Lecture Notes: Variables, Distributions & Statistical Analysis PDF
Document Details
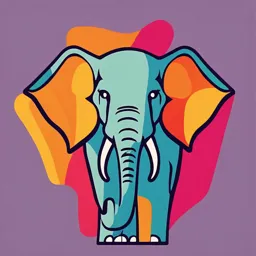
Uploaded by mackenzie
Tags
Summary
These lecture notes cover fundamental statistical concepts including variables, distributions, z-scores, t-tests, ANOVA, correlation, and regression. It discusses hypothesis testing, effect sizes, and various statistical methods for analyzing data. The notes also cover topics such as sampling distributions, confidence intervals, and the interpretation of statistical results.
Full Transcript
Lexicon un Mean The standard error of mean Formula: z X 0...
Lexicon un Mean The standard error of mean Formula: z X 0 Formula: s 0x or 84 M H X symbolizes average mean score symbolizes “sum of” Confidence intervals Deviation from the mean Formulas: 95 % (1 x = = 1. 96(0x) 68 % (1 x = = 1(0x) Formula: ~ XX range of values we are confident contains true men of population determines how far away score is from mean Single sample t-test Sum of squared deviations from the mean Formula: = EY M XM or Formula: (x(2 i s M describes total overall variability in set of scores Sampling Distribution of the Difference Between Means: Variance 2 Formula: Mx = N, My Formula: S 82 (X , - = y - or , m N average variability in set of scores SE of the Sampling Distribution of the Difference Between Means Standard Deviation t Formula: um SorO-E) - Formula: Sx , x , Si Recall that we don’t know the standard Calculating distances (or “deviations”) from deviation of the population (σ1 and σ2 ), so we the mean… must estimate it using the standard deviations of Standard deviation: average deviation from the samples (s1 and s2) the mean ↑ z- Score Pooled Variance: Average of both variances If samples have equal variances (which we Formula: Score X ear - assume), best estimate of the population Standard Deviation variance includes all the data Z-test formula: Sx , x Formula: M X , " g Where, SpXX , , xx n , 1 + (kz- Part 1.1: Variables and Distributions mini Variables Relative property of scales Variable: Anything that varies A At least 2 instances (AKA, “levels”) Often many/infinite levels (e.g., height in mm) Fruit, people, temperature, life (dead, alive), favorite food Quantifying Variables Measurement on ratio-level scales can always be “re- Quantification: Turning something into a number scaled” down to a lower level, but not up Allows for mathematical operations (calculations, e.g., performance in a race; temperature statistical analyses) Aim for ratio or interval scales so we can Quantify variables by assigning numbers to the mathematically manipulate data levels of a variable Scales of Measurement What Type of Variable is This? What is your favorite color? Four scales of measurement Nominal Nominal Scales How old are you? (in years) Used to classify attributes into categories Ratio “Nom” = Name Number of correct answers on a 10-item test? Arbitrary numbering scheme Ratio Not true quantification I like to gossip (1 = strongly disagree; 2 = disagree; 3 = Cannot be manipulated mathematically neutral; 4 = agree; 5 = strongly agree) Examples: Gender, Eye color, University Major Ordinal Ordinal Scales I like to gossip (-2 = strongly disagree; -1 = disagree; 0 = Rank according to amount of attribute neutral; +1 = agree; +2 = strongly agree) “Ord” = order Ordinal No info about degree/amount of difference I like to gossip (1 = True; 0 = false) between ranks/numbers Ratio Still cannot be mathematically manipulated Examples: Position in a race, Ranking of height, Percentile rank Variable Sets Interval Scales For any variable, we can have a set of instances (or Scale contains equal distances between units, but observations) no true zero point e.g., how much everyone in the course enjoys math “Interval” = equal intervals Can be manipulated mathematically (e.g., calculate Visualizing Variables (Percentages) averages) Pie Chart But cannot infer ratios (e.g., 30C is not twice as hot Nominal data we as 15C) Example: Temperature Visualizing Variables (Frequencies) Ratio Scales # of people choosing option on frequency Properties of interval scales, plus a true zero point distribution table Can manipulate mathematically, including ratios (dividing one score by another to compare Central Tendency proportion) Identifies single value that is most representatibe of entire Examples: Weight, Height, Speed, Distance, distribution Mode: Most popular - X Median: Middle score · X - Mean: Numeric average - Describe the following sets of happiness scores Degrees of Freedom (df) by referencing their means: Why do we divide by N – 1? A mean can be obtained from numerous possible J Which set of scores combinations of variable cases has the higher mean? When revealing the numbers in sequence, the ◦Green: 30/6=5 specific cases are “free to vary,” EXCEPT FOR THE ◦Blue:30/6=5 LAST CASE Thus, df = N – 1 Some other Important Features of Distributions In what way could you say the two sets of scores How many bumps? =J are different? Unimodal, bimodal, etc. ◦The blue set has a higher/more variability Symmetrical or asymmetrical? Skewness Positive vs. negative Comparing the Variable Sets Flat or pointy? Kurtosis Calculating Standard Deviation: Quantifying variability Platykurtic vs. leptokurtic Calculating distances (or “deviations”) from the mean… Standard deviation: average deviation from the mean formula: j Each Value – mean = Deviation from the mean Calculating squared deviations from the mean The Sum of the Squared Deviations: total overall variability in set of scores Square the deviation = Find the Sum Formula: Example: Part 1.2: Extremity and Probability were Z-Scores Z Table J Score Formula: w ear Standard Deviation Properties of z-scores: Scores equal to mean: z = 0 i s eie score lies exactly at mean of data set Scores above mean: z > 0 (positive number) re s e a a Scores below mean: z < 0 (negative number) meetene Scores far from mean: z = higher absolute value - Comparing Scores and Z-Scores Moving Beyond a Set of Scores Using a standardized norm-referenced score (i.e., z- Population: Considers all scores from a theoretically score) allows you to more easily compare scores across infinite set of scores variables that do not use a common metric. Refers to entire group of individuals or scores Using a standardized norm-referenced score (i.e., a z- relevant to the sample score) allows you to more easily compare scores across Allows for generalization within context of a much variables that do not use a common metric. broader group Practice Example Note: On an aptitude test, Andrea scored 245 on the nu Any single score can still be norm-referenced verbal scale and 175 on the mathematics scale. On (mean, sd), but now it will be referenced relative to which scale did she achieve a better result? the entire population. Verbal: mean = 220; st.dev = 50 z = XM2452288 5. - S 50 The Standard Normal Distribution Math: mean = 150; st.dev = 25 z = XM75158 Properties - nee S 25 Symmetrical: Left half of curve mirrors right half Mean = median = mode: All denoted as 0 Conclusion: She did better on the mathematics portion. - Unimodal: Single peak (mode) Even though her raw score was higher on the verbal scale compared to the math Scale, her standardized score was J HIGHER on the math scale. Z-Scores and Percentile Ranks Z-scores can also be stated with reference to their probability values Percentile rank: Indicates the number of scores in the distribution that fall below the observed score Probability Density Normal Curve: J Probability Density: Refers to how likely different outcomes are in a given distribution The properties of standard normal distribution allow us to make inferences about probability Since standard normal distribution has known properties (e.g., mean = 0, standard deviation = 1), we can use z-scores to determine probability of Practice Example observing a score within a certain range What percentile ranks did Andrea achieve on her z-table: Provides cumulative probability (area under the Verbal and Math scores, respectively? curve) for different z-scores Probability values associated with z-scores 65 % verbal : 2 0 5 =. Math : 1 = = 84 1 %. 1.3: Making Inferences seem Making Inferences Using z-Scores Usually, we don’t know the parameters of populations of Z-Scores can be used to determine the probability of interest… obtaining score x. A few exceptions: e.g., Standardized test scores Example: mu Often cannot calculate parameters directly Sally seems depressed, but you’re not sure. You ask Samples: A subset of the population (can vary in size) Sally to take a standardized depression test for which Can easily calculate descriptive statistics (mean, SD) the average score among clinically depressed people of a sample is 10, with a standard deviation of 3. Sally scores 4. Can use samples to make inferences about population parameters T J Inferential Statistics Null Hypothesis Testing (when parameters are known or assumed to be specific values): Deriving the probability of obtaining a sample with specific characteristics given what is known about the s e - population What is the likelihood (i.e., probability) that Sally is e.g., if mean IQ score of Acadia Students is 105, with clinically depressed? a standard deviation of 10, how likely am I to get a mean of 110 from a sample of 25 students? J Parameter Estimation (when parameters are not known): 002. Sampling from the population to estimate the parameters of that population i s e.g., sample of IQ scores among students at Acadia used to estimate the average IQ of all Acadia Students (pop.) Making Inferences about Populations Oftentimes we are more interested in populations of people (groups) than we are in individuals. Population = All members of a specific group Not everyone in a population is the same, but we can describe populations and make inferences i s about them. e.g., How intelligent are graduates of a specific school? e.g., How much does studying improve test scores? Population Parameters Parameters: Measurable characteristics of a population (mean, standard deviation) Greek letters used to symbolize these parameters. μ (mu) = mean of the population i σ (sigma) = standard deviation of the population s e 1.3.1: Null Hypothesis Testing nee Null Hypothesis Testing How Willing Am I to be Wrong? Z-scores tell us the likelihood of obtaining a score from a Alpha (α): Probability of making a Type I error w population Beta (β): Probability of making a Type II error u Infinite possible answers Null hypothesis testing entails asking, “Did this score Setting Alpha come from this population?” Alpha can be directly set by the researcher Two possible answers: Yes or No Typically, alpha is set to.05 Involves creating a (relatively arbitrary) cut-off point Only a 5% chance of making a type I error If the likelihood is less than the cut-off value, I will Critical Value: Point at which a score falls into region of say “no, too unlikely.” Otherwise, I will say “yes, rejection (i.e., exceeds alpha). seems probable.” Obtaining a score beyond this point merits rejection Often necessary for making behavioural decisions of H0 = If yes, then I will do Y, but if no, then I should do Z. Testing Hypotheses with Samples Core question in hypothesis testing: Was my sample ~ drawn from this population? Knowing what I know about the population, what is The Acadia Example the likelihood that I would get a sample mean of this Administration at Acadia suspect their graduates are value? smarter than the general population. To test the validity of If unlikely, conclude that must NOT have been this reasoning, they administer an IQ test to a random sample of 100 of their graduates. The sample mean is 102.5. NOTE: IQ drawn from this population w i tests are designed such that µ = 100 and σ = 15 Must come from a different population - Working backwards H0: The mean IQ score of Acadia Graduates is equal or Statistical hypotheses are tested by assuming they less than the mean of the general population. are false, and then showing that this assumption is Directional: ~ Acadia General pop very unlikely to be true. Non-Directional - Version: Acadia General pop H1: The mean IQ score of Acadia Graduates is higher than Operating under the Null Hypothesis the mean of the general population. Null hypothesis (H0): The mean of the population from Directional: ~ Acadia General pop which the sample is drawn from, is equal to the mean of Non-Directional mm Version: m Acadia / General pop the known population Extracting the details of the example: µsample = µknown Sample mean = 102.5 Alternative hypothesis (H1 sometimes Ha): The mean of µ = 100 the population from which the sample is drawn, from is σ = 15 not equal to the mean of the known population Z formula will not work here µsample ≠ µknown (i.e., not H0) Because now X represents a sample mean rather than a single score. Directional vs. Non-Directional Hypotheses It may not be realistic for one µ to be less than (or Sampling Distributions more than) the other µ. Theoretical distribution of sample meansfrom an infinite Directional Hypothesis Structure: number of same-sized samples from that population H0: The mean of the population from which the sample is ~ In other words, a distribution representing all possible drawn from, is less than or equal to the mean of the sample means (of size n) derived from a population. known population µsample ≤ µknown H1: The mean of the population from which the sample is - drawn from, is greater than the mean of the known population. µsample > µknown The Magic of Sampling Distributions The sampling distribution of the mean always approximates the normal distribution, even when the population is non-normal. Distribution becomes increasingly normal as the size of samples (N) within the sampling distribution increases The mean of the sampling distribution of the mean is always equal to the mean of the population. The standard deviation of the sampling distribution is always smaller than that of the population. NOTE: Standard deviation of the sampling distribution of the mean is referred to as the standard error of the mean. As N increases, the standard error decreases. Using Sampling Distributions to Determine Probabilities Judging how likely (or unlikely) a certain sample-mean is to have come from a population, involves comparing that sample-mean to the sampling distribution of the mean derived from that population. Sampling Distribution J raise of the Mean: - The Z-test Remember z-test formula: XMS Mx M). we g 0 Ox standard error of mean (SEM) u s e M or o Therefore: 21 M 2 X M - or i s G 62 H H Finding z-value for Acadia Example Sampling distribution of mean IQ scores (n=100) Finding the SEM: Finding the z-value: 0x 0 See = M 0x 15 & 108 0x = 15 = 1 5. Remember: Alpha is typically set at.05 use Critical Value for alpha =.05 Two-tailed test: z = 1.96 One-tailed test: z = 1.645 1.3.2: Parameter Estimation viii Parameter Estimation The Acadia Example Changing the nature of the question Assuming the administration wanted to estimate the Null Hypothesis Testing: Does this sample come from this mean IQ of all Acadia students, it could use its sample as population? (yes or no) an estimate Compare mean of sample to mean of population Sample mean = 102.5, Standard deviation = 15 (with known parameters) Standard error (N = 100) = 1.5 Parameter Estimation: What is the mean of the Calculating the Confidence Interval: population from which I drew my sample? Mean of the sample provides an estimate of the 95 % (1 X = 1. 96(0x) mean of the population = 102 5 11 96 (1 5)... = 102 512 94 Standard Error of the Mean.. = [99 56 103 44]. -. Error in the Estimate The mean of a sample will not always equal the mean of the population Sometimes overestimate, sometimes underestimate Our confidence in its accuracy depends upon the size of the sample Larger sample = lower standard error of the estimate Can have more confidence in parameter estimates that come from larger samples o or Confidence Intervals Mean of sample is our best estimate for the mean of the population, but we are not certain that it is correct/accurate Confidence Interval: A range of values that we are relatively confident contains the true parameter (i.e., true mean of the population) How confident do we want to be? 95 % (1 x : = 1. 96(0x)68 % C : X = /(0x) i s vie Part 2: t-tests 2.1: Single Sample t-test - - Beyond the z-test Single Sample t-test z-tests used for null hypothesis testing when population parameters are known (µ, σ) Formula: v = X M - - Example: IQ tests are standardized so µ = 100, σ = 15 ~ Sx in many real-world scenarios, we don't know the exact population parameters. Sx = symbol for the estimate of the standard error of the When we don't know the population standard sampling distribution of the mean deviation (σ), we can't directly use the z-test. T-test: Instead of using σ (the population standard deviation), we use the sample standard deviation, as an Sx = S Therefore + = X S M estimate for σ. M Example: The t-Distribution Are Students in This Class Getting Enough Sleep? Not the same as the standard normal, but approaches Doctors recommend that people get 8 hours of sleep the standard normal as df approaches ∞ per night. Sometimes this isn’t possible (i.e., it is Consequently, the probability densities of z-distribution variable), but do people get 8 hours on average? do not apply, which changes critical values for alpha Does µ = 8? We could test this, but we don’t know anything about A Closer Look at the Tail(s) the variability in sleep duration (σ is unknown). extreme values (outliers) are more likely under t- Let’s assume we randomly sampled 9 students in the distribution than under z-distribution, which accounts class to ask them how much sleep they got last night. for extra uncertainty when estimating population The mean of our sample was 6.5 (hours of sleep) with a standard deviation from the sample. standard deviation of 1. What is the probability that this sample comes from a t-Distribution vs. z-Distribution population whose mean is 8? The t-distribution has a shape similar to standard normal distribution, but it is wider and flatter— * 5-8 M 6 145 t especially with smaller sample sizes. = S n 9 J (h 1) - Critical- (t) value : t = - 4 55 , df = 8 -. Corresponding value at 95 % Cl -2 306 -. Instead of wanting to know (yes vs. no) if students are getting enough sleep, we could ask “How much sleep are students getting on average?” More open-ended question Sample mean = 6.5 Standard error (df = 8) =.333 Calculating the Confidence Interval ~ 95 % (1 * = = 1. 96(6y) = 6. 511 96) 333).. = 6 5. 0 652. = 5 848 7 152]. -. 2.2: Independent Samples t-test - So far Condition/Grouping Variable (AKA, independent variable We have looked at cases where we want to or IV) estimate the mean of a population (Parameter The variable that represents the different conditions Estimation) or derive the likelihood that a sample or groups comes from a population with a mean that is known/ Manipulated by researcher in an experimental assumed (Null Hypothesis Testing). design Single - sample z-test e.g., Hot vs. neutral temperature µ and σ are both known Test Variable (AKA, dependent variable or DV) Single ~ sample t-test The variable that is being compared across the µ is known (or can be assumed), but σ is unknown groups e.g., aggressiveness Estimating Effects Most often in psychology, we are less interested in the The Bad and the Ugly mean of a population and more interested in the effect of Extraneous Variables: Any variable (other than the IV) that one variable on another variable. could affect the outcome of the study (i.e., the DV). e.g., Does heat affect aggression? These are “bad” because they can create extra Still mean a population-level question variability in the DV. e.g., Is the average aggressiveness in the Extraneous variables are inevitable. Try to minimize population when it is hot different than the average and control them as much as possible aggressiveness in the population when it is not hot? Confounding Variables: Any variable other than the IV Experimental design: Involves manipulating variable of that differs between the conditions or groups interest (heat) IV is therefore not the ONLY difference between But we know nothing about the population means. groups ◦How can we proceed? Don't need to know These are “ugly” because they threaten the population mean integrity of the grouping variable and invalidate the study Was it the IV that influenced the DV, or was it the Logic of Testing for Effects confound? (Impossible to know) Collect ~ two samples from each population of interest Compare the means of the two samples Quantifying effect: Difference between means = Extraneous Variables estimate of the population effect Do not confuse extraneous variables with confounds! - Null Hypothesis Significance Testing Extraneous variables can become confounds, but ONLY if they are differentially represented in the - Question: “Do these samples come from populations with equal means?” levels of the independent variable All samples should be equal to zero e.g., gender can be a confound if higher proportion Underlying logic: “I think there is an effect of heat of men-to-women in one condition than the other(s) on aggression (H1), so I will assume there is no effect Controlling extraneous variables (H0) and calculate the likelihood of getting the Random assignment observed difference from two samples drawn from Measure extraneous variable (build it into the populations with equal means.” study) Two sample designs Study conducted to determine the likelihood that two samples were drawn from populations with equal means. Assumptions Involved in Doing Independent Pooled Variance: Average of both variances Samples t-tests If samples have equal variances (which we assume), Both groups are assumed to be drawn from populations in best estimate of the population variance includes all which the scores are normally distributed the data This makes less difference when N is high Homogeneity of variance Variances of the population(s) formula: - Sx , x from which the groups are drawn are assumed to be equal , " (same mean, same SD) This is an assumption for all between subject Where, SpXX , , xx designs n , 1 + (kz- Robust to violations when N is high or Ns are equal Homogeneity of variance can be tested (and Comparing Two Groups corrected, statistically) if violated. Levene’s test of equal variances (look for this in R output Determines if samples drawn from populations have equal whenever you have a between-subjects design) means Tests if variances are equal between population from Between-subjects visite which groups are drawn from Independent samples t-test Consider ~ the formula for a moment What would make the t-value bigger? Sampling Distribution of the Difference Between Smaller variance Means: Larger sample Formula: Larger differences between conditions Mx N, = My - e y - , What is the mean of this sampling distribution? Independent samples t-test formula: L. X , Xz r e s a e i - u s e Mean = difference between µ1 and µ2 What we n H0: Mean = 0 is the standard error ofe thisr samplinge distribution? - SS M, M2 Involves combining the error variance from each sample The variance sum law re s e a r The Parameter Estimation Approach The variance of the sampling distribution of the Null hypothesis approach: difference between means is equal to the sum of the Determines whether or not samples come from variances of the sampling distribution of the mean of populations with same mean each of the two populations Parameter Estimation Approach: (i.e., variance of SDM1 + SDM2) Determines the difference or true effect effect between means of the population from which samples are drawn from Variance Sum Law Can rearrange the t-formula to create a 95% Confidence SE of the Sampling Distribution of the Difference Between Interval for the true value of the difference us e m e re Means ~ Recall that we don’t know the standard deviation of Formula: 95 % (1 - X, - Y= t critical (Sx - 2) the population (σ1 and σ2 ), so we must estimate it , using the standard deviations of the samples (s1 and s2) Si formula: ~ SX , Xa 2.3: Effect Size and Power 2.4: Paired Samples t-test vin missi Estimating the Effect Size Types of Comparisons Effect size quantifies the magnitude of a treatment effect Between-subjects design or the difference between two conditions. Conditions are represented as different groups of Cohen’s d: Difference between conditions (i.e., the effect) participants. expressed in units of standard deviation Comparing the conditions involves a comparison between the groups. Formula: ↓ Difference Between Conditions , -* mu , d = Within-subjects design (AKA Repeated Measures) Pooled Standard Deviation Sp Same participants provide data under all conditions. Conditions are compared within each participant. How Big is a Big Effect Size? NO random assignment Cohen’s Rule of Thumb ◦Small effect = between.20 and.49 Within-Subjects Designs ◦Medium effect = between.50 and.79 Advantages - ◦Large effect = larger than.80 Reduced cost Increased statistical sensitivity Each participants serves as their own control, so Significance and Power Individual differences can be factored out Statistical Power: The probability of rejecting the null More sensitive to detecting real differences hypothesis when it is, in fact, false. Disadvantages A study with low power is likely to miss real effects - Carry-over effects: Can be overcome through counterbalancing Three Ways to Increase Power Increase Alpha Counterbalanced Within-Subjects Experiment However, this means increasing the likelihood of Random assignment to order of conditions making a Type I error. Increase Effect Size (i.e., difference between means) Comparing Sampling Distributions This is usually impossible. Between Subjects t-test (independent samples) The size of the effect represents something that is - Sampling dist. of the difference between means germane to the population. Mean = difference between µ1 and µ2 (which, under Increase Sample Size H0 = 0) This will reduce the std. error, thereby reducing Beta Std error = a summation of two standard errors i.e., variance sum law Calculating Power Within a - Subjects t-test (paired samples) Three major types of power calculation Sampling dist. of the mean-difference Post hoc: Given the observed effect size and the size of Mean = difference between µ1 and µ2 (which, under my sample, how much power did I have in my study? H0 = 0) A priori: Assuming a given effect size and specifying a Std error = the standard deviation of the difference decent amount of power, how many participants will I scores need in my study? Variability due to individual differences subtracted Sensitivity Analysis: Given the number of participants in out my sample, what sized effect can I expect to be able to Much smaller than the std error for the between- detect with a decent amount of power? subjects t-test standard error for mean difference (within subjects) Formula: vi Sp2 H Hypothesis Statements Essentially the same as for the between-subjects t-test H0: µ1 = µ2 H1: µ1 ≠ µ2 t-tests Paired samples t-test (within-subjects) Formula: t X , x2 = - um Sp2 H Parameter Estimation, Effect Size, and Power: Paired Samples t-test Parameter Estimation: 5 (1 ~. = X , -77 t critical (St) me Effect Size formula: Writing up results State hypothesis, type of test conducted, mention IV and DV Report t-statistic, p-value, and effect size: t(df) = #.##, p =.###, d =.## Interpret results with (minimal) reference to hypothesis. Part 3: Oneway ANOVA w Comparing More than Two Conditions How Can We Tell if There is a Difference Among What if we want to compare more than two conditions? the Conditions? e.g., 40c vs. 20c vs. 0c Reconsidering the t-test formula Why not do a series of t-tests? m u s t What does the numerator of this formula 40c vs. 20c represent? 40c vs. 0c ◦Variability between conditions 20c vs. 0c What does the denominator of the formula Family-wise error: Probability of making 1 or more Type I represent? errors in a family of comparisons ◦Variability within conditions Alpha is set at.05 for one test Which means 95% chance of correctly retain H0 This same basic ratio forms the basis of ANOVA, except Three tests means:.95 x.95 x.95 =.86 ratio is expressed in units of variance. Leaves a 14% change of making a Type I error!! Variance between the conditions (alpha =.14) ◦The difference among the condition means Variance within the conditions Analysis of Variance (ANOVA) ◦The dispersion among the participants within the Statistical procedure used to simultaneously conditions compare multiple conditions at a set level of alpha. It controls for the Type I error rate (which would The F-Distribution increase if you did multiple t-tests) helps determine whether the observed variation between Many types of ANOVA group means is large enough to be considered Factors: Number of independent variables within the statistically significant research design Characteristics: Levels (often symbolized as “k”): Number of categories Positively skewed within a factor (at least 2 levels) Variance can never be negative, so the F-statistic is One-Way ANOVA: Involves only one factor with at least 3 always positive (No negative values) levels All tests are one-tailed Simplest type of ANOVA Hypothesis Statements Under ANOVA Null Hypothesis v i i i H0: µ1 = µ2 = µ3… = µk (All µs are equal) The populations from which the samples were drawn have equal means Alternative visitin Hypothesis H1 = not all µs are equal (at least one mean is different from the others) The populations from which the samples were drawn do not have equal means ◦One or more of the samples was drawn from a distinct population 3.1: Between-Subjects Oneway ANOVA ~ A Visual Example Sums of Squares (SS) Calculations Distribution of 10 scores in 3 independent Total Variability: Represents overall variation in data, conditions regardless of the groups. It tells us how much each individual score deviates from the grand mean (the mean of all the scores, across all conditions). Sum of the squared differences between individual scores and grand mean. SST = [(X - Y )2 The Logic of ANOVA Within-Conditions Variability: Measures variation that Between Conditions Variability exists within each group or condition. This reflects random v i n variability in the means of the different conditions variability or error. Detects Real differences in the means of the Sum of the squared differences between individual populations from which the samples were drawn scores and their condition mean. If this variability is large, it suggests that there are real differences between the conditions Ssw = [(X Y)2 - Why are the condition means not all the same? v i n i s i Error Variability: Random variability associated with Between-Conditions Variability: Measures variation the specific samples (e.g., individual differences and between group means relative to the grand mean. Tells us other unknown factors how much group means differ from overall mean of all Within Conditions Variability scores, indicating the effect of treatment or factor you are wa s Why are the scores within a condition not all the same? testing. Reflective only of individual differences and other Sum of the squared differences between condition unknown factors (only Error Variability) means and grand mean. Comparing the 2 types of variability: sum of squared differences is multiplied by number If between-conditions variability is significantly of participants within each condition to account for larger than within-conditions variability, it suggests the fact that each condition-mean contains the weight that the differences between the conditions are real of n individuals. and not just due to random error. If the two variabilities are similar, it suggests that SSB = [R(x-* )2 the differences between groups are likely due to random chance, and there is no real treatment SST (Total Sum of Squares): Total variability in the data. effect. SST = SSB + SSW The F-Ratio Calculating the F-Ratio Formula for variance: ) SS Compares variance between the groups (explained mmmm gf(X-* variance) and variance within the groups (error variance) H -1 of Between Conditions Variance Variance is thus a sort of mean of the sum of (Explained variance + Error Variance F = squared deviations Within Conditions Variance (Error Variance A mean is a sum divided by n If H0 is correct: Explained variance = 0 ANOVA has a special term for variance: Mean ~ That is, there is no differences between the Squares (MS) conditions (i.e., group membership does not explain differences in scores) F-ratio = Approximately 1 If H0 is false: Explained variance > 0 F-ratio = >1 Effect Size for ANOVA A Priori Comparisons Unlike t-test, effect size cannot be reported in terms of Can often be used instead of doing ANOVA difference between conditions (Cohen’s d) But convention is to report omnibus ANOVA results, More than 2 conditions; difference between which and then report planned (i.e., a priori) comparisons. conditions? Note: “Omnibus” refers to the full ANOVA, which Instead, effect size is best reported as the compares difference of all conditions proportion of variance explained by the IV Some constraints ◦SSbetween + SSwithin Comparisons must honestly be planned in advance ◦Eta squared (η2) Should not conduct more than k – 1 comparisons ◦For example, if you have 5 groups (k=5), you would ideally only plan to make 4 comparisons. Now What? The F-Ratio only tells us that there is a difference among Computing A Priori Comparisons the means, but doesn’t tell us which means are different from which other means. Pooled variance for 3 conditions: Specific two-condition (pairwise) comparisons are needed (i.e., t-tests) ~MS Swithin (MSe) = Error of within Two strategies for probing a significant F: Y -z A priori comparisons Priori comparison formula: t = , use Used when you had a specific hypothesis MSe Me + - a priori means “from before” (prior) Post hoc comparisons Used when you did not have a specific hypothesis Post hoc means “after this” (post) Why Does it Matter? Recall i e ~ s the problem s of family-wise e error Multiple tests inflate the chances of making a type I error Posthoc tests involve doing numerous two- condition comparisons to determine which ones are different, and therefore must adjust alpha so that it does not exceed.05 A priori comparisons involve testing a specific expectation (thus requiring fewer tests), so there is no adjustment of alpha. Which approach is more likely to produce significant differences? Post Hoc Comparisons Many types of post hoc tests: Tukey’s, Bonferroni, Newman Keul’s, Scheffe, etc. Essentially, these tests vary in the amount that they adjust alpha In general, a higher number of comparisons means more adjustment to alpha Some tests adjust more or less, despite same number of comparisons (e.g., Bonferroni adjusts more than Tukey’s) There is no right and wrong type of post hoc test 3.2: Within-Subjects Oneway ANOVA ~ Within-Subjects ANOVA (Repeated Measures Sums of Squares for Oneway ANOVA: Within-Subjects ANOVA) Used when analyzing the effect of an within- SStotal = [(x-)2 subjects IV with 3 or more levels Each participant has data for all levels of the IV Sum of the squared differences between individual scores Essentially a comparison of 3 or more measures of and grand mean. [h (condition - ) participants on the same DV or construct. SSBetween = e.g., depression scores at pre-treatment vs. post- treatment vs. follow-up Sum of the squared differences between condition means e.g., memory for material that is studied vs. and grand mean, multiplied by the number of subjects/ semantically related to the studied material vs. not participants. SSSubjects [K (subject- studied material = Sum of squared differences between each subject’s mean Assumptions of Within Subjects ANOVA and the grand mean, multiplied by the number of Variances at each level of IV is normally distributed conditions. in the population SSResidual 2(X-[subject) (condition)? ◦Not overly important, especially when n is = - relatively high Sum of squared deviations between (the differences Sphericity: Variances of the differences between levels of between individual scores and subject’s mean score) and IV are equal (the difference between the condition mean that the variance of diff. between level 1 and level 2 = individual score falls within and the grand mean). variance of diff. between level 1 and level 3 = OR; SSresidual = SStotal – SSbetween – SSsubjects variance of diff. between level 2 and level 3. Important because violations produce an inflated F- ratio Calculating degrees of freedom Can test for violations using Mauchly’s test of dftotal (k)(n) = - 1 sphericity - If violation exists, can statistically correct for it etween · = k-1 (e.g., Greenhouse-Geisser adjustment in degrees of If subjects = H-1 freedom) re s e of residual = (K-1)(n-1) or aftotal of between ofsubject Sources of Variability - SST = SSB + SSW Within-Conditions Variability (SSwithin): Reflects inherent Calculating F variation, due to individual differences, randomness, and error term in within subjects anova is MSresidual unexplained factors SSsubjects: Variation due to individual differences can be F = MSBetween = SSbetween/af between pulled out in a within-subjects ANOVA MSResidual SsResidual/df residual SSresidual: Variation left over after extracting SSsubjects S (i.e., variability left unexplained) Between-Conditions Variability (SSbetween): Reflects the Effect Size for Within Subjects ANOVA variation attributable to treatment condition (explained) + inherent variation (unexplained) SsBetween Mp2 Between orbetween +Srelas Probing a Significant Effect The a priori method: Same as with between-subjects ANOVA, except now t-tests are paired-samples t-tests Once again, should not do more than k – 1 tests t = Y , - Xz S Where sD2 refers to variance of the difference between two means being compared. Can simply do a paired samples t-test with the two levels of the IV that you seek to compare. Part 3.3: Factorial ANOVA ~ Factorial ANOVA Main Effects vs. Interaction Effects Factor: An independent variable (IV) within the research Main Effects: Variance explained by a single factor, design irrespective (independent) of the other factor(s) in the Oneway ANOVA = one factor design design. Factorial ANOVA = design with two or more Within a 2x2, a main effect refers to the average factors difference between the two levels of one factor, when Levels: Number of different categories within the collapsing across the levels of the other factor. factor(s) Interaction Effects (AKA moderation): Variance explained Each factor contains two or more levels by a combination of factors. Notation ~ The effect of one factor on the effect of the other # levels within Factor1 # levels within Factor2 …. factor. e.g., 3 x 4 x 5 Difference among the levels of one factor depends Simplest factorial design = 2 x 2 (read “2 by 2”) on the level of the other factor(s). e.g., Effect of Factor A appears only in one level of Types of Factorial Designs Factor B Between Subjects Factorial Design When all IVs are between-subjects. The Many Different Looks of an Interaction Participants are randomly assigned to a single Attenuation: Effect of B (i.e., difference between B1 and combination of IV-levels. B2) is larger in A1 than in A2. J Within-Subjects Factorial Design When all IVs are within-subjects. Participants are represented in all of the conditions 7 (i.e., they have data for all levels of the IVs). Knockout: Effect of B is only present in A1. In A2 condition, Mixed Factorial Design effect of B is gone. When one or more IV is between, and one or more is within. Participants are randomly assigned to some conditions, but are represented in more than a single J condition (i.e., they have data for all levels of at least Reversal: Direction of effect of B changes across levels of one IV). Factor A. In A1, B1>B2, while in A2, B1