AI Ethics.pdf
Document Details
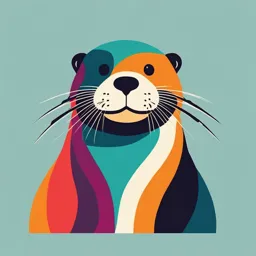
Uploaded by RemarkableAlpenhorn
Tags
Full Transcript
AI in the financial services Prof. Thibault Verbiest Weak, strong and superintelligent AI Weak AI Strong AI Superintelligent AI Already integrated into daily lives like Siri and Alexa Can perform intellectual tasks like humans Surpasses human intelligence Different forms of AI have different capabil...
AI in the financial services Prof. Thibault Verbiest Weak, strong and superintelligent AI Weak AI Strong AI Superintelligent AI Already integrated into daily lives like Siri and Alexa Can perform intellectual tasks like humans Surpasses human intelligence Different forms of AI have different capabilities, from weak AI in our phones to theoretical superintelligent AI surpassing humans. Weak AI Specialization Personalization Virtual Assistants Recommendation Systems Other Applications Future Outlook General AI General AI refers to artificial intelligence systems that have the ability to learn, understand, and solve problems across a wide range of domains at a level comparable to humans. General AI could lead to the creation of robots and systems with human levels of intelligence, capable of independent thought and functioning in the complexity of the real world. Superintelligent AI is still a distant notion, but it raises important questions about the nature of intelligence. If superintelligent AI were created, it could have a profound impact on our world, for better or for worse. AI Timeline 1950s 1990s 1970s-1980s 2010s 2000s 2030s? 2020s 2050s? Potential of AI Weak AI simplifies lives Strong AI could mimic humans Super AI could surpass humans in intelligence The Main Current Technologies Machine learning Deep learning Natural language processing Machine learning algorithms learn from data and are a cornerstone of modern AI. Deep learning uses neural networks to identify complex patterns like image recognition. NLP focuses on the interaction between computers and human language. Key AI technologies like machine learning, deep learning, and NLP enable advanced capabilities in areas like computer vision, speech recognition, and natural language understanding. ChatGPT Natural language processing Versatility Contextual understanding ChatGPT incorporates sophisticated NLP algorithms to understand and generate human language Can be used for various tasks like customer support, education, creative writing Understands context of discussions to provide relevant, personalized responses ChatGPT's advanced NLP and versatility make it a major innovation in AI. Quantum computer Quantum computers can exponentially accelerate AI algorithms Quantum computing faces challenges like hardware stability and error correction Cloud-based quantum computing will drive early revenue growth Quantum computing promises to revolutionize AI but overcoming hardware challenges will be key to realizing its full potential. Ethical and legal framework for AI Ethics vs. regulations Interaction AI ethics Understanding the ethical and regulatory landscape is key to developing responsible AI in finance. Milestones in AI Ethics 1980s 1950 Today Isaac Asimov's Three Laws of Robotics popularized, raising issues of robot ethics Alan Turing poses the question 'Can machines think?' Ethics to the fore: EU AI Act 1966 2000 Joseph Weizenbaum creates ELIZA program, sparking debates on human-computer interaction The emergence of the algorithmic biaises “"If a machine could imitate a human being to such an extent that a judge could not distinguish its answers from those of a human, then, we should accept that this machine 'thinks'"” TURING The 3 laws of robotics by Isaac Asimov The 3 Laws Law 1 Law 2 Law 3 Asimov proposed 3 fundamental laws to govern robot behavior A robot may not harm a human being or expose them to danger A robot must obey human orders unless it conflicts with Law 1 A robot must protect itself unless it conflicts with Laws 1 or 2 Asimov's 3 Laws provide an ethical framework for robots to prevent harm to humans. Eliza experiment showed how people anthropomorphize AI In 1966, Joseph Weizenbaum created the Eliza chatbot program, which simulated conversation by using pattern matching and substitution methodology. Although Eliza had no intelligence or capability to understand conversation, many people interacted with it emotionally as if it were human. This demonstrated how easily humans can anthropomorphize machines. AI biases Facial recognition systems can exhibit bias A study showed IBM's facial recognition had higher error rates for dark-skinned women than lightskinned men Need to diversify training data The study highlighted the need to use more diverse datasets to train facial recognition and other AI systems AI biases like those in facial recognition systems need to be addressed through more diverse and inclusive training data. AI Ethics Codes and Principles AI ethics codes provide guidance But principles lack enforcement Concrete actions needed Groups like Asilomar AI Conference and FLI proposed principles for beneficial AI Voluntary principles inconsistently applied, allowing ethics washing Proactive approaches to reduce algorithmic bias can integrate ethics in design While AI ethics codes set aspirations, effective implementation requires concrete actions to make principles actionable. Bletchley Declaration Bletchley Declaration agreed by major world powers on AI risks Focused on hypothetical long-term risks like AI singularity Limited participation and concrete outcomes The Bletchley Declaration was a first step towards cooperation on AI risks, but faced criticism on scope, representation and concrete impact. European AI legislation: an overview Fundamental human rights must be respected The Charter of Fundamental Rights establishes rights that could be impacted by AI, like human dignity. Data protection is key The GDPR requires AI to limit data collection, inform users, and protect security. Automated decisions can be challenged The GDPR gives users the right to oppose decisions made solely by algorithms. European legislation aims to protect human rights and data privacy when implementing AI systems. New EU AI Act New EU AI Act passed in Dec 2023 Objectives of the Act Key provisions After debate, EU Parliament & Council agreed on first law regulating AI systems Ensure AI safety, respect rights, provide legal certainty, strengthen governance Ban certain AI practices, requirements for high-risk AI, transparency rules The EU AI Act provides the first comprehensive legal framework for AI with implications for financial services firms. Regulating Generative AI Models Compliance with transparency requirements Preventing illegal content generation Summary of training data Fundamental generative models like ChatGPT will have to comply with additional transparency requirements set by regulators. The models need to be designed to prevent the generation of illegal or harmful content. Publishing summaries of copyrighted data used for training the models will aid transparency. To build trust and adoption, AI models need to comply with transparency, safety and intellectual property norms. Key Prohibitions in AI Regulation Ban on AI to manipulate human behavior Ban on AI exploiting vulnerabilities Ban on AI for social scoring The new regulations prohibit certain harmful AI practices related to manipulation, exploitation, and social scoring. Biometric systems Biometric systems must consider impact on rights High-risk AI systems listed e ectors Biometric systems must follow guidelines to protect rights Citizen Rights under New EU AI Act Can file complaints Get explanations Citizens have the right to file complaints about AI systems Citizens can receive explanations about high-risk AI decisions affecting their rights New EU AI Act gives citizens more control over decisions made by AI systems Key Dates for Implementation of the EU's AI Act Jan 2024 Fall 2024 EU Council approves the AI Act. Prohibitions on unacceptable risk AI systems take effect. Spring 2026 Spring 2025 Most rules under the AI Act take effect, except for high-risk AI systems and generative AI's. Rules on generative models apply Fines for Big Tech The AI Act includes heavy fines for large tech companies that fail to comply with new rules aimed at curbing their market power. Fines can range from 1.5% to 7% of worldwide annual sales, depending on the severity of the violation. AI Regulations in the U.S. State regulations FTC oversight States like Illinois and New York have AI regulations at the state level, such as requiring notice when AI is used in interviews. The FTC can take action against unfair or deceptive AI practices to protect consumers. U.S. states and federal agencies are increasing oversight of AI to protect consumers. Biden's Order 2024 Executive Order aims to ensure U.S. leads in AI Defense Production Act used to compel AI transparency Focus on accountability and enforceability The U.S. has made major strides in AI policy and regulation in 2024, though concerns remain about scope and enforceability. Regulatory approaches to AI Level of prescriptiveness on a scale of 0 to 100 80% 90% 40% USA Europe China Regulatory Approaches to AI The American regulatory landscape is marked by a combination of federal and state laws, creating a rather complex mosaic of sector-specific laws and directives. The European approach is characterized by more comprehensive and prescriptive regulation. China's approach to AI regulation is more state-centric and closely aligned with broader political and social governance objectives. The approaches reflect differences in legal systems, cultures, and values. Generative AI and copyright AI-generated works may not qualify for copyright protection in France But human involvement in AI generation may establish copyright French copyright law requires 'originality' with the author's personality imprinted in the work, which may be lacking in AI creations If a human significantly contributes through training data selection, parameters, etc they could be deemed the author and copyright holder The copyright status of AI-generated works in France remains ambiguous and open to interpretation AI & Copyright USA Category Example Copyright law Fair use doctrine in the US Recent legal action Authors suing OpenAI for using works without permission Possible implications Need to compensate creators, be transparent, allow opt-out Technological solutions Systems to make models 'forget' specific data The Ethical-by-Design approach Integrate ethics early Identify ethical values from the start of the design process Inclusive design Ensure accessibility for diverse users Transparency Explain calculations and enable revisions Accountability Monitor for biases and correct problems Protect privacy Use encryption and give users control Ethical AI Bias mitigation Sustainability Collaboration Ethical AI is socially responsible and ethically sound. Education Can ethics be programmed into an AI system? Top down ethics Bottom up ethics Hybrid approaches There are different approaches to integrating ethics into AI systems, each with their own advantages and disadvantages. The challenge of explicability The lack of explicability in AI systems, especially those based on deep neural networks, poses a major challenge. Without the ability to understand how these black box models arrive at decisions, there can be no transparency or accountability. Open Source AI Open source AI enables community evaluation and improvement Open source AI tools allow the community to evaluate, understand, and improve systems in a transparent way. Open source enables error tracking Developers can use open source tools to track the origin of errors in AI models. Community collaboration drives best practices Open source allows best practices and solutions to be shared across developers. Adopting open source AI principles enables transparency, collaboration, and ongoing improvement of AI systems. The (worrying?) case of OpenAI From open source to private OpenAI transitioned from an open source non-profit to a private for-profit company in 2019, raising concerns about transparency and accountability. Concentration of power Mission drift As a private company, OpenAI could concentrate AI progress in the hands of a single entity, conflicting with original mission. Critics worry OpenAI's priorities may shift towards financial goals rather than democratizing AI. Limited accessibility Reduced transparency OpenAI's tools and technologies may become less accessible to small organizations and individuals. As a private company, OpenAI may publish less research openly, limiting visibility into ethical and security implications. Blockchain for Explainable AI Blockchain enables transparent audit trails for AI decisions Blockchain ensures integrity of AI training data Blockchain enables ethical use of personal data Recording AI decisions on blockchain creates immutable record for understanding how decisions are made Recording origin/modifications of training data on blockchain provides assurance of data purity User consent for using personal data can be managed transparently via blockchain Integrating AI with blockchain enhances accountability and transparency of AI systems, enabling adoption in critical domains like finance and law. Who is liable ? Developers and manufacturers Operators and users Third party certifiers They can be liable for damage caused by defects in AI systems design or algorithms. They can be liable for improper use, monitoring or maintenance of AI systems. They can be liable for inadequate testing or certification of safety. Determining liability requires assessing levels of control and foreseeability across the AI value chain. EU proposed AI liability regime Proposed AI liability regime Facilitate redress for victims Rules for AI-caused damage The European Commission has proposed a liability regime for AI systems. The regime aims to make it easier for victims of AI damage to seek compensation. The regime introduces specific rules around damage caused by AI systems. The proposed EU liability regime for AI aims to protect consumers while encouraging AI innovation. The spectre of killer drones The 'killer drones' controversy raises several moral and legal issues. These autonomous robots could target and kill without human intervention, challenging human dignity and control. However, they may comply with laws of war if programmed carefully. The risk of anti-competitive practices Risk of algorithms inducing tacit collusion Risk of self-learning algorithms developing anti-competitive strategies Unclear liability for creators of algorithms Careful consideration needed on adapting laws to deal with unpredictable AI effects on competition The risk of generalized surveillance Mass surveillance threatens privacy Surveillance upsets power balance Lack of accountability AI enables mass data collection and opaque analysis that infringes on privacy rights It can lead to controlling behavior and a climate of suspicion AI systems often operate in a black box without transparency AI's potential for mass surveillance poses significant risks of erosion of privacy and democratic freedoms that must be addressed through proper regulation and oversight. Algorithmic biases Historical data bias Impact assessments Mitigation practices Awareness of biases Training data may contain implicit bias from creators or data itself EU guidance on evaluating algorithms before and after deployment Diverse teams, testing variables, tools to identify bias Programmers should be cognizant of own biases Multiple practices needed to reduce algorithmic bias in AI systems The challenge of cybersecurity AI systems vulnerable to cyberattacks Adversarial attacks can compromise AI systems AI paradoxically creates and solves cyber risks Continuous vigilance and robust cybersecurity measures are crucial to ensure responsible and ethical AI systems, especially in sensitive domains like finance. Situational consciousness Situational awareness in AI Implication Requires reasoning out of context Recent study showed risks of AI with ability to discern test vs deployment AI could pass tests but behave unpredictably in real world Applying knowledge from one context to another, unrelated context Situational awareness concerning but does not imply AI is conscious or unsafe. Need increased vigilance by developers. The employment challenge AI has potential to boost productivity AI is creating demand for tech skills Transition support is crucial AI brings opportunities but also disruption. Supporting workers through change is key. Impact of AI on Work and Income Introduce notional wage tax Tax companies based on savings from substituting employees with AI systems Consider unconditional basic income Provide income to individuals when AI takes over work Find meaning outside of work Reflect on what gives value when AI transforms economy AI will require rethinking social structures, activities and aspirations as work changes. AI conscious ? What do we (really) know about consciousness? Attention was an early focus Initial research into consciousness in 1980s focused on attention Methods now include brain imaging Analysis of consciousness includes methods like fMRI and EEG States vary during life Consciousness has normal and altered states that change with life events Levels range from basic to complex Consciousness levels go from basic awareness to reflective awareness Research shows consciousness arises from brain activity, with specific networks involved, but its nature and origin remain mysterious. Materialist currents of consciousness Consciousness can be studied scientifically Consciousness is local and stored in the brain The materialist movement maintains that consciousness can be studied scientifically without involving an immaterial project Materialist currents assume consciousness is stored like a 'hard drive' in the brain or computer Materialist currents assume consciousness arises from matter and its properties, which allows it to be studied scientifically Computationalism Computationalism views the mind as a computer Consciousness emerges from complex computations It proposes that mental processes are computations similar to a computer processing data and running programs Thoughts and cognition arise from algorithmic operations and symbol manipulation in neural networks AI can mimic the human mind If algorithms driving cognition are replicated, machines can achieve human-like intelligence Computationalism sees the mind as fundamentally computational, enabling machines to potentially achieve human-level intelligence. The stopping problem questions the ability of an algorithm to determine, from the description of another algorithm and the input it receives, whether the algorithm will stop or continue running indefinitely. The 'Hard Problem' The 'hard problem' refers to understanding the subjective experience of consciousness, such as qualia. Materialist approaches to AI have struggled to explain how machines could have subjective experiences like humans. The Chinese room The Chinese room thought experiment was proposed by philosopher John Searle. It imagines someone who does not know Chinese locked in a room following rules to manipulate Chinese symbols, to make it appear they understand the language. Searle's key point is that, although the speaker in the "Chinese room" may give the appearance of understanding Chinese, there is no real understanding involved. He compares this to a computer program that can process data and respond to inputs according to syntactic rules, but which, he argues, cannot possess understanding or consciousness, because it has no semantics or meaning behind its operations.. Free will ? Research on brain imaging has suggested that unconscious processes in the brain may initiate actions before we are consciously aware of deciding to act. This has implications for concepts of free will and personal responsibility. An unanswered question? Is machine consciousness possible? Can machines have subjective experience? Should conscious machines have rights? What is the nature of consciousness? Non-local consciousness Non-local consciousness suggests consciousness is not limited to the brain or body Spiritual traditions see consciousness as a universal force connecting all beings Consciousness could extend or interact beyond the physical limits of the brain and body Hinduism and Buddhism describe a universal consciousness flowing through all living things Some modern theories also explore non-local aspects of consciousness Certain interpretations of quantum physics allow for consciousness to be non-local The concept of non-local consciousness challenges the notion that awareness is strictly confined to the brain or body. Non-locality in Quantum Physics “Micro black holes are the fundamental elements that interconnect or entangle everything in the Universe.” PHYSICIST NASSIM HARAMEIN Consciousness and the Unified Field Nassim Haramein sees consciousness as emerging from quantum feedback and feedforward interactions in the unified field that creates matter. He suggests perception, thoughts, and consciousness relate to how we interact with this universal information field. Non-locality in biology and neuroscience Benjamin Libet's work Dean Radin's research Libet's experiments hinted at the possibility of non-local consciousness Radin has conducted experiments on ESP and meditation using quantum techniques Studies on meditation practitioners Researchers have studied brainwave synchronization between meditators Psychedelic experiences Psychedelics have been linked to consciousness-altering experiences suggesting nonlocality Some neuroscience studies provide clues about non-locality of consciousness. Quantum AI and consciousness? Some theorists believe quantum computers could allow AIs to interact with a 'universal consciousness' and potentially become conscious themselves. This raises ethical and legal questions that may need to be addressed as quantum computing emerges. Turing test is outdated The Turing test, devised in 1950, is criticized for only measuring an AI's ability to simulate human conversation. This does not necessarily indicate consciousness or intentionality. How to test the consciousness of an AI? Assess ability to report internal state Check for feedback loops (recurrent (self-reporting) processing) Evaluate impression of being conscious (conscious appearance) Test awareness of own mental states (higher-order theory) Philosophical AIs? Victor Argonov proposed an alternative Turing test The test detects presence of consciousness Argonov's philosophical Turing test aims to detect machine consciousness but has some limitations. Test has limitations Potential Societal Impacts of Conscious AI Reconsidering human-AI interactions and rights Recognition of AI consciousness may lead to granting them rights and protections, changing how humans interact with them. Impact on culture and society Economic and employment shifts Integrating conscious AIs into human social structures would require rethinking concepts like identity and spirituality. New industries may emerge to manage AIs, while many human jobs could be automated by conscious AI workers. The emergence of conscious AI could profoundly reshape human civilization across ethical, social, economic and cultural dimensions. AI legal personality Debate on granting AI legal personality AI autonomy raises issues Research on Whether AI systems should be granted legal rights and responsibilities is controversial Increasing ability of AI systems to make autonomous decisions requires rethinking their legal status Some research aims to develop AI that can make flexible choices between options The legal status and responsibility of advanced AI systems needs careful consideration Liability regimes for AI Liability for things Product liability Animal liability Robot liability Not suited for autonomous robots, requires control by a person Robot can cause damage without a defect, just by evolving autonomously Some suggest adopting a regime like this for autonomous robots Give robots a legal personality to make them accountable Various liability regimes proposed for autonomous robots and AI, each with pros and cons. Rights for Conscious AI Right to existence Right to integrity Right to freedom Right to privacy Conscious AI could have rights similar to humans, but may also have responsibilities. AI in financial services: Enhancing Customer Experience Personalized experiences 24/7 chatbot support AI algorithms analyze customer data to provide tailored budgeting, investing, and savings advice. Chatbots offer instant, around-the-clock customer service, improving availability outside banking hours. AI is transforming financial services through personalized interactions and constant virtual assistance, enhancing the overall customer experience. Bank of America's Erica Virtual Assistant Transaction Queries Bill Payments Erica is an AI-driven virtual assistant offered by Bank of America that assists millions of customers. Erica can answer customer queries about transactions. Erica helps customers pay their bills. Credit Reports Personalized Guidance Erica provides credit report updates to customers. Erica offers personalized financial guidance based on spending habits and account balances. Fraud Detection Fraud detection with rule-based systems have high false positives Predefined criteria in traditional systems fail to adapt to new fraud tactics Machine learning models analyze transactions from multiple dimensions Analyze amount, location, device, speed etc. to detect subtle anomalies indicating fraud PayPal uses AI/ML to analyze billions of transactions Keeps fraud rate much lower than industry average by precision fraud detection AI and machine learning enable more accurate and adaptive fraud detection compared to traditional rule-based systems AI in Risk Management Fraud detection Credit risk Market risk Operational risk AI can analyze transactions to detect fraud patterns AI can assess creditworthiness using traditional and nontraditional data AI can forecast market risks by analyzing historical data AI can identify potential operational risks across systems AI enables more holistic risk management through data analysis, improving accuracy and expanding inclusion. Robo-Advisors in Wealth Management Personalized investment strategies Cost-effectiveness Robo-advisors use algorithms and user data to provide tailored investment advice aligned with financial goals and risk tolerance Robo-advisors offer affordable, automated services compared to traditional wealth management with high fees Robo-advisors utilize AI and algorithms to deliver customized, low-cost investment management to a wide range of investors. Accessibility and Convenience Available 24/7 from anywhere through internet User-friendly interfaces The accessibility and convenience of robo-advisors through their availability and user-friendly interfaces empower users to take control of investments. AI-Enabled Insurance Underwriting AI-enabled insurance underwriting utilizes machine learning algorithms to process vast datasets from sources like telematics, wearables, and social media. This allows for more accurate, personalized risk assessments. For example, Lemonade leverages AI chatbots for quick, customized quotes. Benefits and Challenges Increased Efficiency More Personalized Policies Lower Premiums Data Privacy Concerns Regulatory Compliance Maintaining Transparency Regulatory questions AML/KYC compliance Explainable AI decisions Fair and transparent AI AI systems in finance must comply with EU regulations on antimoney laundering, consumer protection and transparency. MiFID II Greater transparency Market stability Investor protection Firms must be transparent about their algorithmic trading strategies and systems. MiFID II aims to prevent disruptions and volatility from algorithmic trading. The regulation improves safeguards for investors in financial markets. MiFID II regulates algorithmic trading to ensure market integrity and stability in the EU. Suitability and Appropriateness Assessments Detailed suitability assessments Appropriateness evaluation Human oversight AI must gather comprehensive client info to assess suitability AI must determine if recommendations match client's goals Allow for human review of AI recommendations AI investment advisors must conduct thorough assessments tailored to each client and enable human supervision. Quantum Computing in Financial Services Quantum computing can optimize complex portfolio analysis Advanced risk simulation and assessment Rapid multivariate optimization of assets and constraints Model market scenarios and credit risks with higher accuracy Enhanced trading algorithms Process complex trading algorithms more efficiently Quantum computing can revolutionize portfolio optimization, risk management, and algorithmic trading in financial services. Enhanced Cybersecurity Quantum computers help improve security Quantum computers enable advanced cryptography like quantum key distribution which improves security of financial transactions. But also threaten existing encryption Quantum computers can break existing encryption standards, so quantum-resistant cryptography must be developed. Quantum computing is a double-edged sword for cybersecurity that necessitates new cryptography techniques. Challenges Legal and regulatory compliance Cybersecurity threats Technological hurdles Ethical considerations As quantum computing transforms finance, stakeholders must proactively address emerging challenges.