Data Analysis Seminar 5 PDF
Document Details
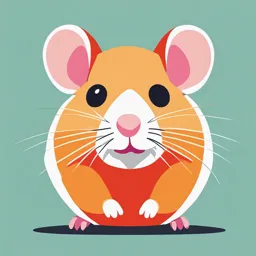
Uploaded by CelebratoryWeasel
Faculty of Management
Dr. Waqas Ahmed
Tags
Summary
This presentation is about data analysis, including quantitative methods like hypothesis testing and regression analysis, and qualitative methods. It covers aspects like data coding, data entry, editing, and transforming data. The presentation emphasizes different methods like T-tests, ANOVA, and how to use software like SPSS and Minitab for analysis.
Full Transcript
Faculty of Management Department of Venture Nizhny Novgorod Creation Data Analysis Seminar 5 Dr. Waqas Ahmed 2 Agenda Quantitative Data Analysis Qualitative Data Analysis ...
Faculty of Management Department of Venture Nizhny Novgorod Creation Data Analysis Seminar 5 Dr. Waqas Ahmed 2 Agenda Quantitative Data Analysis Qualitative Data Analysis 3 Getting the Data Ready for Analysis Data coding: assigning a number to the participants’ responses so they can be entered into a database. Data Entry: after responses have been coded, they can be entered into a database. Raw data can be entered through any software program (e.g., SPSS) 4 Editing Data An example of an illogical response is an outlier response. An outlier is an observation that is substantially different from the other observations. Inconsistent responses are responses that are not in harmony with other information. Illegal codes are values that are not specified in the coding instructions. 5 Transforming Data 6 Getting a Feel for the Data 7 Frequencies 8 Descriptive Statistics: Central Tendencies and Dispersions 9 Reliability Analysis Quantitative Data Analysis: Hypothesis Testing Quantitative Data Analysis: Hypothesis Testing 11 Testing Hypotheses on a Single Mean One sample t-test: statistical technique that is used to test the hypothesis that the mean of the population from which a sample is drawn is equal to a comparison standard. 12 Testing Hypotheses about Several Means Analysis Of Variance (ANOVA) helps to examine the significant mean differences among more than two groups on an interval or ratio-scaled dependent variable. 13 Regression Analysis Simple regression analysis is used in a situation where one metric independent variable is hypothesized to affect one metric dependent variable. 14 What test to use when 15 Conceptual Model Moderator is qualitative (e.g., gender, race, class) Gender or quantitative (e.g., level of reward) that affects the direction and/or strength of the relation Perceived between dependent and independent variable Intelligence + + + Physical Likelihood Attractiveness to Date + Communality of + Perceived Interests Fit Mediating/intervening variable accounts for the relation between the independent and dependent variable 16 Hypothesis based on conceptual model Based on the conceptual model following hypotheses that could be tested: Main effect hypothesis: "Physical attractiveness positively predicts the likelihood to date." Moderation hypothesis: "The relationship between perceived intelligence and likelihood to date is moderated by gender." Mediation hypothesis: "The effect of communality of interests on likelihood to date is mediated by perceived fit." Multiple predictor hypothesis: "Perceived intelligence, physical attractiveness, and perceived fit will each independently predict likelihood to date." Interaction hypothesis: "There is an interaction effect between perceived intelligence and physical attractiveness on likelihood to date." 17 Model Validation 1. Face validity: signs and magnitudes make sense 2. Statistical validity: Model fit: R2 Model significance: F-test Parameter significance: t-test Strength of effects: beta-coefficients Discussion of multicollinearity: correlation matrix 3. Predictive validity: how well the model predicts Out-of-sample forecast errors Slide 18 Step 1 Mode l Summ ary Adjus ted St d. Error of Model R R Square R Square the Estimate 1.963.927.923 3. 020 ANOVA Sum of Model Squares df Mean Square F Sig. 1 Regression 10745.603 5 2149.121 235.595.000 Residual 848.357 93 9.122 Total 11593.960 98 19 Step 1 cont’d Coefficients Unstandardized Standardized Coefficients Coefficients Model B Std. Error Beta t Sig. 1 (Cons tant) 17.094 2.497 6.846.000 PERC_INTGCE.030.029.044 1.039.301 PHYS_ATTR.517.018.836 29.269.000 GENDER -.783 2.379 -.036 -.329.743 PI_GENDER.122.038.356 3.201.002 COMM_INTER.212.019.319 11.187.000 significant effect on dep. var. 20 Step 2 Mode l Summ ary Adjus ted St d. Error of Model R R Square R Square the Estimate 1.977.955.955 2. 927 ANOVA Sum of Model Squares df Mean Square F Sig. 1 Regression 17720.881 1 17720.881 2068.307.000 Residual 831.079 97 8.568 Total 18551.960 98 21 Step 2 cont’d Coefficients Unstandardized Standardized Coefficients Coefficients Model B Std. Error Beta t Sig. 1 (Cons tant) 8.474 1.132 7.484.000 COMM_INTER.820.018.977 45.479.000 significant effect on mediator 22 Step 3 Mode l Summ ary Adjus ted St d. Error of Model R R Square R Square the Estimate 1.966.934.930 2. 885 ANOVA Sum of Model Squares df Mean Square F Sig. 1 Regression 10828.336 6 1804.723 216.862.000 Residual 765.624 92 8.322 Total 11593.960 98 23 Step 3 cont’d Coefficients Unstandardized Standardized Coefficients Coefficients Model B Std. Error Beta t Sig. 1 (Cons tant) 14.969 2.478 6.041.000 PERC_INTGCE.019.028.028.688.493 PHYS_ATTR.518.017.839 30.733.000 GENDER -2.040 2.307 -.094 -.884.379 PI_GENDER.142.037.412 3.825.000 COMM_INTER -.051.085 -.077 -.596.553 PERC_FIT.320.102.405 3.153.002 insignificant effect of indep. var on dep. Var. significant effect of mediator on dep. var. 24 Softwares for Quantitative Data Analysis SPSS https://www.ibm.com/products/spss-statistics Minitab https://www.minitab.com/en-us/products/minitab/ free-trial/ Stata https://www.stata.com/ Qualitative Data Analysis Qualitative Data Analysis 26 Qualitative Data Data that are not easily reduced to numbers Data that are related to concepts, opinions, values and behaviours of people Data that can be broken down through the process of classifying or coding; the pieces of data are then categorized. 27 What is Qualitative Data Analysis? Qualitative Data Analysis (QDA) is the range of processes and procedures whereby we move from the qualitative data that have been collected into some form of explanation, understanding or interpretation of the people and situations we are investigating. 28 What is Qualitative Data Analysis Data analysis is a process of breaking down data into smaller units, determining their importance, and putting pertinent units together in a more general form. 29 Qualitative Data Collection Observation (field notes, checklist….) Interviews Documents (reports, meeting minutes) Focus Groups Tape Recorder Audio/Video Recording Questionnaires (open-ended) 30 Coding Coding is a process of reducing the data into smaller groupings so they are more manageable. The process also helps you to begin to see relationships between categories and patterns of interaction. Sections of text transcripts may be marked by the researcher in various ways (underlining in a colored pen, given a numerical reference, or bracketed). 31 Categories/Themes A major step in analyzing qualitative data is coding speech/ words/text into meaningful categories/themes. As you read and reread through the data, you can compile the data into categories or themes A theme/category is generated when similar issues and ideas were expressed by participants. The theme or category may be labeled by a word or expression taken directly from the data or by one created by the researcher because it seems to best characterize the essence of what is being said. 32 Organize Data The field work is over Attempt to make sense of the data as a whole Researcher starts with a large set of data and seeks to narrow into small groups of key data Organizing the materials by type: all observations, all interviews, all field notes……. 33 Exploring Data The first step in data analysis is to explore the data Read through data and make sure all information is complete and legible before proceeding to analysis Obtain a general sense of the data Memoing - read and write memos about all field notes, observer comments to get an initial sense of the data 34 Steps in Coding the Data 1. Get a sense of the whole 2. Pick one document (e.g. one interview, one field note….). Go through it, asking the question “what is this person talking about?” 3. Identifying text segments, placing a bracket around them and assigning a code word or phrase that describes the meaning of the text 4. After coding an entire text, make a list of all the code words. Group similar codes and look for redundancy codes 5. Take the list and go back to the data. Circle specific quotes from participants that support the codes 6. Reduce the list of codes to get five to seven themes/categories 35 Identifying Themes Are there patterns that emerge? - Events that keep repeating themselves - Key phrases that participants use to describe their feelings 36 Themes Like codes, themes have labels. Types: Ordinary themes – themes that a researcher might expect to find Unexpected themes – themes that are surprises Hard-to-classify themes – themes that contain ideas that do not easily fit into one theme or that overlap Major and minor themes – themes that represent the major ideas and the minor secondary ideas 37 Summarizing your Data After you have coded a set of data, write a summary of what you are learning. Similarly, summarize the key themes that emerge. With your data coded and summarized you are ready to look across the various summaries and synthesize your findings across multiple data sources. 38 RQ: Why do students have problems with critical thinking? Major and Minor Themes from Teacher s’ Interview Question Major Theme Minor Theme What are some of the Reading Challenges Time constraints challenges that your students face in developing their critical thinking skills? Need authentic learning Greater immersion in experience reading How do you help to enhance the critical thinking skills of your students? Need to Read newspapers Reading of Journals What supplementary materials do you encourage your students to read within the 39 Collating Data into a Table of Coded Responses Observation Questionnaire Interview (teachers) (students) (students) Lack of group cohesion Reading challenges Unwilling to work in group Request teacher’s assistance too frequently Need authentic learning Laziness and fatigue experience Limited independent thinking Need to read newspaper Tardiness 40 Explanation of Themes Write up and explain the themes in narrative format under the specific research question Use a few actual quotes from the participants responses to validate your narrative (3 -5 is enough) Do this for each major theme that emerged from the data 41 Example of Narrative Format RQ: Why do students have problem with critical thinking? Reading challenges. When asked what are the challenges that students face in developing critical thinking skills the teachers interviewed felt that students had reading challenges. Many students were reading below their grade levels with limited vocabulary. This made it difficult for students to decipher the meaning of written work. The following are some direct quotation from the participants: “Students do not read on their own. Hence they cannot think critically when given the opportunity”. “Their reading level”. “They are unable to decipher the meaning of some key terms used in the question”. 42 Software for Qualitative Analysis https://atlasti.com/atlas-ti-web https://lumivero.com/solutions/analyze-data-analytics/qualitative-sentiment-analysis/ 43 Exercise Use this interview text to identify themes and give codes Artificial intelligence (AI) is one of today’s most frequently debated tools. While AI has potential to revolutionise multiple industries, the transformative opportunities it presents are simultaneously loaded with challenges around accuracy, ethics and the impact automation may have on jobs. The construction industry needs to understand the potential implications of AI and how it is likely to influence the sector, whether embraced or ignored. We spoke to four rising stars from across our global offices, Siti Norman, Director (Singapore), Guilherme Lombardo, Senior Digital Manager (Sau Paulo, Brazil), Michaela Fiserova, Associate Director (London, UK), and Habel Mwashigadi, Project Manager (Kenya, Africa), to consider the use of AI in their respective countries and how they believe AI will impact the construction sector and wider society. 44 Q. How much is AI being used or investigated in construction schemes in your region of the world? A. Siti: There has been a lot of buzz around ChatGPT in Singapore, however AI tools have been used in the construction market for the past five years. This includes a particular interest in generative AI for design optioneering, automated ruled-based 3D model checking and site safety for compliance. We have also seen universities setting up labs for innovation and incubating ideas. Guilherme: In Brazil, AI is being used mainly in image and document analysis to improve processes such as budgeting, construction monitoring and contract management. Some start-ups are also investigating the use of robots and drones with AI for inspections and monitoring. This is on a small scale, and mostly in the testing phase but it is a great opportunity to introduce AI to consulting. Michaela: AI tools have been used in the UK construction market for a while even though the construction industry is one of the least digitalised industries. I’ve seen some interesting use cases recently in health and safety where AI robots are tracking sites to prevent accidents and injuries. We’ve also been trialling using machine learning for quantity take-offs and pricing. Habel: AI has been deployed in Africa across various markets, however the uptake within the construction space still is in its early stages. The use of AI powered analytics through products such as OpenSpace has been used on fit-out and brownfield projects, as well as for reality capture on site. Matterport is also being used for virtual reality capture of spaces and design during execution on site. Major technology players such 45 Q. What do you think are the advantages and disadvantages to applying AI in construction? A. Siti: The advantages of applying AI in construction will be having access to data that makes a difference. It will change the attitude we have towards collecting data and make it purposeful. It also gives us the opportunity to level the playing field. AI can create space for diversity and inclusion, as it has the potential to allow more people to contribute to the workplace. One of the potential disadvantages of applying AI in the workplace is the risk of exploitative business practices. AI presents large corporations with the potential to develop service offerings for clients with the potential to eliminate whole teams. What AI cannot replace, however, is the human interventions that make our lived experiences unique. AI is a great enabler, but it is not the only solution. Habel: AI gives the construction industry vast opportunities to combat a legacy of productivity issues. Design processes have been made faster with the generative capabilities of AI through predictive design processes that introduce more feasible variations. This allows both designers and enabled designs to be optimised and executed to avoid repetitive mistakes. Through AI-enabled analytics, the decision-making process within the construction process has also been shortened, allowing for faster and more accurate decisions to be made. Michaela: The disadvantages of AI are more likely to be caused by an incorrect implementation rather than AI itself. This could include insufficient regulation leading to misuse, inability to remove bias from the dataset or insufficient investment in change management. It’s also important to consider the leaps in 46 Q. What do you envisage for an AI-powered construction industry in the future? Where and how do you see it being applied, and what benefits will AI deliver? A. Guilherme: I imagine that in the future AI will assist at all stages of construction, from planning and design to the maintenance of buildings. Projects designed by engineers with the best support from AI will be faster and of higher quality. Robots, 3D printers, and engineers wearing AR (augmented reality) glasses will speed up construction, while intelligent sensing systems will monitor structures. This will allow for more sustainable and safer projects. Siti: Although AI is not a time machine, it will provide us with the ability to look through archives and find examples of best practice. AI will help with continuous learning and will also free up workers by removing redundant tasks and allowing more time to solve problems and provide better tailored solutions that focus on the needs of the client. Habel: The possibilities are endless, but I envisage a productive industry. An industry where the design process is inclusive and interactive, and that is rich in data. It will also enable minimal waste generation as the concept of retrofitting and re-purposing of an assets is encompassed at 47 Q. What do you think the longer-term impacts of AI use could be on the world of work, and society more widely? A. Siti: The potential impact of AI technologies such as automation and voice activation, and the shift this will have on inclusivity is profound. Imagine the doors that would open for individuals who currently face barriers to using traditional technology. This innovation doesn’t just make life easier for the average user, it revolutionises the landscape for those who have been marginalised or left out of the workforce. A world of opportunity emerges for differently abled individuals to contribute their talents in ways that were previously inaccessible to them, ushering a new era of empowerment, where technology becomes the bridge to inclusion and a catalyst for a more diverse workforce. Guilherme: I believe that AI will bring more productivity and greater quality of life, but it will also require careful policies and continued training to manage human redundancies. It will be necessary to rethink income distribution to include both creative and technological jobs that can’t be replaced by machines. Michaela: It’s important we are comfortable not being able to foresee all potential impacts of AI. Technology has rapidly evolved over recent decades, and nobody was able to predict where developments would lead us. The is key is the ability to adapt fast. I see AI as a positive enabler to transform working patterns that can generate time for us to solve the big challenges of today such as sustainability, inclusion and change of lifestyle habits. AI is an enabler of transformation, not the driver. It’s up to us how we embrace it to see whether the impact will be positive or negative. Habel: It is imperative that we understand that AI, in whatever shape and form, is here to stay. If the advantages of AI come into fruition, the long-term impact will be extrapolated efficiencies across multiple markets. If deployed within ethical confines, AI has the potential to enrich human lifestyle and augment standards of living.