9_AI%20Ethics%20and%20Governance.pdf
Document Details
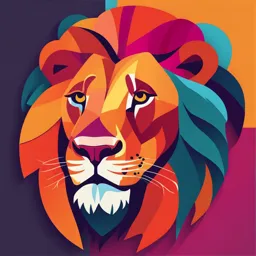
Uploaded by DelightedPolonium
Stanford University
Tags
Related
Full Transcript
AI Ethics and Governance AI for Innovation and Entrepreneurship Tristan Post | Dec 11th, 2023 | TUM ‹#› ‹#› ‹#› ‹#› AI Ethics and Governance 11th December Module Overview The "AI Ethics and Governance" module delves into the crucial aspects of ethical AI development and deployment...
AI Ethics and Governance AI for Innovation and Entrepreneurship Tristan Post | Dec 11th, 2023 | TUM ‹#› ‹#› ‹#› ‹#› AI Ethics and Governance 11th December Module Overview The "AI Ethics and Governance" module delves into the crucial aspects of ethical AI development and deployment. This session is designed to explore how to construct and manage AI in a manner that is responsible, fair, and minimizes harm. It will cover essential concepts such as data privacy, bias, security, and responsible AI product management. The focus will be on establishing processes and developing AI that not only avoids harm but also mitigates it when it occurs. This module offers a hands-on approach to AI ethics, providing practical insights and methodologies to ensure the resilient and ethically aligned deployment of AI. Why Attend This Session This session is indispensable for those who aspire to deploy and manage AI in ways that are ethically sound and resilient. It provides practical insights and approaches to AI ethics, helping students understand how to develop and manage AI that aligns with ethical standards and minimizes harm. Attendees will explore concepts like data privacy, bias, and security, gaining the knowledge to implement responsible AI product management. Whether you are a developer, a manager, or someone interested in the ethical dimensions of AI, this module will equip you with the essential knowledge and skills to navigate the ethical landscape of AI development and deployment effectively. ‹#› AI Ethics and Governance Learning Objectives 1. Understanding of AI Ethics: Gain insights into AI ethics, learning how to develop and deploy AI that is responsible, fair, and minimizes harm. 5. Hands-on Approach to AI Ethics: Receive practical and hands-on insights into AI ethics, preparing to navigate and manage the ethical dimensions of AI effectively. . 2. Knowledge of Data Privacy and Security: Explore the crucial concepts of data privacy and security in AI, enhancing the ability to protect sensitive information and secure AI systems. 3. Insights into Bias and Responsible AI Product Management: Delve into the concepts of bias and responsible AI product management, acquiring the skills to develop AI that is equitable and manages harm effectively. 4. Skills in Ethical AI Development and Deployment: Learn practical methodologies and approaches to ethical AI development and deployment, ensuring the creation of resilient and ethically aligned AI. ‹#› Agenda 1 AI Ethics 4 System 2 Data 5 People & Trust 3 Model 6 ‹#› AI Ethics ‹#› • Values tell us what’s good — They are the things we strive for, desire and seek to protect • Principles tell us whats right — outlining how we may or may not achieve our values • Purpose is our rease for being — it gives life to your values and principles ‹#› ‹#› AI Ethics focuses on the considerations that stakeholders must keep in mind to ensure that artificial intelligence is developed and deployed responsibly. The goal is to avoid causing harm, and this is achieved by adhering to principles of safety security, and environmental sustainability. ‹#› Because AI operates at much larger scale and speed than human, the potential for harm is significantly amplified if things go awry. ‹#› Data ‹#› ‹#› ‹#› ‹#› Data Privacy Compliance with data protection laws and regulation. Focus on how to collect, process, share, archive and delete the data ≠ Data Security Measure that an organization is taking in order to prevent any third party from unauthorized access ‹#› news “The protection of natural persons in relation to the processing of personal data is a fundamental right. Article 8(1) of the Charter of Fundamental Rights of the European Union (the ‘Charter’) and Article 16(1) of the Treaty on the Functioning of the European Union (TFEU) provide that everyone has the right to the protection of personal data concerning him or her.” Personal Data ‹#› ‹#› ‹#› ‹#› ‹#› ‹#› ‹#› ‹#› ‹#› ‹#› ‹#› ‹#› ‹#› Model ‹#› ‹#› ‹#› ‹#› Bias AI bias refers to the systematic erroors or anomalies in the outpur of machine learning algorithms ‹#› Bias ‹#› IN = OUT ‹#› ‹#› Societal/Historical/Structural Bias: This bias arises from societal norms, beliefs, and practices that have historically favoured certain groups over others. It’s embedded in the data we collect often reflecting systemic discrimination or societal inequalities. ‹#› ‹#› ‹#› Statistical/Representation/Sampling Bias: This bias arises when certain groups or characteristics are overrepresented or underrepresented in the training data, leading to skewed results when the model is applied to the broader population. ‹#› ‹#› Measurement Bias: This bias occurs when there are systematic errors in the way data is collected, recorded, or measured, leading to consistent and reproducible inaccuracies in the data. ‹#› Human Errors • Data dame due to network failures • Data stored in wrong place • Data file transportation Human Errors • Data recording mistakes • Wrong data alteration • Deleting files by mistake Malicious Acts • Data damage due to malware, hacking etc. • Cyber data security threats such as data loss, data theft, etc. ‹#› Evaluation Bias: This bias arises when the metrics, datasets, or methods used to evaluate an Al model's performance are skewed or do not accurately represent the broader context in which the model will operate. Aggregation Bias: This bias occurs when data from diverse sources or groups is combined or aggregated without considering the nuances or differences between them. As a result, the aggregated data may not accurately represent any specific group within the broader dataset. ‹#› Deployment Bias (Function/Model Creep): This bias occurs when an Al model is repurposed or deployed in scenarios or applications beyond its original design and intent, leading to potential inaccuracies or unintended consequences. ‹#› ‹#› ‹#› ‹#› ‹#› Model Cards ‹#› Security ‹#› Adversarial Attacks ‹#› ‹#› ‹#› ‹#› ‹#› System ‹#› ‹#› ‹#› Outcome based approach ‹#› ‹#› ‹#› ‹#› ‹#› Human + AI ‹#› Human in the Loop ‹#› Human in the Loop ‹#› Human in the Loop ‹#› People & Trust ‹#› Trust ‹#› ‹#› ‹#› ‹#› Expectation Management ‹#› ‹#› ‹#› ‹#› AI and Society – Social Credit ‹#› Regulation ‹#› ‹#›