AAB- AGRI- S4 -S5 AUG 30.pdf
Document Details
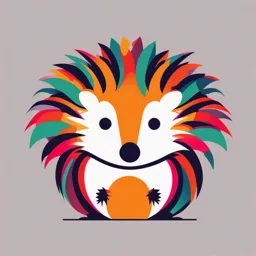
Uploaded by IntuitiveRiver
2023
Tags
Full Transcript
Applied Analytics for Business – Agriculture Dr. Ravi Shankar Prof – Data Science (AI -ML) Dean – Enterprise Solutions & Academic Collaborations https://www.linkedin.com/in/ravi-shankar-70584b1/ [email protected] Fourth & Fifth Session , AUG 30TH , 2023 AI-ML | Precision Farming | Use cases & Re...
Applied Analytics for Business – Agriculture Dr. Ravi Shankar Prof – Data Science (AI -ML) Dean – Enterprise Solutions & Academic Collaborations https://www.linkedin.com/in/ravi-shankar-70584b1/ [email protected] Fourth & Fifth Session , AUG 30TH , 2023 AI-ML | Precision Farming | Use cases & Real World Examples Faculty Profile : Dr. Ravi Shankar INTRODUCTION • Background: PhD - Econometrics, Entrepreneur, Start-up Mentor, Social Impact Investor, Data Science Thought Leader, Senior Venture Partner • Current Focus: AI adoption in Business, Deep Learning, XAI, ML Operations, Design Thinking, OB & Strategy • Sectoral Exposure: multiple sectors straddling BFSI / Pharma / Manufacturing / Retail / Tech • Overall Experience : 30 Yrs. •Global spending on smart, connected agricultural technologies and systems, including AI and machine learning, is projected to triple in revenue by 2025, reaching $15.3 billion, according to BI Intelligence Research. •Spending on AI technologies and solutions alone in Agriculture is predicted to grow from $1 billion in 2020 to $4 billion in 2026, attaining a Compound Annual Growth Rate (CAGR) of 25.5%, according to Markets&Markets. •IoT-enabled Agricultural (IoTAg) monitoring is smart, connected agriculture's fastest-growing technology segment projected to reach $4.5 billion by 2025, according to PwC. 10 Ways AI Has The Potential To Improve Agriculture In 2021-22 1. identifies animal or human breaches 2. crop yield prediction 3. Yield mapping 4. pest management 5. smart tractors, agribots and robotics 6. track-andtraceability of agricultural supply chains 7. Optimize the right mix of biodegradable pesticides 8. Price forecasting for crops based on yield rates 9. optimizing irrigation system 10. Monitoring livestock’s health According to UN Food and Agriculture Organization, the population will increase by 2 billion by 2050. However, only 4% additional land will come under cultivation by then. In this context, use of latest technological solutions to make farming more efficient, remains one of the greatest imperatives. While Artificial Intelligence (AI) sees a lot of direct application across sectors, it can also bring a paradigm shift in how we see farming today. AI-powered solutions will not only enable farmers to do more with less, it will also improve quality and ensure faster go-to-market for crops. In this lecture / session, we will discuss how AI can change the agriculture landscape, the application of drone-based image processing techniques, precision farming landscape, the future of agriculture and the challenges ahead. Top five areas where the use of cognitive solutions can benefit agriculture. Growth driven by IOT Image-based insight generation Identification of optimal mix for agronomic products Health monitoring of crops Automation techniques in irrigation and enabling farmers Research Applications More Research Applications Private Ag-Tech Firms Assignment: Research these AI in Agri Startups and write a page each on the problem-solution framework: 1. Prospera 2. Blue River Tech 3. Farmbot Machine Learning in Agriculture: What It Can Do Now and in the Future Smart Farming Species Identification Selective Breeding Agrochemical Production Soil Analysis and Disease Detection Weed Detection Water Analysis Livestock Management Livestock Health Control Grazing Control https://youtu.be/0tQ__k3G17g https://youtu.be/OHzLCjys2HI https://youtu.be/XH-EFtTa6IU AI in Agriculture — Multiple Applications Technology has been the key factor in achieving optimal yield and minimum wastage in agriculture for the past decades through the use of heavy machines on farms and as well as digital computing. With the advent of Big Data and Artificial Intelligence, the agricultural sector has received an incredible boost in solving most of its challenges as well as ensuring maximum quality in produce. For example, Israel is a global leader in exporting fresh agricultural produce despite having a geography not conducive for agriculture. This is because of the extensive use of digital computing and intelligent systems. Artificial Intelligence has been continually used to improve agricultural produce, storage and analytics since the rise of machine learning and deep learning, assisting to effectively: • obtain accurate health data of crops • detect pest and plant diseases • automate harvesting and crop sorting • obtain real-time data on soil conditions • aid the implementation of precision irrigation Uses of AI-ML in Agriculture Artificial Intelligence is being used in various sectors from home to office and now in the agriculture sectors. Machine learning in agriculture used to improve the productivity and quality of the crops in the agriculture sector. Retailers • The seed retailers use this agriculture technology to churn the data to create better crops. While the pest control companies are using them to identify the various bacteria’s, bugs and vermins. AI is used to boost the yield of crops • The AI technologies are used to determine which corn and which conditions will produce the best yield. It will also determine which weather condition will give the highest return. AI helps to identify bug hunters • One of the companies named Rentokil is using AI to kill all the bugs and vermin. Other companies are making use of Android app which is developed by Accenture to find bugs. The app takes the pictures of the bug and runs the app called as PestID. When a bug is found app will provide an immediate solution which helps the technician to take further actions. It will also recommend the chemical to be used to kill the bugs. Most Popular Applications of Machine Learning (ML) in Agriculture Agriculture Robot Monitor crop and soil • Most of the companies are now programming • Companies are now making use of and designing robots to handle the essential technologies and deep learning algorithms. task related to agriculture. This includes The data are then collected using the drones harvesting crops and works faster than then and other software to monitor the crops and human laborers. This is the best example of also the soil. They also use the software to machine learning in agriculture. control the fertility of the soil. Machine Learning (ML) Models Used in the Agriculture Industry The agricultural farmers are now taking advantage of the machine learning models and their innovations. Using AI and machine learning is good for the food tech segments. The Farmers Business Network that is being created for the farmers a social network will make use of the ML and the analytic tools to drive the results of data on pricing. Robots are now managing the crops and also monitoring them. Sensors are helping to collect the data related to crops. According to research if AI and ML are being used in agriculture, then the agriculture sector will grow in the coming years. Rising Opportunities of Machine Learning (ML) in Digital Agriculture Real-life ML Example • There is a rise in digital agriculture, which uses a secured approach to give maximum agricultural productivity by reducing the impact on the environment. The data that is generated in modern agriculture is based on various sensors that will help in better understanding of an environment like the crop, soil and the weather conditions and also about the agricultural machines. These data will help us to take quick and fast result-oriented decisions. To yield more, we need to apply machine learning to agriculture data. • A Mexico based Startup Company Descartes Labs are combining the satellite images, ML, Cloud computing and sensors to a better understanding of industries related to agriculture and energy. The company uses new technology in agriculture to discover where crops are situated and how good and healthy the crops are. • The machine learning tools which were reserved for some institutions are now accessible to all small and capable members. A small startup is making use if AI and machine learning to bring change in the modern agriculture sector. They are trying to reshape the contemporary agriculture sector by making use of innovative technologies. What is Precision Farming? 22 Precision Farming The phrase “Right Place, Right Time, Right Product” sums up precision farming. This is a more accurate and controlled technique that replaces the repetitive and labor-intensive part of farming. It also provides guidance about crop rotation, optimum planting and harvesting time, water management, nutrient management, pest attacks and so on Key technologies that enable precision farming are give below: • • • • • High precision positioning system Automated steering system Geo mapping Sensor and remote sensing Integrated electronic communication • Variable rate technology optimum planting and harvesting time, water management, nutrient management, pest attacks and so on Goals for precision farming: • Examples of precision farming management: • Profitability: Identifying crops and market strategically as well as predicting ROI based on cost and margin. • Efficiency: By investing in precision algorithm, better, faster and cheaper farming opportunities can be utilized. This enables overall accuracy and efficient use of resource • Sustainability: Improved social, environmental and economic performance ensures incremental improvements each season for all the performance indicators • Identification of stress level in a plant is obtained from highresolution images and multiple sensor data on plants. This large set of data from multiple sources needs to be used as an input for Machine Learning to enable data fusion and feature identification for stress recognition. • Machine learning models trained on plant images can be used to recognize stress levels in plants. The entire approach can be classified into four stages of identification, classification, quantification and prediction to make better decisions Precision Farming Benefits Agronomic Economic Ergonomic Production Management Return on Investment (ROI) Comfort, Fatigue, Organization Monitoring trends of field fertility and plant health over time to better build a sustainable operation without starving or over applying resources Managing product inputs by controlling rates and zones, increasing the productivity of an entire operation without over applying resources Introducing systems to make an operation more integrated, increasing time of productivity, and optimizing controllability Seven Practical Applications of AI in Agriculture Crop and soil monitoring Insect and plant disease detection Livestock health monitoring Intelligent spraying Automatic weeding Aerial survey and imaging Produce grading and sorting Crop and soil monitoring we can now use drones (UAVs) to capture aerial image data, and train computer vision models to use this for intelligent monitoring of crop and soil conditions. Visual sensing AI can analyze and interpret this data to: •track crop health •make accurate yield predictions. •detect crop malnutrition much faster than humans AI models can inform farmers of specific problem areas so that they can take immediate action. Insect and plant disease detection • Using image recognition technology based on deep learning, we can now automate detection of plant diseases and pests. This works using classification, detection, and image segmentation methods to build models that can “keep an eye” on plant health. • As with our previous examples, the alternative to computer vision requires a lot of labor-intensive human searching and evaluation. Fortunately for farmers, the AI model in this study was able to identify and diagnose disease severity with an accuracy of 90.4%! • Researchers in another study went even further by using an improved YOLO v3 algorithm to detect multiple diseases and pests on tomato plants. • Armed with a digital camera and a smartphone, the researchers took photos at local tomato greenhouses and identified 12 different cases of either disease or pests. Livestock health monitoring Computer vision can also: •Count animals, detect disease, identify unusual behavior, and monitor significant activities such as giving birth. •Collect data from cameras and drones (UAVs). •Combine with other technologies to keep farmers informed on animal health and access to food or water. ASSIGNMENT : MONITORING CALVING BEHVIOR OF COW VIA IMAGE CLASSIFICATION MODELING. Intelligent spraying UAVs equipped with computer vision AI make it possible to automate spraying of pesticides or fertilizer uniformly across a field. With real-time recognition of target spraying areas, UAV sprayers are able to operate with high precision both in terms of the area and amount to be sprayed. This significantly reduces the risk of contaminating crops, humans, animals, and water resources. Automatic weeding • Intelligent sprayers aren’t the only AI getting into weed… er, weeding. There are other computer vision robots taking an even more direct approach to eliminating unwanted plants. • Now, spotting a weed in the same way that computer vision can spot an insect or oddlybehaving chicken doesn’t actually eliminate very much work for the farmer. To be of even greater help the AI needs to both find and remove the weed. Aerial survey and imaging • AI can analyze imagery from drones and satellites to help farmers monitor crops and herds. That way they can be notified immediately if something looks amiss without having to constantly observe the fields themselves. • Aerial imaging is also useful for boosting the precision and efficiency of pesticide spraying. As mentioned previously, ensuring that pesticides only go where they’re intended saves money as well as the surrounding environment. Produce grading and sorting • Finally, AI computer vision can continue to help farmers even once the crops have been harvested. • Just as they are able to spot defects, disease, and pests as the plants are growing, imaging algorithms can also be used to sort “good” produce from the defective or just plain ugly. • By inspecting fruit and vegetables for size, shape, color, and volume, computer vision can automate the sorting and grading process with accuracy rates and speed much higher than even a trained professional. AI in Agriculture — Detecting defects in Apples ● Leverage Artificial Intelligence through the use of computer vision and deep learning to automate apple detection, sorting and identification of damaged ones. ● Training an AI for counting and detecting damaged apples ● This solution will assist farmers in increasing agricultural productivity, preventing soil degradation in cultivated land, reducing chemical use in crop production, and maximizing water resource efficiency. Assignment – Develop functional E2E Python coding Step1 — Getting Training Data Step2 — Train your AI model Step 3 — Start using your trained Models AI in Agriculture — Detecting defects in Apples | by Moses Olafenwa | DeepQuestAI | Medium Thank you Supporting Slides for AgTech Startup’s Business models in the agritech space could be trifurcated into: ► Margin-based models where players create market linkages on the input or output side, and earn margins on the buy-sell spread ► Subscription-based models where players offer a mix of software, hardware and services to help farmers improve crop yields, track quality of produce or trace the produce across value chain ► Transaction-based models where players charge based on the number of transactions served such as loans or insurance policies Agritech – offers hope and optimism in catalyzing many unexplored models of rural entrepreneurship. Agritech – a word often used as a substitute for new age innovations in agriculture – offers hope and optimism in catalyzing many unexplored models of rural entrepreneurship. There are at least fifteen such models – which can come alive on the growing agritech prowess. Some of these emerging livelihood models in rural India anchored by agritech models. These models have potential to create millions of jobs in villages, making rural economy robust and pandemic-agnostic. Business models in India’s agritech sector •Margin-based model: Segments such as market linkage – farm inputs, supply chain technology, and output market linkage operate through this model where the agritech player earns a margin by creating marketplace linkages at the input or output side, and by executing the promised services. •Subscription-based model: Agritech players operating in segments like precision agriculture and farm management as well as quality management and traceability offer a mix of hardware, software, and services-based solutions throughout the year and collect monthly or annual subscription charges from their customers. •Transaction-based model: Agritech companies dealing in the financial services segment follow this model based on the number of loans or insurance policies served. 15 AgFoodTech models Farm-gate valuecreators Microwarehousing Digital soil doctors Drone-preneurs Quality assaying as a service (QUAS) Silage stations Mobile picking stations Water management specialists Cooling as a Service (CAAS) Pollination as a Service (PAAS) Vet services at farmer doorsteps Farm equipment management services Management services for Farmer Producer Companies (FPOs) First and Last mile hustlers Insurance agents Agritech provides opportunity to plug several pain points that exist in the agriculture sector at present across the value chain, thereby expanding the market potential. Leveraging technology in India’s agriculture sector can create opportunities for investment through modernizing and introducing systemic efficiencies. How agritech aids productivity and efficiency in India’s agriculture sector •Market linkage – farm inputs: Digital marketplace and physical infrastructure to link farmers to inputs. •Biotech: Research on plant and animal life sciences and genomics. •Farming as a service: Farm equipment for rent on a pay-per-use basis. •Precision agriculture and farm management: Use of geospatial or weather data, IOT, sensors, robotics etc. to improve productivity; farm management solutions for resource and field management, etc. •Farm mechanization and automation: Industrial automation using machinery, tools and robots in seeding, material handling, harvesting, etc. •Farm infrastructure: Farming technologies, such as greenhouse systems, indoor-outdoor farming, drip irrigation, and environmental control, such as heating and ventilation, etc. •Quality management and traceability: Post-harvest produce handling, quality check and analysis, produce monitoring, and traceability in storage and transportation. •Supply chain tech and output market linkage: Digital platform and physical infrastructure to handle postharvest supply chain and connect farm output with the customers. •Financial services: Credit facilities for input procurement, equipment, etc. as well as insurance or reinsurance of crop. •Advisory/ Content: Information platforms online platform for agronomic, pricing, market information. Some ways in which this can be achieved are: •Facilitating input market linkages supported with robust physical infrastructure network can address the existing issues related to volatility in input prices as well sub-optimal input selection. •Precision agriculture can enhance yield by up to 30 percent. •Digitalizing records through farm management can improve operational efficiencies and save costs. •Introducing quality management and traceability will help farmers attain better outcomes in terms of high quality produce, further incentivizing them to continue with modern methods. •Facilitating output market linkages through efficient post-harvest supply chain by eliminating inefficiencies, such as high wastage of farm produce, which is a win-win for both farmers as well as consumers. •Offering better financial services, which could serve 30 percent of farmer households through access to credit, and 65 percent of farmer households through access to crop. Growing segments within agritech in India Out of the total projected agritech market potential of US$24 billion, • The supply chain technology and output markets segments have the highest potential in India, worth US$12.1 billion, • followed by financial services (US$4.1 billion), • precision agriculture and farm management (US$3.4 billion), • quality management and traceability (US$3.0 billion), and • market linkages-farm inputs (US$1.5 billion).