E-Commerce (II) PDF
Document Details
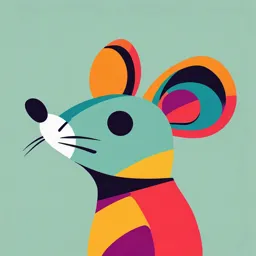
Uploaded by UnconditionalDiction
The Chinese University of Hong Kong
Tags
Summary
This document discusses e-commerce and the use of artificial intelligence in the field. It covers topics such as different business models, the impact of the Covid-19 pandemic on e-commerce landscapes, and different operational models within the E-Commerce market. It also explores the role of large language models in e-commerce contexts.
Full Transcript
E-Commerce (II) Artificial Intelligence (AI) in E-Commerce and Engaging Customers 1. The YOHO Case B2C E-Commerce Platform Electronics and home appliances retailer 2. First-Party Selling Model (1P Model) YOHO procures products directly from suppliers → Manages...
E-Commerce (II) Artificial Intelligence (AI) in E-Commerce and Engaging Customers 1. The YOHO Case B2C E-Commerce Platform Electronics and home appliances retailer 2. First-Party Selling Model (1P Model) YOHO procures products directly from suppliers → Manages inventory and handle customer orders → Distribute products and provide after-sales services High Control Asset-heavy Model 3. E-commerce Landscape in Hong Kong Limited Adoption: ○ about ⅓ individuals aged 15+ shop online ○ about ⅓ enterprises had a web presence Impact of Covid-19 Pandemic: ○ Accelerated shift ○ Increase in digital payments ○ Online sales grew by 27% 4. Strength and Weakness of E-Commerce Strength Weakness High accessibility Lack of Physical Experience Convenience Delivery Delays Cost efficiency Security Concerns Flexible Business Models Limited Personal Interaction 5. Online-Merge-Offline Model (OMO) Online + Offline stores 6. Porter’s Five Forces Model The Threat of New Entrants - Low barriers of entry facilitates competitors and increasing market competition The Bargaining Power of Buyers - customers have significant power due to numerous choices - compelling business to offer competitive pricing and quality The Bargaining Power of Suppliers - many suppliers exist, generally lower their bargaining power and allow E-commerce firms to negotiate better terms The Threat of Substitute Products - high availability of substitutes increases competition, as customers can easily switch Competitive Rivalry within Industry - increase competition among established players lead to price wars and innovation pressures 7. Generative (Gen) AI Foundation Models ○ Foundation models serve as a base for multiple applications, moving away from task-specific AI ○ They are trained on vast amounts of unstructured data, enabling them to perform diverse tasks Generative Capabilities ○ Generative Al models predict and generate content, such as predicting the next word in a sentence ○ With tuning and prompting, these models can adapt to specific natural language processing tasks with minimal labeled data Advantages of Foundation Models ○ Performance: Foundation models excel due to their extensive training on large datasets, outperforming traditional models ○ Productivity Gains: They require less labeled data for task- specific applications, thanks to their pre-training Disadvantages and Challenges ○ Compute Costs: Training and running these models can be expensive, posing challenges for smaller enterprises ○ Trustworthiness: Concerns arise from the unvetted, diverse data these models are trained on, leading to potential biases Applications Beyond Language ○ Vision Models: Technologies like DALL-E 2 generate images from text ○ Code Assistance: Tools like GitHub Copilot or ChatGPT help developers write code efficiently ○ Specialized Domains: IBM is applying foundation models in areas like chemistry and climate change research 8. Large Language Models LLMs are designed to generate human-like responses to text-based inputs and perform a wide range of natural language processing tasks, including language translation, text summarization, and sentiment analysis LLMs are distinguished by their massive size, with hundreds of millions to billions of parameters, and their ability to learn from vast amounts of data They utilize deep learning algorithms, such as transformers, to process and understand text data, enabling them to learn patterns, identify relationships, and generate accurate responses Performance Boost ○ Foundation models leverage vast datasets ○ Leading to superior performance in various applications compared to traditional models ○ Makes them attractive for businesses aiming for efficiency Generative Capabilities: ○ These models can generate new content, such as text or images ○ By predicting the next word or visual element ○ Opens up innovative possibilities in creative fields Adaptability: ○ Foundation models can be fine-tuned with minimal labeled data ○ Making them versatile tools for specific natural language processing tasks ○ Saving time and resources Data Dependency: ○ Effectiveness of these models relies heavily on the quality and quantity of data they were trained on ○ Raising concerns about potential biases and reliability in outputs Cost Barrier: ○ High computational requirements for training and running large models can pose significant financial challenges for smaller companies ○ Limiting widespread adoption Trust Issues: ○ Uncurated nature of the training data can lead to ethical concerns ○ These models may inadvertently generate biased or harmful content Broad Application: ○ Beyond text, foundation models are being applied in multiple domains including visual content generation and scientific research ○ Showcasing their versatility and potential impact across industries 9. YOHO AI Assistant - Empowered By Generative AI Collaborated with OpenAI and Microsoft’s Azure OpenAI Functions: product search, recommendations, 24/7 customer services etc. Powerful business tool Insights from data patterns How could Gen AI help? ○ Boost visibility → Personalised Service → Increase sales → Stay competitive ○ Keep up with rapidly evolving AI technology ○ Enhance SEO with GAI to drive more traffic ○ Leverage GAI for tailored customer experiences from presales to aftersales ○ More traffic and better engagement lead to higher conversions 10. Customer Relationship Management Functions of CRM Systems i. Capture and integrate customer data from all over the organization ii. Consolidate and analyze customer data iii. Distribute customer information to various systems and customer touch points across enterprise iv. Provide single enterprise view of customers CRM Software ○ From niche tools to large scale enterprise applications ○ More comprehensive packages have modules for: a. Partner relationship management (PRM) Integrating lead generation, pricing, promotions, order configurations, and availability Tools to assess partners’ performances b. Employee relationship management (ERM) Setting objectives, employee performance management, performance-based compensation, employee training CRM Software Capabilities ○ Sales Force Automation (SFA) Sales prospect and contact information Sales quote generation capabilities ○ Customer Service Assigning and managing customer service requests Web-based self-service capabilities ○ Marketing Capturing prospect and customer data, scheduling and tracking direct-marketing mailings or e-mail Cross-selling Customer Loyalty Management Roadmap Operational and Analytical CRM Operational CRM Analytical CRM Customer-facing applications Based on data warehouses Sales force automation call center populated by operational CRM and customer service support systems and customer touch Marketing automation points Analyzes customer data (OLAP, data mining, etc.) Customer lifetime value (CLTV) Analytical CRM Data Warehouse Business Value of CRM Systems Business value of CRM systems Churn rate Increased customer satisfaction Number of customers who stop Reduced direct-marketing costs using or purchasing products or More effective marketing services from a company Lower costs for customer Indicator of growth or decline of acquisition/retention firm’s customer base Increased sales revenue