Data Analytics Fundamentals PDF
Document Details
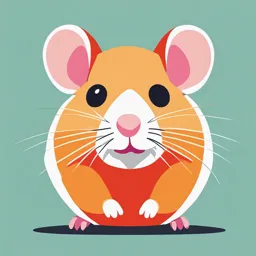
Uploaded by FlawlessFantasy4551
Tags
Summary
This document provides an introduction to data analytics, focusing on descriptive analytics. It explains the purpose of data analytics as a way to extract insights and improve decision-making. The document emphasizes the use of descriptive analysis to identify what has happened, and shows an example in the context of an ecommerce website.
Full Transcript
Data Analytics Fundamentals.. Explore Data Analytics Types.. Gathering data points is just a first step. What do you do with all that data? You need to put the information together to help people make decisions. This is the core goal of data analytics. And this module introduces you to the differen...
Data Analytics Fundamentals.. Explore Data Analytics Types.. Gathering data points is just a first step. What do you do with all that data? You need to put the information together to help people make decisions. This is the core goal of data analytics. And this module introduces you to the different types of data analytics, especially descriptive analytics, and how they’re used in common business cases. Watch the following video from Rafael "Raf" Lopes, Senior Cloud Technologist at AWS. The quiz at the end of this unit asks questions about the content of this video. Be sure to watch so you get the information you need to answer the questions at the end of this unit. Hello. If you are here, you probably have an interest in data analytics, and that's great. So let me start sharing with you the value proposition of what data analytics is. first thing we need to think about when talking about data analytics is how to use the collected data to produce information that will be useful for future business needs. Those are called insights. Sometimes, this journey of generating insights out of data can be big and complex, involving the use of machine learning. Or sometimes, it can be quick and simple, if the dataset is ready, and you just want to perform descriptive data analysis. That being said, data analytics is the science of handling data collected by computer systems in order to generate the insights that will improve decision making with facts based on data. Nowadays, data analytics is widely used for ecommerce and social media. But the knowledge can, and should, be applied into information security, logistics, factory operations, Internet of Things, and much more. There are four main types of data analytics, which, in order of complexity, are listed as descriptive, diagnostic, predictive, and prescriptive. Let me talk a bit regarding each one of those. I will spend a bit more time on the descriptive analytics because, in this introductory course, this is what I will be mostly covering. Descriptive analysis is a data analysis type that is mainly used to give you information regarding what happened. It is intended to allow you to use data collected by a system in order to help you identify what was wrong, what could be improved, or which metric is not reporting as it should. As this type of data analysis is widely used to summarize large datasets in order to describe outcomes to stakeholders, think about descriptive analysis as something that just reports what's going on, and nothing more. The most relevant metrics informed by those systems are mostly known as KPIs, or key performance indicators. Identifying what happened can be extremely important for some market verticals, and sometimes are enough to satisfy the need for further investigation into an issue. Let me give you an example on how descriptive data analysis can be useful in terms of identifying the correct KPIs to keep stakeholders aware on taking data-driven decisions to fix potential issues. Imagine an ecommerce website where you collect metrics regarding the time required for a payment to be processed. In this website, you are using an external payment gateway to complete the purchases. So, every time a customer buys something in your website, the customer is redirected to that payment gateway, and you have a confirmation that the customer had paid when he or she did so. A relevant set of KPIs for doing an effective descriptive analysis, in this case, could be metrics regarding the time required to complete the transaction, number of completed transactions, and number of canceled transactions. Now, if you see a spike on both number of canceled transactions and time required to complete the transaction, that may be a good indicator that those transactions are being canceled because they are taking too long. It may be a good indicator that those KPIs might be related to each other, which could help systems administrators and business owners to start troubleshooting a potential issue that may be impacting on sales. The very same concept applies with the time to complete the transactions. If you have something that drills down into that metric by separating each step within the transaction time, you would have even more granular information to pinpoint the right place to solve the issue. The last thing you want is to be informed about system malfunctions via social media feeds or customer inputs. In this case, monitoring is key, and we use a very simple set of metrics in order to start troubleshooting a business-related issue. In a nutshell, descriptive data analysis is a concept that informs you what's going on. You can also do descriptive data analysis if you have data regarding user activity, social media feeds, Internet of Things or system security logs. As I said, the use case can vary a lot, but one thing is for sure. Once you have the knowledge on how to perform descriptive data analysis, you can, and should, use that same knowledge to work with different datasets. Great, now we have a solid fundamentals regarding what is descriptive data analysis. What about the other three? Well, remember in our example, that you have the matrix regarding the transaction time and number of failed transactions. In that case, you were the one responsible for having the insights, for having the idea, to correlate those two metrics together in order to identify the issue. The system did not connect them together, and gave you a consolidated, or a projected, metric called probability of issue with the payment gateway. It is very common nowadays to have hundreds, or even thousands, of those metrics in systems. And diagnostic analysis helps by going over the scope of just informing, but diagnosing by further investigating and correlating those KPIs in order to give you suggestions on where the issue could potentially be. I like to refer to diagnostic analysis as a set of actions a system can do to help stakeholders understand why something happened. The word you need to remember here is why. Our third type of data analysis is the predictive analysis. Predictive data analysis involves more complexity, because, as the name suggests, it predicts what is likely to happen in the future based on data from the past, or based on doing a data crossover between multiple datasets and sources. In a nutshell, it kind of tries to predict the future based on actions from the past. The use of neural networks, regression, and decision trees are very common on diagnostic analysis, and we will be covering that in another course. Now last, but not least, prescriptive analysis, which is basically a sum of all the previous. Prescriptive analysis can go ahead and suggest stakeholders what are the most data-driven decisions that needs to be taken based on past events and outcomes. Prescriptive analysis highly relies on machine learning strategies in order to find patterns and their corresponding remediations by looking and crossing large datasets. Regardless of the type you decide to learn and apply, data analysis exists at the intersection of using information technology, statistics, and domain knowledge, like social media, business, or industry verticals. In this course, we will be focusing on how to use AWS services to perform descriptive analysis about what's going on with an AWS account by using security logs. Now that you understood the different types of data analysis, let's continue the journey by exploring some more examples on where data analysis is present in your life right now. Understand Common Data Analysis Use Cases.. Use Data Analytics for a Complex World. What do gaming, commerce, and social media have in common? These verticals each produce a lot of data that organizations use to improve their services, as well as detect and troubleshoot issues. In the following video, Raf explores the common verticals and use cases where data analysis is present in everyday life. Are we talking about 100 lines of data? 1,000? In some cases, it can be hundreds of thousands—or even millions! How do you work with it all? Now that you know the difference between the different types of data analysis, let me show you some examples on how data analytics is very likely to be present right now in your life, both as a consumer, and perhaps professionally. Data analytics is widely present in many verticals today, such as gaming; social media feeds; ecommerce, online stores; websites; statistics, also known as clickstreaming; recommendation engines; Internet of Things, or IoT; log processing; and much more. Let me give you a couple of examples on where data analytics is valuable in some of those scenarios, so you grasp what is the exact purpose of data analytics in these contexts. Let's say you like to play computer games, like me. Who doesn't, right? So, if you like to play games, either in your phone, your computer, or in the game console, you may be familiar with the check box you may need to check before you starting play. That chat box usually says something like Send anonymous data statistics for game developers to improve gaming experiences, or such. What this does is basically allowing to collect information regarding the way you play the game in order to detect potential crashes, design failures, and other data. It is clear in this case, that real-life data, such as you playing your game, is being transformed into information that helps developers to circumvent potential issues and enhance the gaming experience. That is exactly why data analytics exists, and why it is so relevant for the modern world. You may ask, why is this a thing nowadays? I've been playing games since childhood, and that was not the case. Games were sold on cartridges. We used it to buy and play, right? Well, yes. But if you think with me, those games were not that complex as the games we have today. And that's what I want to conclude. Analytics helps people develop insights, and those insights help them to deal with complex problem solving. No matter if it is regarding gaming, stock markets, real-estate data, traffic information, fashion computer systems, web server or security logs, data analytics help to provide answers to complex scenarios. With storage prices going down day after day, companies often collect data that they may currently not have a use for. However, if a question arises tomorrow, the answer can be in the data they had previously collected. The world nowadays is becoming more complex than it was 10 years ago. And having the help of computer systems is instrumental for two main reasons. Scalability, and data-driven decision making. Another major part of data analysis is log analytics. Let me dig a little deeper into this one, because that is where I will mainly focus during this course, specifically regarding security logs. When we talk about log analysis, we're usually talking about the information produced by computer systems, based on events. That event can be an HTTP request made to a webpage, user logging information, API calls, or any other type of requests. API is the acronym for application programming interface, which is basically a computing interface which defines interactions between multiple software intermediaries. It defines the kinds of calls or requests that can be made, how to make them, the data formats that should be used, the conventions to follow, etc. And for the data analytics standpoint, it is very common to log all those activities somewhere. A classic example of data analytics is using web server logs to extract insights about visitors in a website. Let's say every request made to an HTTP server is logged into files in a file system. Those are mostly called access logs. If you have one new line added to the access log for every visit in your website, you can say that the number of lines in this log is equivalent to the number of requests that was served by the web server. If you just have one server and a small website with a couple of visits per minutes, you can use some basic tools, like text editors, to parse those files and extract what you're looking for. But if you want to do something slightly more useful than just summing up lines in the log file, the usage of a data analysis tool is key. We encourage using data analysis tools everywhere, but we need professional ones that handle scale when we want to do log aggregation and visualization. Imagine you have tens of web servers attempting thousands of users per second. You can estimate that each log file on each server will be filled up pretty quick. So, you need to have all that data concentrated somewhere. In addition, you may need to have a way to visualize that data in a line chart, which could easily help you with identifying spikes, also called deviations or outliers. Another big use of data analysis nowadays is data security. If you have systems producing security logs in a way you can quickly get to in order to extract analytics, you were in clear advantage if you need to pinpoint when a request was made, by whom, from when, from where, and what was the system's response to that request. If you reach into the level of doing predictive analysis on top of this data, you can even reach a state where you will automatically block bad requests to computer systems before they occur, or creating a self-healing architecture that starts building a failover environment when a current environment is presenting degradation. That can be achieved with the help of infrastructure automation tools in the cloud. There is an AWS service called AWS CloudTrail, which logs API activity made to an AWS account, and another AWS service called Amazon S3, which is a storage service. Let me briefly talk about them. This is what CloudTrail stores every time you or someone logs in to your AWS account by using the AWS Management Console. That is stored in Amazon S3, and contains information such as who made the request, from which IP address, what was the request for, what was the answer to that request, and some other useful compliance information that can quickly turn into evidence, if needed. Because of that nature, CloudTrail is a service that enables infrastructure governance, operational auditing, and risk auditing for your AWS account. But if you need to dig into CloudTrail text data every time, it may be something hard to achieve. So learning data analytics helps a lot to unleash what you can do with all this compliance data. If you had data visualization tools on information produced by CloudTrail, you can have security dashboards containing graphics and alerts of unusual activities. If you suddenly start seeing logs of login-failure activities, it may be because someone is trying to log in to your AWS account, or because you changed the password and forgot it. I usually say that data security analytics is not good only for compliance reports, but also very useful for troubleshooting. If you apply that concept to firewall packets, networking activity, load balancer, and server logs, and other kind of infrastructure topics, you can easily identify outliers and turn yourself into a quick problem solver. But always think about what else could you be using data analytics for, and how it helps you on getting stronger insights about what's going on, no matter if it is regarding security, product improvement, better customer experience, or any other part of the data analysis spectrum. Since the sky is the limit, in the next video, I will be talking about why doing all these in the cloud gives you some serious advantages and how it helps on enabling data analytics everywhere, anytime, for everyone. Take Data Analytics to the Cloud.. Build Your Data Analytics Solution in the Cloud.. As business has gotten more complex over time, tools and services have gotten more powerful to enable organizations to keep up. A prime example is the evolution of data analytics from expensive, on-premises hardware to cloud-based architectures. You may already know that the cloud is more flexible, scalable, secure, distributed, and resilient. But I want to give a more data-related approach in terms of why cloud computing is relevant for data analytics. In this section, I will explain why the cloud is the best way to perform data analytics nowadays, and why it has been solid for operating big data workloads. So, let's get started. Before we start talking about cloud, allow me to go back in time, maybe a decade, and tell you a brief story. After going back in time, it will be natural for you to understand why everybody loves doing data analytics in the cloud. Ready for the journey? Get your beverage of choice, and buckle up! (cup hitting the floor) (whirring sound) Years ago, the most common approach for companies to have compute infrastructure, big data included, was to buy servers and install them into data centers. This is usually called a collocation, or colo. The thing is, servers utilized for data operations are not cheap, because they need lots of storage, consume lots of electricity, and require careful maintenance regarding data durability. Hence, entire dedicated infrastructure teams. And trust me, I've been one of those infrastructure analysts working with data centers. It is expensive and overwhelming. With that scenario, only big companies were able to work with big data. And consequently, data analytics was not popular. It was very common for those servers to have a RAID storage controller that replicates data across the disks, increasing the cost and maintenance care even more. In the early 2000s, big data operations were closely related to the underlying hardware, such as mainframes and server clusters. Although this was extremely profitable for the ones selling hardware, it was expensive and not flexible for the consumers. Then, something fantastic started to happen. And the name of this fantastic thing is Apache Hadoop. Mostly, what Hadoop does is replacing all that fancy hardware by software installed in operating systems. Yeah, that's right. With the help of Hadoop and computing frameworks, data could be distributed and replicated across multiple servers by using distributed systems, and eliminating the need of those expensive data-replication hardware to start working with big data. All you needed was efficient network equipment, and the data were synchronized over the network to other servers. By embracing failures instead of trying to avoid them, Hadoop helped reduce hardware complexity. And when you reduce hardware complexity, you reduce cost. And by reducing cost, you start to democratize big data, because smaller companies could start leveraging it as well. Welcome to the big data boom. I brought up Hadoop originally, because Hadoop is the most popular open source, big data ecosystem. There are others. And what I wanted to highlight here, is the concept, and not specific frameworks or vendors. The thing is, by baselining hardware to a basic level and applying all big data concepts to software, such as data replication, we can start thinking about running big data operations on providers that are capable to provide virtual machines with storage and a network card attached. We can start thinking about using the cloud to build entire data lakes, data warehousing, and data analytics solutions. Since then, cloud computing has emerged as an attractive alternative because it is exactly what it does. You can get virtual machines, install the software that will handle the data replication, distributed file systems, and entire big data ecosystems, and be happy without having to spend lots of money in hardware. The advantage is that cloud does not stop there. Many cloud providers, such as Amazon Web Services, started to see that customers were spinning up virtual machines to install big data tools and frameworks. And then based on that, Amazon started to create offerings with everything already installed, configured, and ready to use. That's why you have AWS services, such as Amazon EMR, Amazon S3, Amazon RDS, Amazon Athena, and many others. Those are what we call managed services. All those are AWS services that operate in the data scope. In a later lesson, I will talk more about some services we will need to build our basic data analytics solution. Another big advantage of running data analytics in the cloud is the ability to stop paying for infrastructure resources when you don't need them anymore. This is very common in the data analytics, because due to the nature of big data operations, you may need to run reports once in a while. And you can easily do that in the cloud by spinning up server or services, using them, getting the report you need, saving that, and turning off everything. In addition, you can temporarily spin more servers to speed up your jobs, and turn off when you're done. And since you mostly pay for time and resources needed, 10 servers running for 1 hour tends to have the same price of one server running for 10 hours. Basically, with the cloud, you're having access to hardware without having to concern with all the burden involved on doing data center operations. It is like the best of both worlds. Correlation and Regression.. Examine Correlation in Data.. Data literacy is the foundation for using and communicating with data with ease. The Data Literacy Basics module describes quantitative variables as numerically measurable characteristics, such as number of hours spent watching television each day, speed measured in miles per hour, total inches of annual rainfall in a city, sales in dollars, and amount spent on marketing. When you are examining relationships within your data, how do you determine how closely two variables, like sales and the amount spent on marketing, are related? Can you use one variable to predict the other? Correlation and regression are important techniques used to discover trends and make predictions. While there are other important forms used in analytics, we focus on the simplest form used in AI and analytics—linear correlation and regression. In this unit, you gain familiarity with the concept of correlation, which describes whether and how closely two variables move in relation to each other. You gain an appreciation of how correlation measures association but doesn’t prove causation. In the next unit, you explore how linear regression can be used to calculate or predict the value of one variable based on another, in addition to measuring how well this model fits your data. What Is Correlation?. Correlation is a technique that can show whether and how strongly pairs of quantitative variables are related. This unit discusses Pearson's correlation. There are other non-linear correlations, which are not covered here. For example, do the number of daily calories consumed and body weight have a relationship? Do people who consume more calories weigh more? Correlation can tell you how strongly peoples’ weights are related to their calorie intake. The correlation between weight and calorie intake is a simple example, but sometimes the data you work with may not have the relationships that you expect. Other times, you may suspect correlations without knowing which are the strongest. Correlation analysis helps you understand your data. When you begin your correlation analysis, you can create a scatter plot to investigate the relationship between two quantitative variables. The variables are plotted as Cartesian coordinates, marking how far along on a horizontal x-axis and how far up on a vertical y-axis each data point is. In the scatter plot below, you see the relationship between sales and the amount spent on marketing. It appears there’s a correlation: As one variable goes up, the other seems to as well. Correlation Versus Causation. Now that you know how correlation is defined and how it is represented graphically, let's discuss how to better understand correlation. First, it’s important to know that correlation never proves causation. Pearson’s correlation tells us only how strongly a pair of quantitative variables are linearly related. It does not explain the how or why they’re related. For example, sales of air conditioners correlate with sales of sunscreen. People aren’t buying air conditioners because they bought sunscreen, or vice versa. The cause of both purchases is hot weather. How Is Correlation Measured?. Pearson’s correlation, also called the correlation coefficient, is used to measure the strength and direction (positive or negative) of the linear relationship between two quantitative variables. When correlation is measured in a sample of data, the symbol used is the letter r. Pearson’s r can range from -1 to 1. When r = 1, there is a perfect positive linear relationship between variables, meaning that both variables correlate perfectly as values increase. When r = -1, there is a perfect negative linear relationship between variables. In a perfect negative correlation, when one variable increases, the other variable decreases with the same magnitude. When r = 0, no linear relationship between variables is indicated. With real data, you would not expect to see r values of -1, 0, or 1. Generally, the closer r is to 1 or to -1, the stronger the correlation, as shown in the following table. r= Correlation 0.90 to 1 Very strong correlation or -0.90 to -1 0.70 to 0.89 Strong correlation or -0.70 to -0.89 0.40 to 0.69 Modest correlation or -0.40 to -0.69 0.20 to 0.39 Weak correlation or -0.20 to -0.39 0 to 0.19 Very weak or no correlation or 0 to -0.19 Some resources on this topic categorize correlations simply as strong, modest, or weak. Linear Correlation Conditions.. For correlations to be meaningful, you need to consider some conditions: they must use quantitative variables, describe linear relationships, and take into account the effect of any outliers. You should check these conditions before you run a correlation analysis.. In 1973, a statistician named Francis Anscombe developed Anscombe’s Quartet to show the importance of graphing data visually, as opposed to simply running statistical tests. The four visualizations in his quartet all show the same trend line equation. The quartet illustrates why visualizations are so important—they help us identify trends within our data that may be obscured by statistical tests. In the example below, only the top-left scatter plot in the quartet meets the criteria of being linear without any outliers. The top-right scatter plot is not showing a linear relationship and a nonlinear model would be more appropriate. The two scatter plots on the bottom each have outliers which can dramatically affect the results. Now that you’re more familiar with the concepts around the statistical technique of correlation, you’re ready for the next unit, where you learn about linear regression. Discover Relationships Using Linear Regression.. What Is Linear Regression?. In the previous unit, you learned that correlation refers to the direction (positive or negative) and the strength (very strong to very weak) of the relationship between two quantitative variables. Like correlation, linear regression also shows the direction and strength of the relationship between two numeric variables, but unlike correlation, regression uses the best-fitting straight line through the points on a scatter plot to predict Y values from X values. With correlation, the values of X and Y are interchangeable. With regression, the results of the analysis will change if X and Y are swapped. The Linear Regression Line.. Just as with correlations, for regressions to be meaningful, you must: Use quantitative variables. Check for linear relationship. Watch out for outliers. Like correlation, linear regression is visualized on a scatter plot. The regression line on the scatter plot is the best-fitting straight line through the points on the scatter plot. In other words, it is a line that goes through the points with the least amount of distance from each point to the line. Why is this line helpful and useful? We can use the linear regression calculation to calculate, or predict, our Y value if we have a known X value. To make this clearer, let's look at an example. A Regression Example.. Let’s say you want to predict how much you will need to spend to buy a house that is 1,500 square feet. Let's use linear regression to predict. Place the variable that you want to predict, home prices, on the y-axis (this is also called the dependent variable). Place the variable you're basing your predictions on, square footage, on the x-axis (this is also called the independent variable). Here is a scatter plot showing house prices (y-axis) and square footage (x-axis). The scatter plot shows homes with more square feet tend to have higher prices, but how much will you have to spend for a house that measures 1,500 square feet? To help answer that question, create a line through the points. This is linear regression. The regression line will help you to predict what a typical house of a certain square footage will cost. In this example, you can see the equation for the regression line. The equation for the line is Y = 113*X + 98,653 (with rounding). What does this equation mean? If you bought a place with no square footage (an empty lot, for example), the price would be $98,653. Here are the steps for how the equation is solved. To find Y, multiply the value of X by 113 and then add 98,653. In this case, we are looking at no square footage, so the value of X is 0. Y = (113 * 0) + 98,653. Y = 0 + 98,653. Y = 98,653. The value 98,653 is called the y-intercept because this is where the line crosses, or intercepts, the y-axis. It is the value of Y when X equals 0. The number 113 is the slope of the line. Slope is a number that describes both the direction and the steepness of the line. In this case, the slope forecasts that for every additional square foot, the house price will increase by $113. So, here’s what you need to spend on a 1,500 square foot house: Y = (113 * 1500) + 98,653 = $268,153. Take another look at this scatter plot. The blue marks are the actual data. You can see that you have data for homes between 1,100 and 2,450 square feet. Note that this equation cannot be used to predict the price of all houses. Since a 500-square-foot house and a 10,000-square-foot house are both outside of the range of the actual data, you would need to be careful about making predictions with those values using this equation. The r-Squared Value.. In addition to the equation in this example, we also see an r-squared value (also known as the coefficient of determination). This value is a statistical measure of how close the data is to the regression line, or how well the model fits your observations. If the data is perfectly on the line, the r-squared value would be 1, or 100%, meaning that your model fits perfectly (all observed data points are on the line). For our home price data, the r-squared value is 0.70, or 70%. Linear Regression Versus Correlation You may now be wondering how to distinguish between linear regression and correlation. See the table below to see a summary of each concept. Linear regression Correlation Shows a linear model and prediction, predicting Y Shows a linear relationship between two from X. values. Uses r-squared to measure the percentage of Uses r to measure the strength and variation explained by the model. direction of the correlation. Does not use X and Y as interchangeable values Uses X and Y as interchangeable (because Y is predicted from X). values. Being familiar with the statistical concepts of correlation and regression helps you to explore and understand the data you work with by examining relationships.