal resources, as well as physical capacities." ▪ For the purpose of this talk, the World Health Organization. Regional Office for Europe, Health Promotion: A Discussion Document on the Concept and Principles. Summary report of the Working Group on Concept and Principles of Health Promotion, Copenhagen, 9–13 July 1984 (WHO Regional Office for Europe, Copenhagen, 1984), http://www.who.int/iris/handle/10665/107835. http://apps.who.int/iris/bitstream/handle/10665/107835/E90607.pdf?sequence=1&isAllowe d=y. wellness definition of health is used as a reference. 6 E CO LO GICAL DEFINITION OF H E A LT H In the mid-1990s, there was a push toward an ecological definition of health and an ecological definition is ▪ "A state in which humans and other living creatures with which they interact can coexist indefinitely." J.M. Last, J.H. Abramson, International Epidemiological Association, A Dictionary of Epidemiology (Oxford University Press, 1995) 7 A good or satisfactory condition of existence; a state characterized by WELLBE IN G ▪ Health ▪ Happiness ▪ Prosperity ▪ Welfare https://www.dictionary.com/browse/well-being 8 Mental health is defined as ▪ a state of well-being in which ▪ every individual realizes his M E N TAL H E A LT H or her own potential, ▪ can cope with the normal stresses of life, ▪ can work productively and fruitfully, and ▪ is able to contribute to her or his community https://www.who.int/news-room/fact-sheets/detail/mental-health-strengthening-ourresponse 9 Let us enlist 10 P HYS I C AL HEALTH PARAM ETERS LI FE ST Y LE PARAME TERS WE L L -BE IN G AN D MENTAL HEALTH PARAMETERS World Health Organisation, https://www.who.int/social_determinants/en/. Ottawa Charter for Health Promotion, https://www.who.int/healthpromotion/conferences/previous/ottawa/en/ Wikipedia, https://en.wikipedia.org/wiki/Health. Wikipedia, https://en.wikipedia.org/wiki/Health_indicator S OC IO-E C O N OMIC PARAMETERS CONTEXTUAL & CULTURAL FACTORS 11 Journey over the past 25 years Version Web Industry Health 1.0 Read-only web (Fleerackers & Meyvis) Mechanization, water power, steam power (Roser) Printed health information (Wikipedia) 2.0 The writing and participating web (Fleerackers & Meyvis) Mass production, assembly line, electricity (Roser) Online communities, social media, patient generated content, wearables (Wikipedia) 3.0 The semantic executing web (Fleerackers & Meyvis) Computer and automation (Roser) Personalized health-related information (Wikipedia) 4.0 Mobile Web - connects all devices in the real and virtual world in realtime. (Fleerackers & Meyvis) Cyber physical systems and Digital Twins (Roser) Virtualization and Personalization (Thuemmler, 2017) Bagaria N., Laamarti F., Badawi H.F., Albraikan A., Martinez Velazquez R., El Saddik A. (2020) Health 4.0: Digital Twins for Health and Well-Being. In: El Saddik A., Hossain M., Kantarci B. (eds) Connected Health in Smart Cities. Springer, Cham 13 ▪ “Health 4.0 is progressive H E A L T H 4.0 virtualization in order to enable the personalization of health and care next to real time for patients, professionals and formal and informal carers.” C. Thuemmler, The Case for Health 4.0, in Health 4.0: How Virtualization and Big Data are Revolutionizing Healthcare, (Springer International Publishing, Cham, 2017), pp. 1–22 14 Principle No. Description Principle 1 Interoperability Principle 2 Virtualization Principle 3 Decentralization Principle 4 Real – time capability Principle 5 Service orientation Principle 6 Modularity Principle 7 Safety, security, and resilience C. Thuemmler, The Case for Health 4.0, in Health 4.0: How Virtualization and Big Data are Revolutionizing Healthcare, (Springer International Publishing, Cham, 2017), pp. 1–22 15 Healthcare challenges Industry 4.0 – Key Technologies Healthcare 4.0 – Key Technologies IoMT AI Cybersecurity Healthcare 4.0 – Applications Personalised Medicine Smart Manufacturing Smart Hospitals Changing the Way Healthcare is Delivered People Technology Money Virtualization of Care Shifting the point of care to the periphery TeleHealth Psychotherapists M-Health Community- Social Care nurse Hospital Physiotherapist Hospices Day-clinics DaySurgery Lack of Knowledge on Technology Huge Gap between R&D ➔ Innovation → Scale Resistance to Change KEY PROBLEMS Lack of Political Will Lack of Regulatory Framework Existing Technology Stack is Siloed Need to upskill existing labour Leadership Gap & need for Mindset shift Solution Healthcare 4.0 The digitization of Health 4.0 is the way forward in healthcare and promises care to be more precise and personalized. Health 4.0 will restructure the healthcare system to the convenience of all stakeholders, most importantly the patient, and it will reduce cost in healthcare. Overall, Health 4.0 looks to be revolutionizing healthcare with the latest technology and for the better. HAAM The Healthcare Analytics Adoption Model (HAAM) provides healthcare organizations with a framework to follow in order to fully leverage the capabilities of analytics and achieve the primary goals of using data in healthcare— to improve patient outcomes while cutting costs, decreasing provider burnout, and maintaining patient satisfaction HAAM The focus on analytics is contributing to the “EHR problem”—doctors prioritizing the EHR over patients. The Healthcare Analytics Adoption Model (HAAM) walks healthcare organizations through nine levels that lay the framework to fully leverage analytic capabilities to improve patient outcomes Level 1. Enterprise Data Operating System Level 2. Standardized Vocabulary & Patient Registries Level 6. Population Health Management & Suggestive Analytics Level 3. Automated Internal Reporting Level 7. Clinical Risk Intervention & Predictive Analytics Level 4. Automated External Reporting Level 8. Personalized Medicine & Prescriptive Analytics Level 5. Waste and Care Variability Reduction Level 9. Direct-ToPatient Analytics & Artificial Intelligence Assignment “The aggressiveness of the EMR’s incursion into the doctor– patient relationship has forced us to declare our loyalties: are we taking care of patients or are we taking care of the EMR?” Is data analytics part of the problem? • Five page note on the evolution of the HAAM framework from Sanders’s Hierarchy of Analytics Needs…..to date. • Three page note about HIMSS (Healthcare Information and Management Systems Society) Thank you Appendix Nomenclation • • • • Industry 1.0 – automation (Steam) Industry 2.0 – Electrification Industry 3.0 – Computerization Industry 4.0 – CPS +++ Industry 4.0 Design Principles • • • • • • Interoperablility Virtualization Dezentralisierung Real-time capability Service Orientation Modularity Herrmann M, Pentak T, Otto B (2015), Design Principles for Industrie 4.0 Scenarios: A Literature Review, Technische Universität Dortmund, Audi Foundation Professorship Supply Net Order Management Industry 4.0 • Industry 4.0 is a major joint Strategy framework by the German Government and the German industry • It is considered beneficial for all major industries such as Manufacturing, Energy, Logistics, etc. • It will add value to processes through the creation of services on top of legacy systems Industry 4.0 • Horizontal integration through value networks • End-to-end integration through engineering across the entire value chain • Vertical integration and networked manufacturing systems • Individual customer and product features and specifications Cyber Academy, Edinburgh; 27.08.2015 Terminal Processor Storage Real World Sensor John Services Virtual World Real World Sensors Services Virtual World Virtual World Services Real World Sensors Domains Cyber Academy, Edinburgh; 27.08.2015 Virtual World Services CPS Real World Sensors Domains Cyber Academy, Edinburgh; 27.08.2015 Hybrid Cloud Architecture for Virtualization of Care Flowchart m-Health Application to support patients with Reflux disease Monitoring pandemics utilizing the social web SDN and MEC for Synchronization of telecom and service net Software Defined Networks • To enhance the Quality of Experience for End-users • Aggregation of different verticals and services • Synchronization of telecommunication- and service network • Adjust Quality of Service (Big Data Analysis) • Solve local problems locally: direct local connection instead of directing every call to the back-end and back, thereby reducing bandwidth requirements. Carbon footprint? • SDN to enhance privacy 5G Networks • More than just more of the same • Not only faster • New frequencies (spectrum negotiations with global standardization bodies in progress) • New functionalities • End-to-end latency down to 5 ms • 1000 times more devices Conclusion • Health 4.0 will virtualize care and generate service layer on top of health legacy systems • More bandwidth and better connectivity needed • SDN to integrate telecom and service network • SDNs for aggregation of services and domains (verticals) • Mobile Edge Cloud to solve local problems locally Cyber Academy, Edinburgh; 27.08.2015 Healthcare Analytics Adoption Model Healthcare Analytics Adoption Model, 2013 By 2013, as health systems were maturing and leveraging analytics at a deeper level, the HAAM followed suit by adding three more levels that focused on automation, machine learning (ML), and a more individualized approach to medicine. The model also matured to emphasize the basics for analytics acumen—levels three and four, the basic internal and external reporting requirements—before moving to levels five and above. The inefficiencies and overwhelming number of quality measures and KPIs in levels three and four were a roadblock for many health systems, so it was critical to increase the efficiency and the productivity at levels four and below in order to move forward. HIMSS and Health Catalyst Adoption Model for Analytic Maturity, 2015 In 2015, Health Catalyst and Healthcare Information and Management Systems Society (HIMSS) saw the need to create a standard model for analytic maturity, so they teamed up to create an official Adoption Model for Analytic Maturity (Figure 3) that could serve as the industry standard—disconnected from any vendor. HAAM 2019 The latest version of HAAM (Figure 4) is based largely on the original model. Currently, Dr. Anne Snowdon, Director of Clinical Research at HIMSS Analytics, and Dale Sanders are working together to ensure the modifications are collaborative and supportive of HIMSS’s initiatives, by adding Level 9— focused on increasing patients’ analytics acumen and helping them become data-driven when it comes to their own care. Progression in the Model The patterns at each level • Data content expands • • Data timeliness increases • • To support faster decision cycles and lower “Mean Time To Improvement” Data governance expands • • Adding new sources of data to expand our understanding of care delivery and the patient Advocating greater data access, utilization, and quality The complexity of data binding and algorithms increases • From descriptive to prescriptive analytics • From “What happened?” to “What should we do?” The Expanding Ecosystem of Data Content • • • • • • • • • • • • • • • • Real time 7x24 biometric monitoring data for all patients in the ACO Genomic data Long term care facility data Patient reported outcomes data* Home monitoring data Familial data External pharmacy data Bedside monitoring data Detailed cost accounting data* HIE data Claims data Outpatient EMR data Inpatient EMR data Imaging data Lab data Billing data * - Not currently being addressed by vendor products 2-4 years 1-2 years 3-12 months Six Phases of Data Governance You need to move through these phases in no more than two years • Phase 6: Acquisition of Data • Phase 5: Utilization of Data • Phase 4: Quality of Data • Phase 3: Stewardship of Data • Phase 2: Access to Data • Phase 1: Cultural Tone of “Data Driven” 2-4 years 1-2 years 3-12 months 7 Healthcare Analytics Adoption Model: One Page Self-Inspection Guide Level 8 Personalized Medicine & Prescriptive Analytics: Analytic motive expands to wellness management, physical and behavioral functional health, and mass customization of care. Analytics expands to include NLP of text, prescriptive analytics, and interventional decision support. Prescriptive analytics are available at the point of care to improve patient specific outcomes based upon population outcomes. Data content expands to include 7x24 biometrics data, genomic data and familial data. The EDW is updated within a few minutes of changes in the source systems. Level 7 Clinical Risk Intervention & Predictive Analytics: Analytic motive expands to address diagnosis-based, fixed-fee per capita reimbursement models. Focus expands from management of cases to collaboration with clinician and payer partners to manage episodes of care, using predictive modeling, forecasting, and risk stratification to support outreach, triage, escalation and referrals. Physicians, hospitals, employers, payers and members/patients collaborate to share risk and reward (e.g., financial reward to patients for healthy behavior). Patients are flagged in registries who are unable or unwilling to participate in care protocols. Data content expands to include home monitoring data, long term care facility data, and protocol-specific patient reported outcomes. On average, the EDW is updated within one hour or less of source system changes. Level 6 Population Health Management & Suggestive Analytics: The “accountable care organization” shares in the financial risk and reward that is tied to clinical outcomes. At least 50% of acute care cases are managed under bundled payments. Analytics are available at the point of care to support the Triple Aim of maximizing the quality of individual patient care, population management, and the economics of care. Data content expands to include bedside devices, home monitoring data, external pharmacy data, and detailed activity based costing. Data governance plays a major role in the accuracy of metrics supporting quality-based compensation plans for clinicians and executives. On average, the EDW is updated within one day of source system changes. The EDW reports organizationally to a C-level executive who is accountable for balancing cost of care and quality of care. Level 5 Waste & Care Variability Reduction: Analytic motive is focused on measuring adherence to clinical best practices, minimizing waste, and reducing variability. Data governance expands to support care management teams that are focused on improving the health of patient populations. Populationbased analytics are used to suggest improvements to individual patient care. Permanent multidisciplinary teams are in-place that continuously monitor opportunities to improve quality, and reduce risk and cost, across acute care processes, chronic diseases, patient safety scenarios, and internal workflows. Precision of registries is improved by including data from lab, pharmacy, and clinical observations in the definition of the patient cohorts. EDW content is organized into evidence-based, standardized data marts that combine clinical and cost data associated with patient registries. Data content expands to include insurance claims (if not already included) and HIE data feeds. On average, the EDW is updated within one week of source system changes. Level 4 Automated External Reporting: Analytic motive is focused on consistent, efficient production of reports required for regulatory and accreditation requirements (e.g. CMS, Joint Commission, tumor registry, communicable diseases); payer incentives (e.g. MU, PQRS, VBP, readmission reduction); and specialty society databases (e.g. STS, NRMI, Vermont-Oxford). Adherence to industry-standard vocabularies is required. Clinical text data content is available for simple key word searches. Centralized data governance exists for review and approval of externally released data. Level 3 Automated Internal Reporting: Analytic motive is focused on consistent, efficient production of reports supporting basic management and operation of the healthcare organization. Key performance indicators are easily accessible from the executive level to the front-line manager. Corporate and business unit data analysts meet regularly to collaborate and steer the EDW. Data governance expands to raise the data literacy of the organization and develop a data acquisition strategy for Levels 4 and above. Level 2 Standardized Vocabulary & Patient Registries: Master vocabulary and reference data identified and standardized across disparate source system content in the data warehouse. Naming, definition, and data types are consistent with local standards. Patient registries are defined solely on ICD billing data. Data governance forms around the definition and evolution of patient registries and master data management. Level 1 Enterprise Data Warehouse: At a minimum, the following data are co-located in a single data warehouse, locally or hosted: HIMSS EMR Stage 3 data, Revenue Cycle, Financial, Costing, Supply Chain, and Patient Experience. Searchable metadata repository is available across the enterprise. Data content includes insurance claims, if possible. Data warehouse is updated within one month of source system changes. Data governance is forming around the data quality of source systems. The EDW reports organizationally to the CIO. Level 0 Fragmented Point Solutions: Vendor-based and internally developed applications are used to address specific analytic needs as they arise. The fragmented point solutions are neither co-located in a data warehouse nor otherwise architecturally integrated with one another. Overlapping data content leads to multiple versions of analytic truth. Basic internal & external reports are labor intensive and inconsistent. Data governance is nonexistent. Healthcare Analytics Adoption Model Level 8 Level 7 Fragmented Point Solutions ● Vendor-based and internally developed applications are used to address specific analytic needs as they arise. ● The fragmented point solutions are neither co-located in a data warehouse nor otherwise architecturally integrated with one another. ● Overlapping data content leads to multiple versions of analytic truth. ● Basic internal & external reports are labor intensive and inconsistent. ● Data governance is non-existent. Level 6 Level 5 Level 4 Level 3 Level 2 Level 1 Level 0 Healthcare Analytics Adoption Model Level 8 Level 7 Integrated, Enterprise Data Warehouse ● At a minimum, the following data are co-located in a single data warehouse, locally or hosted: HIMSS EMR Stage 3 data, Revenue Cycle, Financial, Costing, Supply Chain, and Patient Experience. ● Searchable metadata repository is available across the enterprise. ● Data content includes insurance claims, if possible. ● Data warehouse is updated within one month of source system changes. ● Data governance is forming around the data quality of source systems. ● The EDW reports organizationally to the CIO. Level 6 Level 5 Level 4 Level 3 Level 2 Level 1 Level 0 Healthcare Analytics Adoption Model Level 8 Level 7 Standardized Vocabulary & Patient Registries ● Master vocabulary and reference data identified and standardized across disparate source system content in the data warehouse. ● Naming, definition, and data types are consistent with local standards. ● Patient registries are defined solely on ICD billing data. ● Data governance forms around the definition and evolution of patient registries and master data management. Level 6 Level 5 Level 4 Level 3 Level 2 Level 1 Level 0 Healthcare Analytics Adoption Model Level 8 Level 7 Automated Internal Reporting ● Analytic motive is focused on consistent, efficient production of reports supporting basic management and operation of the healthcare organization. ● Key performance indicators are easily accessible from the executive level to the front-line manager. ● Corporate and business unit data analysts meet regularly to collaborate and steer the EDW. ● Data governance expands to raise the data literacy of the organization and develop a data acquisition strategy for Levels 4 and above. Level 6 Level 5 Level 4 Level 3 Level 2 Level 1 Level 0 Healthcare Analytics Adoption Model Level 8 Automated External Reporting ● Analytic motive is focused on consistent, efficient production of reports required for regulatory and accreditation requirements (e.g. CMS, Joint Commission, tumor registry, communicable diseases); payer incentives (e.g. MU, PQRS, VBP, readmission reduction); and specialty society databases (e.g. STS, NRMI, Vermont-Oxford). ● Adherence to industry-standard vocabularies is required. Level 1 ● Clinical text data content is available for simple key word searches. Level 0 ● Centralized data governance exists for review and approval of externally released data. Level 7 Level 6 Level 5 Level 4 Level 3 Level 2 Healthcare Analytics Adoption Model Level 8 Level 7 Waste & Care Variability Reduction (1) ● Analytic motive is focused on measuring adherence to clinical best practices, minimizing waste, and reducing variability. ● Data governance expands to support care management teams that are focused on improving the health of patient populations. ● Population-based analytics are used to suggest improvements to individual patient care. ● Permanent multidisciplinary teams are in-place that continuously monitor opportunities to improve quality, and reduce risk and cost, across acute care processes, chronic diseases, patient safety scenarios, and internal workflows. Level 6 Level 5 Level 4 Level 3 Level 2 Level 1 Level 0 continued Healthcare Analytics Adoption Model Level 8 Level 7 Waste & Care Variability Reduction (2) ● Precision of registries is improved by including data from lab, pharmacy, and clinical observations in the definition of the patient cohorts. ● EDW content is organized into evidence-based, standardized data marts that combine clinical and cost data associated with patient registries. ● Data content expands to include insurance claims (if not already included) and HIE data feeds. ● On average, the EDW is updated within one week of source system changes. Level 6 Level 5 Level 4 Level 3 Level 2 Level 1 Level 0 Healthcare Analytics Adoption Model Level 8 Population Health Management & Suggestive Analytics (1) Level 7 ● The “accountable care organization” shares in the financial risk and reward that is tied to clinical outcomes. ● At least 50% of acute care cases are managed under bundled payments. Analytics are available at the point of care to support the Triple Aim of maximizing the quality of individual patient care, population management, and the economics of care. ● Data content expands to include bedside devices, home monitoring data, external pharmacy data, and detailed activity based costing. Level 6 Level 5 Level 4 Level 3 Level 2 Level 1 Level 0 continued Healthcare Analytics Adoption Model Level 8 Level 7 Population Health Management & Suggestive Analytics (2) ● Data governance plays a major role in the accuracy of metrics supporting quality-based compensation plans for clinicians and executives. ● On average, the EDW is updated within one day of source system changes. ● The EDW reports organizationally to a C-level executive who is accountable for balancing cost of care and quality of care. Level 6 Level 5 Level 4 Level 3 Level 2 Level 1 Level 0 Healthcare Analytics Adoption Model Level 8 Level 7 Level 6 Level 5 Clinical Risk Intervention & Predictive Analytics (1) ● Analytic motive expands to address diagnosis-based, fixed-fee per capita reimbursement models. ● Focus expands from management of cases to collaboration with clinician and payer partners to manage episodes of care, using predictive modeling, forecasting, and risk stratification to support outreach, triage, escalation and referrals. ● Physicians, hospitals, employers, payers and members/patients collaborate to share risk and reward (e.g., financial reward to patients for healthy behavior). Level 4 Level 3 Level 2 Level 1 Level 0 continued Healthcare Analytics Adoption Model Level 8 Level 7 Clinical Risk Intervention & Predictive Analytics (2) ● Patients are flagged in registries who are unable or unwilling to participate in care protocols. ● Data content expands to include home monitoring data, long term care facility data, and protocol-specific patient reported outcomes. ● On average, the EDW is updated within one hour or less of source system changes. Level 6 Level 5 Level 4 Level 3 Level 2 Level 1 Level 0 Healthcare Analytics Adoption Model Level 8 Level 7 Personalized Medicine & Prescriptive Analytics ● Analytic motive expands to wellness management, physical and behavioral functional health, and mass customization of care. ● Analytics expands to include NLP of text, prescriptive analytics, and interventional decision support. ● Prescriptive analytics are available at the point of care to improve patient specific outcomes based upon population outcomes. ● Data content expands to include 7x24 biometrics data, genomic data and familial data. ● The EDW is updated within a few minutes of changes in the source systems. Level 6 Level 5 Level 4 Level 3 Level 2 Level 1 Level 0