5%20AI%20Ethics%20Canvas%20(1).pdf
Document Details
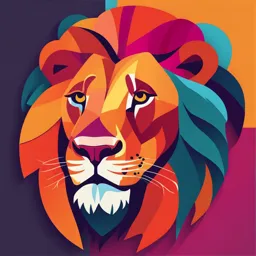
Uploaded by DelightedPolonium
Stanford University
Tags
Full Transcript
AI Ethics and Deployment Resilience Canvas Name: Team: Engaging with this canvas is crucial as it aids in identifying and addressing the myriad of potential risks, challenges, and ethical considerations inherent in AI deployment. It helps in foreseeing and mitigating adverse effects, legal complic...
AI Ethics and Deployment Resilience Canvas Name: Team: Engaging with this canvas is crucial as it aids in identifying and addressing the myriad of potential risks, challenges, and ethical considerations inherent in AI deployment. It helps in foreseeing and mitigating adverse effects, legal complications, security vulnerabilities, and unforeseen repercussions, ensuring the responsible, secure, and beneficial implementation of AI systems. It promotes the alignment of AI deployments with organizational values, societal norms, legal frameworks, and ethical standards, enabling the development of AI solutions that are robust, fair, transparent, and trustworthy. The AI Ethics and Deployment Resilience Canvas is a comprehensive framework designed to guide through a systematic exploration of the multifaceted challenges and risks associated with AI deployment. It covers diverse areas including Data, Model, AI System, People & Organizational Deployment, Society, and Misuse, Security & Unintended Consequences. It provokes critical thinking around legal, ethical, organizational, societal, and security aspects, fostering a deeper understanding of the implications and responsibilities entailed in AI adoption and usage. Data Model Name of Use Case: Stakeholder Mapping: Decision Makers: Users: Affected People: Other Stakeholders: Use Case Description: Date: AI System People & Organizational Deployment Society - Laws, Regulations, and Public Perception Misuse, Security & Unintended Consequences This section revolves around the identification and mitigation of possible misuse, security vulnerabilities, and the unintended repercussions of AI, emphasizing the protection of system integrity and responsible use of technology to avoid any harm or disruption to individuals or society. This section examines the various aspects of data, focusing on its quality, integrity, privacy, and relevance, to ensure the responsible use and management of data in AI systems. This section delves into the methodologies and approaches used in model development, validation, interpretation, and addressing biases, emphasizing transparency, fairness, and robustness in AI model development and deployment. This section explores the comprehensive and holistic aspects of the AI system, focusing on its integration, performance, security, monitoring, and the guidance needed for responsible and effective deployment and operation in real-world settings. This section delves into the human and organizational aspects of AI deployment, addressing user acceptance, influence dynamics, and strategies for smooth initial deployment within the organizational context, while emphasizing trust and harmony. This section evaluates the societal implications of AI, focusing on adherence to legal norms, management of intellectual property, public perception, and organizational reputation, ensuring alignment with societal values and legal frameworks. Proper understanding and management of data are crucial as it is the foundation of AI systems. The integrity, privacy, and representativeness of data have a direct impact on the effectiveness and reliability of AI applications. Completing this section ensures responsible data practices, compliance with regulations, and the mitigation of potential data-related risks. The model is the core of an AI system, and its reliability, fairness, and transparency are crucial for the successful and responsible deployment of AI. Filling out this section ensures that the model is developed and validated rigorously, biases are addressed, and its workings are transparent and understandable to all stakeholders. Understanding and addressing the holistic aspects of the AI system, beyond model-specific considerations, are crucial for responsible AI deployment. This section ensures that the deployed AI system is integrated effectively, performs reliably, is secure, and can be monitored and improved continually, aligning with user needs and organizational goals. Addressing this section ensures an inclusive and comprehensive deployment plan that considers the human and organizational dimensions, fostering an environment where AI can be trusted, accepted, and used effectively. The emphasis is on harmonious introduction and operation of AI systems with organizational dynamics and human aspects. Addressing this section is vital for maintaining lawful and ethical AI deployment, balancing societal expectations, and upholding organizational reputation. It aids in foreseeing and managing the societal impacts of AI on external stakeholders, mitigating risks, and fostering a harmonious relationship between AI technology and societal norms. System Integration and Compatibility User Acceptance and Trust Legal and Regulatory Compliance Data Quality, Relevance, and Integrity Model Development and Validation Assesses the accuracy, reliability, and relevance of the data, ensuring it is suitable for the AI application. How do you ensure the data is accurate, reliable, and relevant for the intended use? Is the data periodically reviewed and updated to maintain its integrity and relevance? Explores the methodologies used for developing and validating the model, ensuring its accuracy and reliability. How is data completeness and consistency maintained across the dataset? How are anomalies and inconsistencies within the data identified and rectified? What steps are taken to ensure the continuous relevance and quality of the data as it evolves over time? This involves evaluating and enhancing the user's willingness to accept and trust the AI system, addressing potential stigmas and resistances. Is the AI system in compliance with all relevant local and international laws, including GDPR and AI Act? How might internal or external entities potentially misuse or abuse the AI system, and what could be their motivations? How are the model's accuracy and reliability tested and validated before deployment? Have you encountered any compatibility issues during system integration, and how have these been addressed? What strategies are employed to maintain transparency and proactive communication to assuage user concerns and mitigate resistance or stigma related to the AI system? Under which risk class does the AI system fall according to the AI Act, and what are the implications for compliance requirements? What mechanisms are in place to detect and respond to such misuses promptly? Have you utilized appropriate validation techniques to prevent overfitting and underfitting? Is there a continuous validation process in place to monitor the model's performance post-deployment? How is the AI system's deployment (on-premise, cloud, or hybrid) aligned with organizational needs, policies, and constraints? How is user feedback regarding trust and acceptance collected and incorporated into the deployment strategy? What are the implications of the deployment choice for system accessibility, scalability, security, and compliance? How is Human in the Loop (HITL) integrated into the AI system to ensure user input and intervention in decision-making processes? How are system integrations tested and validated to ensure seamless interoperability? How is compatibility with existing infrastructure maintained as the system evolves? Data Privacy and Security Focuses on the model's ability to be understood and interpreted correctly by various stakeholders. How is the model's decision-making process made transparent and understandable to non-technical stakeholders? How are data protection and privacy communicated to the data subjects? How are data subjects informed about the data collection, processing, and storage practices, and is their consent obtained where necessary? What techniques are utilized to enhance the model's interpretability and explainability? Who has access to the data, and how are access rights managed and reviewed? How are the limitations and potential inaccuracies of your model communicated to stakeholders or end-users proactively? How are data breaches detected and addressed to prevent data compromise? How are the model's outputs justified and validated to ensure trustworthy AI? How is personal data handled, anonymized, or pseudonymized to comply with data protection regulations? Are model cards, or similar documentation, developed to provide comprehensive information about the model’s purpose, performance, and limitations? How are different categories of data, based on sensitivity levels like public, internal-only, confidential, and restricted, identified and managed within the system? How does HITL contribute to enhancing the reliability, accuracy, and user acceptance of the AI system’s outputs? How are model cards utilized to communicate model characteristics and behaviors to different stakeholders? What specific measures are being implemented to ensure compliance with the AI Act, especially if the model falls under a higher-risk category? How is ongoing legal compliance monitored and ensured? How are risks of misuse of personal and sensitive data mitigated, especially when the AI system interacts with external entities or public platforms? Are there specific scenarios envisioned where the AI system’s outputs can be misinterpreted or manipulated, and how are such scenarios addressed? How is compliance with emerging laws and regulations ensured as the legal landscape evolves? How are legal and compliance risks assessed and mitigated proactively? What mechanisms are in place to facilitate effective interaction and feedback between humans and the AI system, and how is this feedback utilized to improve system performance? Intellectual Property and Usage Rights System Performance and Reliability Model Explainability and Interpretability What measures are in place to protect data from unauthorized access and disclosure? Analyzes the possible intentional misuse or abuse of the AI system by internal or external actors and assesses motivations behind such actions. What are potential sources of resistance or stigma from users and how can they be identified and addressed to facilitate user acceptance and trust in the AI system? What criteria are used to select appropriate validation techniques for the model? Is the dataset compliant with data protection laws such as GDPR, and how is compliance maintained? Assesses conformity with applicable laws and regulations, emphasizing ongoing compliance with legal frameworks like the AI Act. How is the AI system integrated and made compatible with existing systems and infrastructures? How are the models periodically reassessed and recalibrated to maintain their accuracy and reliability? Considers how data is safeguarded and how privacy is maintained, focusing on compliance with data protection regulations like GDPR. Potential Misuse and Abuse How are the methodologies chosen for model development and validation aligned with the objectives of the AI system? How is human oversight incorporated during model development and validation to ensure the model's accuracy, fairness, and reliability? Are there processes in place to address and rectify inaccuracies in the data? Investigates how the AI system integrates and aligns with existing systems and infrastructures. Addressing this section is fundamental for maintaining the responsible and secure use of AI, avoiding disruptions, and ensuring technology-induced changes are beneficial and do not harm individuals or society. It promotes ethical conduct, security, and well-being by acknowledging and addressing potential pitfalls and adverse alterations induced by AI deployment. Explores the reliability and performance of the AI system under different conditions and workloads. How is the performance of the AI system measured, and how does it perform under varying conditions and workloads? Are there any established performance benchmarks, and how does the system align with them? Influence Dynamics and Initial Deployment Strategies This focuses on identifying and engaging with key influencers and formulating strategies for successful initial deployment. How are key influencers and worker councils identified and engaged to facilitate the smooth adoption of the AI system and to model positive interactions with the system? How is system reliability ensured, and what mechanisms are in place to address system downtimes or failures? What strategies and plans are in place for the initial deployment or pilot testing to address potential concerns and validate system performance? What are the potential repercussions of system performance issues, and how are they mitigated? What criteria are being used to determine the optimal conditions and scope for a pilot deployment of the AI system? How is the AI system tested in real-world conditions to ensure its reliability and performance meet the predefined criteria? How will the outcomes of the pilot phase inform the subsequent full-scale rollout of the system? What mechanisms are in place to address any discrepancies or issues identified during field testing promptly? What strategies are in place to ensure a smooth rollout of the AI system post-pilot phase? How are the potential consequences of incorrect predictions or errors by the AI system analyzed and addressed? How will feedback and learnings from initial deployment stages be incorporated to refine rollout strategies? What proactive measures are implemented to minimize the risks and impacts of inaccurate outputs and predictions on users and the organization? How does product management ensure that the AI system is aligned with user needs, organizational goals, and market demands throughout its lifecycle? Security Vulnerabilities and Adversarial Attacks Addresses the legalities concerning the utilization and protection of data and the outputs produced by the AI system. How are the intellectual property and usage rights of the AI-generated data and outputs managed and protected? Investigates potential security gaps and the risk of attacks that could affect the system’s functionality and safety. Are all legal aspects pertaining to intellectual property clearly documented and resolved? How are open-source components utilized within the AI system managed to avoid intellectual property and copyright infringements? What measures are implemented to ensure compliance with the licenses of the incorporated open-source components? How are potential security vulnerabilities identified and addressed to protect the system against (adversarial) attacks? What proactive measures are implemented to secure the system and its users from potential breaches? How are copyright issues, especially related to open-source components and third-party content, identified, addressed, and documented to avoid legal complications? How does the organization ensure that the use of copyrighted material complies with applicable laws and does not infringe on the rights of the copyright holders? What mechanisms are in place to monitor and manage copyright compliance continuously, especially in the context of evolving legal frameworks and organizational needs? What processes are established by product management to oversee the development, deployment, and maintenance of the AI system effectively? Unintended Consequences and Repercussions Model Bias and Fairness Data Bias and Representativeness Evaluates the unforeseen impacts and societal changes due to AI deployment, such as behavioral alterations or ethical dilemmas. Examines the presence of biases in the model and measures taken to address them to ensure fairness in model outcomes. Analyzes the diversity and representativity of the data to mitigate biases and ensure fairness in AI outputs. How do you ensure that the data is representative of various demographics and is free from biases? Have inherent biases in your data that could affect model predictions been identified and mitigated? How are potential biases in the model addressed and mitigated during development? What mechanisms are in place to detect and rectify any biases in the data? How are biases addressed at each stage to ensure fair and unbiased model outcomes? How is diversity within the dataset maintained, and how are underrepresented groups included? Is there ongoing monitoring for bias in model outcomes, and are adjustments made as needed? Have potential biases and their impacts been assessed and documented? What mechanisms are in place to receive and address feedback regarding model bias and fairness? How is model fairness validated across diverse demographic groups? Public Perception and Reputation System Security and Adversarial Defense Examines the defenses in place to secure the AI system against malicious attacks and unauthorized access. What security measures are implemented to protect the AI system from adversarial attacks and unauthorized access? How is the AI system’s resilience against malicious inputs and attacks tested and ensured? How are security vulnerabilities identified, addressed, and communicated to relevant stakeholders? How are security incidents managed, and what protocols are in place for incident response and recovery? Explores how data is managed throughout its lifecycle, focusing on maintaining its quality, security, and compliance from acquisition to disposal. Model Robustness and Generalization Training and Communication How are the benefits and value propositions of the AI system communicated to the public to foster understanding and acceptance? Centers on communicating effectively and providing necessary training to foster trust and ensure proper usage of the AI system. How is the model's robustness tested against variations in input and environmental conditions? How are data handling, storage, and disposal practices documented and communicated within the organization? How is data lifecycle management reviewed and improved to maintain compliance and security? Is there an established procedure for the secure and compliant disposal of data? How is the model's ability to generalize learning to unseen data evaluated and ensured? What structured training and communication plans are in place to foster user trust and ensure proper usage of the AI system? What mechanisms are in place to receive and address inquiries, concerns, or feedback from external stakeholders or the public regarding the AI system? How are the training needs of different user groups identified and addressed to ensure effective use of the AI system? Is there a strategy in place to address public perception and mitigate potential negative impacts on organizational reputation related to AI use? How are the effectiveness and impact of training and communication initiatives measured and improved upon? How is public feedback on AI deployment considered and integrated into system refinement? How are changes in system functionality communicated to end-users? If details of the AI system were to be featured in a newspaper, how would the organization ensure that all aspects would be perceived positively by the public? Develops strategies and response mechanisms to prevent misuse and manage the ramifications of security incidents effectively. What strategies are developed to prevent the misuse and unintended consequences of the AI system? Responsibility and Accountability How is the AI system monitored for performance, errors, and user feedback? What maintenance activities are performed to ensure the system's continued reliability and performance? Addresses the clear assignment of responsibility and accountability to manage the AI system effectively within the organizational context. How are responsibility and accountability for the AI system’s deployment and management clearly defined and assigned within the organization? What immediate response mechanisms are in place to mitigate harm in the event of incorrect outputs or system errors? How does the organization plan to manage and rectify the impacts of mistakes made by the AI system on end-users and stakeholders? Consultation and Guidance How does the organization plan to manage any potential negative publicity or reputational damage arising from the deployment of the AI system? Assesses the strategies and procedures for ongoing system monitoring, maintenance, and improvement. How are system updates and improvements communicated to users and other stakeholders? How are the model’s limitations and potential weaknesses identified, communicated, and addressed? How effective are the response and mitigation plans in place in the event of security breaches or system misuse? Responsibility and Accountability What are the contingency plans in place to address any instances of unauthorized access, misuse of data, or other security breaches promptly and effectively? Defines responsibility in the event of system malfunctions or failures and establishes clear accountability for maintaining standards and legal compliance. What mechanisms are in place to ensure that responsible parties are accountable for the proper functioning and outcomes of the AI system? How are incidents and issues related to the AI system escalated and resolved, and who is accountable for addressing them? Consultation and Guidance Identifies and consults experts for insights and guidance on model development, biases, and ethical considerations. Have robust response strategies been developed to promptly address any malfunctions or compliance failures? Consultation and Guidance Consultation and Guidance Identifies and consults experts for insights and guidance on system development, deployment, and management. How are users educated and informed about the appropriate and responsible use of the AI system to prevent unintentional misuse or harm? Who is designated as responsible and accountable for addressing any legal, compliance, or reputational issues arising from the AI system? Consultation and Guidance Identifies and consults with experts for guidance on addressing data-related challenges, compliance, and best practices. How does the organization respond to and rectify any adverse impacts arising from mistakes made by the AI system on individuals or communities? Prevention and Mitigation Strategies How is feedback on system performance and errors integrated to improve the system continually? Is there a mechanism in place to adapt the model to evolving data patterns and changing environments? How are potential unintended consequences of errors or wrong predictions by the AI system identified, assessed, and mitigated? Should the use of AI solutions be proactively disclosed to external stakeholders, and if so, how is this disclosure managed to balance transparency and strategic interests? System Monitoring and Maintenance Assesses the model's ability to generalize learning and perform reliably under various conditions and input variations. What policies and procedures are in place to govern the data throughout its lifecycle? Are there mechanisms in place to continuously monitor and mitigate unforeseen negative impacts after deployment? Explores the potential public opinions and their impact on the organization's standing, considering proactive measures to maintain a positive image. How is ongoing support and training provided to users to ensure effective use of the AI system? Data Governance and Lifecycle Management What are the conceivable unintended consequences of deploying the AI system, and how are they being addressed? Seeks advice or insights from relevant experts on organizational deployment and user acceptance. Who within or outside the organization can provide guidance on data-related challenges and compliance? Who can provide insights or guidance on model development, bias mitigation, and ethical considerations within or outside your organization? How are consultations with data experts conducted, and how is their advice implemented? How are consultations with modeling experts conducted, and how is their advice implemented in the model development process? Who can provide insights or guidance on system development, deployment, and improvement within or outside your organization? Who are the experts within or outside the organization that can provide insights and guidance on addressing user acceptance and organizational deployment challenges? Are there regular consultations to stay abreast of changes in data-related laws and best practices? How are insights and advice from experts documented, communicated, and integrated to ensure responsible model development? How are consultations with system experts conducted, and how is their advice implemented in the system development and management process? How are the insights and advice from these experts incorporated into deployment strategies to address challenges effectively? How are insights from consultations communicated and applied across the organization? How often are consultations conducted to ensure the model's alignment with best practices and evolving standards? How are insights and advice from experts documented and integrated to ensure responsible system management? How frequently are consultations conducted to review and refine deployment strategies based on expert insights and changing organizational needs? Identifies expert advice and internal support structures aimed at addressing misuse, reinforcing security, and managing unintended consequences. Consultation and Guidance Seeks expert advice on the legal, regulatory, and public perception aspects related to AI to refine deployment strategies. Who are the experts consulted for advice on legal, regulatory, and public perception matters related to AI deployment? Who are the go-to experts or entities, internally or externally, for advice and guidance on misuse and security concerns? How is consultation with experts integrated into the strategy to strengthen security and address potential misuse? How are the insights from consultations used to adjust deployment strategies to evolving legal and societal considerations? How often are consultations conducted to ensure the system’s alignment with best practices and evolving standards? Assess the overall risk related to that specific area, rating it on a scale from 1 to 5, with 1 representing the lowest risk and 5 representing the highest. Reflect upon all the elements and discussions within the section to determine this overall risk score. Following the rating, list the top identified risks in that section, focusing on the ones with substantial potential impact and higher likelihood of occurrence. Data Risk Assessment Rate the overall risk of this section on a scale from 1 to 5. (1-Lowest, 5-Highest) Overall Risk Rating: List the Top Identified Risks: 1 Model Risk Assessment 2 3 4 5 Rate the overall risk of this section on a scale from 1 to 5. (1-Lowest, 5-Highest) Overall Risk Rating: List the Top Identified Risks: 1 2 AI System Risk Assessment 3 4 5 Rate the overall risk of this section on a scale from 1 to 5. (1-Lowest, 5-Highest) Overall Risk Rating: List the Top Identified Risks: 1 2 People & Org. Risk Assessment 3 4 5 Rate the overall risk of this section on a scale from 1 to 5. (1-Lowest, 5-Highest) Overall Risk Rating: List the Top Identified Risks: © Tristan Post (2023), [email protected] 1 2 3 Misuse Risk Assessment Society Risk Assessment 4 5 Rate the overall risk of this section on a scale from 1 to 5. (1-Lowest, 5-Highest) Overall Risk Rating: List the Top Identified Risks: 1 2 3 4 5 Rate the overall risk of this section on a scale from 1 to 5. (1-Lowest, 5-Highest) Overall Risk Rating: List the Top Identified Risks: 1 2 3 4 5